 Applied Mathematics, 2011, 2, 1387-1392 doi:10.4236/am.2011.211196 Published Online November 2011 (http://www.SciRP.org/journal/am) Copyright © 2011 SciRes. AM Higher-Order Duality for Minimax Fractional Type Programming Involving Symmetric Matrices Caiyun Jin, Cao-Zong Cheng College of Applied Sciences, Beijing University of Technol ogy, Beijing, China E-mail: {jincaiyun, czcheng}@bjut.edu.cn Received September 1, 2011; revised October 16, 2011; accepted Octo ber 23, 2011 Abstract Convexity and generalized convexity play important roles in optimization theory. With the development of programming problem, there has been a growing interest in the higher-order dual problem and a lot of related generalized convexities are given. In this paper, we give the convexity of (,,,,,)Fdb vector-pseudo- quasi-Type I and formulate a higher-order duality for minimax fractional type programming involving sym- metric matrices, and give the weak, strong and strict converse duality theorems under the condition of higher-order (,,,,,)Fdb vector-pseudoquasi-Type I. Keywords: Higher-Order (,,,,,)Fdb Vector-Pseudoquasi-Type I, Higher-Order Duality, Minimax Fractional Type Programming, Positive Semidefinite Symmetric Matrix 1. Introduction In this paper, we focus on the following nondifferen- tiable minimax fractional programming problem: 1 2 1 2 (, )() sup min (, )() T nyY xR T fxy xBx hxy xCx ()P subjectto () 0,, n xxR where is a compact subset of , Yl R,:nl hR RR and :nm RR nl RR are continuously differentiable func- tions on and , respectively, and n R 1 2 (, )()0, T fxy xBx 1 2 (, )()>0,(, ) T hxyxCxxyRR nl , B and C are two positive semidefinite symmetric matrices. nn When , (P) is a differentiable minimax fra- ctional programming problem. ==0BC The duality of programming problem involving sym- metric matrix has been investigated widely. Schmiten- dorf [1] established necessary and sufficient optimality conditions for a particular case of the following problem under convexity conditions. ()P 1 2 min( ,)() sup T yY xyxBx () P subject to () 0.gx Under the optimality conditions of [1], Tanimoto [2] defined a first-order dual problem of , which gene- ralized the duality theorems for convex minimax pro- gramming problems considered by Weir [3] and relaxed the convexity assumptions in the sufficient optimality of [1]. Mishra and Rueda [4] introduced generalized second-order type I functions and considered the mini- max programming problem involving those fun- ctions and established second-order duality theorems for problem ()P ()P ()P . Husian, Anurag Jaysural and Ahmad [5] established two types of second-order dual models for problem ()P , which extends some previously known results on minimax programming. With the development of programming problem, there has been a growing interest in the higher-order dual pro- blem. Mangasarian [6] first formulated a class of second- and higher-order du al problems for a nonlinear program- ming problem involving twice differentiable functions. In [7], Zhang considered the following nondifferentiable mathematical programming problem: 1 2 Minimize( )()T xxBx () P subject to () 0,gx under higher-order invexity assumptions. Mishra and Rueda [8] generalized the results of Zhang [7] to higher-
