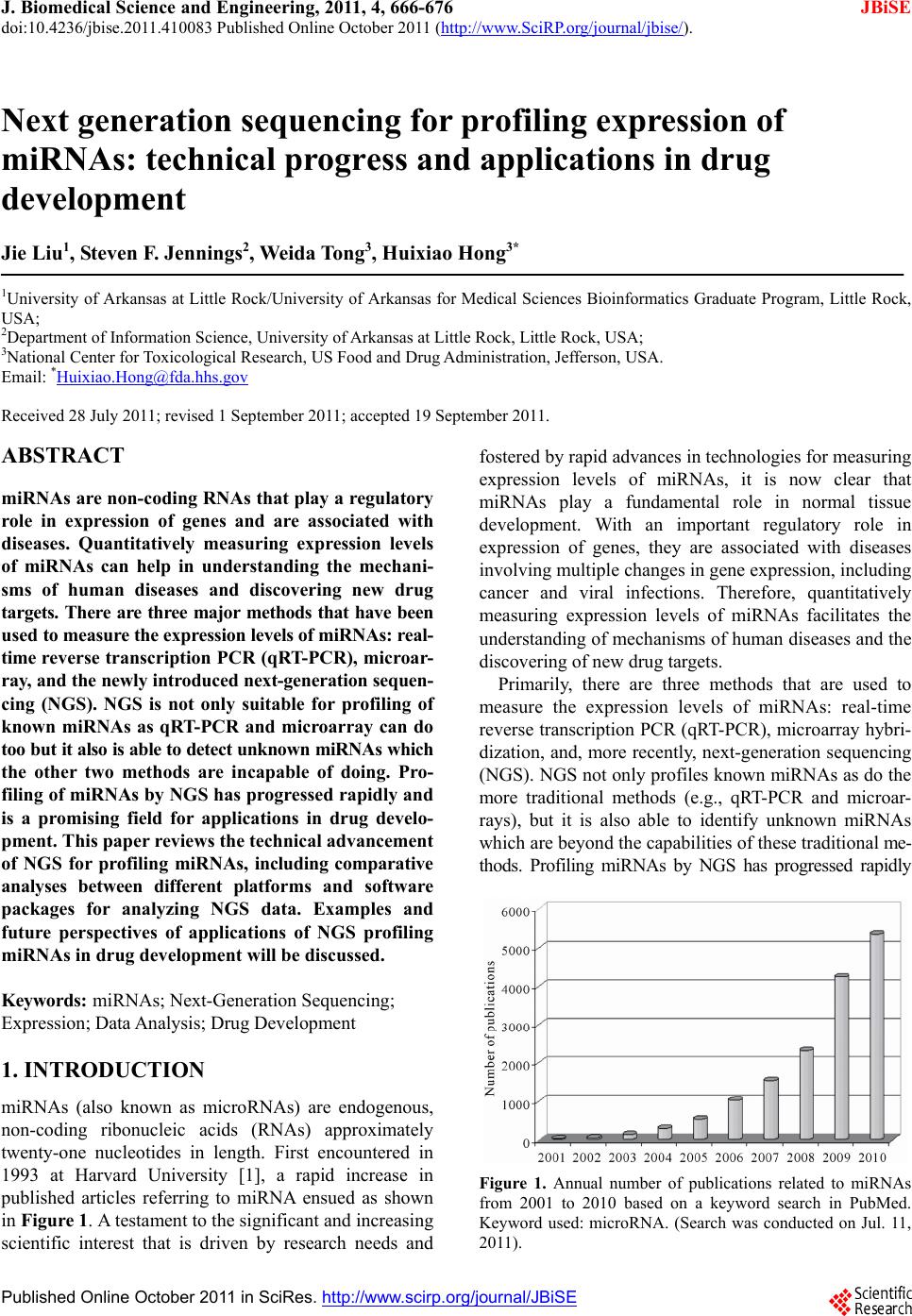 J. Biomedical Science and Engineering, 2011, 4, 666-676 doi:10.4236/jbise.2011.410083 Published Online October 2011 (http://www.SciRP.org/journal/jbise/ JBiSE ). Published Online October 2011 in SciRes. http://www.scirp.org/journal/JBiSE Next generation sequencing for profiling expression of miRNAs: technical progress and applications in drug development Jie Liu1, Steven F. Jennings2, Weida Tong3, Huixiao Hong3* 1University of Arkansas at Little Rock/University of Arkansas for Medical Sciences Bioinformatics Graduate Program, Little Rock, USA; 2Department of Information Science, University of Arkansas at Little Rock, Little Rock, USA; 3National Center for Toxicological Research, US Food and Drug Administration, Jefferson, USA. Email: *Huixiao.Hong@fda.hhs.gov Received 28 July 2011; revised 1 September 2011; accepted 19 September 2011. ABSTRACT miRNAs are non-coding RNAs that play a regulatory role in expression of genes and are associated with diseases. Quantitatively measuring expression levels of miRNAs can help in understanding the mechani- sms of human diseases and discovering new drug targets. There are three major methods that have been used to measure the expression levels of miRNAs: real- time reverse transcription PCR (qRT-PCR), microar- ray, and the newly introduced next-generation sequen- cing (NGS). NGS is not only suitable for profiling of known miRNAs as qRT-PCR and microarray can do too but it also is able to detect unkno wn miRNAs which the other two methods are incapable of doing. Pro- filing of miRNAs by NGS has progressed rapidly and is a promising field for applications in drug develo- pment. This paper reviews the technical advancement of NGS for profiling miRNAs, including comparative analyses between different platforms and software packages for analyzing NGS data. Examples and future perspectives of applications of NGS profiling miRNAs in drug development will be discussed. Keywords: miRNAs; Next-Generation Sequencing; Expression; Data Analysis; Drug Development 1. INTRODUCTION miRNAs (also known as microRNAs) are endogenous, non-coding ribonucleic acids (RNAs) approximately twenty-one nucleotides in length. First encountered in 1993 at Harvard University [1], a rapid increase in published articles referring to miRNA ensued as shown in Figure 1. A testament to the significant and increasing scientific interest that is driven by research needs and fostered by rapid advances in technologies for measuring expression levels of miRNAs, it is now clear that miRNAs play a fundamental role in normal tissue development. With an important regulatory role in expression of genes, they are associated with diseases involving multiple changes in gene expression, including cancer and viral infections. Therefore, quantitatively measuring expression levels of miRNAs facilitates the understanding of mechanisms of human diseases and the discovering of new drug targets. Primarily, there are three methods that are used to measure the expression levels of miRNAs: real-time reverse transcription PCR (qRT-PCR), microarray hybri- dization, and, more recently, next-generation sequencing (NGS). NGS not only profiles known miRNAs as do the more traditional methods (e.g., qRT-PCR and microar- rays), but it is also able to identify unknown miRNAs which are beyond the capabilities of these traditional me- thods. Profiling miRNAs by NGS has progressed rapidly Figure 1. Annual number of publications related to miRNAs from 2001 to 2010 based on a keyword search in PubMed. Keyword used: microRNA. (Search was conducted on Jul. 11, 2011).
