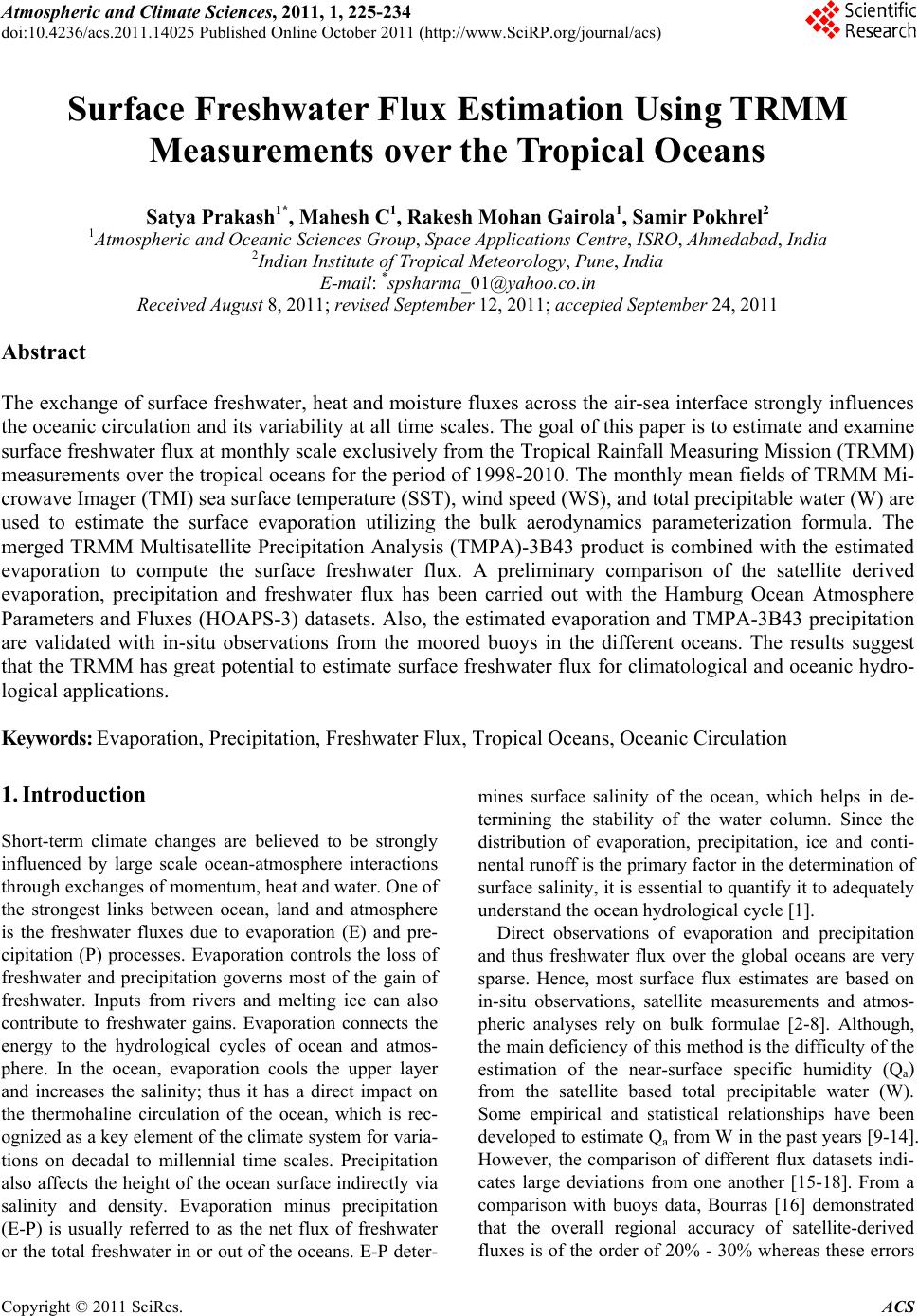 Atmospheric and Climate Sciences, 2011, 1, 225-234 doi:10.4236/acs.2011.14025 Published Online October 2011 (http://www.SciRP.org/journal/acs) Copyright © 2011 SciRes. ACS Surface Freshwater Flux Estimation Using TRMM Measurements over the Tropical Oceans Satya Prakash1*, Mahesh C1, Rakesh Mohan Gairola1, Samir Pokhrel2 1Atmospheric and Oceanic Sciences Group, Space Applications Centre, ISRO, Ahmedabad, India 2Indian Institute of Tropical Meteorology, Pune, India E-mail: *spsharma_01@yahoo.co.in Received August 8, 2011; revised September 12, 2011; accepted September 24, 2011 Abstract The exchange of surface freshwater, heat and moisture fluxes across the air-sea interface strongly influences the oceanic circulation and its variability at all time scales. The goal of this paper is to estimate and examine surface freshwater flux at monthly scale exclusively from the Tropical Rainfall Measuring Mission (TRMM) measurements over the tropical oceans for the period of 1998-2010. The monthly mean fields of TRMM Mi- crowave Imager (TMI) sea surface temperature (SST), wind speed (WS), and total precipitable water (W) are used to estimate the surface evaporation utilizing the bulk aerodynamics parameterization formula. The merged TRMM Multisatellite Precipitation Analysis (TMPA)-3B43 product is combined with the estimated evaporation to compute the surface freshwater flux. A preliminary comparison of the satellite derived evaporation, precipitation and freshwater flux has been carried out with the Hamburg Ocean Atmosphere Parameters and Fluxes (HOAPS-3) datasets. Also, the estimated evaporation and TMPA-3B43 precipitation are validated with in-situ observations from the moored buoys in the different oceans. The results suggest that the TRMM has great potential to estimate surface freshwater flux for climatological and oceanic hydro- logical applications. Keywords: Evaporation, Precipitation, Freshwater Flux, Tropical Oceans, Oceanic Circulation 1. Introduction Short-term climate changes are believed to be strongly influenced by large scale ocean-atmosphere interactions through exchanges of momentum, heat and water. One of the strongest links between ocean, land and atmosphere is the freshwater fluxes due to evaporation (E) and pre- cipitation (P) processes. Evaporation controls the loss of freshwater and precipitation governs most of the gain of freshwater. Inputs from rivers and melting ice can also contribute to freshwater gains. Evaporation connects the energy to the hydrological cycles of ocean and atmos- phere. In the ocean, evaporation cools the upper layer and increases the salinity; thus it has a direct impact on the thermohaline circulation of the ocean, which is rec- ognized as a key element of the climate system for varia- tions on decadal to millennial time scales. Precipitation also affects the height of the ocean surface indirectly via salinity and density. Evaporation minus precipitation (E-P) is usually referred to as the net flux of freshwater or the total freshwater in or out of the oceans. E-P deter- mines surface salinity of the ocean, which helps in de- termining the stability of the water column. Since the distribution of evaporation, precipitation, ice and conti- nental runoff is the primary factor in the determination of surface salinity, it is essential to quantify it to adequately understand the ocean hydrological cycle [1]. Direct observations of evaporation and precipitation and thus freshwater flux over the global oceans are very sparse. Hence, most surface flux estimates are based on in-situ observations, satellite measurements and atmos- pheric analyses rely on bulk formulae [2-8]. Although, the main deficiency of this method is the difficulty of the estimation of the near-surface specific humidity (Qa) from the satellite based total precipitable water (W). Some empirical and statistical relationships have been developed to estimate Qa from W in the past years [9-14]. However, the comparison of different flux datasets indi- cates large deviations from one another [15-18]. From a comparison with buoys data, Bourras [16] demonstrated that the overall regional accuracy of satellite-derived fluxes is of the order of 20% - 30% whereas these errors