 C. Y. JIN ET AL. 1388 order type I functions. In [9], Ahmad, Husain and Sharma considered the nondifferentiable minimax programming problem ()P , and formulated a unified higher -order dual of (P) , and established weak, strong and strict converse duality theo - rems under higher-order (,,,) d -Type I assump- tions. In [10], Jayswal and Stancu-Minasian formulated the weak, strong and strict converse duality of (P) un- der generalized convexity of higher-order (,,,) d - Type I. For problem (P), H. C. Lai and K. Tanaka gave the necessary and sufficient conditions under the conditions of pseudo-conv ex, strictly pseudo-convex and qu asi-con- vex [11]. In this paper, we will establish a higher-order dual of (P) and give the weak, strong and strict converse duality theorems under (,,,,,)Fdb vector-pseudoquasi- Type I assumptions. The convexity conditions in this paper generalized the convexity in [8], and hence, pre- sents an answer o f a question raised in [10]. 2. Preliminaries Let be the n-dimensional Euclidean space, n Rn R be its nonegative orthant and be an open subset of . Let be the set of all feasible solutions of (P). Denote n R S =1,2, , m. For each (,) ySY, we define ()=: ()=0 j JxjM gx 1 2 1 2 1 2 1 2 (, )() ()= : (, )() (,) () =sup (,)() T T T zY T fxy xBx Yxy Y hxy xCx fxz xBx hxz xCx 12 12 =1 ()=(,,):1 1, = (,,,)with =1,=(,,,)and (),=1,2,, sls s s s isi i KxstyN RRsn ttttR tyyyy yYxis Definition 1: A function :n XXRR is said to be sublinear in its third argument, if ,, xX 1) (subadditivity) 12 ,, n aa R 121 2 (,;)(,;)(,;); xxa aFxxaFxxa 2) (positive homogeneous) ,, n RaR (,;)=(,;). xx aFxxa Let :nl RR R ,: \{XX R, , , 2 12 =( ,)R 0} n R 12 , , and ,:bd XXR :n eR R:RR . Let , :nl n wRRRR :nn lR R and be three diffe- rentiable functions. We assume that :kR nn R m R is a sublinear functional throughout this paper. Definition 2 : (,) e is said to be higher-order (,,,,Fd,)b vector-pseudoquasi-Type I at X with respect to , if for all n pR S and ()yYx , 1 (, )(,)(, )(,,) (,,)<0 (, ,(, )((,,) <(,) () (,)(,0 ( ,,( ,)((,)))( ,). Tp p Tp p bxxfxyxfxywxyp pwxyp Fxx xxwxyp dxx exlxpplxp Fxx xxlxpxx 2 1 2 22 )) ) TT x d Definition 3: (,) e ) is said to be strictly higher-order (,,,,,Fdb vector-pseudoquasi-Type I at X with respect to , if for all n pR xS and ()yYx , 1 2 1 2 22 )) ) TT x d (, )(,)(, )(,,) (,,) 0 (, ,(, )((,,) <(,) () (,)(,0 ( ,,( ,)((,)))( ,). Tp p Tp p bxxfxyxfxywxyp pwxyp Fxx xxwxyp dxx exlxpplxp Fxx xxlxpxx Obviously, when is subadditive