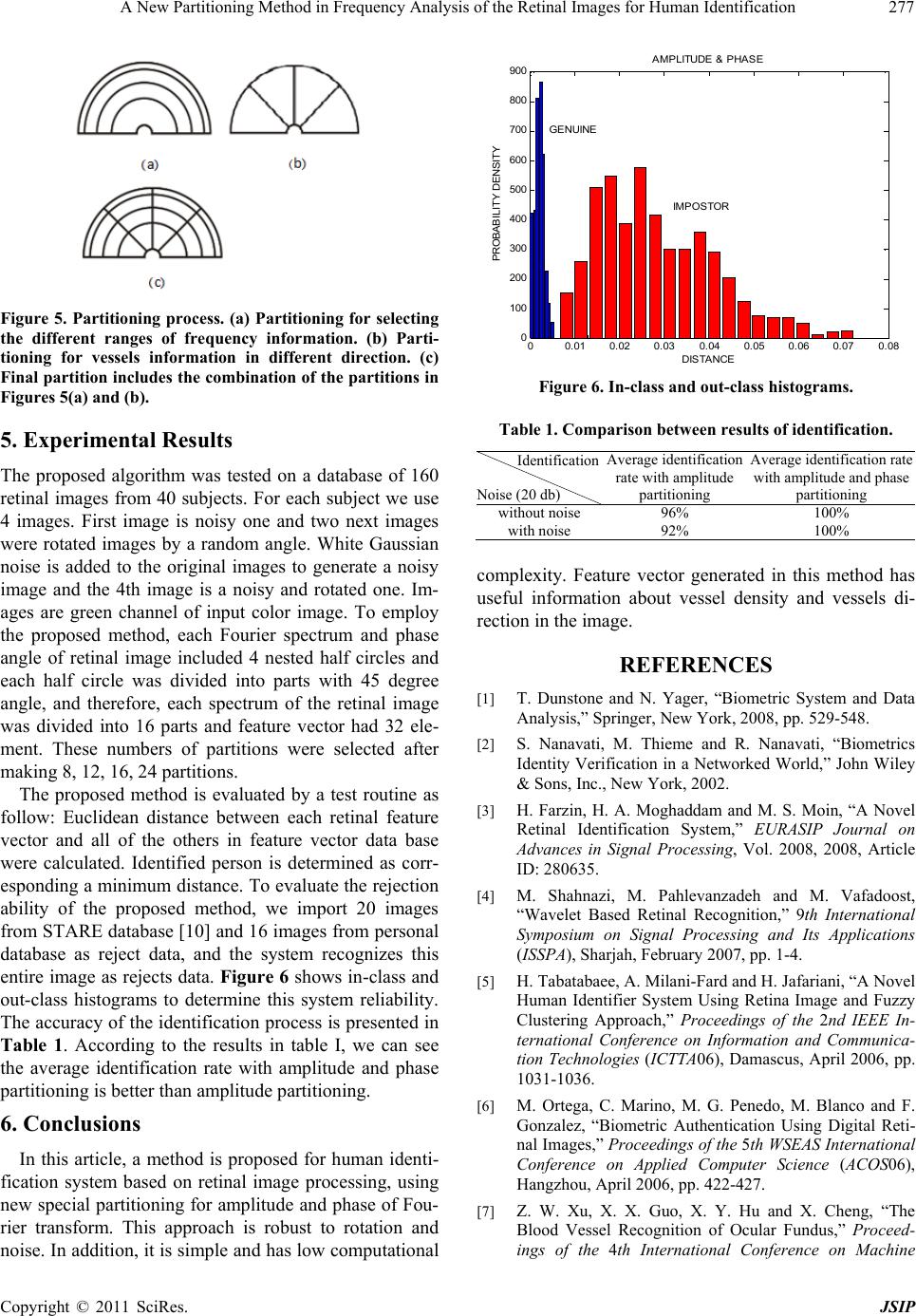
A New Partitioning Method in Frequency Analysis of the Retinal Images for Human Identification277
Figure 5. Partitioning process. (a) Partitioning for selecting
the different ranges of frequency information. (b) Parti-
tioning for vessels information in different direction. (c)
Final partition includes the combination of the partitions in
Figures 5(a) and (b).