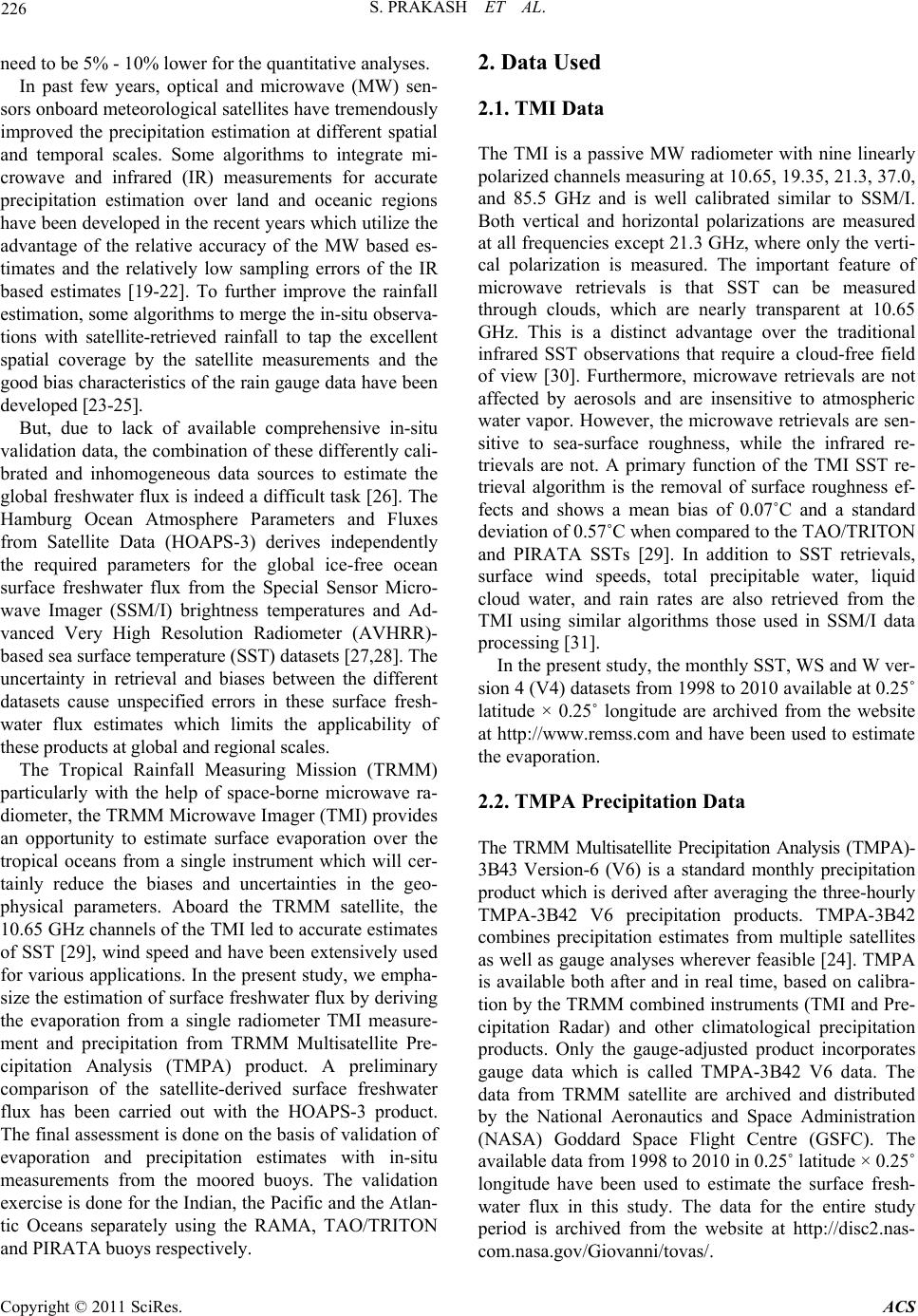 S. PRAKASH ET AL. 226 need to be 5% - 10% lower for the quantitative analyses. In past few years, optical and microwave (MW) sen- sors onboard meteorological satellites have tremendously improved the precipitation estimation at different spatial and temporal scales. Some algorithms to integrate mi- crowave and infrared (IR) measurements for accurate precipitation estimation over land and oceanic regions have been developed in the recent years which utilize the advantage of the relative accuracy of the MW based es- timates and the relatively low sampling errors of the IR based estimates [19-22]. To further improve the rainfall estimation, some algorithms to merge the in-situ observa- tions with satellite-retrieved rainfall to tap the excellent spatial coverage by the satellite measurements and the good bias characteristics of the rain gauge data have been developed [23-25]. But, due to lack of available comprehensive in-situ validation data, the combination of these differently cali- brated and inhomogeneous data sources to estimate the global freshwater flux is indeed a difficult task [26]. The Hamburg Ocean Atmosphere Parameters and Fluxes from Satellite Data (HOAPS-3) derives independently the required parameters for the global ice-free ocean surface freshwater flux from the Special Sensor Micro- wave Imager (SSM/I) brightness temperatures and Ad- vanced Very High Resolution Radiometer (AVHRR)- based sea surface temperature (SST) datasets [27,28]. The uncertainty in retrieval and biases between the different datasets cause unspecified errors in these surface fresh- water flux estimates which limits the applicability of these products at global and regional scales. The Tropical Rainfall Measuring Mission (TRMM) particularly with the help of space-borne microwave ra- diometer, the TRMM Microwave Imager (TMI) provides an opportunity to estimate surface evaporation over the tropical oceans from a single instrument which will cer- tainly reduce the biases and uncertainties in the geo- physical parameters. Aboard the TRMM satellite, the 10.65 GHz channels of the TMI led to accurate estimates of SST [29], wind speed and have been extensively used for various applications. In the present study, we empha- size the estimation of surface freshwater flux by deriving the evaporation from a single radiometer TMI measure- ment and precipitation from TRMM Multisatellite Pre- cipitation Analysis (TMPA) product. A preliminary comparison of the satellite-derived surface freshwater flux has been carried out with the HOAPS-3 product. The final assessment is done on the basis of validation of evaporation and precipitation estimates with in-situ measurements from the moored buoys. The validation exercise is done for the Indian, the Pacific and the Atlan- tic Oceans separately using the RAMA, TAO/TRITON and PIRATA buoys respectively. 2. Data Used 2.1. TMI Data The TMI is a passive MW radiometer with nine linearly polarized channels measuring at 10.65, 19.35, 21.3, 37.0, and 85.5 GHz and is well calibrated similar to SSM/I. Both vertical and horizontal polarizations are measured at all frequencies except 21.3 GHz, where only the verti- cal polarization is measured. The important feature of microwave retrievals is that SST can be measured through clouds, which are nearly transparent at 10.65 GHz. This is a distinct advantage over the traditional infrared SST observations that require a cloud-free field of view [30]. Furthermore, microwave retrievals are not affected by aerosols and are insensitive to atmospheric water vapor. However, the microwave retrievals are sen- sitive to sea-surface roughness, while the infrared re- trievals are not. A primary function of the TMI SST re- trieval algorithm is the removal of surface roughness ef- fects and shows a mean bias of 0.07˚C and a standard deviation of 0.57˚C when compared to the TAO/TRITON and PIRATA SSTs [29]. In addition to SST retrievals, surface wind speeds, total precipitable water, liquid cloud water, and rain rates are also retrieved from the TMI using similar algorithms those used in SSM/I data processing [31]. In the present study, the monthly SST, WS and W ver- sion 4 (V4) datasets from 1998 to 2010 available at 0.25˚ latitude × 0.25˚ longitude are archived from the website at http://www.remss.com and have been used to estimate the evaporation. 