function and sa- tisfies 0(a)a0 ,,,,,), higher-order ( u Fdb v ector-pseudo quasi-Type I is th e con- vexity condition of Theorem 3.1 in [10]. In the following section, we will use Lemma 1 and Lemma 2 which were given in [11]. Lemma 1: (Necessary Condition) If , is an optimal solution of problem (P) satisfying and >0, >0 TT xBx xCx () () j xj Jx are li- near independent, then there exist (,,)() ty Kx R , and ,n uv R , m R such that =1 =1 (, )((, )) ()=0 s ii i B i m jj j tfxyhxyu Cv gx (2.1) 11 22 (, )()(,)( ) 0, =1,2, , TT ii fxyxBx hxyxCx is (2.2) =1 ()= m jj i gx 0 (2.3) Copyright © 2011 SciRes. AM
 C. Y. JIN ET AL.1389 s =1 =1,0, =1,2,, s ii j tti (2.4) 1 2 1 2 1, 1, =() , =() . TT TT TT uBu vCv xBu xBx xCv xBx (2.5) Lemma 2: Let be a positive semidefinite symme- tric matrix of order . Then for all Bn,n uR, 11 22 ()() TT T . Bux Bxu Bu The equality holds when =Bx Bu for some 0 . Evidently, if 1 2 () T uBu 1, we have 1 2 () TT . Bux Bx 3. Duality Model We consider the following dual model . (WD) (,,) () (,,,,,)(,,), sup max styK zzuvpH sty where (,, ) sty ,,,)v p denote the set of all satisfying (,, nnn m zu RRRRRR n =1 =1 (, ,)((,))0 sm ipip jj ij twzypBuCv kzp (3.11) 1, 1, TT uBu vCv (3.12) =1 =1 (, )(, ), (,,)(,, )0, sTT ii i i sT ii pi i tfzyhzyzBu zCv twzyppwzyp (3.13) =1 ()(, )((, ))0. mT jjjjp jj j gzkzp pkzp (3.14) If for a triplet (,, )() ty Kz , the set (,, )=Hsty (,, ), then we define the supremum over sty to be . Next, we establish the duality of type (WD). Theorem 3.1 (Weak Duality) Let and (,,,,,,,,)zuv styp be feasible solutions of (P) and (WD), respectively. Assume that 1) =1 =1 (,)(,) ,() sm iii jj ij tf yhyg is higher-order (,,,,,) uCv Fdb () 0aa vect or -pseu d oquasi Type I at , z 2) 0, (,)>0,bxz 12 12 0 (,) (,) xz xz . Then 1 2 1 2 (, )(). sup (, )() T yY T fxy xBx hxy xCx Proof: From (3.14), we know that =1 ()(,)((,))0, mT jjjjp jj j gzkzp pkzp then follows form 1) and 2(,)>0xz , we have 2 2 =1 2 ,;((,))(,). (,) m pjj j xzk zpdxz xz Since is sublinear in its third argument, by (3.11) we can get =1 =1 =1 =1 =1 2 2 2 0=,;( ,,) ((,)) =,; (,,) (,;((,)) ,;(,, ) (,). (,) s ip i i m pjj j s ip i i m pjj j s ip i i Fxztwzyp Bu Cvkz p ) xztwzy pBuCv Fxzk zp xztwzy pBuCv dxz xz Furthermore, by 12 12 0 (,) (,)xz xz and 1(,)>0,xz we have 1=1 2 1 ,;(,)(,, ) (,), s ip i i xzxztwzy pBuCv dxz which implies that =1 =1 =1 (,)(,)(, ) (, )(, )() (, ,)(, ,) 0. sTT ii i i sTT ii i i sT ii pi i bxztf xyhxyxBuxCv tfzyhzyzBu zCv twzyppwzyp Copyright © 2011 SciRes. AM
