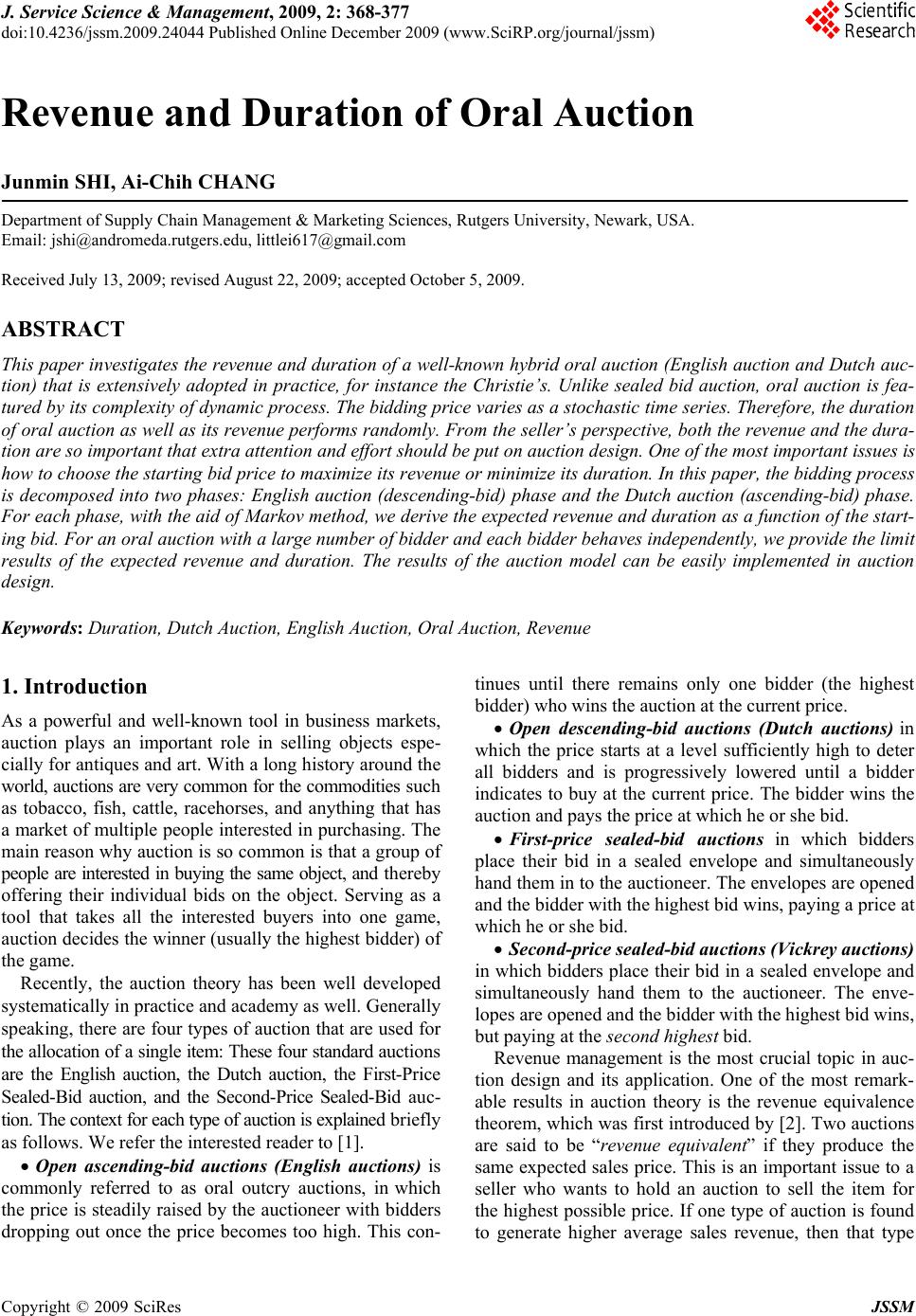 J. Service Science & Management, 2009, 2: 368-377 doi:10.4236/jssm.2009.24044 Published Online December 2009 (www.SciRP.org/journal/jssm) Copyright © 2009 SciRes JSSM Revenue and Duration of Oral Auction Junmin SHI, Ai-Chih CHANG Department of Supply Chain Management & Marketing Sciences, Rutgers University, Newark, USA. Email: jshi@andromeda.rutgers.edu, littlei617@gmail.com Received July 13, 2009; revised August 22, 2009; accepted October 5, 2009. ABSTRACT This paper in vestigates the revenue and duratio n of a well-known h ybrid oral auction (English auction and Dutch auc- tion) that is extensively adopted in practice, for instance the Christie’s. Unlike sealed bid auction, oral auction is fea- tured by its complexity of dynamic process. The bidding price varies as a stochastic time series. Therefore, the duration of oral auction as well as its revenue performs randomly. From the seller’s perspective, both the revenue and the dura- tion are so important that extra attention and effort should be put on auction design. One of the most important issues is how to choose the starting bid price to maximize its revenue or minimize its duration. In this paper, the bidding process is decomposed into two phases: English auction (descending-bid) phase and the Dutch auction (ascending-bid) phase. For each phase, with the aid of Markov method, we derive the expected revenue and duration as a function of the start- ing bid. For an oral auction with a large number of bidder and each bidder behaves independently, we provide the limit results of the expected revenue and duration. The results of the auction model can be easily implemented in auction design. Keywords: Duration, Dutch Auction, English Auction, Oral Auction, Revenue 1. Introduction As a powerful and well-known tool in business markets, auction plays an important role in selling objects espe- cially for antiques and art. With a long history around the world, auctions are very common for the commodities su ch as tobacco, fish, cattle, racehorses, and anything that has a market of multiple people interested in purchasing. Th e main reason why auction is so common is that a group of people are interested in buying the same object, and t her eb y offering their individual bids on the object. Serving as a tool that takes all the interested buyers into one game, auction decides the winner (usually the highest bidder) of the game. Recently, the auction theory has been well developed systematically in practice and academy as well. Generally speaking , there are four typ es of auction that are used for the alloca tion of a single item: Th ese four standard au cti o n s are the English auction, the Dutch auction, the First-Price Sealed-Bid auction, and the Second-Price Sealed-Bid auc- tion. The context for each type of auction is explained briefl y as follows. We refer the interested reader to [1]. Open ascending-bid auctions (English auctions) is commonly referred to as oral outcry auctions, in which the price is steadily raised by the auctioneer with bidders dropping out once the price becomes too high. This con- tinues until there remains only one bidder (the highest bidder) who wins the auction at the current price. Open descending-bid auctions (Dutch auctions) in which the price starts at a level sufficiently high to deter all bidders and is progressively lowered until a bidder indicates to buy at the current price. The bidder wins the auction and pays the price at which he or she bid. First-price sealed-bid auctions in which bidders place their bid in a sealed envelope and simultaneously hand them in to the auctioneer. The envelopes are opened and the bidder with the highest bid wins, paying a price at which he or sh e bid . Second-price sealed-bid auctions (Vickrey