2.2. TMPA Precipitation Data The TRMM Multisatellite Precipitation Analysis (TMPA)- 3B43 Version-6 (V6) is a standard monthly precipitation product which is derived after averaging the three-hourly TMPA-3B42 V6 precipitation products. TMPA-3B42 combines precipitation estimates from multiple satellites as well as gauge analyses wherever feasible [24]. TMPA is available both after and in real time, based on calibra- tion by the TRMM combined instruments (TMI and Pre- cipitation Radar) and other climatological precipitation products. Only the gauge-adjusted product incorporates gauge data which is called TMPA-3B42 V6 data. The data from TRMM satellite are archived and distributed by the National Aeronautics and Space Administration (NASA) Goddard Space Flight Centre (GSFC). The available data from 1998 to 2010 in 0.25˚ latitude × 0.25˚ longitude have been used to estimate the surface fresh- water flux in this study. The data for the entire study period is archived from the website at http://disc2.nas- com.nasa.gov/Giovanni/tovas/. Copyright © 2011 SciRes. ACS
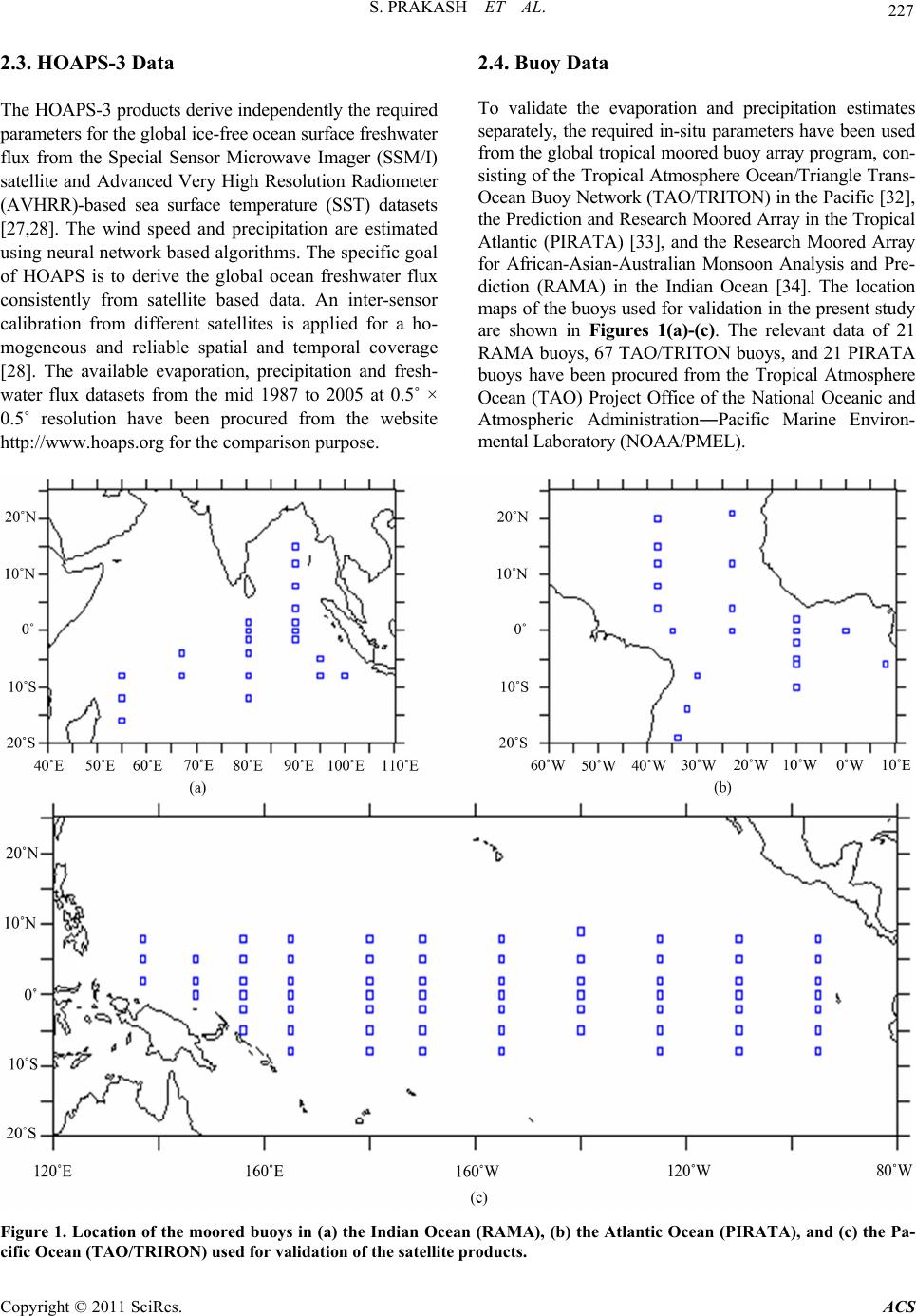 S. PRAKASH ET AL. Copyright © 2011 SciRes. ACS 227 2.3. HOAPS-3 Data 2.4. Buoy Data To validate the evaporation and precipitation estimates separately, the required in-situ parameters have been used from the global tropical moored buoy array program, con- sisting of the Tropical Atmosphere Ocean/Triangle Trans- Ocean Buoy Network (TAO/TRITON) in the Pacific [32], the Prediction and Research Moored Array in the Tropical Atlantic (PIRATA) [33], and the Research Moored Array for African-Asian-Australian Monsoon Analysis and Pre- diction (RAMA) in the Indian Ocean [34]. The location maps of the buoys used for validation in the present study are shown in Figures 1(a)-(c). The relevant data of 21 RAMA buoys, 67 TAO/TRITON buoys, and 21 PIRATA buoys have been procured from the Tropical Atmosphere Ocean (TAO) Project Office of the National Oceanic and Atmospheric Administration―Pacific Marine Environ- mental Laboratory (NOAA/PMEL). The HOAPS-3 products derive independently the required parameters for the global ice-free ocean surface freshwater flux from the Special Sensor Microwave Imager (SSM/I) satellite and Advanced Very High Resolution Radiometer (AVHRR)-based sea surface temperature (SST) datasets [27,28]. The wind speed and precipitation are estimated using neural network based algorithms. The specific goal of HOAPS is to derive the global ocean freshwater flux consistently from satellite based data. An inter-sensor calibration from different satellites is applied for a ho- mogeneous and reliable spatial and temporal coverage [28]. The available evaporation, precipitation and fresh- water flux datasets from the mid 1987 to 2005 at 0.5˚ × 0.5˚ resolution have been procured from the website http://www.hoaps.org for the comparison purpose. Figure 1. Location of the moored buoys in (a) the Indian Ocean (RAMA), (b) the Atlantic Ocean (PIRATA), and (c) the Pa- cific Ocean (TAO/TRIRON) used for validation of the satellite products.
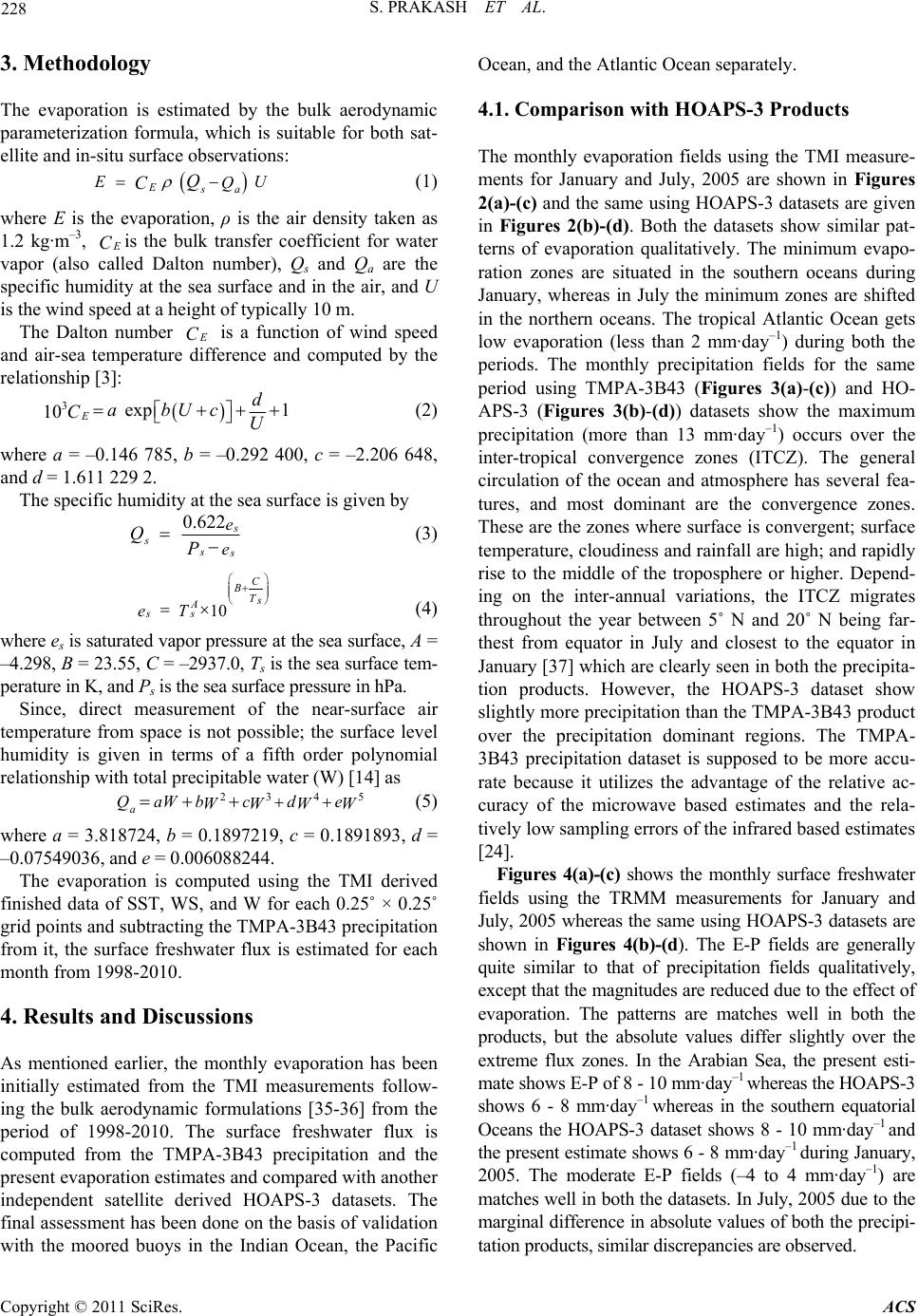 S. PRAKASH ET AL. 228 3. Methodology The evaporation is estimated by the bulk aerodynamic parameterization formula, which is suitable for both sat- ellite and in-situ surface observations: Ea s U Q Q C (1) where E is the evaporation, ρ is the air density taken as 1.2 kg·m–3, Cis the bulk transfer coefficient for water vapor (also called Dalton number), Qs and Qa are the specific humidity at the sea surface and in the air, and U is the wind speed at a height of typically 10 m. The Dalton number C is a function of wind speed and air-sea temperature difference and computed by the relationship [3]: 3 exp1 10 E d abUc CU (2) where a = –0.146 785, b = –0.292 400, c = –2.206 648, and d = 1.611 229 2. The specific humidity at the sea surface is given by 0.622 s s e e QP (3) 10 C BT A s s eT (4) where es is