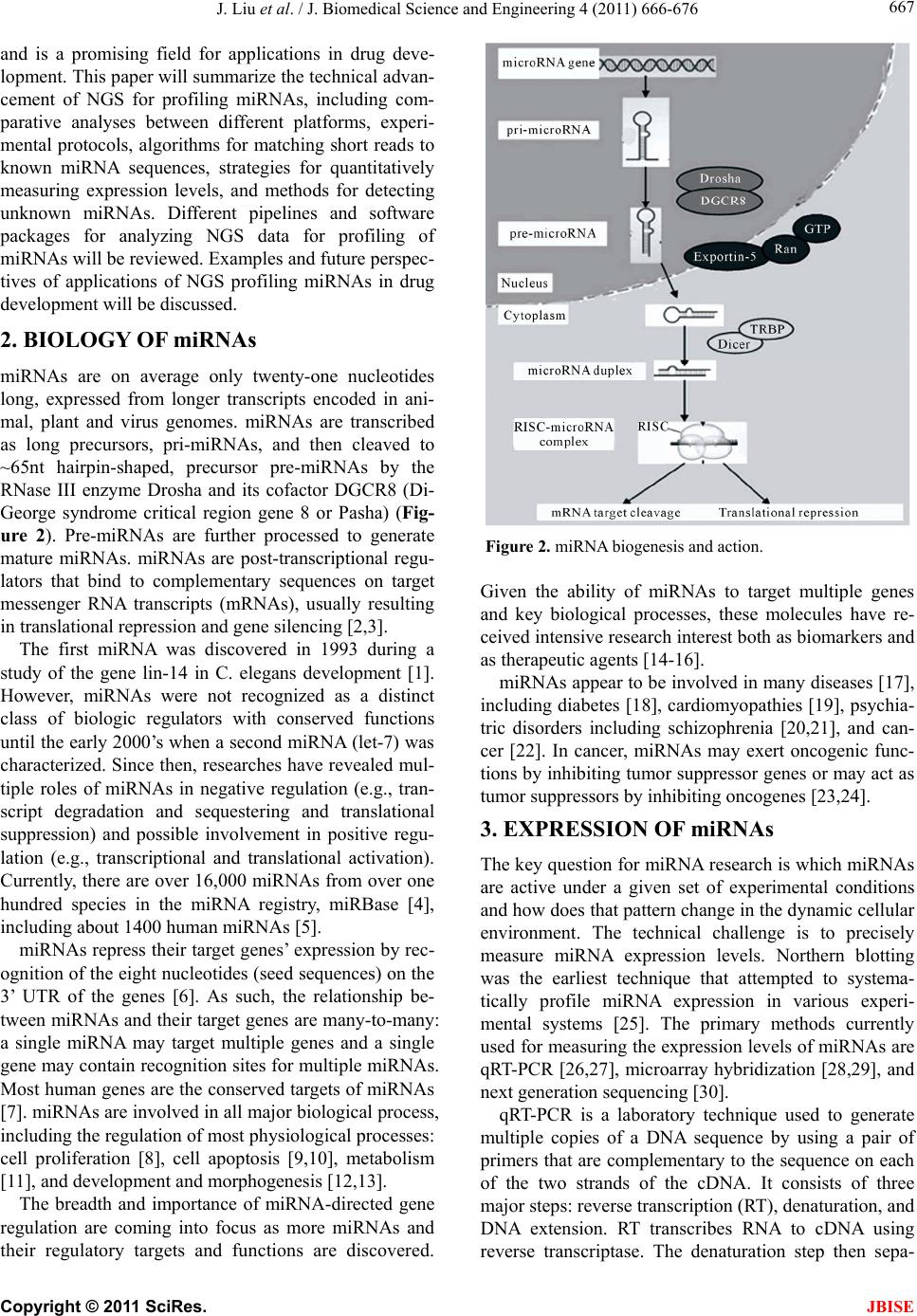 J. Liu et al. / J. Biomedical Science and Engineering 4 (2011) 666-676 667 and is a promising field for applications in drug deve- lopment. This paper will summarize the technical advan- cement of NGS for profiling miRNAs, including com- parative analyses between different platforms, experi- mental protocols, algorithms for matching short reads to known miRNA sequences, strategies for quantitatively measuring expression levels, and methods for detecting unknown miRNAs. Different pipelines and software packages for analyzing NGS data for profiling of miRNAs will be reviewed. Examples and future perspec- tives of applications of NGS profiling miRNAs in drug development will be discussed. 2. BIOLOGY OF miRNAs miRNAs are on average only twenty-one nucleotides long, expressed from longer transcripts encoded in ani- mal, plant and virus genomes. miRNAs are transcribed as long precursors, pri-miRNAs, and then cleaved to ~65nt hairpin-shaped, precursor pre-miRNAs by the RNase III enzyme Drosha and its cofactor DGCR8 (Di- George syndrome critical region gene 8 or Pasha) (Fig- ure 2). Pre-miRNAs are further processed to generate mature miRNAs. miRNAs are post-transcriptional regu- lators that bind to complementary sequences on target messenger RNA transcripts (mRNAs), usually resulting in translational repression and gene silencing [2,3]. The first miRNA was discovered in 1993 during a study of the gene lin-14 in C. elegans development [1]. However, miRNAs were not recognized as a distinct class of biologic regulators with conserved functions until the early 2000’s when a second miRNA (let-7) was characterized. Since then, researches have revealed mul- tiple roles of miRNAs in negative regulation (e.g., tran- script degradation and sequestering and translational suppression) and possible involvement in positive regu- lation (e.g., transcriptional and translational activation). Currently, there are over 16,000 miRNAs from over one hundred species in the miRNA registry, miRBase [4], including about 1400 human miRNAs [5]. miRNAs repress their target genes’ expression by rec- ognition of the eight nucleotides (seed sequences) on the 3’ UTR of the genes [6]. As such, the relationship be- tween miRNAs and their target genes are many-to-many: a single miRNA may target multiple genes and a single gene may contain recognition sites for multiple miRNAs. Most human genes are the conserved targets of miRNAs [7]. miRNAs are involved in all major biological process, including the regulation of most physiological processes: cell proliferation [8], cell apoptosis [9,10], metabolism [11], and development and morphogenesis [12,13]. The breadth and importance of miRNA-directed gene regulation are coming into focus as more miRNAs and their regulatory targets and functions are discovered. Figure 2. miRNA biogenesis and action. Given the ability of miRNAs to target multiple genes and key biological processes, these molecules have re- ceived intensive research interest both as biomarkers and as therapeutic agents [14-16]. miRNAs appear to be involved in many diseases [17], including diabetes [18], cardiomyopathies [19], psychia- tric disorders including schizophrenia [20,21], and can- cer [22]. In cancer, miRNAs may exert oncogenic func- tions by inhibiting tumor suppressor genes or may act as tumor suppressors by inhibiting oncogenes [23,24]. 3. EXPRESSION OF miRNAs The key question for miRNA research is which miRNAs are active under a given set of experimental conditions and how does that pattern change in the dynamic cellular environment. The technical challenge is to precisely measure miRNA expression levels. Northern blotting was the earliest technique that attempted to systema- tically profile miRNA expression in various experi- mental systems [25]. The primary methods currently used for measuring the expression levels of miRNAs are qRT-PCR [26,27], microarray hybridization [28,29], and next generation sequencing [30]. qRT-PCR is a laboratory technique used to generate multiple copies of a DNA sequence by using a pair of primers that are complementary to the sequence on each of the two strands of the cDNA. It consists of three major steps: reverse transcription (RT), denaturation, and DNA extension. RT transcribes RNA to cDNA using reverse transcriptase. The denaturation step then sepa- C opyright © 2011 SciRes. JBISE
 J. Liu et al. / J. Biomedical Science and Engineering 4 (2011) 666-676 668 Table 1. Some popular miRNA microarray platforms. Company Platform miRBase Species Agilent Human miRNA Microarray 8x60K 16.0 (2010) Human Agilent Mouse miRNA Microarray 8x60K 16.0 (2010) Mouse Agilent Rat miRNA Microarray 8x15K 16.0 (2010) Rat Affymetrix GeneChip® miRNA 2.0 Array 15.0 (2010) multiple Illumina MicroRNA Expression Profiling Assay 12.0 (2008) multiple Exiqon miRCURY LNA™ microRNA Array 16.0 (2010) multiple Life Tech- nologies NCode™ Human miRNA Microarray V3 10.0 (2007) Human rates the strands and the primers can bind again at lower temperatures and begin a new chain reaction. Finally, DNA extension from the primers is done with thermo- stable Taq DNA polymerase. qRT-PCR is a sensitive me- thod for measuring expression levels of precursor or ma- ture miRNAs. It requires low amounts of starting mate- rial. With the design of RT primers with high specificity towards individual mature miRNA species, multiple primers could be potentially combined in a single pool that would enable much higher throughput profiling than is currently possible with individual sample analyses. qRT-PCR is suitable for quantification of a few known miRNAs in a large number of samples. miRNA microarray hybridization is a popular method for measuring miRNA expression levels because a large number of miRNAs can be measured simultaneously [29]. Several companies offer miRNA microarray plat- forms which are designed and developed based on the same miRNAs database, miRBase [4]. Therefore, the major difference among the miRNA microarray plat- forms is the miRNAs on the arrays. Because this field is evolving very rapidly, users should select a platform based on the version of miRBase which contains the most relevant information for their samples. Companies frequently update their contents to reflect a newer miRBase release. Table 1 lists some popular miRNA microarray platforms. 