 C. Y. JIN ET AL. 1390 From 2) and (3.13), we can get =1 =1 =1 (, )(, ) (, )(, )() (, ,)(, ,) 0. sTT ii i i sTT ii i i sT ii pi i tfxyhxyxBu xCv tfzyhzy zBu zCv twzyp pwzyp Therefore, following from (3.12) and Lemma 2, 11 22 =1 =1 (, )()(, )() (,)(,) 0. sTT ii i i sTT iii i t fxyxBxhxyxCx tfxyhxyxBu xCv Since , , 12 =( ,,,)0 s ttt t0, =1,2,, i tis i y and 1 2 (, )()>0,(, ) Tnl hxyxCxxyRR s , at least ex- ists one , such that {1,2,, }q 1 2 1 2 (,) (), (,) () T q T q fxy xBx hxy xCx which implies that 1 2 1 2 (, )(). sup (, )() T yY T fxy xBx hxy xCx Theorem 3.2 (Strong duality) Let >0,x be an optimal solution of (P) satisfying and let >0 TT xBx Cx (), () j xjJx =1,2, ,is be linear independent. Assume that for any (, ,0)=0, i wx y (, ,0)=(, )(, ), pi ii wx yfx yhx y and for any ()jJx (,0)=0, (,0)=(). jpj kxkx gx j Then there exist (,, )() ty Kx )(,, ) and (,,, ,, uv (,,, ,,xuv (,,, ,,xuv p Hsty ,,,=0)st y p ,,,=0)st y p such that is a feasible solution of (WD) and the two objectives have the same values. Furthermore, if the assumptions of weak duality hold for all feasible solutions of (P) and (WD), then is an optimal solu- tions of (WD). Proof: Since ,x is an optimal solution of (P) satisfy- ing and >0 >0 TT xBx Cx (), () j xjJx is linearly independent, by Lemma 1, there exist (,, )(), ty Kx and ,n uv R , m RR such that BuCv =1 =1 (, )) ()= s ii i i m jj j tfxyy gx (, 0 hx (2.1) 11 22 () =0, TT xCx (, )()(, ) =1,2, , ii fxyx Bxhxy is s (2.2) =1 ()=0 m jj i gx (2.3) =1 =1,0, =1,2,, s ii j tti (2.4) 1 2 1 2 1, 1, =() , =() . TT TT TT uBu vCv xBu xBx xCv xBx (2.5) By (2.1) (2.2) (2.3) (2.5) and the conditions of theorem 3.2, we know that (,,, ,,=0)(,, ) uvp Hsty , ,,,,,=0)st yp ,,,=0)st y p , that is is an feasible solutions of (WD). Furthermore, (3.2) implies that is an optimal solu- tions of (WD). (,,xuv ,, ,,v (,xu Theorem 3.3 (Strict Converse Duality) Let be a feasible solution of (P) and be a feasible solution of (WD). Suppose that (,,, ,zuv , )p 1)' 1 2 1 2 (, )()=; sup (, )() T yY T fxyx Bx hxyx Cx 2)' =1 =1 (, )(, ),() sm iii jj ij tfy hyg is strictly higher-order (,,,,,) uCv Fdb >0 >0,aa vector-pseudoquasi- Type I at and; z ) 3)' ( (,)>bx z 0, 12 12 0 (,)(,)xz xz Then =;zx that is z is an optimal solution of (WD). Proof: Suppose that the contradiction is not true, that is zx . Similar to the proof of Theorem 3.1 we Copyright © 2011 SciRes. AM
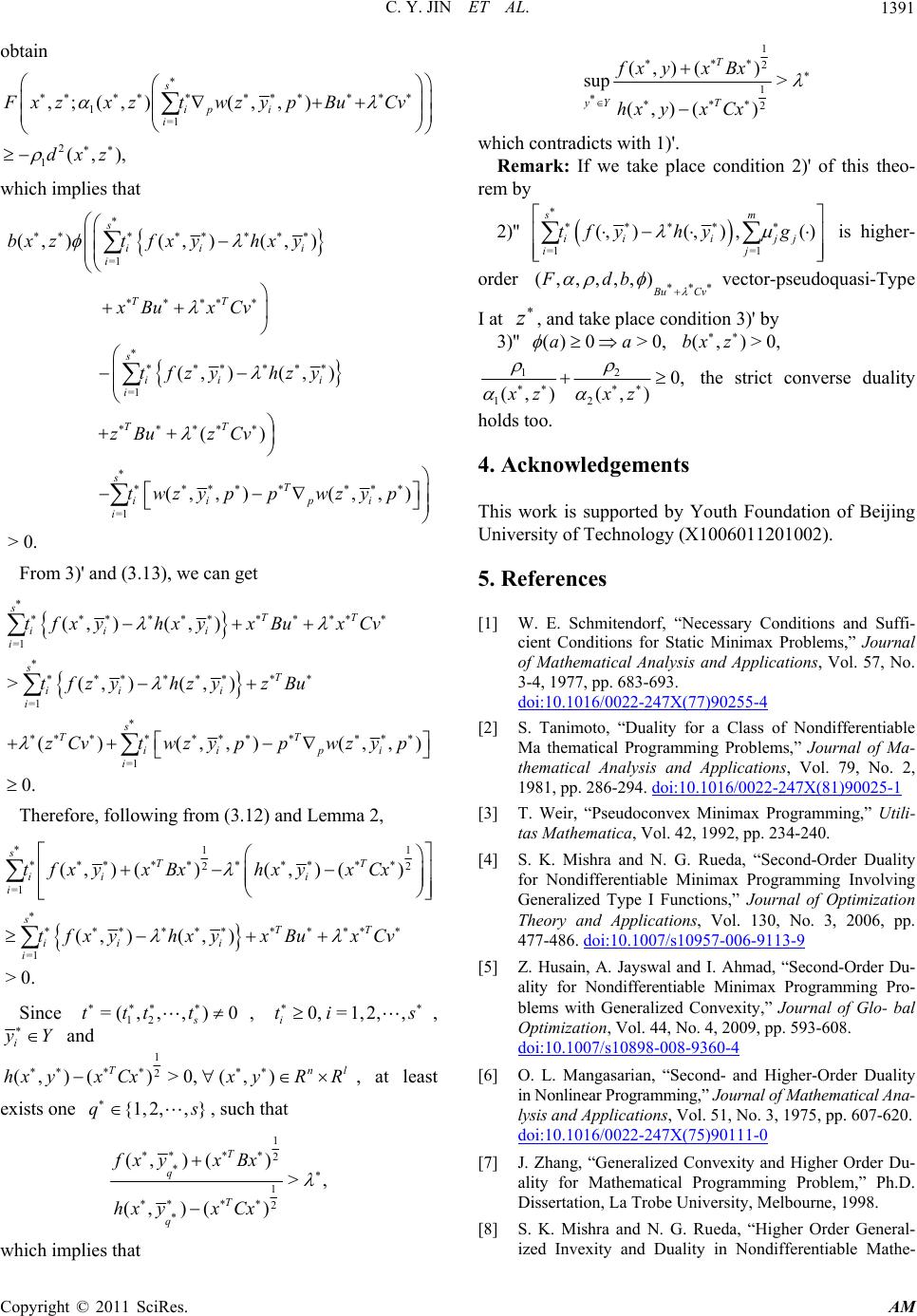 C. Y. JIN ET AL.1391 obtain 1=1 2 1 ,;(,)(,, ) (,), s ip i i FxzxztwzypBuCv dxz which implies that =1 =1 =1 (,)(,)(,) (, )(, ) () (, , )(,,) >0. s iii i TT s ii i i TT sT ii pi i bxztfxyhxy xBu xCv tfzy hzy zBuzCv twzyppwzyp From 3)' and (3.13), we can get =1 =1 =1 (, )(, ) >(,)(,) ()(, ,)(,,) 0. sTT ii i i sT iii i s TT ii pi i tfxyhxyxBuxCv tfzyhzy zBu zCvtwzyppwzyp Therefore, following from (3.12) and Lemma 2, 11 2 ) 2 =1 =1 (, )()(, )( (, )(,) >0. sTT ii i i sTT iii i tfxy xBxhxy xCx tfxyhxy xBuxCv s Since , , 12 =( ,,,)0 s ttt t 0,=1,2,, i ti i Y and 1 2 (, )()>0,(, ) Tnl hxyx CxxyRR s , at least exists one , such that {1,2,, }q 1 2 1 2 (, )()>, (, )() T q T q fxyx Bx hxyx Cx which implies that 1 2 1 2 (,)( )> sup (,)( ) T yY T fxyxBx hxyxCx which contradicts with 1)'. Remark: If we take place condition 2)' of this theo- rem by 2)'' =1 =1 (, )(, ),() sm iii jj ij tfy hyg is higher- order (,,,,,) uCv Fdb vector-pseudoquasi-Type I at , and take place condition 3)' by z 3)'' () 0>0,aa (,)>0,bx z 12 12 0, (,)(,)xz xz the strict converse duality olds too. h 4. Acknowledgements This work is supported by Youth Foundation of Beijing University of Technology (X1006011201002). 