saturated vapor pressure at the sea surface, A = –4.298, B = 23.55, C = –2937.0, Ts is the sea surface tem- perature in K, and Ps is the sea surface pressure in hPa. Since, direct measurement of the near-surface air temperature from space is not possible; the surface level humidity is given in terms of a fifth order polynomial relationship with total precipitable water (W) [14] as 23 4 aaW bcde QWWWW 5 (5) where a = 3.818724, b = 0.1897219, c = 0.1891893, d = –0.07549036, and e = 0.006088244. The evaporation is computed using the TMI derived finished data of SST, WS, and W for each 0.25˚ × 0.25˚ grid points and subtracting the TMPA-3B43 precipitation from it, the surface freshwater flux is estimated for each month from 1998-2010. 4. Results and Discussions As mentioned earlier, the monthly evaporation has been initially estimated from the TMI measurements follow- ing the bulk aerodynamic formulations [35-36] from the period of 1998-2010. The surface freshwater flux is computed from the TMPA-3B43 precipitation and the present evaporation estimates and compared with another independent satellite derived HOAPS-3 datasets. The final assessment has been done on the basis of validation with the moored buoys in the Indian Ocean, the Pacific Ocean, and the Atlantic Ocean separately. 4.1. Comparison with HOAPS-3 Products The monthly evaporation fields using the TMI measure- ments for January and July, 2005 are shown in Figures 2(a)-(c) and the same using HOAPS-3 datasets are given in Figures 2(b)-(d). Both the datasets show similar pat- terns of evaporation qualitatively. The minimum evapo- ration zones are situated in the southern oceans during January, whereas in July the minimum zones are shifted in the northern oceans. The tropical Atlantic Ocean gets low evaporation (less than 2 mm·day–1) during both the periods. The monthly precipitation fields for the same period using TMPA-3B43 (Figures 3(a)-(c)) and HO- APS-3 (Figures 3(b)-(d)) datasets show the maximum precipitation (more than 13 mm·day–1) occurs over the inter-tropical convergence zones (ITCZ). The general circulation of the ocean and atmosphere has several fea- tures, and most dominant are the convergence zones. These are the zones where surface is convergent; surface temperature, cloudiness and rainfall are high; and rapidly rise to the middle of the troposphere or higher. Depend- ing on the inter-annual variations, the ITCZ migrates throughout the year between 5˚ N and 20˚ N being far- thest from equator in July and closest to the equator in January [37] which are clearly seen in both the precipita- tion products. However, the HOAPS-3 dataset show slightly more precipitation than the TMPA-3B43 product over the precipitation dominant regions. The TMPA- 3B43 precipitation dataset is supposed to be more accu- rate because it utilizes the advantage of the relative ac- curacy of the microwave based estimates and the rela- tively low sampling errors of the infrared based estimates [24]. Figures 4(a)-(c) shows the monthly surface freshwater fields using the TRMM measurements for January and July, 2005 whereas the same using HOAPS-3 datasets are shown in Figures 4(b)-(d). The E-P fields are generally quite similar to that of precipitation fields qualitatively, except that the magnitudes are reduced due to the effect of evaporation. The patterns are matches well in both the products, but the absolute values differ slightly over the extreme flux zones. In the Arabian Sea, the present esti- mate shows E-P of 8 - 10 mm·day–1 whereas the HOAPS-3 shows 6 - 8 mm·day–1 whereas in the southern equatorial Oceans the HOAPS-3 dataset shows 8 - 10 mm·day–1 and the present estimate shows 6 - 8 mm·day–1 during January, 2005. The moderate E-P fields (–4 to 4 mm·day–1) are matches well in both the datasets. In July, 2005 due to the marginal difference in absolute values of both the precipi- tation products, similar discrepancies are observed. Copyright © 2011 SciRes. ACS