auctions) in which bidders place their bid in a sealed envelope and simultaneously hand them to the auctioneer. The enve- lopes are opened an d the bidder with t he highest bid wins, but paying at the second highest bid. Revenue management is the most crucial topic in auc- tion design and its application. One of the most remark- able results in auction theory is the revenue equivalence theorem, which was first introduced by [2]. Two auctions are said to be “revenue equivalent” if they produce the same expected sales price. This is an important issue to a seller who wants to hold an auction to sell the item for the highest possib le price. If one type of auction is foun d to generate higher average sales revenue, then that type
 Revenue and Duration of Oral Auction369 auction will obviously be preferred by the sellers. In other words, the revenue equivalence theorem states that, if all bidders are risk-neutral bidder and have independent pri- vate value for the auctioned items, then all four of the standard single unit auctions have the same expected sales price (or seller’s revenue). To analyze and formulate a dynamic auction, most lit- erature adopts the stochastic process approach. For ex- ample, an online auctions problem is studied by [3] and they design a (1-1/e) competitive (optimal) algorithm for the online auction problem. Vu lcano, G. et a l. analyze a dynamic auction, in which a seller with C units to sell faces a sequence of buyers separated into T time periods [4]. They assume each group of buyers has independent, private values for a single unit. Buyers compete directly against each other within a period, as in a traditional auc- tion. For this setting, they prove that dynamic variants of the first-price and second-price auction mechanisms maxi- mize the seller's expected revenue. E. J. Pinker, et al. analyze the current state of management science research on on lin e auctions [5]. They develop a broad research agenda for issues such as the b ehavior of online auction participants, the optimal design of online auctions, and so on. Most recently, Z. J. Shen and X. Su provide a detailed and up-to-date review of customer behavior in the revenue management and auction literatures and suggest several future research directions [6] . In the auction study, an increasing number of empirical studies apply a structural econometric approach within the theoretical framework of the independent private values model and the common value model [7,8]. In common value auctions, a bidder's value of an item depends en- tirely on other bidders' values of it. By contrast, in private value auctions, the value of the good depends only on the bidder's own preferences. In auction design, efficiency and optimality are the two primary goals: the former fo- cuses on the social welfare of the whole seller-bidder system, whereas the latter emphasizes the revenue-maximizing from the seller’s perspective [9]. Markov theory was developed by the Russian mathe- matician A. A. Markov. The theory provid es a foun da tion for modeling a stochastic process whose future state de- pends solely on its current state and is completely inde- pendent of its past states. This property is well known as memorylessness [10]. Markov process has been wildly applied to model the auction process. For example, S. Park, et al. devise a new strategy that an agent can use to determine its bid price based on a more tractable Markov chain model of the auction process [11]. They show that this strategy is particularly effective in a “seller’s market”. A. Segev, et al. model an online auction in terms of a Markov process on a state space defined by the current price of the auctioned item and the number of bidders that were previously “bumped” [12]. They first convert an online auction into a