4. NGS Rapid determination of DNA sequence base pairs, first reported by Sanger [35,36], provided a tool to decipher genes. However, low throughput and—more impor- tantly—high cost hindered using this sequencing tech- nology for deciphering the human genome. A break- through came in 2005 when the sequencing-by—syn- thesis technology developed by 454 Life Sciences was published [37]. Since then, several NGS platforms, such as Illumina Genome Analyzer (Illumina, Inc., San Diego, CA, USA) and SOLiDTM (Life Technologies Corpora- tion, Carlsbad, CA, USA), have been developed and applied to various fields of biological and medical re- search, including measuring expression levels of known miRNAs and detecting unknown miRNAs. 4.1. NGS Platforms Different NGS platforms use divergent sequencing chemistries. Table 2 compares the main features of three NGS platforms, but it is important to note that these values are constantly changing as newer models are re- leased. Both Illumina Genome Analyzer system and SOLiD use short-read sequencing technologies. The Roche 454 Genome Sequencer has the advantage of longer sequence reads and is the best choice for denovo sequencing of new genomes. 4.1.1. Illumina Genome Analyzer The Illumina Genome Analyzer system currently is the most widely-used, short-read sequencing platform. It applies the sequencing-by-synthesis method. miRNAs are first reversely translated to DNA and the DNA sam- ples are randomly sheared into fragments, then ligated to oligonucleotide adapters at both ends. Single-stranded DNA fragments are attached to reaction chambers and are extended and amplified by bridge PCR amplification with fluorescently-labeled nucleotides for sequencing. The Genome Analyzer is widely used by many genome sequencing projects for its consistent data quality and proper read lengths. The new Illumina HiSeq 2000 has dramatically increased throughput. 4.1.2. Roche 454 Genome Sequencer FL X The 454 Genome Sequencer uses the principle of pyro- Table 2. Comparison of NGS platforms. Platform Illumina Roche 454 SOLiD Sequencing- by-synthesis Pyrosequencing Ligation-based sequencing ApplificationBridge PCR Emulsion PCR Emulsion PCR Read length35 - 150 bp ~400 bp 75/35 bp Paired ends/ separation Yes/200 bp Yes/3000 bp Yes/3000 bp Mb/run 1300 Mb 100 Mb 3000 Mb Time/run (paired ends)4 days 7 hours 5 days Comments Most widely used Longer reads, fast run, higher cost Good data quality C opyright © 2011 SciRes. JBISE
 J. Liu et al. / J. Biomedical Science and Engineering 4 (2011) 666-676 669 sequencing. Sheared DNA fragments are ligated to speci- fic oligonucleotide adapters and are amplified by emul- sion PCR on the surfaces of agarose beads. The current maximum read length of the 454 platform is 600 bp which is the longest short-read among all of the NGS plat- forms. Thus, the 454 Genome Sequencer FLX is best suited for applications requiring longer reads, such as RNA isoform identification in RNA-seq and de novo assembly of microbes in metagenomics [38]. 4.1.3. Applied Biosystems SOLiD Sequencer The Applied Biosystems SOLiD sequencer uses the sequencing-by-ligation approach and may offer the best data quality. It amplifies sheared DNA fragments by an emulsion PCR approach with small magnetic beads. But the DNA library preparation procedures prior to sequenc- ing currently take five days which is both tedious and time consuming. 4.2. Sequence Alignment A NGS experiment generates huge amount of sequence data. Consequently, there is a high demand for bioin- formatics tools to cope with these large amounts of sequencing data. The key process in NGS data analysis is to align the huge amount of short reads to a given genome. A variety of algorithms and software packages have been specifically developed for dealing with mil- lions of NGS short-read alignments (Table 3). Bowtie (http://bowtie.cbcb.umd.edu/) is an ultrafast and efficient alignment program for aligning short sequences to large genomes [39]. Bowtie indexes the re- ference genome using a scheme based on the Burrows- Wheeler index to keep its memory footprint small. It does not use an exact matching algorithm, which is quite common in other tools, because exact matching does not directly allow for sequencing errors or genetic variations. The two key algorithmic strategies that make Bowtie ex- tremely fast in alignment of short reads are backtracking and double indexing. Backtracking allows mismatches and favors high-quality alignments, while the double in- Table 3. Short-read sequence alignment tools. Name Description Bowtie Uses a Burrows-Wheeler transform to create a per- manent, reusable index of the genome; faster run for short sequence alignment to reference genome BWA Slower than bowtie but allows indels in alignment MAQ performs only ungapped alignments and allows up to three mismatches SeqMap Up to 5 mixed substitutions and insertions/deletions. Various tuning options and input/output formats. SOAP Allow up to 3 gaps and mismatches. SOAP2 uses bidirectional BWT to build the index of reference and increases the running speed. TopHat Splice junction mapper for RNA-Seq reads dexing avoids excessive backtracking. BWA (http://maq.sourceforge.net/) is a software pack- age for aligning short sequencing reads against a large reference sequence such as the human genome [40]. This alignment algorithm is based on a backward search with the Burrows-Wheeler Transform (BWT) of the reference genome. It allows mismatches and gaps for single-end reads. BWA also supports paired-end mapping. It ge- nerates a mapping quality index and gives multiple hits if requested. MAQ (http://maq.sourceforge.net/) is a software pack- age for rapidly mapping shotgun short reads to a re- ference genome and using quality scores to derive geno- type calls of the consensus sequence of a diploid genome [41]. MAQ searches for the ungapped match with lowest mismatch score. For each alignment, a quality score is assigned to measure the probability that the true align- ment is not the one found by MAQ. Using MAQ, users can map reads, call consensus sequences including single nucleotide polymorphisms (SNPs) and indel variants, simulate diploid genomes and read sequences, and post- process the results in various ways. SeqMap (http://www.stanford.edu/group/wonglab/jiangh/ seqmap/) is a tool for aligning a large number of short sequences to a reference genome [42]. It explores the whole reference genome for each short read se- quence from NGS data. Multiple substitutions and inser- tions/deletions are allowed in SeqMap. It accepts FASTA input format and output results in various formats. Parallel computing with SeqMap on a cluster of com- puters is supported. This program is fast, usually taking just a few hours on a desktop PC for a typical alignment of NGS data. SOAP (http://soap.genomics.org.cn/) is a program for efficient gapped and ungapped alignment of short read sequences onto reference sequences [43]. It adopts a seed-and-hash look-up table algorithm to accelerate its alignment process. First, short reads and the reference sequences are converted to a numeric data type using a 2-bits-per-base encoding system. Then the look-up table is checked to determine how many bases are different between a short read and a reference sequence. SOAP is a command-driven program and supports multi-threaded, parallel computing. It accepts FASTA format for refer- ence and both FASTA and FASTQ formats for input short reads. With SOAP, users can do single-read or pair- end resequencing, small RNA discovery, and mRNA tag sequence mapping. TopHat (http://tophat.cbcb.umd.edu/) is designed to align RNA-Seq reads and to create a view of the junctions, or to align to a known set of junctions [44]. The TopHat pipeline consists of multiple steps. Initially, all the short reads are aligned to the reference genome C opyright © 2011 SciRes. JBISE