5. Experimental Results
The proposed algorithm was tested on a database of 160
retinal images from 40 subjects. For each subject we use
4 images. First image is noisy one and two next images
were rotated images by a random angle. White Gaussian
noise is added to the original images to generate a noisy
image and the 4th image is a noisy and rotated one. Im-
ages are green channel of input color image. To employ
the proposed method, each Fourier spectrum and phase
angle of retinal image included 4 nested half circles and
each half circle was divided into parts with 45 degree
angle, and therefore, each spectrum of the retinal image
was divided into 16 parts and feature vector had 32 ele-
ment. These numbers of partitions were selected after
making 8, 12, 16, 24 partitions.
The proposed method is evaluated by a test routine as
follow: Euclidean distance between each retinal feature
vector and all of the others in feature vector data base
were calculated. Identified person is determined as corr-
esponding a minimum distance. To evaluate the rejection
ability of the proposed method, we import 20 images
from STARE database [10] and 16 images from personal
database as reject data, and the system recognizes this
entire image as rejects data. Figure 6 shows in-class and
out-class histograms to determine this system reliability.
The accuracy of the identification process is presented in
Table 1. According to the results in table I, we can see
the average identification rate with amplitude and phase
partitioning is better than amplitude partitioning.
6. Conclusions
In this article, a method is proposed for human identi-
fication system based on retinal image processing, using
new special partitioning for amplitude and phase of Fou-
rier transform. This approach is robust to rotation and
noise. In addition, it is simple and has low computational
00.01 0.02 0.03 0.04 0.05 0.06 0.070.08
0
100
200
300
400
500
600
700
800
900
DISTANCE
PROBABILITY DENSITY
AMPLITUDE & PHASE
GENUINE
IMPOSTOR
Figure 6. In-class and out-class histograms.
Table 1. Comparison between results of identification.
Identification
Noise (20 db)
Average identification
rate with amplitude
partitioning
Average identification rate
with amplitude and phase
partitioning
without noise 96% 100%
with noise 92% 100%
complexity. Feature vector generated in this method has
useful information about vessel density and vessels di-
rection in the image.
REFERENCES
[1] T. Dunstone and N. Yager, “Biometric System and Data
Analysis,” Springer, New York, 2008, pp. 529-548.
[2] S. Nanavati, M. Thieme and R. Nanavati, “Biometrics
Identity Verification in a Networked World,” John Wiley
& Sons, Inc., New York, 2002.
[3] H. Farzin, H. A. Moghaddam and M. S. Moin, “A Novel
Retinal Identification System,” EURASIP Journal on
Advances in Signal Processing, Vol. 2008, 2008, Article
ID: 280635.
[4] M. Shahnazi, M. Pahlevanzadeh and M. Vafadoost,
“Wavelet Based Retinal Recognition,” 9th International
Symposium on Signal Processing and Its Applications
(ISSPA), Sharjah, February 2007, pp. 1-4.
[5] H. Tabatabaee, A. Milani-Fard and H. Jafariani, “A Novel
Human Identifier System Using Retina Image and Fuzzy
Clustering Approach,” Proceedings of the 2nd IEEE In-
ternational Conference on Information and Communica-
tion Technologies (ICTTA06), Damascus, April 2006, pp.
1031-1036.
[6] M. Ortega, C. Marino, M. G. Penedo, M. Blanco and F.
Gonzalez, “Biometric Authentication Using Digital Reti-
nal Images,” Proceedings of the 5th WSEAS International
Conference on Applied Computer Science (ACOS06),
Hangzhou, April 2006, pp. 422-427.
[7] Z. W. Xu, X. X. Guo, X. Y. Hu and X. Cheng, “The
Blood Vessel Recognition of Ocular Fundus,” Proceed-
ings of the 4th International Conference on Machine
Copyright © 2011 SciRes. JSIP