small-to-medium sized auction. Then the transition probability matrix of states is derived and the price trajectory of the small-scale Markov proc- ess is obtained. Finally, the final price prediction can be determined based on the obtained transition probability matrix. Duration of auction is another factor under considera- tion in auction design. D. Reiley, et al. show that the length of the auction positively influences the auction price [13]. To the best of our knowledge, except the aforementioned reference, there are very few literatures considering the duration of auction. At this point, one effort of this paper is to bridge the gap via deriving the duration as a function of the starting bid. Unlike sealed bid auction, oral auction is featured by its dynamic complexity of the bidding process. In practice, oral auction is more widely preferred than sealed bid auction. For instance, Christie's has au ctioned off artwork and personal possessions mostly via oral auctions [14]. Christie's was founded in London, England, on 5th December 1766 by James Christie. Christie's soon established a repu- tation as a leading auction house, and took advantage of London's new found status as the major centre of the international art trade after the French Revo lution. Christ ie's has held the greater market share against its longtime rival, Sotheby's, for several years and is currently the world's largest auction house by revenues. In addition to Christie's, a variety of world famous auction organizations adopt oral auction in their business. The bidding process of the oral auction under study is explained as follows. The auctioneer begins the auction with an announced starting bid. This bid is referred to as the starting bid. Then the auctioneer will ask the bidders for their response by open cries. If nobody responses for the bid, then the auctioneer announce “Going once” for a short while. If there is still no response from bidders, then “going twice” is announced for another short while. If no response again, then the auctioneer deduces the bid, and ask the bidder for their response. The similar process continues until there is bidder responding to the revised bid. Such bid-deceasing phase will be stopped since all bidders shall response to a revised bid while it gets low enough. Once a bidder response to a revised bid, the auc- tioneer increases the bid and asks for the response with “going once, going twice” as aforementioned crying out process. The process proceeds and stops until there is no response within the sequential announcement of “going once, going twice and gone”. In other words, once no bidder is willing to raise the revised bid, the object is “hammered down”, and the last bidder (with the highest bid) wins the auction. From the bidding process described above, we can see the oral auction is composed of two phase: descend- ing-bid (Dutch auction) and ascending-bid (English auc- tion), and thereby the oral auction is referred to as a hy- brid auction. For the hybrid oral auction and from the Copyright © 2009 SciRes JSSM
 Revenue and Duration of Oral Auction Copyright © 2009 SciRes JSSM 370 practice point of view, the final bid and the total time spent on bidding are two important factors investigated in auction design. The final bid of the auction is referred to as the revenue and the total time of bidding is referred to as the duration. Each of them can be employed to evalu- ate the efficiency of the auction. Maximum revenue or minimum duration can be obtained via choosing an opti- mal starting bid. In this paper, it is of our interest to de- rive the expected revenue and duration as a function of the starting bid. To this end, we shall first formulate an oral auction model based on Markovian property. Then we decompose the hybrid oral auction