 J. Liu et al. / J. Biomedical Science and Engineering 4 (2011) 666-676 670 using Bo wtie . The short reads that have not been aligned to the genome are set aside as “initially unmapped reads”. The aligned short reads are then assembled to generate possible splices between neighboring exons se- quences flanking potential donor/acceptor splice sites with neighboring exons joined together to generate po- tential splice junctions. Then, the “initially unmapped reads” are aligned with these potential splice junction sequences. 4.3. NGS for Profiling miRNAs 4.3.1. miRNA Databases NGS short reads are aligned to a known reference sequence database. These miRNA databases are the repo- sitory for known miRNA sequence and annotation data. They are the core of NGS data analysis for expression profiling of miRNAs. The most important and popular miRNA databases include, but not limited to: miRBase [4], deepBase [45], microRNA.org [46], miRGen 2.0 [47], miRNAMap [48], and PMRD [49]. The miRBase database (http://www.mirbase.org) is an online database repository for known miRNAs. It pro- vides an integrated web interface to analyze miRNA sequence data and to predict gene targets. The miRBase database has three main functions: 1) the miRBase Registry acts as an independent arbiter of miRNA gene nomenclature, assigning names prior to publication of novel miRNA sequences; 2) the miRBase Sequences provides known miRNA sequence data, references, and links to other resources; and 3) the miRBase Targets is used for the prediction of miRNA target genes. The deepBase (http://deepbase.sysu.edu.cn/) is a com- prehensive web-based database for annotating and dis- covering small and long non-coding RNAs, ncRNAs (miRNAs, siRNAs, piRNAs, etc.), from high-throughput deep sequencing data. In the current version of deepBase, NGS data from 185 small RNA libraries from diverse tissues and cell lines of seven organisms (human, mouse, chicken, Ciona intestinalis, Drosophila melanogaster, Caenhorhabditis elegans, and Arabidopsis thaliana) have been curated. Its integrative, interactive, and versatile web graphical interface facilitates analyzing and visua- lizing NGS data on the internet. The microRNA.org (http://www.microrna.org/ microrna/ home.do) is a database for experimentally-observed miRNA expression patterns and predicted miRNA targets and target downregulation scores. Through its graphical interface, the microRNA.org web resource provides several functions: 1) exploring genes that are potentially regulated by a particular miRNA; 2) searching for the set of miRNAs that potentially regulate a particular gene cooperatively; and 3) comparing miRNA expression profiles in different tissues. The strategy for miRNA target prediction is to treat miRNAs as adaptors of genes in the 3’-UTR region by using near-prefect, base-pairing in a small region in the 5’ end (positions 2-8) of the miRNA. The most valuable information of this web resource is the experimental data that can be used to verify miRNA regulation. The miRGen 2.0 (http://diana.cslab.ece.ntua.gr/ mirgen/) is a database of miRNA genomic information and regu- lation. It contains 812 human miRNA coding transcripts and 386 mouse miRNA coding transcripts as well as expression profiles of 548 human and 451 mouse miRNAs and over 172 human and 68 mouse small RNA libraries derived from cell lines and tissues. It is imple- mented in a MySQL relational database management system. Its interface allows users to search for miRNAs and transcription factors of interest. The miRNAMap (http://mirnamap.mbc.nctu.edu.tw/) provides genomic maps of miRNAs and their target genes in mammalian genomes. Experimentally-verified miRNAs and experimentally-verified miRNA target genes in human, mouse, rat, and other metazoan geno- mes are collected in this database. Target genes of miRNAs are predicted using three bioinformatics tools: miRanda [50], TargetScan [51], and RNAhybrid [52] independently. To reduce false positives for predicted target genes, three strategies were used: 1) a predicted target site is one that is predicted by at least two of the three bioinformatics tools; 2) the target site must be within an accessible region of a gene; and 3) the target gene has to have multiple target sites. Its interface is well designed to facilitate access to the data and analyz- ing data as well as visualizing the data and associated analysis results. PMRD (http://bioinformatics.cau.edu.cn/PMRD/) is a plant miRNA database. This database contains 8433 miRNAs from 121 plant species and possible target genes for each miRNA with a predicted interaction site. The data in PMRD include available plant miRNA data deposited in the public database, the ones curated from literature, and miRNA profiling data generated in-house. 4.3.2. miRNA NGS Data Analysis Tools There are several web servers and standalone programs for analysis of miRNA expression profiling and novel miRNA discovery from NGS data. The web servers include miRanalyzer [53,54] and miRCat [55]. Examples of standalone analysis tools are miRDeep [56] and miRExpress [57]. miRanalyzer (http://web.bioinformatics.cicbiogune.es/ microRNA/) is a web server for identifying and analy- zing miRNA in deep-sequencing data. After inputting NGS data (a list of unique reads and their copy numbers) into the web server tool, users can conduct data analysis in three steps: 1) detect all known miRNA sequences C opyright © 2011 SciRes. JBISE
