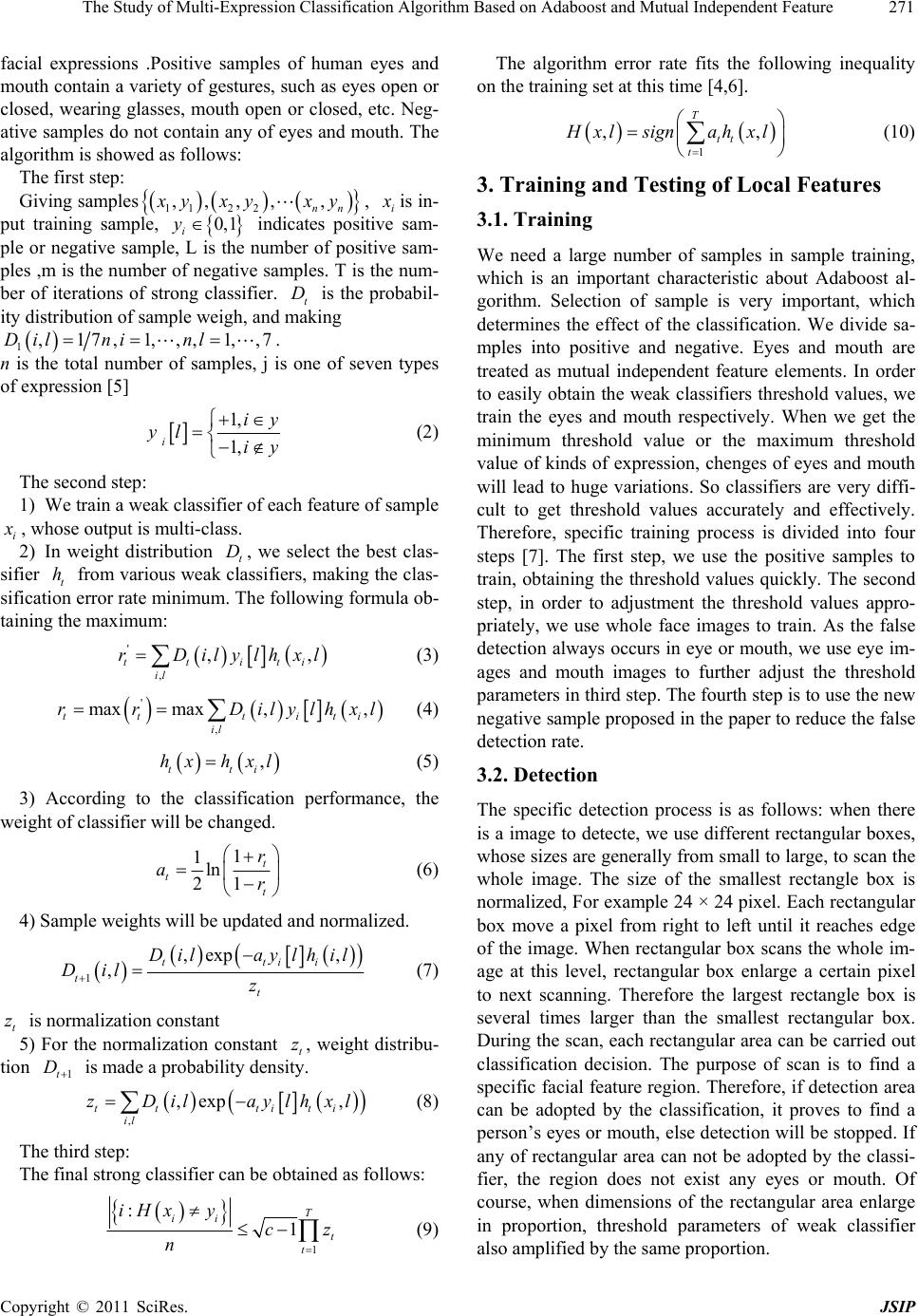
The Study of Multi-Expression Classification Algorithm Based on Adaboost and Mutual Independent Feature271
facial expressions .Positive samples of human eyes and
mouth contain a variety of gestures, such as eyes open or
closed, wearing glasses, mouth open or closed, etc. Neg-
ative samples do not contain any of eyes and mouth. The
algorithm is showed as follows:
The first step:
Giving samples
112 2
,,,, ,
nn
yxy xy, i
is in-
put training sample, indicates positive sam-
ple or negative sample, L is the number of positive sam-
ples ,m is the number of negative samples. T is the num-
ber of iterations of strong classifier. t is the probabil-
ity distribution of sample weigh, and making
0,1
i
y
D
1,17,1,,,1,, 7nlDil ni .