into two phases: descending-bid phase and ascending-bid phase. For each phase, we derive the Markov transition matrixes which are referred to as the downward for the descending-bid phase and upward matrix for the ascending-bid phase. With the aid of Markov approach, we finally obtain the revenue and duration of the auction as a function of the starting bid . where and . Let and F denote the starting bid and the final bid, respectively. Let B denote the lowest bid level over the bidding process, where and B. For each bid, let T denote the whole period length of announcement by the auc- tioneer (briefly referred to as the announcement period for each bid). Let denote the number of responds to the bid from bidders within announcement period . In the descending phase, if for bid , then the auctioneer will decrease the bid, otherwise, the descending-bid phase will stop and proceed to the as- cending-bid phase. In the ascending phase, if for bid , the auctioneer will increase the bid, otherwi se, the auction will end with the final bid . Figure 1 depicts a sample path of the oral auction bidding process. 00P= B£ i P i P 1ii PP + < F£ () i NT S ()= S T0 i NT F = i P 0>() i NT i P From the auction process described above, we have some following conclusions. This paper makes the following contributions. First, it presents an exploratory analysis of the hybrid auction and obtains a closed form expressions for the auction revenue and duration. Secondly, for a large group of bidders, the limit performance has been analyzed. These results can be applied directly in p ractice as an aid in auction design . 1) All the items could be auctioned off since the bid- ders are willing to take the au ction at a low enough pr ice, say . The refore, the final bid of the auction is at least . 1 P1 P 2) Within the bidding process, there are two bidding phase: descending and ascending. In the descending phase, bidding price decreases from start price to the lowest bid . In the ascending phase, bidding price increases from the lowest bid to the final bid . S F B B The remaining of this paper is organized as follows. The model of oral auction is formulated in § 2. The ex- pected revenue is derived in § 3, and the duration is de- rived in § 4. Finally, § 5 concludes the paper. The random variable corresponding to bid governs the bidding process. If , namely, () i NT i P0() i NT> 2. Model Formulation Let the di scre te bi d level s be denote d by 0,1,2,3,... :} i Pi=, there are some bidders willing to take the auction with bid , then the au c tione er w ill rev is e the bid and incre ase i P 05 10 15 0 20 40 60 80 100 120 Time Bidding Price Bidding Process Ending Price Starting Price Figure 1. A sample path of the bidding process
 Revenue and Duration of Oral Auction 371 from to . Let i P1i P+ 0Pr{( )} i NT q== i (1) then, 01Pr{( )} ii NT q>=- (2) Since each is given and constant, the transition probability from to or is solely de- i q i P1i P+1i P- termined by . It implies that the bidding process is a Markovian. i q From previous discussion, we can see there are three typical processes which are possibly incurred in practice. Case 1. Descending-bid (Dutch auction) In the descending-bid process, the bidding is monoto- nously decreasing from starting price to final bid F. Figure 2 depicts a sample path of such process. S Case 2. Ascending-bid (English auction ) In the ascending-bid process, the bidding is monoto- nously increasing from starting price to final bid . Figure 3 depicts a sample path of such process. S F 00.5 11.5 22.5 33.5 44.55 0 5 10 15 20 25 30 Time Bidding Price Biddi ng Proces s Ending Price Starting Price Figure 2. A sample path of the descending-bid proce ss 00.5 11.5 22.5 33.544.5 5 20 30 40 50 60 70 80 90 100 110 Time Bidding Price Biddi n g Process Start ing P rice Ending Price Figure 3. Ascending-bid proce ss Copyright © 2009 SciRes JSSM