 J. Liu et al. / J. Biomedical Science and Engineering 4 (2011) 666-676 671 annotated in miRBase; 2) match against other libraries of transcribed sequences; and 3) predict new miRNAs. It accepts two different input file formats: 1) a tab-se- parated file with a row representing a short read se- quence and its count and 2) a multi-FASTA format file with the copy number of the unique short reads (read count) as the description in the header. To detect the ex- pression levels of known miRNAs—a major objective of many miRNAs studies—miRanalyzer aligns the short reads to the known miRNA sequences using the miRBase repository [4] which offers mature (the mature sequences of known miRNAs), mature-star (the sequence which pairs with the mature miRNA in the pre-miRNA secondary structure), and precursor miRNA sequences (sequence of the hairpin). Since a group of miRNAs that can be ali- gned with the same read normally belong to the same family, miRanalyzer reports these ambiguous matches, sta- ting all miRNAs where alignments were found. After known miRNAs are detected, their corresponding target genes (the genes predicted to be regulated by the detec- ted miRNA) are predicted and precalculated ontological analyses are given. For the remaining reads that are not aligned to the known miRNAs, miRanalyzer maps them to databases of transcribed sequences as miRNA, non- coding RNA, and (retro)-transposons. The mapping step is “perfect”, meaning that no mismatch is allowed in the mapping. In the last step—the most important task of miRNA NGS data analysis—detecting previously-un- reported miRNAs is conducted. A machine-learning ap- proach based on the random forest method [58] is used to detect new miRNAs in miRanalyzer. The miRCat (http://srna-tools.cmp.uea.ac.uk/) is a miRNA NGS data analysis tool that was developed for identification of mature miRNAs and their precursors. It takes a FASTA format file of small RNA reads as input and then maps them to a reference sequence database using PatMaN [59]. Analysis results from miRCat are output in three files: 1) a comma-separated text file with the details for predicted miRNA candidates; 2) the RNAfold output for candidate precursors; and 3) a FASTA format file of predicted mature miRNA se- quences. The miRCat program has been tested on several high-throughput plant sRNA datasets and showed high sensitivity and specificity. The miRDeep package (http://www.mdc-berlin.de/en/ research/research_teams/systems_biology_of_gene_regu latory_elements/projects/miRDeep/) is a stand-alone tool for identifying known and novel miRNAs from NGS data. The miRDeep program employs a probabilistic model of miRNA biogenesis to score the compatibility of the nucleotide position and frequency of sequenced RNA reads with the secondary structure of the miRNA precursor. The false positive rate and the sensitivity of its predictions are statistically controlled in miRDeep. There- fore, not only known and novel miRNAs can be detected from deep-sequencing data by using miRDeep, but also the quality of the detection results can be estimated. The key function of miRDeep is the detection of miRNAs by analyzing how sequenced RNAs are compatible with the way miRNA precursors are processed in the cell. It should be noted is that miRDeep is designed to detect miRNAs without cross-species comparisons. The miRExpress (http://miRExpress.mbc.nctu.edu. tw) software package is written in the C++ programming language and can be executed on 32- or 64-bit Linux machines. It was developed to extract miRNA expres- sion profiles from sequencing reads obtained by NGS technology. The data analysis pipeline of miRExpress consists of three steps. In the first step, the identical short reads are merged into a unique read with “a count of reads.” Each unique short read is also checked to deter- mine whether it contains a full or a partial adaptor sequence. In the second step, each unique short read is aligned with the sequences of known mature miRNAs in miRBase [4]. In the third step, expression levels of miRNAs are measured by computing the sum of read counts for each miRNA according to the alignment criteria (e.g., the length of the read equals the length of the miRNA sequence and the identity of the alignment is 100%). The cutoff of alignment identity can be set by users based on their requirements when using miR- Express. 5. APPLICATIONS OF NGS IN DRUG DEVELOPMENT Drug development is a complex and lengthy process (Figure 3) that starts from pharmaceutical target identi- fication and validation followed by lead identification and optimization (usually termed as drug discovery). The lead compounds are subsequently subjected to precli- nical tests and clinical trials of increasing levels of com- plexity (usually termed as drug development). Following the completion of all three phases of clinical trials, a pharmaceutical company analyzes all of the data and files a new drug application with the FDA. If the data on the new drug successfully demonstrate both safety and effectiveness in careful review, the FDA approves the new drug to be distributed on the market. For some drugs, the FDA requires additional trials (commonly called Phase IV) to evaluate long-term effects. This whole process (including discovery and development) for a new drug takes about ten to five years and costs around $1 - $2 billion [60,61]. In a relatively short time, high-throughput sequencing of small RNAs have provided a great potential for the profiling of known and novel small RNAs. Given the C opyright © 2011 SciRes. JBISE
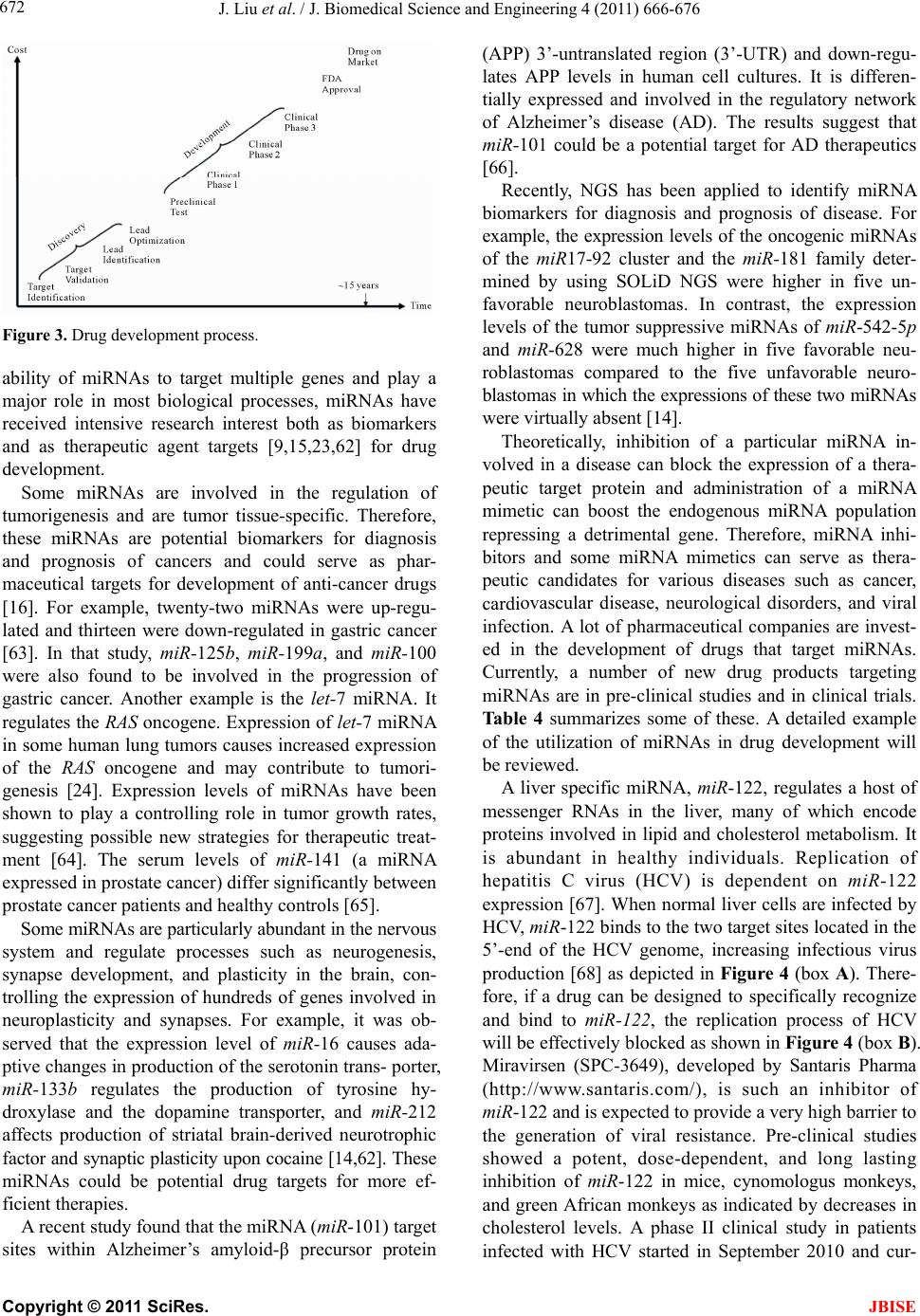 J. Liu et al. / J. Biomedical Science and Engineering 4 (2011) 666-676 672 Figure 3. Drug development process. ability of miRNAs to target multiple genes and play a major role in most biological processes, miRNAs have received intensive research interest both as biomarkers and as therapeutic agent targets [9,15,23,62] for drug development. Some miRNAs are involved in the regulation of tumorigenesis and are tumor tissue-specific. Therefore, these miRNAs are potential biomarkers for diagnosis and prognosis of cancers and could serve as phar- maceutical targets for development of anti-cancer drugs [16]. For example, twenty-two miRNAs were up-regu- lated and thirteen were down-regulated in gastric cancer [63]. In that study, miR-125b, miR-199a, and miR-100 were also found to be involved in the progression of gastric cancer. Another example is the let-7 miRNA. It regulates the RAS oncogene. Expression of let-7 miRNA in some human lung tumors causes increased expression of the RAS oncogene and may contribute to tumori- genesis [24]. Expression levels of miRNAs have been shown to play a controlling role in tumor growth rates, suggesting possible new strategies for therapeutic treat- ment [64]. The serum levels of miR-141 (a miRNA expressed in prostate cancer) differ significantly