n is the total number of samples, j is one of seven types
of expression [5]
1,
1,
i
iy
yl iy
(2)
The second step:
1) We train a weak classifier of each feature of sample
i
, whose output is multi-class.
2) In weight distribution t
D, we select the best clas-
sifier t
h from various weak classifiers, making the clas-
sification error rate minimum. The following formula ob-
taining the maximum:
'
,,
ttiti
il
rDilylhx
,l
(3)
'
,
max max,,
tt tit
il
rr Dilylhx
i
l
(4)
,
tti
hx hxl (5)
3) According to the classification performance, the
weight of classifier will be changed.
1
1ln
21
t
tt
r
ar
(6)
4) Sample weights will be updated and normalized.
1
,exp ,
,ttii
tt
Dil aylhil
Dil z
(7)
t
z is normalization constant
5) For the normalization constant t, weight distribu-
tion is made a probability density.
z
1t
D
,,exp ,
tt titi
il
zDil aylhxl
(8)
The third step:
The final strong classifier can be obtained as follows:
1
:1T
ii
t
t
iHx yc
n
The algorithm error rate fits the following inequality
on the training set at this time [4,6].
z
,
(9)
1
,
T
tt
t
xlsignahxl
(10)
3. Training and Testing of Local Features
3.1. Training
We need a large number of samples in sample training,
which is an important characteristic about Adaboost al-
gorithm. Selection of sample is very important, which
determines the effect of the classification. We divide sa-
mples into positive and negative. Eyes and mouth are
treated as mutual independent feature elements. In order
to easily obtain the weak classifiers threshold values, we
train the eyes and mouth respectively. When we get the
minimum threshold value or the maximum threshold
value of kinds of expression, chenges of eyes and mouth
will lead to huge variations. So classifiers are very diffi-
cult to get threshold values accurately and effectively.
Therefore, specific training process is divided into four
steps [7]. The first step, we use the positive samples to
train, obtaining the threshold values quickly. The second
step, in order to adjustment the threshold values appro-
priately, we use whole face images to train. As the false
detection always occurs in eye or mouth, we use eye im-
ages and mouth images to further adjust the threshold
parameters in third step. The fourth step is to use the new
negative sample proposed in the paper to reduce the false
detection rate.
3.2. Detection
The specific detection process is as follows: when there
is a image to detecte, we use different rectangular boxes,
whose sizes are generally from small to large, to scan the
whole image. The size of the smallest rectangle box is
normalized, For example 24 × 24 pixel. Each rectangular
box move a pixel from right to left until it reaches edge
of the image. When rectangular box scans the whole im-
age at this level, rectangular box enlarge a certain pixel
to next scanning. Therefore the largest rectangle box is
several times larger than the smallest rectangular box.
During the scan, each rectangular area can be carried out
classification decision. The purpose of scan is to find a
specific facial feature region. Therefore, if detection area
can be adopted by the classification, it proves to find a
person’s eyes or mouth, else detection will be stopp ed. If
any of rectangular area can not be adopted by the classi-
fier, the region does not exist any eyes or mouth. Of
course, when dimensions of the rectangular area enlarge
in proportion, threshold parameters of weak classifier
also amplified by the same proportion.
Copyright © 2011 SciRes. JSIP