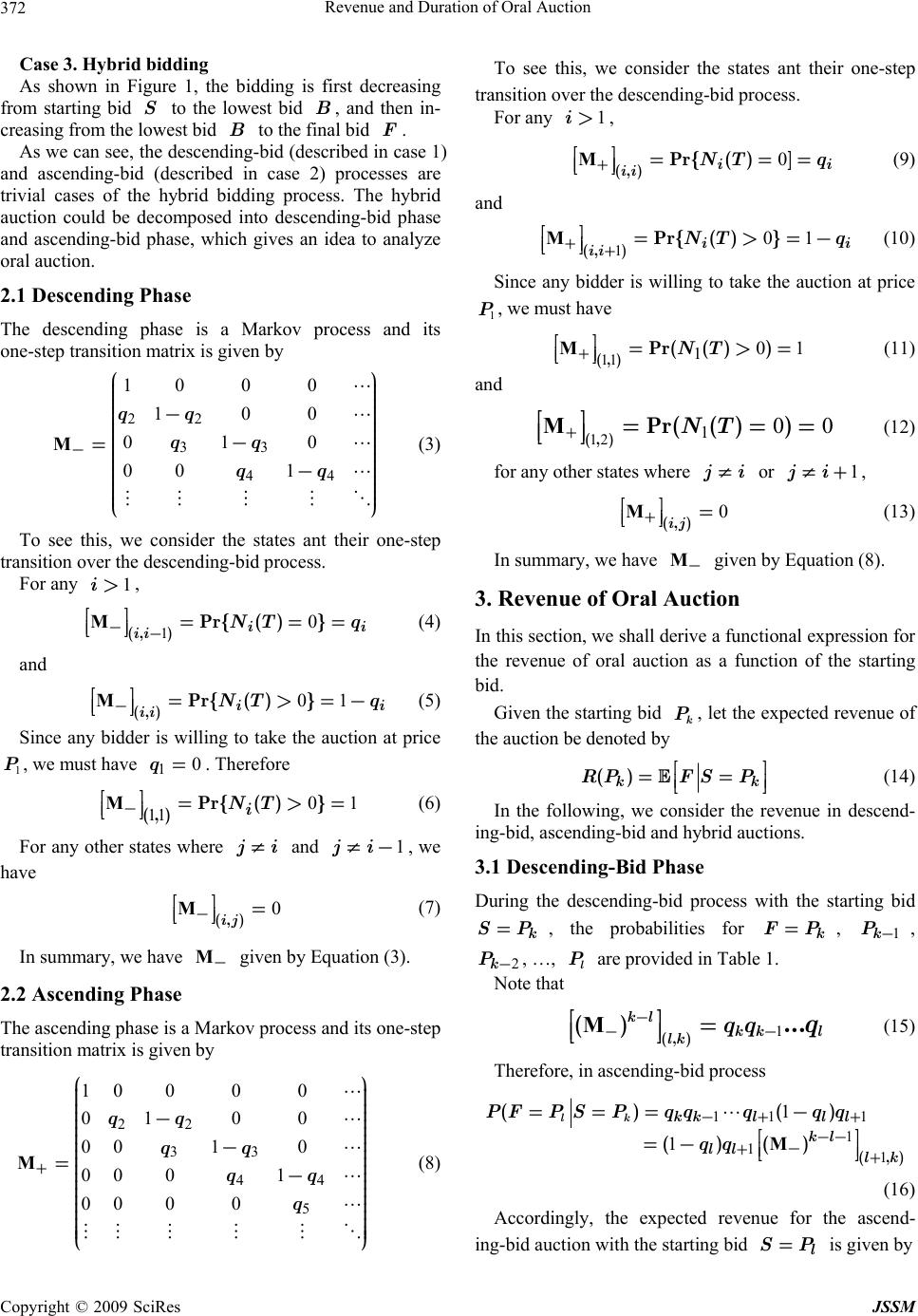 Revenue and Duration of Oral Auction 372 Case 3. Hybrid bidding As shown in Figure 1, the bidding is first decreasing from starting bid to the lowest bid , and then in- creasing from the lowest bid to t he final bid . S B B F As we can see, the descending-bid (described in case 1) and ascending-bid (described in case 2) processes are trivial cases of the hybrid bidding process. The hybrid auction could be decomposed into descending-bid phase and ascending-bid phase, which gives an idea to analyze oral auction. 2.1 Descending Phase The descending phase is a Markov process and its one-step transition matrix is given by 22 33 44 1000 100 010 00 1 M qq qq qq - æö ç÷ ç÷ ç÷ ç÷ - ç÷ ç÷ ç÷ - ç÷ =ç÷ ç ç - ç ç ç÷ ç÷ ç÷ èø ÷ ÷ ÷ ÷ = i - 1 = (3) To see this, we consider the states ant their one-step transition over the descending-bid process. For any , 1i> 10M(, )Pr{( )} ii ii NT q -- éù == êú ëû (4) and 01M(,) Pr{( )} i ii NT q - éù=>= êú ëû (5) Since any bidder is willing to take the auction at price , we must have . Therefore 1 P10q= 11 0M(,) Pr{( )} i NT - éù=> êú ëû (6) For any other states where i¹ and 1 i¹-, we have 0M(, )ij - éù= êú ëû (7) In summary, we have given by Equation (3 ). M- 2.2 Ascending Phase The ascending phase is a Markov process and its one-step transition matrix is given by 22 33 44 5 10 000 010 0 001 0 00 01 00 00 M qq qq qq q + æö ç÷ ç÷ ç÷ ç÷ - ç÷ ç÷ ç÷ - ç÷ ç÷ =ç÷ ç÷ - ç÷ ç÷ ç÷ ç ç ç ç ç ç è To see this, we consider the states ant their one-step transition over the descending-bid process. For any , 1i> 0M(,) Pr{()] ii ii NT q + ù== êú = û (9) and 101M(, )Pr{( )} ii ii NT q ++ ù=>= êú - û (10) Since any bidder is willing to take the auction at price , we must have 1 P 11 10M(,) Pr(( ))NT +1 ù=> êú = û (11) and 12 10 (, )Pr(( ))NT +0 ù== êú = û M (12) for any other states where i¹ or 1 i¹+, 0M(, )ij + ù= êú û (13) In summary, we have given by Equation (8 ). M- 3. Revenue of Oral Auction In this section, we shall derive a functional expression for the revenue of oral auction as a function of the starting bid. Given the starting bid , let the expected revenue of the auction be denoted by k P () k RPFS P k ù == ú û (14) In the following, we consider the revenue in descend- ing-bid, ascending-bid and hybrid auctions. 3.1 Descending-Bid Phase During the descending-bid process with the starting bid , the probabilities for , , , …, are provided in Table 1. k SP= k P- k FP=1k P- 2l Note that P 1 (, ) () ... kl kk l lk qq q - -- ù= êú û M (15) Therefore, in ascending-bid process ÷ ÷ ÷ ÷ ÷ ÷ ø (8) 11 1 1 11 1 1M (,) () () ()() lkkkll l kl ll lk PFPSPqqqq q qq -+ + -- - ++ === - éù =- êú ëû (16) Accordingly, the expected revenue for the ascend- ing-bid auction with the starting bid is given by l SP= Copyright © 2009 SciRes JSSM
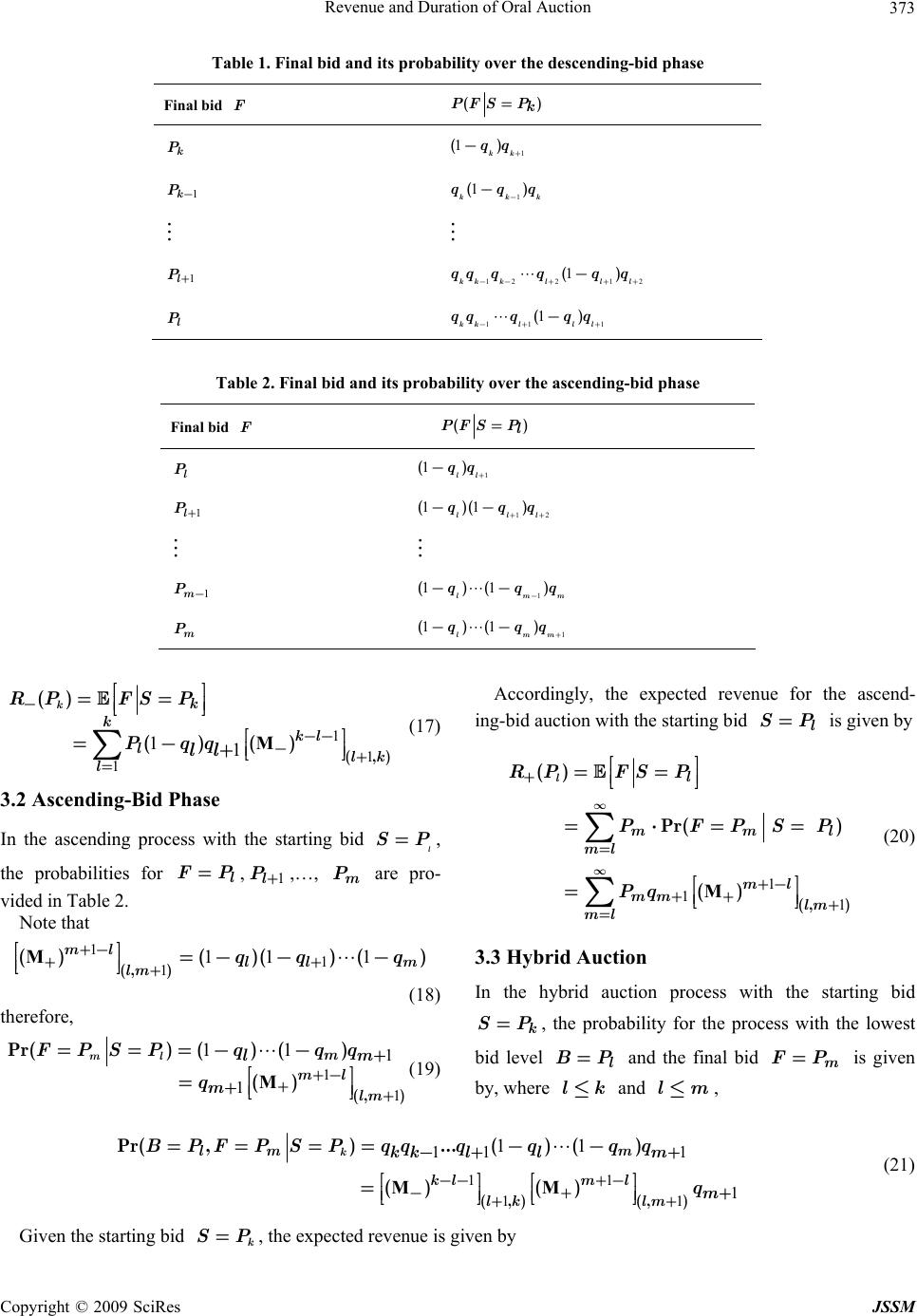 Revenue and Duration of Oral Auction373 Table 1. Final bid and its probability over the descending-bid phase Final bid F()PFS P k = k P 1 1() kk qq + - 1k P- 1 1() kk qqq - -k 1+ 1l P+ 1221 2 1() kk klll qq qqqq --+++ - l P 11 1() kkll l qqqq q -+ - Table 2. Final bid and its probability over the ascending-bid phase Final bid F()PFS P l = l P 1 1() ll qq + - 1l P+ 12 11()( ) lll qqq ++ -- 1m P- 1 11()( ) lm qq - -- m q m m P 1 11()( ) lm qqq + -- 1 1 1 11M(, () ()() kk k kl llk l RPFSP Pqq ll - -- -+ = éù == êú ëû éù =- +êú ëû å ) ) (17) 3.2 Ascending-Bid Phase In the ascending process with the starting bid , the probabilities for ,,…, are pro- vided in Table 2. l SP= l FP=1l P+m P Note that 11 111 1M (, ) ()( )()( ml m ll lm qq q +- ++ + éù =- -- êú ëû (18) therefore, 1 1 1 1 11 M (, ) Pr()() () () ml m ml lm l m FPSP qqq q+- ++ + + ===-- éù =êú ëû Accordingly, the expected revenue for the ascend- ing-bid auction with the starting bid is given by l SP= 1 11 M(, ) () Pr( ) () ll mm ml ml mm lm ml RPFSP PFPSP Pq ¥ ¥ + = +- ++ + = éù == êú ëû =⋅== éù =êú ëû å å l (20) 3.3 Hybrid Auction m (19) In the hybrid auction process with the starting bid , the probability for the process with the lowest bid level and the final bid is given by, where and l, k SP= l BP= lk£ m FP= m£ 11 11 11 1 1 11 MM (,)(,) Pr(,)...