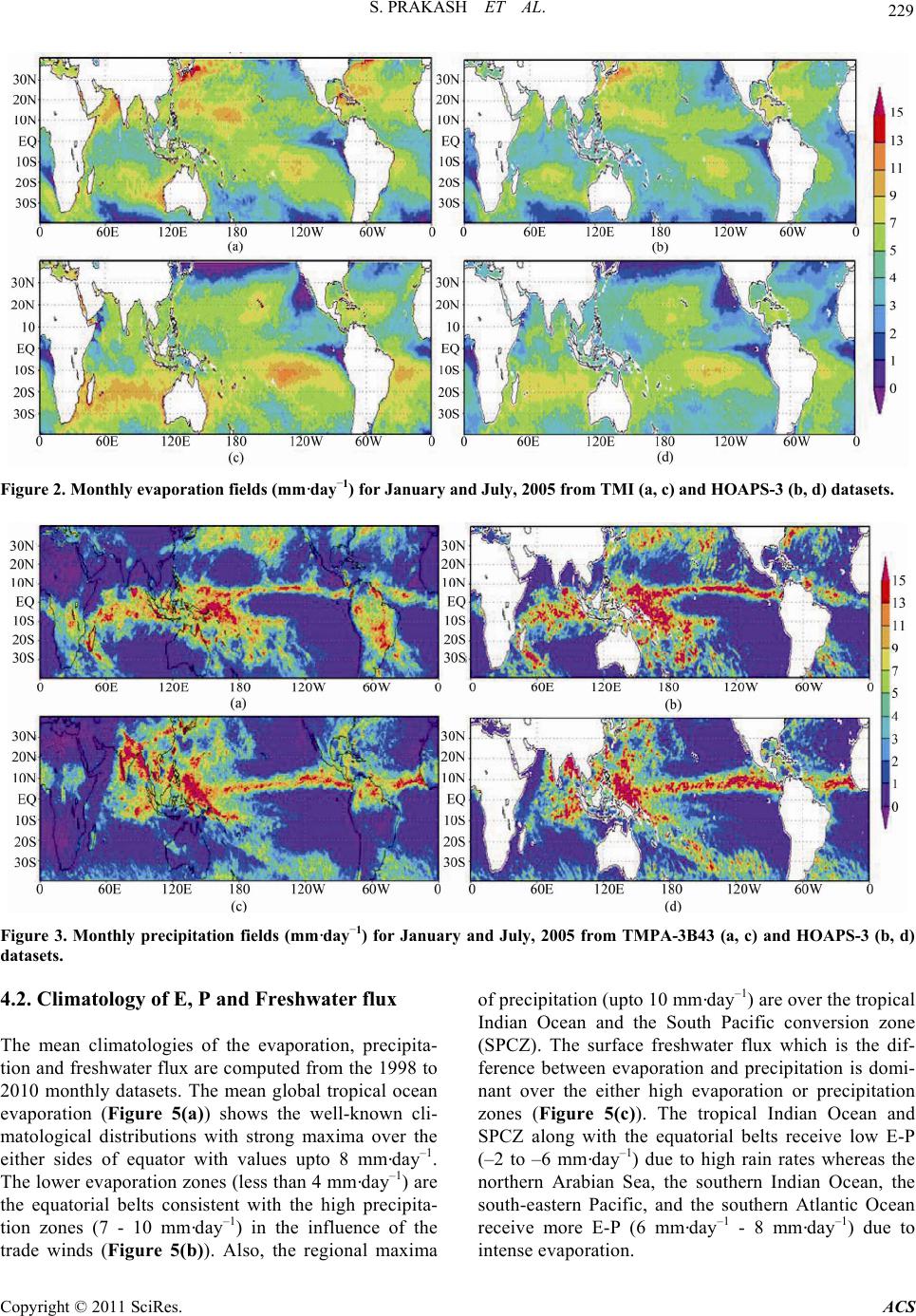 229 S. PRAKASH ET AL. Figure 2. Monthly evaporation fields (mm·day–1) for January and July, 2005 from TMI (a, c) and HOAPS-3 (b, d) datasets. Figure 3. Monthly precipitation fields (mm·day–1) for January and July, 2005 from TMPA-3B43 (a, c) and HOAPS-3 (b, d) datasets. 4.2. Climatology of E, P and Freshwater flux The mean climatologies of the evaporation, precipita- tion and freshwater flux are computed from the 1998 to 2010 monthly datasets. The mean global tropical ocean evaporation (Figure 5(a)) shows the well-known cli- matological distributions with strong maxima over the either sides of equator with values upto 8 mm·day–1. The lower evaporation zones (less than 4 mm·day–1) are the equatorial belts consistent with the high precipita- tion zones (7 - 10 mm·day–1) in the influence of the trade winds (Figure 5(b)). Also, the regional maxima of precipitation (upto 10 mm·day–1) are over the tropical Indian Ocean and the South Pacific conversion zone (SPCZ). The surface freshwater flux which is the dif- ference between evaporation and precipitation is domi- nant over the either high evaporation or precipitation zones (Figure 5(c)). The tropical Indian Ocean and SPCZ along with the equatorial belts receive low E-P (–2 to –6 mm·day–1) due to high rain rates whereas the northern Arabian Sea, the southern Indian Ocean, the south-eastern Pacific, and the southern Atlantic Ocean receive more E-P (6 mm·day–1 - 8 mm·day–1) due to intense evaporation. Copyright © 2011 SciRes. ACS
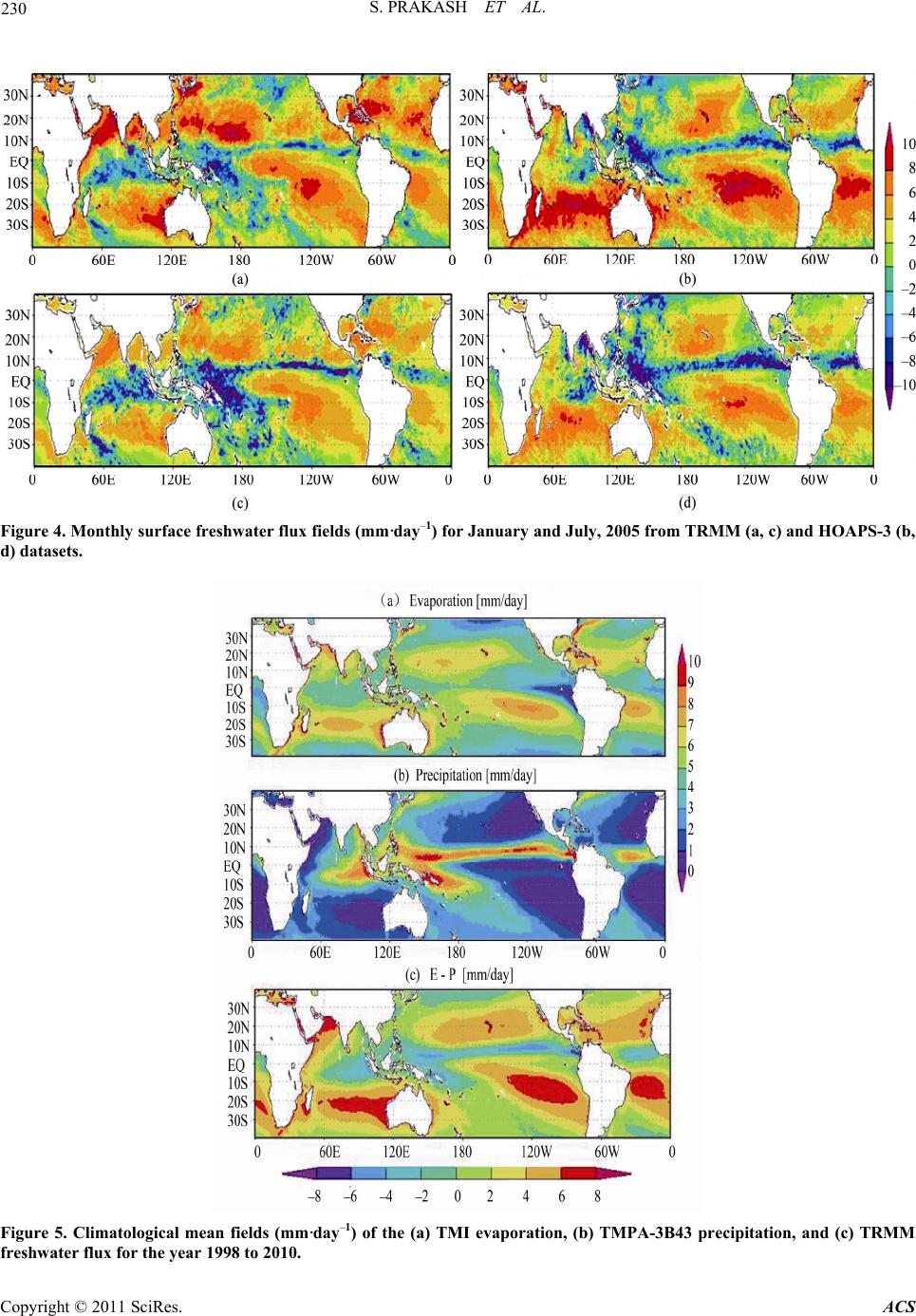 S. PRAKASH ET AL. 230 Figure 4. Monthly surface freshwater flux fields (mm·day–1) for January and July, 2005 from TRMM (a, c) and HOAPS-3 (b, d) datasets. Figure 5. Climatological mean fields (mm·day–1) of the (a) TMI evaporation, (b) TMPA-3B43 precipitation, and (c) TRMM freshwater flux for the year 1998 to 2010. Copyright © 2011 SciRes. ACS
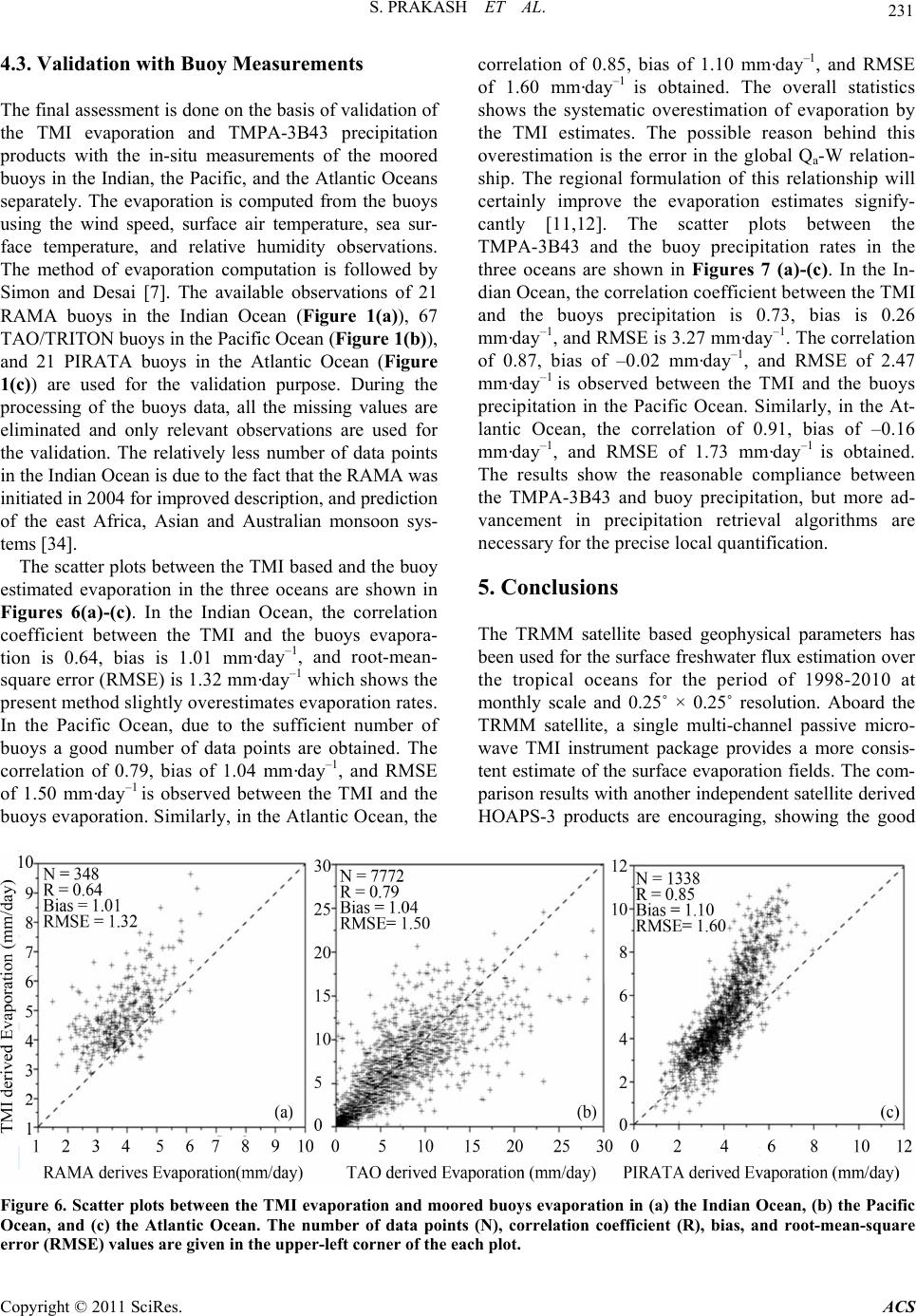 231 S. PRAKASH ET AL. 4.3. Validation with Buoy Measurements The final assessment is done on the basis of validation of the TMI evaporation and TMPA-3B43 precipitation products with the in-situ measurements of the moored buoys in the Indian, the Pacific, and the Atlantic Oceans separately. The evaporation is computed from the buoys using the wind speed, surface air temperature, sea sur- face temperature, and relative humidity observations. The method of evaporation computation is followed by Simon and Desai [7]. The available observations of 21 RAMA buoys in the Indian Ocean (Figure 1(a)), 67 TAO/TRITON buoys in the Pacific Ocean (Figure 1(b)), and 21 PIRATA buoys in the Atlantic Ocean (Figure 1(c)) are used for the validation purpose. During the processing of the buoys data, all the missing values are eliminated and only relevant observations are used for the validation. The relatively less number of data points in the Indian Ocean is due to the fact that the RAMA was initiated in 2004 for improved description, and prediction of the east Africa, Asian and Australian monsoon sys- tems [34]. The scatter plots between the TMI based and the buoy estimated evaporation in the three oceans are shown in Figures 6(a)-(c). In the Indian Ocean, the correlation coefficient between the TMI and the buoys evapora- tion is 0.64, bias is 1.01 mm·day–1, and root-mean- square error (RMSE) is 1.32 mm·day–1 which shows the present method slightly overestimates evaporation rates. In the Pacific Ocean, due to the sufficient number of buoys a good number of data points are obtained. The correlation of 0.79, bias of 1.04 mm·day–1, and RMSE of 1.50 mm·day–1 is observed between the TMI and the buoys evaporation. Similarly, in the Atlantic Ocean, the correlation of 0.85, bias of 1.10 mm·day–1, and RMSE of 1.60 mm·day–1 is obtained. The overall statistics shows the systematic overestimation of evaporation by the TMI estimates. The possible reason behind this overestimation is the error in the global Qa-W relation- ship. The regional formulation of this relationship will certainly improve the evaporation estimates signify- cantly [11,12]. The scatter plots between the TMPA-3B43 and the buoy precipitation rates in the three oceans are shown in Figures 7 (a)-(c). In the In- dian Ocean, the correlation coefficient between the TMI and the buoys precipitation is 0.73, bias is 0.26 mm·day–1, and RMSE is 3.27 mm·day–1. The correlation of 0.87, bias of –0.02 mm·day–1, and RMSE of 2.47 mm·day–1 is observed between the TMI and the buoys precipitation in the Pacific Ocean. Similarly, in the At- lantic Ocean, the correlation of 0.91, bias of –0.16 mm·day–1, and RMSE of 1.73 mm·day–1 is obtained. The results show the reasonable compliance between the TMPA-3B43 and buoy precipitation, but more ad- vancement in precipitation retrieval algorithms are necessary for the precise local quantification. 