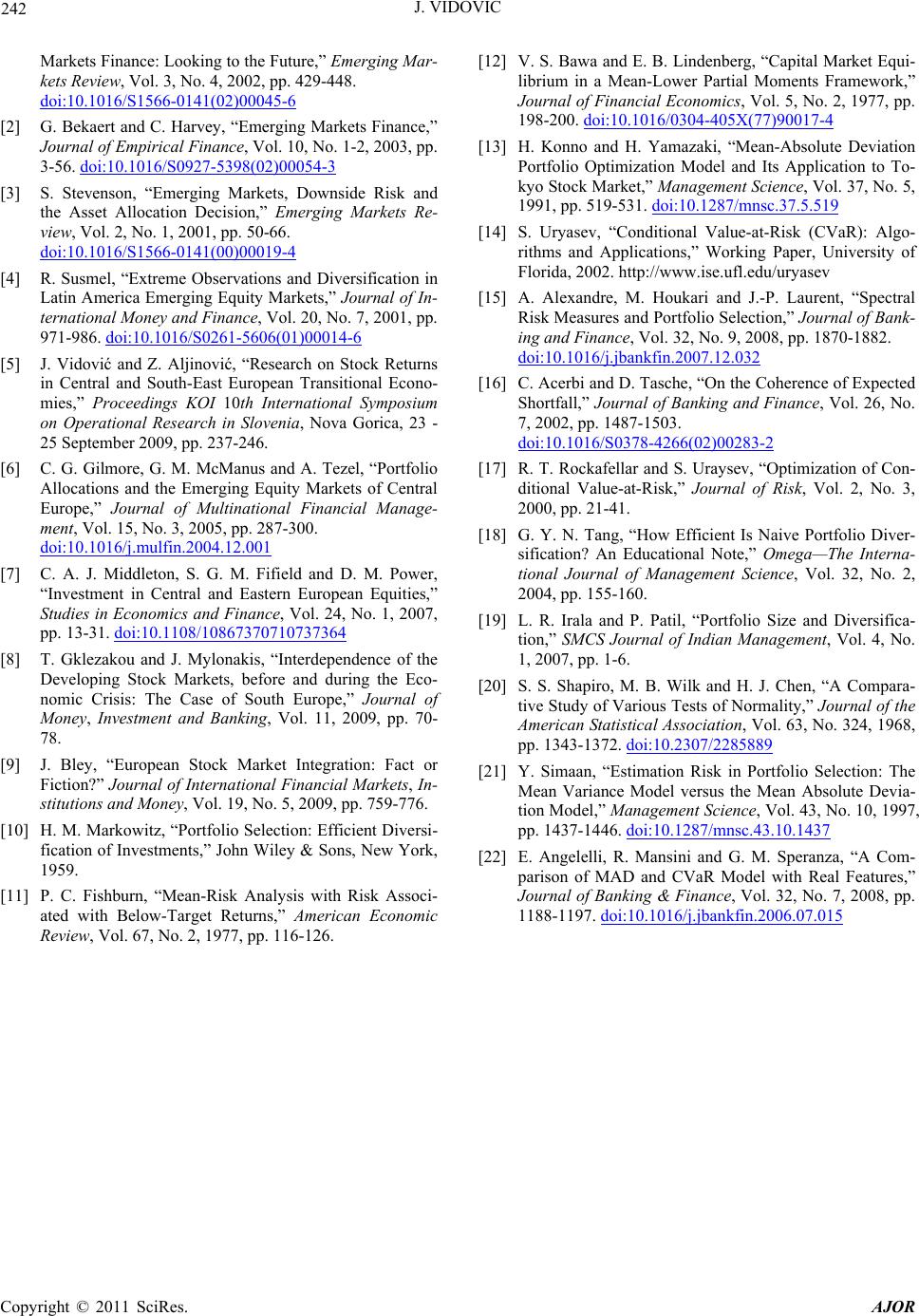
J. VIDOVIC
242
Markets Finance: Looking to the Future,” Emerging Mar-
kets Review, Vol. 3, No. 4, 2002, pp. 429-448.
doi:10.1016/S1566-0141(02)00045-6
[2] G. Bekaert and C. Harvey, “Emerging Markets Finance,”
Journal of Empirical Finance, Vol. 10, No. 1-2, 2003, pp.
3-56. doi:10.1016/S0927-5398(02)00054-3
[3] S. Stevenson, “Emerging Markets, Downside Risk and
the Asset Allocation Decision,” Emerging Markets Re-
view, Vol. 2, No. 1, 2001, pp. 50-66.
doi:10.1016/S1566-0141(00)00019-4
[4] R. Susmel, “Extreme Observations and Diversification in
Latin America Emerging Equity Markets,” Journal of In-
ternational Money and Finance, Vol. 20, No. 7, 2001, pp.