between prostate cancer patients and healthy controls [65]. Some miRNAs are particularly abundant in the nervous system and regulate processes such as neurogenesis, synapse development, and plasticity in the brain, con- trolling the expression of hundreds of genes involved in neuroplasticity and synapses. For example, it was ob- served that the expression level of miR-16 causes ada- ptive changes in production of the serotonin trans- porter, miR-133b regulates the production of tyrosine hy- droxylase and the dopamine transporter, and miR-212 affects production of striatal brain-derived neurotrophic factor and synaptic plasticity upon cocaine [14,62]. These miRNAs could be potential drug targets for more ef- ficient therapies. A recent study found that the miRNA (miR-101) target sites within Alzheimer’s amyloid-β precursor protein (APP) 3’-untranslated region (3’-UTR) and down-regu- lates APP levels in human cell cultures. It is differen- tially expressed and involved in the regulatory network of Alzheimer’s disease (AD). The results suggest that miR-101 could be a potential target for AD therapeutics [66]. Recently, NGS has been applied to identify miRNA biomarkers for diagnosis and prognosis of disease. For example, the expression levels of the oncogenic miRNAs of the miR17-92 cluster and the miR-181 family deter- mined by using SOLiD NGS were higher in five un- favorable neuroblastomas. In contrast, the expression levels of the tumor suppressive miRNAs of miR-542-5p and miR-628 were much higher in five favorable neu- roblastomas compared to the five unfavorable neuro- blastomas in which the expressions of these two miRNAs were virtually absent [14]. Theoretically, inhibition of a particular miRNA in- volved in a disease can block the expression of a thera- peutic target protein and administration of a miRNA mimetic can boost the endogenous miRNA population repressing a detrimental gene. Therefore, miRNA inhi- bitors and some miRNA mimetics can serve as thera- peutic candidates for various diseases such as cancer, cardiovascular disease, neurological disorders, and viral infection. A lot of pharmaceutical companies are invest- ed in the development of drugs that target miRNAs. Currently, a number of new drug products targeting miRNAs are in pre-clinical studies and in clinical trials. Ta b l e 4 summarizes some of these. A detailed example of the utilization of miRNAs in drug development will be reviewed. A liver specific miRNA, miR-122, regulates a host of messenger RNAs in the liver, many of which encode proteins involved in lipid and cholesterol metabolism. It is abundant in healthy individuals. Replication of hepatitis C virus (HCV) is dependent on miR-122 expression [67]. When normal liver cells are infected by HCV, miR-122 binds to the two target sites located in the 5’-end of the HCV genome, increasing infectious virus production [68] as depicted in Figure 4 (box A). There- fore, if a drug can be designed to specifically recognize and bind to miR-122, the replication process of HCV will be effectively blocked as shown in Figure 4 (box B). Miravirsen (SPC-3649), developed by Santaris Pharma (http://www.santaris.com/), is such an inhibitor of miR-122 and is expected to provide a very high barrier to the generation of viral resistance. Pre-clinical studies showed a potent, dose-dependent, and long lasting inhibition of miR-122 in mice, cynomologus monkeys, and green African monkeys as indicated by decreases in cholesterol levels. A phase II clinical study in patients infected with HCV started in September 2010 and cur- C opyright © 2011 SciRes. JBISE
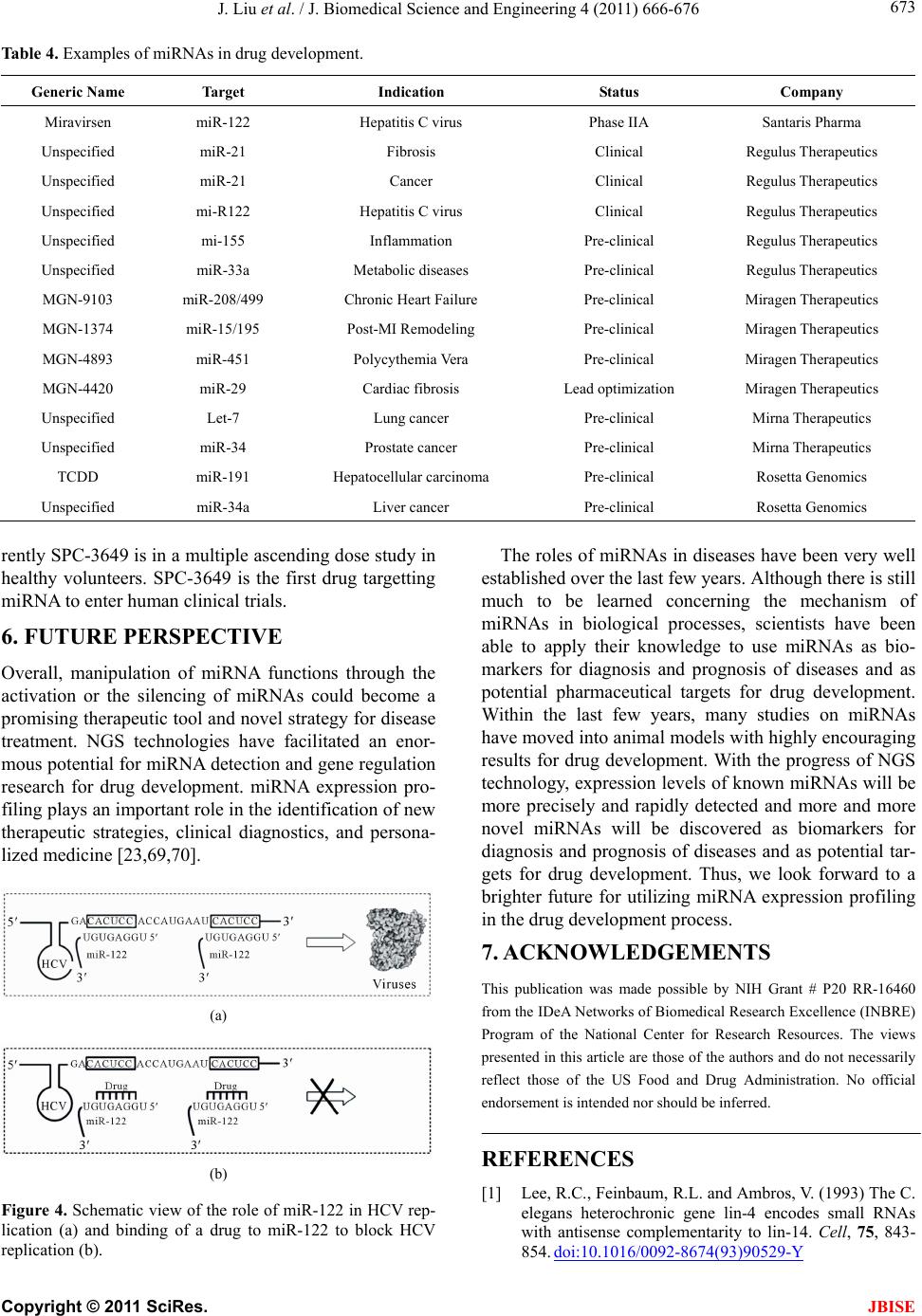 J. Liu et al. / J. Biomedical Science and Engineering 4 (2011) 666-676 Copyright © 2011 SciRes. 673 Table 4. Examples of miRNAs in drug development. Generic Name Target Indication Status Company Miravirsen miR-122 Hepatitis C virus Phase IIA Santaris Pharma Unspecified miR-21 Fibrosis Clinical Regulus Therapeutics Unspecified miR-21 Cancer Clinical Regulus Therapeutics Unspecified mi-R122 Hepatitis C virus Clinical Regulus Therapeutics Unspecified mi-155 Inflammation Pre-clinical Regulus Therapeutics Unspecified miR-33a Metabolic diseases Pre-clinical Regulus Therapeutics MGN-9103 miR-208/499 Chronic Heart Failure Pre-clinical Miragen Therapeutics MGN-1374 miR-15/195 Post-MI Remodeling Pre-clinical Miragen Therapeutics MGN-4893 miR-451 Polycythemia Vera Pre-clinical Miragen Therapeutics MGN-4420 miR-29 Cardiac fibrosis Lead optimization Miragen Therapeutics Unspecified Let-7 Lung cancer Pre-clinical Mirna Therapeutics Unspecified miR-34 Prostate cancer Pre-clinical Mirna Therapeutics TCDD miR-191 Hepatocellular carcinoma Pre-clinical Rosetta Genomics Unspecified miR-34a Liver cancer Pre-clinical Rosetta Genomics rently SPC-3649 is in a multiple ascending dose study in healthy volunteers. SPC-3649 is the first drug targetting miRNA to enter human clinical trials. The roles of miRNAs in diseases have been very well established over the last few years. Although there is still much to be learned concerning the mechanism of miRNAs in biological processes, scientists have been able to apply their knowledge to use miRNAs as bio- markers for diagnosis and prognosis of diseases and as potential pharmaceutical targets for drug development. Within the last few years, many studies on miRNAs have moved into animal models with highly encouraging results for drug development. With the progress of NGS technology, expression levels of known miRNAs will be more precisely and rapidly detected and more and more novel miRNAs will be discovered as biomarkers for diagnosis and prognosis of diseases and as potential tar- gets for drug development. Thus, we look forward to a brighter future for utilizing miRNA expression profiling in the drug development process. 6. FUTURE PERSPECTIVE Overall, manipulation of miRNA functions through the activation or the silencing of miRNAs could become a promising therapeutic tool and novel strategy for disease treatment. NGS technologies have facilitated an enor- mous potential for miRNA detection and gene regulation research for drug development. miRNA expression pro- filing plays an important role in the identification of new therapeutic strategies, clinical diagnostics, and persona- lized medicine [23,69,70]. 7. ACKNOWLEDGEMENTS This publication was made possible by NIH Grant # P20 RR-16460 from the IDeA Networks of Biomedical Research Excellence (INBRE) Program of the National Center for Research Resources. The views presented in this article are those of the authors and do not necessarily reflect those of the US Food and Drug Administration. No official endorsement is intended nor should be inferred. (a) REFERENCES (b) [1] Lee, R.C., Feinbaum, R.L. and Ambros, V. (1993) The C. elegans heterochronic gene lin-4 encodes small RNAs with antisense complementarity to lin-14. Cell, 75, 843- 854. doi:10.1016/0092-8674(93)90529-Y Figure 4. Schematic view of the role of miR-122 in HCV rep- lication (a) and binding of a drug to miR-122 to block HCV replication (b). JBISE