() () () () kmm l klm l lk lm kk llm m BPFPSP qqqqqq q -- +- -+ ++ -+ + + == ==-- éùé ù =êúê ú ûë û (21) Given the starting bid , the expected revenue is given by k SP= Copyright © 2009 SciRes JSSM
 Revenue and Duration of Oral Auction 374 1 1 1 1 1 1 1 1 MM MM (, )(,) (, )(,) () , () () () () l k lml k lml m m k klm l m lk lm klm l mlk lm kk RPFS PFS PBP Pq Pq ¥ -+ == ¥ -+ == + + - + -+- + +- ù éùéù ===== êú êúêú ëûëû ëû éùé ù =êúê ú ëûë û éùé ù =êúê ú ëûë û åå åå (22) 3.4 An Example of Auction with a Large Number of Bidders In this subsection, we consider an example of oral auc- tion where there are a large number of potential bidders. We assume that each bidder responds to the bid inde- pendently and the probability of responding to bid i P over the announcement period is small. To begin with, we give a limit theory as follows. Lemma 1: Let X be a binomial random variable with parameters , then approached to Pois- son random variable with parameter as n gets large and gets small. (,np)X np= l p Proof: since is binomial, we have X 1 11 1 11 11 ! Pr()( ) !( )! ()() ()() ! ()() ()/( ! ini ini ini i n Xip p ini nnn i inn nnn i in n n - - == - ⋅- --+ =- --+ =- ll ll l )- Then, for a large enough and a small enough , we have the following limits, n p 11 1 ()( ) lim i n nnni n ¥ --+ =, 1lim ()n ne n - ¥ -= l l, 11lim ()i nn ¥ -= l. Finally, Pr() ! i Xi e i - == l l. (Q.E.D) In view of Lemma 1, for a large and small , the number of responding bidders within a unit time in- terval is a Poisson random variable with arrival rate . It follows that the number of responding bidders within the announcement period T, follows a Poisson distribution, that is . Since , therefore . Let further , then ni p i np=⋅li i () i NT () i n Tl ( ) ii nnpT Po Po ) pp -a ) i isso isso ) ii i np=⋅l nT =a N Pr = Pr(( ) i NT ()NT~ ( ii sson pa 0ex) (== exp(p--a ()T (qN Poi~ (23) Accordingly, ( ) iT (24) and . Substituting Equation (24) into Equation (21), we have 01)>= 1 1 exp 1 Pr( ,) (ln( exp())) km l km ij il jl m BPFPSP pp =+ = + == = =- -+-- åå aa a p j (25) Substituting Equation (25) into Equation (22), we finally have 11 1 exp 1()(ln(exp())) k m lml km ki il jl m RPP ppp ¥ == =+ = + =--+-- ååå å aa a (26) Figure 4 depicts the sketch of the functional relation- ship between the expected revenue and the starting bid. Graphically, the peak point in the curve represents the optimal starting bid as well as its corresponding expected revenue. 4. Duration of Oral Auction In this section, we consider the expected length of the oral auction. In practice, each bid is announced for at most 3 times. For each bid, let , and denote 1 T2 T3 T Copyright © 2009 SciRes JSSM
 Revenue and Duration of Oral Auction375 050 100150200 250 300 350 40 0 5 10 15 20 25 30 35 40 45 Starting Price Revenue Revenue vs Starting Price Optimal Revenue Figure 4. Revenue vs starting bid 05 10 1520 25 30 35 2 4 6 8 10 12 14 16 18 Time Bid din g p ri c e Time Series of Bidding Starting Ending Figure 5. Time evolution of a bidding process the time length of the periods between the announce- ments of the bid beginning, “going once” “going twice” and “gone”, respectively. Let . For example, when the auctioneer announces the bid , if there is no response up to , that is , then 12 TTTT=++ P 10() i NT = 3 2 3 i 1 T the auctioneer announces “going once”; if there is still no response up to , that is then “going twice” will be announce; if there is no re- sponse up to , that is , then “gone” is announced. Figure 5 depicts a sample path of the time evolution of the bidding process in term of a step function. 1 TT+ 12 TT++ 120() i NT T+= 0() i NT=T Copyright © 2009 SciRes JSSM