5. Conclusions The TRMM satellite based geophysical parameters has been used for the surface freshwater flux estimation over the tropical oceans for the period of 1998-2010 at monthly scale and 0.25˚ × 0.25˚ resolution. Aboard the TRMM satellite, a single multi-channel passive micro- wave TMI instrument package provides a more consis- tent estimate of the surface evaporation fields. The com- parison results with another independent satellite derived HOAPS-3 products are encouraging, showing the good Figure 6. Scatter plots between the TMI evaporation and moored buoys evaporation in (a) the Indian Ocean, (b) the Pacific Ocean, and (c) the Atlantic Ocean. The number of data points (N), correlation coefficient (R), bias, and root-mean-square error (RMSE) values are given in the upper-left corner of the each plot. Copyright © 2011 SciRes. ACS
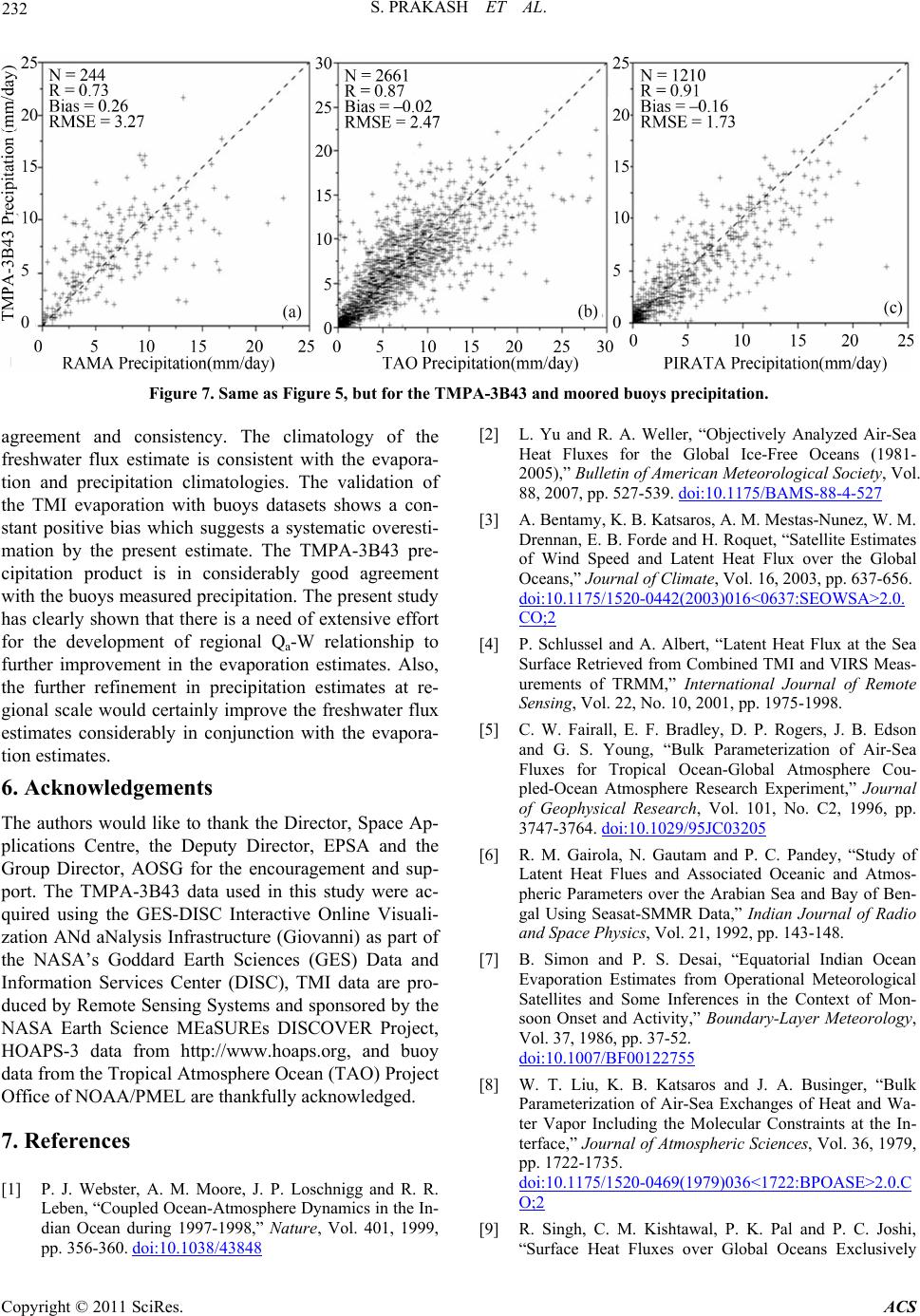 S. PRAKASH ET AL. 232 Figure 7. Same as Figure 5, but for the TMPA-3B43 and moored buoys precipitation. agreement and consistency. The climatology of the freshwater flux estimate is consistent with the evapora- tion and precipitation climatologies. The validation of the TMI evaporation with buoys datasets shows a con- stant positive bias which suggests a systematic overesti- mation by the present estimate. The TMPA-3B43 pre- cipitation product is in considerably good agreement with the buoys measured precipitation. The present study has clearly shown that there is a need of extensive effort for the development of regional Qa-W relationship to further improvement in the evaporation estimates. Also, the further refinement in precipitation estimates at re- gional scale would certainly improve the freshwater flux estimates considerably in conjunction with the evapora- tion estimates. 6. Acknowledgements The authors would like to thank the Director, Space Ap- plications Centre, the Deputy Director, EPSA and the Group Director, AOSG for the encouragement and sup- port. The TMPA-3B43 data used in this study were ac- quired using the GES-DISC Interactive Online Visuali- zation ANd aNalysis Infrastructure (Giovanni) as part of the NASA’s Goddard Earth Sciences (GES) Data and Information Services Center (DISC), TMI data are pro- duced by Remote Sensing Systems and sponsored by the NASA Earth Science MEaSUREs DISCOVER Project, HOAPS-3 data from http://www.hoaps.org, and buoy data from the Tropical Atmosphere Ocean (TAO) Project Office of NOAA/PMEL are thankfully acknowledged. 7. References [1] P. J. Webster, A. M. Moore, J. P. Loschnigg and R. R. Leben, “Coupled Ocean-Atmosphere Dynamics in the In- dian Ocean during 1997-1998,” Nature, Vol. 401, 1999, pp. 356-360. doi:10.1038/43848 [2] L. Yu and R. A. Weller, “Objectively Analyzed Air-Sea Heat Fluxes for the Global Ice-Free Oceans (1981- 2005),” Bulletin of American Meteorological Society, Vol. 88, 2007, pp. 527-539. doi:10.1175/BAMS-88-4-527 [3] A. Bentamy, K. B. Katsaros, A. M. Mestas-Nunez, W. M. Drennan, E. B. Forde and H. Roquet, “Satellite Estimates of Wind Speed and Latent Heat Flux over the Global Oceans,” Journal of Climate, Vol. 16, 2003, pp. 637-656. doi:10.1175/1520-0442(2003)016<0637:SEOWSA>2.0. CO;2 [4] P. Schlussel and A. Albert, “Latent Heat Flux at the Sea Surface Retrieved from Combined TMI and VIRS Meas- urements of TRMM,” International Journal of Remote Sensing, Vol. 22, No. 10, 2001, pp. 1975-1998. [5] C. W. Fairall, E. F. Bradley, D. P. Rogers, J. B. Edson and G. S. Young, “Bulk Parameterization of Air-Sea Fluxes for Tropical Ocean-Global Atmosphere Cou- pled-Ocean Atmosphere Research Experiment,” Journal of Geophysical Research, Vol. 101, No. C2, 1996, pp. 3747-3764. doi:10.1029/95JC03205 [6] R. M. Gairola, N. Gautam and P. C. Pandey, “Study of Latent