 J. Liu et al. / J. Biomedical Science and Engineering 4 (2011) 666-676 674 [2] Bartel, D.P. (2004) MicroRNAs: Genomics, biogenesis, mechanism, and function. Cell, 116, 281-297. doi:10.1016/S0092-8674(04)00045-5 [3] Bartel, D.P. (2009) MicroRNAs: Target recognition and regulatory functions. Cell, 136, 215-233. doi:10.1016/j.cell.2009.01.002 [4] Griffiths-Jones, S., Saini, H.K., van Dongen, S. and En- right, A.J. (2008) miRBase: Tools for microRNA genom- ics. Nucl eic Acid s Resear c h, 36, D154-D158. [5] Sheng, Y., Engstrom, P.G. and Lenhard, B. (2007) Mam- malian microRNA prediction through a support vector machine model of sequence and structure. PLoS One, 2, e946. doi:10.1371/journal.pone.0000946 [6] van den Berg, A., Mols, J. and Han, J. (2008) RISC- target interaction: Cleavage and translational suppression. Biochimica Biophysica Acta, 1779, 668-677. [7] Friedman, R.C., Farh, K.K., Burge, C.B. and Bartel, D.P. (2009) Most mammalian mRNAs are conserved targets of microRNAs. Genome Research, 19, 92-105. [8] Bueno, M.J., de Castro, I.P. and Malumbres, M. (2008) Control of cell proliferation pathways by microRNAs. Cell Cycle, 7, 3143-3148. doi:10.1101/gr.082701.108 [9] He, L. and Hannon, G.J. (2004) MicroRNAs: small RNAs with a big role in gene regulation. Nature Reviews Ge- netics, 5, 522-531. doi:10.1038/nrg1379 [10] Jovanovic, M. and Hengartner, M.O. (2006) microRNAs and apoptosis: RNAs to die for. Oncogene, 25, 6176- 6187. doi:10.1038/sj.onc.1209912 [11] Krutzfeldt, L. and Stoffel, M. (2006) MicroRNAs: A new class of regulatory genes affecting metabolism. Cell Matabolism, 4, 9-12. doi:10.1016/j.cmet.2006.05.009 [12] Stefani, G. and Slack, F.J. (2008) Small noncoding RNAs in animal development. Nature Reviews Molar Cell Bi- ology, 9, 219-230. doi:10.1038/nrm2347 [13] He, X., Eberhart, J.K. and Postlethwait, J.H. (2009) Mi- croRNAs and micro-managing the skeleton in diease, development, and evolution. Journal of Cellular and Mo- lecular Medicine, 13, 606-618. doi:10.1111/j.1582-4934.2009.00696.x [14] Schulte, J.H., Marschall. T., Martin, M., et al. (2010) Deep sequencing reveals differential expression of mi- croRNAs in favorable versus unfavorable neuroblastoma. Nucleic Aci ds Research, 38, 5919-5928. doi:10.1093/nar/gkq342 [15] Fasanaro, P., Greco, S., Ivan, M., Capogrossi, M.C. and Martelli, F. (2010) microRNA: Emerging therapeutic tar- gets in acute ischemic diseases. Pharmacology Thera- peutics, 125, 92-104. doi:10.1016/j.pharmthera.2009.10.003 [16] Trang, P., Weidhaas, J.B. and Slack, F.J. (2008) Mi- croRNAs as potential cancer therapeutics. Oncogene, 27, S52-S57. doi:10.1038/onc.2009.353 [17] Jiang, Q., Wang, Y., Hao, Y., et al. (2009) miR2Disease: A manually curated database for microRNA deregulation in human disease. Nucleic Ac id Research, 37, D98-D104. doi:10.1093/nar/gkn714 [18] Hennessy, E. and O’Driscoll, L. (2008) Molecular medi- cine of microRNAs: Structure, function, and implications for diabetes. Expert Reviews in Molecular Medicine, 10, e24. doi:10.1017/S1462399408000781 [19] van Rooij, E., Sutherland, L.B., Liu, N., et al. (2006) A signature pattern of stress-responsive microRNAs that can evoke cardiac hypertrophy and heart failure. Pro- ceedings of the National Academy of Sciences, 103, 18255-18260. doi:10.1073/pnas.0608791103 [20] Barbato, C., Giorge, C., Catalanotto, C. and Cogoni C. (2008) Thinking about RNA? MicroRNAs in the brain. Mammalian Genome, 19, 541-551. doi:10.1007/s00335-008-9129-6 [21] Beveridge, N.J., Gardiner, E., Carroll, A.P., et al. (2009) Schizophrenia is associated with an increase in cortical microRNA biogenesis. Molecular Psychiatry, 15, 1176- 1189. doi:10.1038/mp.2009.84 [22] Medina, P.P. and Slack, F.J. (2008) microRNAs and can- cer: An overview. Cell Cycle, 7, 2485-2492. doi:10.4161/cc.7.16.6453 [23] Nana-Sinkam, S.P. and Croce, C.M. (2011) MicroRNAs as therapeutic targets in cancer. Translational Research, 157, 216-225. doi:10.1016/j.trsl.2011.01.013 [24] Chen, C.Z. (2005) MicroRNAs as oncogenes and tumor suppressors. The New England Journal of Medicine, 353, 1768-1771. doi:10.1056/NEJMp058190 [25] Lagos-Quintana, M., Rauhut, R., Lendeckel, W. and Tuschl, T. (2001) Identification of novel genes coding for small expressed RNAs. Science, 294, 853-858. doi:10.1126/science.1064921 [26] Chen, C., Ridzon, D.A., Broomer, A.J., et al. (2005) Real- time quantification of microRNAs by stem-loop RT-PCR. Nucleic Aci ds Research, 33, e179. doi:10.1093/nar/gni178 [27] Shi, R. and Chiang, V.L. (2005) Facile means for quanti- fying microRNA expression by real-time PCR. Biotech- niques, 39, 519-525. doi:10.2144/000112010 [28] Yin, J.Q., Zhao, R.C. and Morris, K.V. (2008) Profiling microRNA expression with microarrays. Trends Bio- technol, 26, 70-76. doi:10.1016/j.tibtech.2007.11.007 [29] Li, W. and Ruan, K. (2009) MicroRNA detection by mi- croarray. Analytical Bioanalytical Chemistry, 394, 1117- 1124. doi:10.1007/s00216-008-2570-2 [30] Hafner, M., Landgraf, P., Ludwig, J., et al. (2008) Identi- fication of microRNAs and other small regulatory RNAs using cDNA library sequencing. Methods, 44, 3-12. doi:10.1016/j.ymeth.2007.09.009 [31] Roush, S. and Slack, F.J. (2008) The let-7 family of mi- croRNAs. Trends in Cell Biology, 18, 505-516. doi:10.1016/j.tcb.2008.07.007 [32] Buermans, H.P., Ariyurek, Y., van Ommen, G., et al. (2010) New methods for next generation sequencing based microRNA expression profiling. BMC Genomics, 11, 716. doi:10.1186/1471-2164-11-716 [33] Metzker, M.L. (2010) Sequencing technologies—the next generation. Nature Reviews Genetics, 11, 31-46. doi:10.1038/nrg2626 [34] ‘t Hoen, P.A, Ariyurek, Y., Thygesen, H.H., et al. (2008) Deep sequencing-based expression analysis shows major advances in robustness, resolution and inter-lab por- tability over five microarray platforms. Nucleic Acids Research, 36, e141. doi:10.1093/nar/gkn705 [35] Sanger, G., Air, G.M., Barrell, B.G., et al. (1977) Nucleo- tide sequence of bacteriophage X174 D. Nature, 265, 687- 695. [36] Sanger, F., Nicklen, S., Coulson, A.R., et al. (1977) DNA sequencing with chain-terminating inhibitors. Proceed- ings of the National Academy of Sciences, 74, 5463- C opyright © 2011 SciRes. JBISE