 Revenue and Duration of Oral Auction 376 Let denote the time length for bid , where can take value of , , or . For any bid , let denote the probability of there is no response during period XiP i 12 TT+Xi1 T ,ij 1 TT+2 3 T+ P iq T , where 12 3,, = = , that is 1 ( i =0 ,P() ) ij qNT (27) Lemma 2. For any bi d , the following holds P i 11121212 0 11 1 ,,,,, [|() ] ()()( )( iiiii i ii XNT TqTTqqTqqq q > -++- +- =- 3 1 , ) i (28) Proof. The conditional probabilities are given as 1 1 11 0 001 , Pr(( )) P(|( ))Pr(( )) i i i ii i q NT XTNT NT q - > =>= = >- 12 12 12 00 00 1 1 ,, Pr(( ))Pr(()) P(|( ))Pr(( )) () ii i ii ii i NT NT XTTNT NT qq q => =+ >=> - =- and 12 3 123 123 0 00 0 1 1 ,, , P(|( )) Pr( ())Pr( ())Pr( ()) Pr(( )) () ii i i ii ii i i XTTTNT NT NTNT NT qq q q =++> == => - =- 0> m X X Then, the proof is completed by the definition of con- ditional expectation. (Q.E.D) Let denote the time length of the bidding process with the starting bid , the lowest bid and the final bid . Thereby kl m T l P k SP= m P=B=F 1 1 lm ij kl m ik jl TXX - + == =++ åå (29) Note that there is no response for any bid along the descending-bid process. Then, Equation (29) can be sim- plified as 1() m j kl m jl TklT = =-++ å (30) Therefore, the duration for the auction with starting bid is, k P (),, m kk kl m DPTSPBPFP é é === ê ê ë ë 1 1 1 1 10 10 M(, () ,, () (),, Pr( ,) () [|()] () m l m l k m BP FPjk jl m BPFPjj jl mk ml jll m j jl kl lk j klTXSPBF kl TXNTBF BPFPSP kl TXNT == = == = == = -- -+ ù ù êú êú =-++= êú êú êú êú ëû ëû ù éù ú êú =-++ > ú êú ú êú ëû û ==== éù êú -+ +> êú êú ëû éù =êú ëû å å åå å 1 1 11 10 M )(,) () () [|()] mk ml lm jll m j jl m j q kl TXNT +- ++ == = + éù êú ëû éù êú -+ +> êú êú û åå å (31) l ù ù = ú ú û û where is given by Equation (28). There, the first equation above holds by the definition and the conditional expectation. The second equation holds by Equation (30). The third equation holds follow- 0[|() jj XNT>] Copyright © 2009 SciRes JSSM
 Revenue and Duration of Oral Auction377 ing the definition of conditional expectation. The last equation follows Equation (21). 5. Conclusions and Discussions This paper studies the revenue and duration of an oral auction, which has a hybrid structure of English auction and Dutch auction. Our effort is to derive the revenue and duration of the auction as a function of the starting bid. To this end, we decomposed the bidding process into two phases: English auction (descending-bid) phase and the Dutch auction (ascending-bid) phase. For each phase, we first gave the one-step transition matrix and the formula for revenue and duration are obtained consequently. For an oral auction with a large number of bidder and each bidder behaves independently, we also derived the limit results of the expected revenue and duration. The results obtained can be implemented in practice directly. In particular, the probability of bidder respond- ing to a bid can be statistically estimated from the ob- served data. Therefore, the one-step Markov transition matrix can be computed directly. The one-step transition matrix for each phase can be used to compute the ex- pected revenue and duration. From the seller’s perspec- tive, the optimal starting bid is of great interest and it can be obtained numerically by some basic searching algo- rithm. With the formula for revenue and duration, we may take their ratio to evaluate the efficiency of the oral auction. This ratio accounts for the revenue as well as the time, and thereby provides