Heat Flues and Associated Oceanic and Atmos- pheric Parameters over the Arabian Sea and Bay of Ben- gal Using Seasat-SMMR Data,” Indian Journal of Radio and Space Physics, Vol. 21, 1992, pp. 143-148. [7] B. Simon and P. S. Desai, “Equatorial Indian Ocean Evaporation Estimates from Operational Meteorological Satellites and Some Inferences in the Context of Mon- soon Onset and Activity,” Boundary-Layer Meteorology, Vol. 37, 1986, pp. 37-52. doi:10.1007/BF00122755 [8] W. T. Liu, K. B. Katsaros and J. A. Businger, “Bulk Parameterization of Air-Sea Exchanges of Heat and Wa- ter Vapor Including the Molecular Constraints at the In- terface,” Journal of Atmospheric Sciences, Vol. 36, 1979, pp. 1722-1735. doi:10.1175/1520-0469(1979)036<1722:BPOASE>2.0.C O;2 [9] R. Singh, C. M. Kishtawal, P. K. Pal and P. C. Joshi, “Surface Heat Fluxes over Global Oceans Exclusively Copyright © 2011 SciRes. ACS
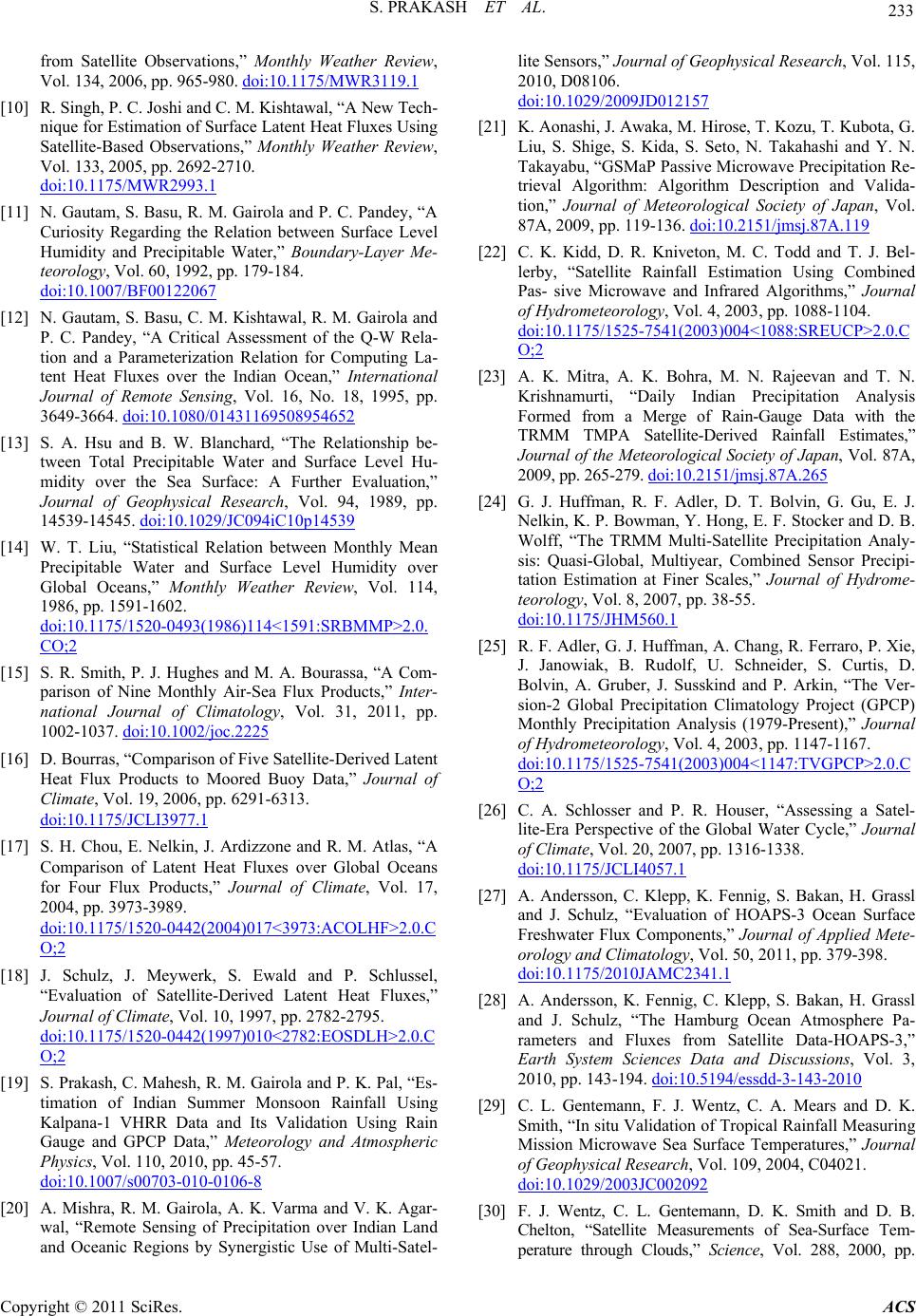 233 S. PRAKASH ET AL. from Satellite Observations,” Monthly Weather Review, Vol. 134, 2006, pp. 965-980. doi:10.1175/MWR3119.1 [10] R. Singh, P. C. Joshi and C. M. Kishtawal, “A New Tech- nique for Estimation of Surface Latent Heat Fluxes Using Satellite-Based Observations,” Monthly Weather Review, Vol. 133, 2005, pp. 2692-2710. doi:10.1175/MWR2993.1 [11] N. Gautam, S. Basu, R. M. Gairola and P. C. Pandey, “A Curiosity Regarding the Relation between Surface Level Humidity and Precipitable Water,” Boundary-Layer Me- teorology, Vol. 60, 1992, pp. 179-184. doi:10.1007/BF00122067 [12] N. Gautam, S. Basu, C. M. Kishtawal, R. M. Gairola and P. C. Pandey, “A Critical Assessment of the Q-W Rela- tion and a Parameterization Relation for Computing La- tent Heat Fluxes over the Indian Ocean,” International Journal of Remote Sensing, Vol. 16, No. 18, 1995, pp. 3649-3664. doi:10.1080/01431169508954652 [13] S. A. Hsu and B. W. Blanchard, “The Relationship be- tween Total Precipitable Water and Surface Level Hu- midity over the Sea Surface: A Further Evaluation,” Journal of Geophysical Research, Vol. 94, 1989, pp. 14539-14545. doi:10.1029/JC094iC10p14539 [14] W. T. Liu, “Statistical Relation between Monthly Mean Precipitable Water and Surface Level Humidity over Global Oceans,” Monthly Weather Review, Vol. 114, 1986, pp. 1591-1602. doi:10.1175/1520-0493(1986)114<1591:SRBMMP>2.0. CO;2 [15] S. R. Smith, P. J. Hughes and M. A. Bourassa, “A Com- parison of Nine Monthly Air-Sea Flux Products,” Inter- national Journal of Climatology, Vol. 31, 2011, pp. 1002-1037. doi:10.1002/joc.2225 [16] D. Bourras, “Comparison of Five Satellite-Derived Latent Heat Flux Products to Moored Buoy Data,” Journal of Climate, Vol. 19, 2006, pp. 6291-6313. doi:10.1175/JCLI3977.1 [17] S. H. Chou, E. Nelkin, J. Ardizzone and R. M. Atlas, “A Comparison of Latent Heat Fluxes over Global Oceans for Four Flux Products,” Journal of Climate, Vol. 17, 2004, pp. 3973-3989. doi:10.1175/1520-0442(2004)017<3973:ACOLHF>2.0.C O;2 [18] J. Schulz, J. Meywerk, S. Ewald and P. Schlussel, “Evaluation of Satellite-Derived Latent Heat Fluxes,” Journal of Climate, Vol. 10, 1997, pp. 2782-2795. doi:10.1175/1520-0442(1997)010<2782:EOSDLH>2.0.C O;2 [19] S. Prakash, C. Mahesh, R. M. Gairola and P. K. Pal, “Es- timation of Indian Summer Monsoon Rainfall Using Kalpana-1 VHRR Data and Its Validation Using Rain Gauge and GPCP Data,” Meteorology and Atmospheric Physics, Vol. 110, 2010, pp. 45-57. doi:10.1007/s00703-010-0106-8 [20] A. Mishra, R. M. Gairola, A. K. Varma and V. K. Agar- wal, “Remote Sensing of Precipitation over Indian Land and Oceanic Regions by Synergistic Use of Multi-Satel- lite Sensors,” Journal of Geophysical Research, Vol. 115, 2010, D08106. doi:10.1029/2009JD012157 [21] K. Aonashi, J. Awaka, M. Hirose, T. Kozu, T. Kubota, G. Liu, S. Shige, S. Kida, S. Seto, N. Takahashi and Y. N. Takayabu, “GSMaP Passive Microwave Precipitation Re- trieval Algorithm: Algorithm Description and Valida- tion,” Journal of Meteorological Society of Japan, Vol. 87A, 2009, pp. 119-136. doi:10.2151/jmsj.87A.119 [22] C. K. Kidd, D. R. Kniveton, M. C. Todd and T. J. Bel- lerby, “Satellite Rainfall Estimation Using Combined Pas- sive Microwave and Infrared Algorithms,” Journal of