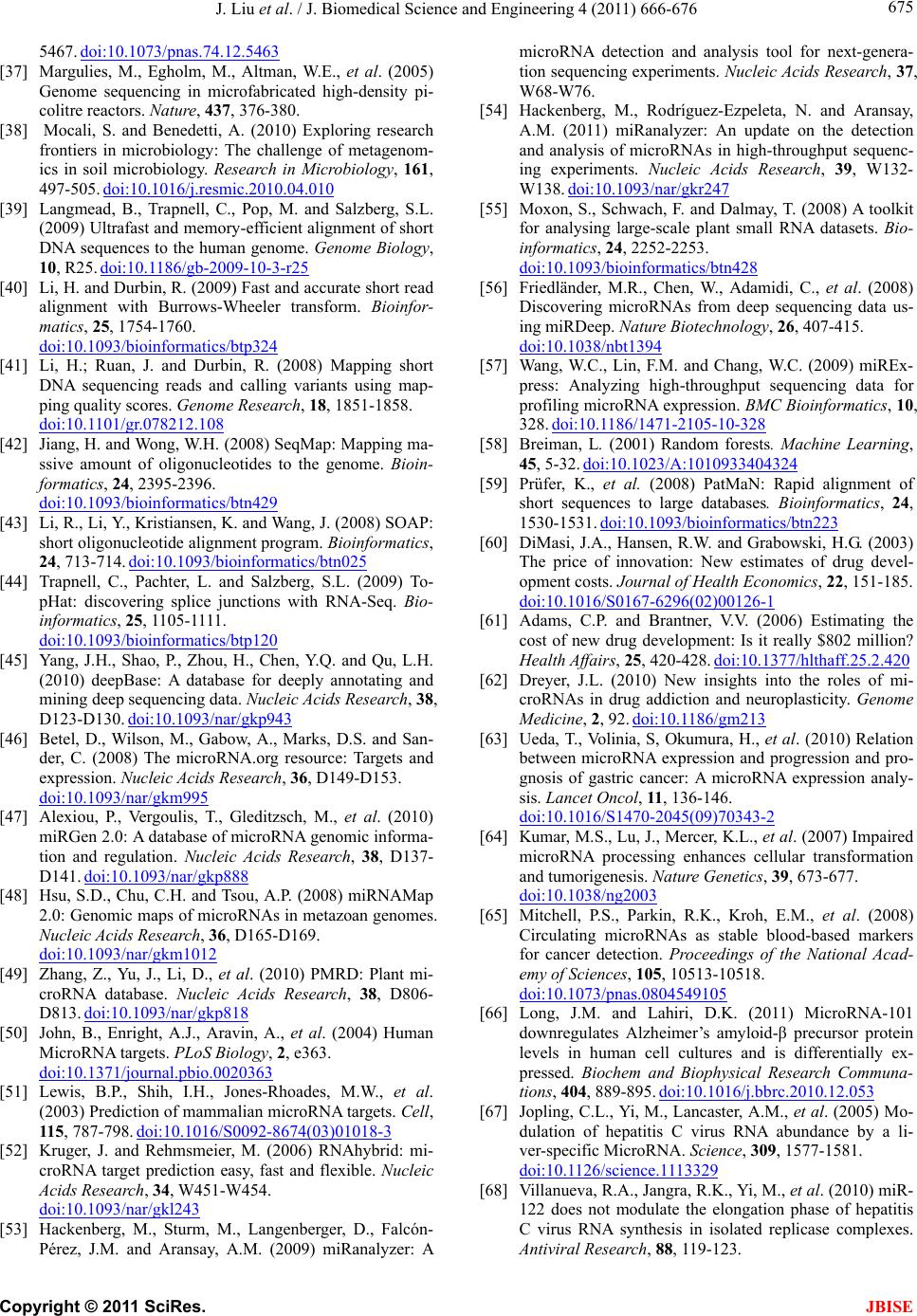 J. Liu et al. / J. Biomedical Science and Engineering 4 (2011) 666-676 675 5467. doi:10.1073/pnas.74.12.5463 [37] Margulies, M., Egholm, M., Altman, W.E., et al. (2005) Genome sequencing in microfabricated high-density pi- colitre reactors. Nature, 437, 376-380. [38] Mocali, S. and Benedetti, A. (2010) Exploring research frontiers in microbiology: The challenge of metagenom- ics in soil microbiology. Research in Microbiology, 161, 497-505. doi:10.1016/j.resmic.2010.04.010 [39] Langmead, B., Trapnell, C., Pop, M. and Salzberg, S.L. (2009) Ultrafast and memory-efficient alignment of short DNA sequences to the human genome. Genome Biology, 10, R25. doi:10.1186/gb-2009-10-3-r25 [40] Li, H. and Durbin, R. (2009) Fast and accurate short read alignment with Burrows-Wheeler transform. Bioinfor- matics, 25, 1754-1760. doi:10.1093/bioinformatics/btp324 [41] Li, H.; Ruan, J. and Durbin, R. (2008) Mapping short DNA sequencing reads and calling variants using map- ping quality scores. Genome Research, 18, 1851-1858. doi:10.1101/gr.078212.108 [42] Jiang, H. and Wong, W.H. (2008) SeqMap: Mapping ma- ssive amount of oligonucleotides to the genome. Bioin- formatics, 24, 2395-2396. doi:10.1093/bioinformatics/btn429 [43] Li, R., Li, Y., Kristiansen, K. and Wang, J. (2008) SOAP: short oligonucleotide alignment program. Bioinformatics, 24, 713-714. doi:10.1093/bioinformatics/btn025 [44] Trapnell, C., Pachter, L. and Salzberg, S.L. (2009) To- pHat: discovering splice junctions with RNA-Seq. Bio- informatics, 25, 1105-1111. doi:10.1093/bioinformatics/btp120 [45] Yang, J.H., Shao, P., Zhou, H., Chen, Y.Q. and Qu, L.H. (2010) deepBase: A database for deeply annotating and mining deep sequencing data. Nuclei c A c i d s Research, 38, D123-D130. doi:10.1093/nar/gkp943 [46] Betel, D., Wilson, M., Gabow, A., Marks, D.S. and San- der, C. (2008) The microRNA.org resource: Targets and expression. Nucleic A cids Research, 36, D149-D153. doi:10.1093/nar/gkm995 [47] Alexiou, P., Vergoulis, T., Gleditzsch, M., et al. (2010) miRGen 2.0: A database of microRNA genomic informa- tion and regulation. Nucleic Acids Research, 38, D137- D141. doi:10.1093/nar/gkp888 [48] Hsu, S.D., Chu, C.H. and Tsou, A.P. (2008) miRNAMap 2.0: Genomic maps of microRNAs in metazoan genomes. Nucleic Aci ds Research, 36, D165-D169. doi:10.1093/nar/gkm1012 [49] Zhang, Z., Yu, J., Li, D., et al. (2010) PMRD: Plant mi- croRNA database. Nucleic Acids Research, 38, D806- D813. doi:10.1093/nar/gkp818 [50] John, B., Enright, A.J., Aravin, A., et al. (2004) Human MicroRNA targets. PLoS Biology, 2, e363. doi:10.1371/journal.pbio.0020363 [51] Lewis, B.P., Shih, I.H., Jones-Rhoades, M.W., et al. (2003) Prediction of mammalian microRNA targets. Cell, 115, 787-798. doi:10.1016/S0092-8674(03)01018-3 [52] Kruger, J. and Rehmsmeier, M. (2006) RNAhybrid: mi- croRNA target prediction easy, fast and flexible. Nucleic Acids Research, 34, W451-W454. doi:10.1093/nar/gkl243 [53] Hackenberg, M., Sturm, M., Langenberger, D., Falcón- Pérez, J.M. and Aransay, A.M. (2009) miRanalyzer: A microRNA detection and analysis tool for next-genera- tion sequencing experiments. Nucleic Acids Research, 37, W68-W76. [54] Hackenberg, M., Rodríguez-Ezpeleta, N. and Aransay, A.M. (2011) miRanalyzer: An update on the detection and analysis of microRNAs in high-throughput sequenc- ing experiments. Nucleic Acids Research, 39, W132- W138. doi:10.1093/nar/gkr247 [55] Moxon, S., Schwach, F. and Dalmay, T. (2008) A toolkit for analysing large-scale plant small RNA datasets. Bio- informatics, 24, 2252-2253. doi:10.1093/bioinformatics/btn428 [56] Friedländer, M.R., Chen, W., Adamidi, C., et al. (2008) Discovering microRNAs from deep sequencing data us- ing miRDeep. Nature Biotechnology, 26, 407-415. doi:10.1038/nbt1394 [57] Wang, W.C., Lin, F.M. and Chang, W.C. (2009) miREx- press: Analyzing high-throughput sequencing data for profiling microRNA expression. BMC Bioinformatics, 10, 328. doi:10.1186/1471-2105-10-328 [58] Breiman, L. (2001) Random forests. Machine Learning, 45, 5-32. doi:10.1023/A:1010933404324 [59] Prüfer, K., et al. (2008) PatMaN: Rapid alignment of short sequences to large databases. Bioinformatics, 24, 1530-1531. doi:10.1093/bioinformatics/btn223 [60] DiMasi, J.A., Hansen, R.W. and Grabowski, H.G. (2003) The price of innovation: New estimates of drug devel- opment costs. Journal of Health Economics, 22, 151-185. doi:10.1016/S0167-6296(02)00126-1 [61] Adams, C.P. and Brantner, V.V. (2006) Estimating the cost of new drug development: Is it really $802 million? Health Affairs , 25, 420-428. doi:10.1377/hlthaff.25.2.420 [62] Dreyer, J.L. (2010) New insights into the roles of mi- croRNAs in drug addiction and neuroplasticity. Genome Medicine, 2, 92. doi:10.1186/gm213 [63] Ueda, T., Volinia, S, Okumura, H., et al. (2010) Relation between microRNA expression and progression and pro- gnosis of gastric cancer: A microRNA expression analy- sis. Lancet Oncol, 11, 136-146. doi:10.1016/S1470-2045(09)70343-2 [64] Kumar, M.S., Lu, J., Mercer, K.L., et al. (2007) Impaired microRNA processing enhances cellular transformation and tumorigenesis. Nature Genetics, 39, 673-677. doi:10.1038/ng2003 [65] Mitchell, P.S., Parkin, R.K., Kroh, E.M., et al. (2008) Circulating microRNAs as stable blood-based markers for cancer detection. Proceedings of the National Acad- emy of Sciences, 105, 10513-10518. doi:10.1073/pnas.0804549105 [66] Long, J.M. and Lahiri, D.K. (2011) MicroRNA-101 downregulates Alzheimer’s amyloid-β precursor protein levels in human cell cultures and is differentially ex- pressed. Biochem and Biophysical Research Communa- tions, 404, 889-895. doi:10.1016/j.bbrc.2010.12.053 [67] Jopling, C.L., Yi, M., Lancaster, A.M., et al. (2005) Mo- dulation of hepatitis C virus RNA abundance by a li- ver-specific MicroRNA. Science, 309, 1577-1581. doi:10.1126/science.1113329 [68] Villanueva, R.A., Jangra, R.K., Yi, M., et al. (2010) miR- 122 does not modulate the elongation phase of hepatitis C virus RNA synthesis in isolated replicase complexes. Antiviral Research, 88, 119-123. C opyright © 2011 SciRes. JBISE
 J. Liu et al. / J. Biomedical Science and Engineering 4 (2011) 666-676 Copyright © 2011 SciRes. 676 JBISE doi:10.1016/j.antiviral.2010.07.004 [69] Su, Z., Ning, B., Fang, H., et al. (2011) Next-generation sequencing and its applications in molecular diagnostics. Expert Review of Molecular Diagnonstics, 11 , 333-343. [70] Rogers, G.B. and Bruce, K.D. (2010) Next-generation sequencing in the analysis of human microbiota:essential considerations for clinical application. Molecular Diag- nosis & Therapy, 14, 343-350.
|