a comprehensive evaluation. Our model is formulated based on Markov assumption, that is, the bidder behaves only according to a function of the bid level. It does not depend on th e bidder’s previous behavior as well as the other bidders’ behavior. Although Markov process models provide a mathematical approach to predict online auction prices, estimating parameters of a Markov process model in practice is a challenging task. For example, S. Chou, et al. propose a simulation-based model as an alternative approach to predict the final price in online auctions [15]. To study the oral auction with bidder inter-dependent behavior, we can extend our model to a multi-space Markov model, in which each state space represents the bidding price for each bidder. This leads to a new topic of further research. It is commonly assumed that the customer behavior is exogenous. For example, market size is often represented using a demand distribution (e.g., the newsvendor model). However, in our real world of oral auction, all bidders do, at some point, actively evaluate alternatives and make choices. This suggests that bidders’ decision is jointly effected together. Thereby, “customer behavior” should be introduced to auction design. In our view, it is impor- tant to adopt a micro-perspective on such biding interac- tions. This requires a high-resolution lens to zoom in on the incentives and decision processes of bidders at their individual level. REFERENCES [1] C. W. Smith, “Auctions: The social construction of value,” University of California Press, Berkeley, Los Angeles, 1990. [2] W. Vickrey, “Counter speculation, auctions, and competi- tive sealed tenders,” Journal of Finance, Vol. 16, pp. 8–37, 1961. [3] N. Buchbinder, K. Jain, and J. Naor, “Online primal-dual algorithms for maximizing ad-auctions revenue,” Lecture Notes in Computer Science, 4698, pp. 253–264, 2007. [4] G. Vulcano, G. V. Ryzin, and C . M aglaras , “O ptimal dynamic auctions for revenue management,” Management Science, Vol. 48, No. 11, pp. 1388–1407, 2002. [5] E. J. Pinker, A. Seidmann, and Y. Vakrat, “Managing on- line auctions: Current business and research issues,” Ma n a g e- ment Science, Vol. 49, No. 11, pp. 1457–1484, 2003. [6] Z. J. Shen and X. Su, “Customer behavior modeling in revenue management and auctions: A review and new re- search opportunities,” Production and Operations Man- agement, Vol. 16, No. 6, pp. 713–728, 2009. [7] K. Hendricks and H. J. Paarsch, “A survey of recent em- pirical work concerning auctions,” Canadian Journal of Economics, Vol. 28, No. 2, pp. 403–426, 1995. [8] J. Laffont, “Game theory and empirical economics: The case of auction data,” European Economic Review, Vol. 41, No. 1, pp. 1–35, 1997. [9] R. L. Zhan and Z. J. Shen, “Optimality and efficiency in auctions design: A survey,” Working Paper, 2006. [10] S. M. Ross, Stochastic Processes, 2nd Edition, Wiley, 1995. [11] S. Park, E. H. Durfee, and W. P. Birmingham, “Use of Markov chains to design an agent bidding strategy for continuous double auctions,” Journal of Artificial Intelli- gence Research, Vol. 22, pp.175–214, 2004. [12] A. Segev, C. Beam, and J. Shanthikumar, “Optimal de- sign of internet-based auctions,” Information Technology and Management, Vol. 2, pp. 121–163, 2001. [13] D. Reiley, D. Bryanz, N. Prasad, and D. Reevesz, “Pen- nies from eBay: The determinants of price in online auc- tions,” The Journal of Industrial Economics, Vol. 55, pp. 223–233, 2007. [14] C. Mason, “The Art of the steal: Inside the Sotheby’s- Christie’s auction house scandal,” Berkley Trade, 2005. [15] S. Chou, C. S. Lin, C. Chen, T. R. Ho, and Y. C. Hsieh, “A simulation-based model for final price prediction in online auctions,” Journal of Economics and Management, Vol. 3 No. 1, pp. 1–16, 2007. Copyright © 2009 SciRes JSSM
|