Hydrometeorology, Vol. 4, 2003, pp. 1088-1104. doi:10.1175/1525-7541(2003)004<1088:SREUCP>2.0.C O;2 [23] A. K. Mitra, A. K. Bohra, M. N. Rajeevan and T. N. Krishnamurti, “Daily Indian Precipitation Analysis Formed from a Merge of Rain-Gauge Data with the TRMM TMPA Satellite-Derived Rainfall Estimates,” Journal of the Meteorological Society of Japan, Vol. 87A, 2009, pp. 265-279. doi:10.2151/jmsj.87A.265 [24] G. J. Huffman, R. F. Adler, D. T. Bolvin, G. Gu, E. J. Nelkin, K. P. Bowman, Y. Hong, E. F. Stocker and D. B. Wolff, “The TRMM Multi-Satellite Precipitation Analy- sis: Quasi-Global, Multiyear, Combined Sensor Precipi- tation Estimation at Finer Scales,” Journal of Hydrome- teorology, Vol. 8, 2007, pp. 38-55. doi:10.1175/JHM560.1 [25] R. F. Adler, G. J. Huffman, A. Chang, R. Ferraro, P. Xie, J. Janowiak, B. Rudolf, U. Schneider, S. Curtis, D. Bolvin, A. Gruber, J. Susskind and P. Arkin, “The Ver- sion-2 Global Precipitation Climatology Project (GPCP) Monthly Precipitation Analysis (1979-Present),” Journal of Hydrometeorology, Vol. 4, 2003, pp. 1147-1167. doi:10.1175/1525-7541(2003)004<1147:TVGPCP>2.0.C O;2 [26] C. A. Schlosser and P. R. Houser, “Assessing a Satel- lite-Era Perspective of the Global Water Cycle,” Journal of Climate, Vol. 20, 2007, pp. 1316-1338. doi:10.1175/JCLI4057.1 [27] A. Andersson, C. Klepp, K. Fennig, S. Bakan, H. Grassl and J. Schulz, “Evaluation of HOAPS-3 Ocean Surface Freshwater Flux Components,” Journal of Applied Mete- orology and Climatology, Vol. 50, 2011, pp. 379-398. doi:10.1175/2010JAMC2341.1 [28] A. Andersson, K. Fennig, C. Klepp, S. Bakan, H. Grassl and J. Schulz, “The Hamburg Ocean Atmosphere Pa- rameters and Fluxes from Satellite Data-HOAPS-3,” Earth System Sciences Data and Discussions, Vol. 3, 2010, pp. 143-194. doi:10.5194/essdd-3-143-2010 [29] C. L. Gentemann, F. J. Wentz, C. A. Mears and D. K. Smith, “In situ Validation of Tropical Rainfall Measuring Mission Microwave Sea Surface Temperatures,” Journal of Geophysical Research, Vol. 109, 2004, C04021. doi:10.1029/2003JC002092 [30] F. J. Wentz, C. L. Gentemann, D. K. Smith and D. B. Chelton, “Satellite Measurements of Sea-Surface Tem- perature through Clouds,” Science, Vol. 288, 2000, pp. Copyright © 2011 SciRes. ACS
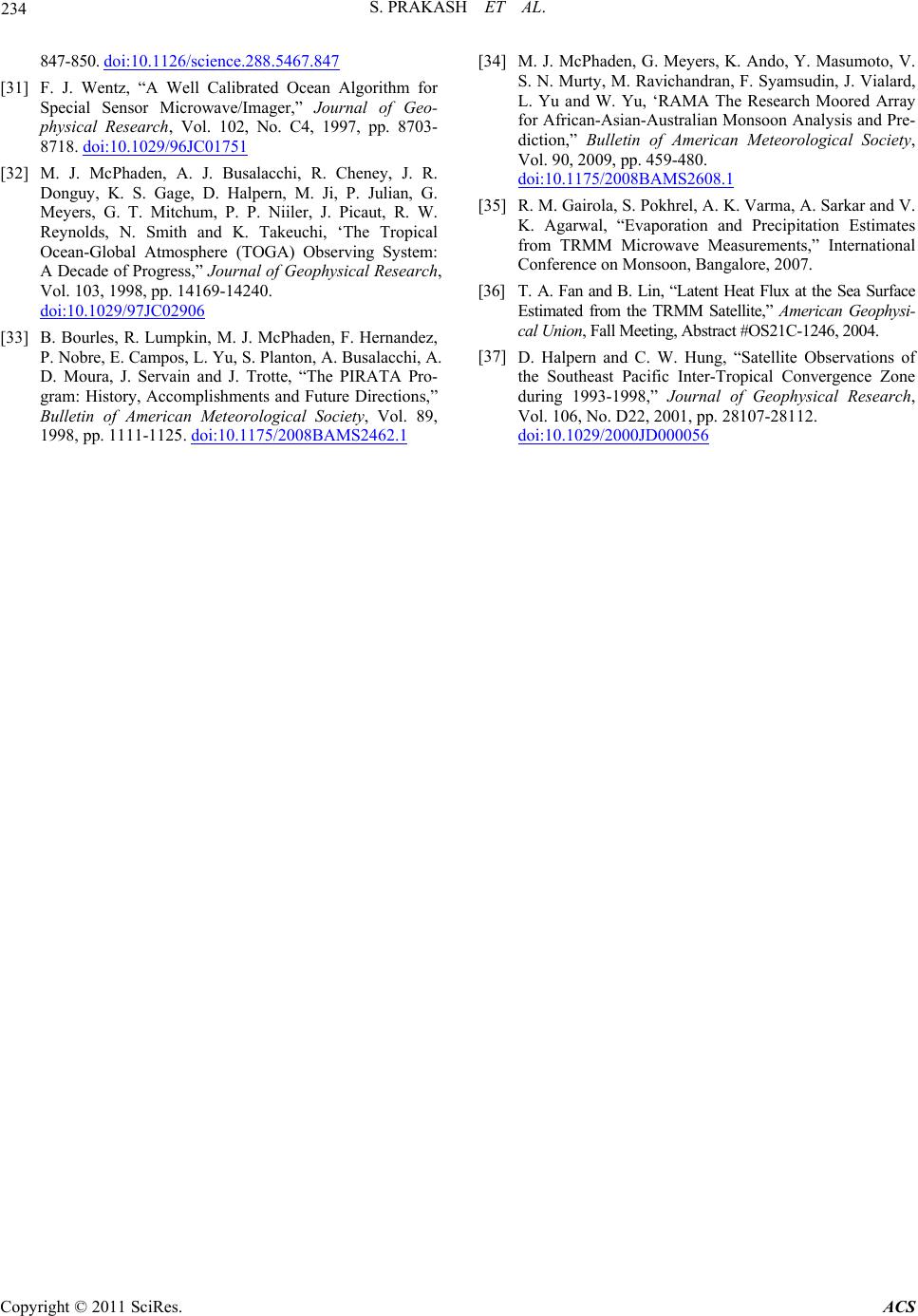 S. PRAKASH ET AL. Copyright © 2011 SciRes. ACS 234 847-850. doi:10.1126/science.288.5467.847 [31] F. J. Wentz, “A Well Calibrated Ocean Algorithm for Special Sensor Microwave/Imager,” Journal of Geo- physical Research, Vol. 102, No. C4, 1997, pp. 8703- 8718. doi:10.1029/96JC01751 [32] M. J. McPhaden, A. J. Busalacchi, R. Cheney, J. R. Donguy, K. S. Gage, D. Halpern, M. Ji, P. Julian, G. Meyers, G. T. Mitchum, P. P. Niiler, J. Picaut, R. W. Reynolds, N. Smith and K. Takeuchi, ‘The Tropical Ocean-Global Atmosphere (TOGA) Observing System: A Decade of Progress,” Journal of Geophysical Research, Vol. 103, 1998, pp. 14169-14240. doi:10.1029/97JC02906 [33] B. Bourles, R. Lumpkin, M. J. McPhaden, F. Hernandez, P. Nobre, E. Campos, L. Yu, S. Planton, A. Busalacchi, A. D. Moura, J. Servain and J. Trotte, “The PIRATA Pro- gram: History, Accomplishments and Future Directions,” Bulletin of American Meteorological Society, Vol. 89, 1998, pp. 1111-1125. doi:10.1175/2008BAMS2462.1 [34] M. J. McPhaden, G. Meyers, K. Ando, Y. Masumoto, V. S. N. Murty, M. Ravichandran, F. Syamsudin, J. Vialard, L. Yu and W. Yu, ‘RAMA The Research Moored Array for African-Asian-Australian Monsoon Analysis and Pre- diction,” Bulletin of American Meteorological Society, Vol. 90, 2009, pp. 459-480. doi:10.1175/2008BAMS2608.1 [35] R. M. Gairola, S. Pokhrel, A. K. Varma, A. Sarkar and V. K. Agarwal, “Evaporation and Precipitation Estimates from TRMM Microwave Measurements,” International Conference on Monsoon, Bangalore, 2007. [36] T. A. Fan and B. Lin, “Latent Heat Flux at the Sea Surface Estimated from the TRMM Satellite,” American Geophysi- cal Union, Fall Meeting, Abstract #OS21C-1246, 2004. [37] D. Halpern and C. W. Hung, “Satellite Observations of the Southeast Pacific Inter-Tropical Convergence Zone during 1993-1998,” Journal of Geophysical Research, Vol. 106, No. D22, 2001, pp. 28107-28112. doi:10.1029/2000JD000056
|