971-986. doi:10.1016/S0261-5606(01)00014-6
[5] J. Vidović and Z. Aljinović, “Research on Stock Returns
in Central and South-East European Transitional Econo-
mies,” Proceedings KOI 10th International Symposium
on Operational Research in Slovenia, Nova Gorica, 23 -
25 September 2009, pp. 237-246.
[6] C. G. Gilmore, G. M. McManus and A. Tezel, “Portfolio
Allocations and the Emerging Equity Markets of Central
Europe,” Journal of Multinational Financial Manage-
ment, Vol. 15, No. 3, 2005, pp. 287-300.
doi:10.1016/j.mulfin.2004.12.001
[7] C. A. J. Middleton, S. G. M. Fifield and D. M. Power,
“Investment in Central and Eastern European Equities,”
Studies in Economics and Finance, Vol. 24, No. 1, 2007,
pp. 13-31. doi:10.1108/10867370710737364
[8] T. Gklezakou and J. Mylonakis, “Interdependence of the
Developing Stock Markets, before and during the Eco-
nomic Crisis: The Case of South Europe,” Journal of
Money, Investment and Banking, Vol. 11, 2009, pp. 70-
78.
[9] J. Bley, “European Stock Market Integration: Fact or
Fiction?” Journal of International Financial Markets, In-
stitutions and Money, Vol. 19, No. 5, 2009, pp. 759-776.
[10] H. M. Markowitz, “Portfolio Selection: Efficient Diversi-
fication of Investments,” John Wiley & Sons, New York,
1959.
[11] P. C. Fishburn, “Mean-Risk Analysis with Risk Associ-
ated with Below-Target Returns,” American Economic
Review, Vol. 67, No. 2, 1977, pp. 116-126.
[12] V. S. Bawa and E. B. Lindenberg, “Capital Market Equi-
librium in a Mean-Lower Partial Moments Framework,”
Journal of Financial Economics, Vol. 5, No. 2, 1977, pp.
198-200. doi:10.1016/0304-405X(77)90017-4
[13] H. Konno and H. Yamazaki, “Mean-Absolute Deviation
Portfolio Optimization Model and Its Application to To-
kyo Stock Market,” Management Science, Vol. 37, No. 5,
1991, pp. 519-531. doi:10.1287/mnsc.37.5.519
[14] S. Uryasev, “Conditional Value-at-Risk (CVaR): Algo-
rithms and Applications,” Working Paper, University of
Florida, 2002. http://www.ise.ufl.edu/uryasev
[15] A. Alexandre, M. Houkari and J.-P. Laurent, “Spectral
Risk Measures and Portfolio Selection,” Journal of Bank-
ing and Finance, Vol. 32, No. 9, 2008, pp. 1870-1882.
doi:10.1016/j.jbankfin.2007.12.032
[16] C. Acerbi and D. Tasche, “On the Coherence of Expected
Shortfall,” Journal of Banking and Finance, Vol. 26, No.
7, 2002, pp. 1487-1503.
doi:10.1016/S0378-4266(02)00283-2
[17] R. T. Rockafellar and S. Uraysev, “Optimization of Con-
ditional Value-at-Risk,” Journal of Risk, Vol. 2, No. 3,
2000, pp. 21-41.
[18] G. Y. N. Tang, “How Efficient Is Naive Portfolio Diver-
sification? An Educational Note,” Omega—The Interna-
tional Journal of Management Science, Vol. 32, No. 2,
2004, pp. 155-160.
[19] L. R. Irala and P. Patil, “Portfolio Size and Diversifica-
tion,” SMCS Journal of Indian Management, Vol. 4, No.
1, 2007, pp. 1-6.
[20] S. S. Shapiro, M. B. Wilk and H. J. Chen, “A Compara-
tive Study of Various Tests of Normality,” Journal of the
American Statistical Association, Vol. 63, No. 324, 1968,
pp. 1343-1372. doi:10.2307/2285889
[21] Y. Simaan, “Estimation Risk in Portfolio Selection: The
Mean Variance Model versus the Mean Absolute Devia-
tion Model,” Management Science, Vol. 43, No. 10, 1997,
pp. 1437-1446. doi:10.1287/mnsc.43.10.1437
[22] E. Angelelli, R. Mansini and G. M. Speranza, “A Com-
parison of MAD and CVaR Model with Real Features,”
Journal of Banking & Finance, Vol. 32, No. 7, 2008, pp.
1188-1197. doi:10.1016/j.jbankfin.2006.07.015
Copyright © 2011 SciRes. AJOR