5. References [1] W. E. Schmitendorf, “Necessary Conditions and Suffi- cient Conditions for Static Minimax Problems,” Journal of Mathematical Analysis and Applications, Vol. 57, No. 3-4, 1977, pp. 683-693. doi:10.1016/0022-247X(77)90255-4 [2] S. Tanimoto, “Duality for a Class of Nondifferentiable Ma thematical Programming Problems,” Journal of Ma- thematical Analysis and Applications, Vol. 79, No. 2, 1981, pp. 286-294. doi:10.1016/0022-247X(81)90025-1 [3] T. Weir, “Pseudoconvex Minimax Programming,” Utili- tas Mathematica, Vol. 42, 1992, pp. 234-240. [4] S. K. Mishra and N. G. Rueda, “Second-Order Duality for Nondifferentiable Minimax Programming Involving Generalized Type I Functions,” Journal of Optimization Theory and Applications, Vol. 130, No. 3, 2006, pp. 477-486. doi:10.1007/s10957-006-9113-9 [5] Z. Husain, A. Jayswal and I. Ahmad, “Second-Order Du- ality for Nondifferentiable Minimax Programming Pro- blems with Generalized Convexity,” Journal of Glo- bal Optimization, Vol. 44, No. 4, 2009, pp. 593-608. doi:10.1007/s10898-008-9360-4 [6] O. L. Mangasarian, “Second- and Higher-Order Duality in Nonlinear Programming,” Journal of Mathematical Ana- lysis and Applications, Vol. 51, No. 3, 1975, pp. 607-620. doi:10.1016/0022-247X(75)90111-0 [7] J. Zhang, “Generalized Convexity and Higher Order Du- ality for Mathematical Programming Problem,” Ph.D. Dissertation, La T ro b e University, Melbourne, 1998. [8] S. K. Mishra and N. G. Rueda, “Higher Order General- ized Invexity and Duality in Nondifferentiable Mathe- Copyright © 2011 SciRes. AM
 C. Y. JIN ET AL. Copyright © 2011 SciRes. AM 1392 matical Programming,” Journal of Mathematical Analysis and Applications, Vol. 272, No. 2, 2002, pp. 496-506. doi:10.1016/S0022-247X(02)00170-1 [9] I. Ahmad, Z. Huasin and S. Sharma, “Higher-Order Du- ality in Nondifferentiable Minimax Programming with Generalized Type I Functions,” Journal of Optimization Theory and Applications, Vol. 141, No. 1, 2009, pp. 1-12. doi:10.1007/s10957-008-9474-3 [10] A. Jayswal and I. Stancu-Minasian, “Higher-Order Dual- ity for Nondifferentiable Minimax Programming Problem with Generalized Convexity,” Nonlinear Analysis: The- ory, Methods & Applications, Vol. 74, No. 2, 2011, pp. 616-625. doi:10.1016/j.na.2010.09.016 [11] H. C. Lai and J. C. Liu, “Necessary and Sufficient Condi- tions for Minimax Fractional Programming,” Journal of Mathematical Analysis and Applications, Vol. 230, No. 2, 1999, pp. 311-328. doi:10.1006/jmaa.1998.6204
|