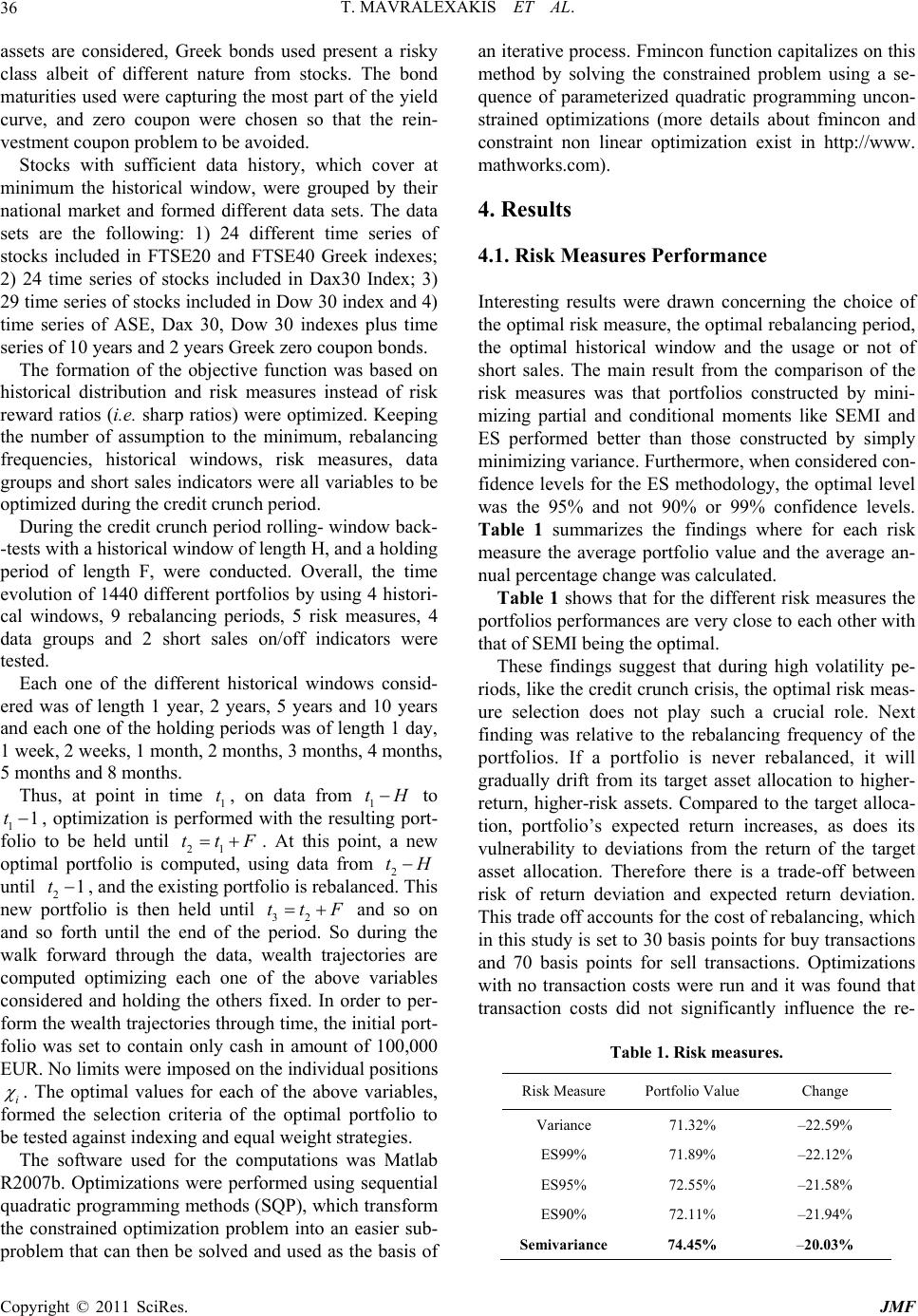
T. MAVRALEXAKIS ET AL.
Copyright © 2011 SciRes. JMF
36
assets are considered, Greek bonds used present a risky
class albeit of different nature from stocks. The bond
maturities used were capturing the most part of the yield
curve, and zero coupon were chosen so that the rein-
vestment coupon problem to be avoided.
Stocks with sufficient data history, which cover at
minimum the historical window, were grouped by their
national market and formed different data sets. The data
sets are the following: 1) 24 different time series of
stocks included in FTSE20 and FTSE40 Greek indexes;
2) 24 time series of stocks included in Dax30 Index; 3)
29 time series of stocks included in Dow 30 index and 4)
time series of ASE, Dax 30, Dow 30 indexes plus time
series of 10 years and 2 years Greek zero coupon bonds.
The formation of the objective function was based on
historical distribution and risk measures instead of risk
reward ratios (i.e. sharp ratios) were optimized. Keeping
the number of assumption to the minimum, rebalancing
frequencies, historical windows, risk measures, data
groups and short sales indicators were all variables to be
optimized during the credit crunch period.
During the credit crunch period rolling- window back-
-tests with a historical window of length H, and a holding
period of length F, were conducted. Overall, the time
evolution of 1440 different portfolios by using 4 histori-
cal windows, 9 rebalancing periods, 5 risk measures, 4
data groups and 2 short sales on/off indicators were
tested.
Each one of the different historical windows consid-
ered was of length 1 year, 2 years, 5 years and 10 years
and each one of the hold ing periods was of length 1 day,
1 week, 2 weeks, 1 month, 2 months, 3 months, 4 months,
5 months and 8 months.
Thus, at point in time 1
t, on data from 1
tH
to
11t, optimization is performed with the resulting port-
folio to be held until 21
ttF . At this point, a new
optimal portfolio is computed, using data from 2
tH
until 21t, and the existing portfolio is rebalanced. This
new portfolio is then held until 32
ttF and so on
and so forth until the end of the period. So during the
walk forward through the data, wealth trajectories are
computed optimizing each one of the above variables
considered and holding the others fixed. In order to per-
form the wealth trajectories through time, the initial port-
folio was set to contain only cash in amount of 100,000
EUR. No limits were imposed on the individual positions
i
. The optimal values for each of the above variables,
formed the selection criteria of the optimal portfolio to
be tested against indexing and equal weight strategies.
The software used for the computations was Matlab
R2007b. Optimizations were performed using sequential
quadratic programming methods (SQP), which transform
the constrained optimization problem into an easier sub-
problem that can then be solved and used as the basis of
an iterative process. Fmincon function capitalizes on this
method by solving the constrained problem using a se-
quence of parameterized quadratic programming uncon-
strained optimizations (more details about fmincon and
constraint non linear optimization exist in http://www.
mathworks.com).
4. Results
4.1. Risk Measures Performance
Interesting results were drawn concerning the choice of
the optimal risk measure, the optimal rebalancing period,
the optimal historical window and the usage or not of
short sales. The main result from the comparison of the
risk measures was that portfolios constructed by mini-
mizing partial and conditional moments like SEMI and
ES performed better than those constructed by simply
minimizing variance. Furthermore, when considered con-
fidence levels for the ES methodology, the optimal level
was the 95% and not 90% or 99% confidence levels.
Table 1 summarizes the findings where for each risk
measure the average portfolio value and the average an-
nual percentage change was calculated.
Table 1 shows that for the different risk measures the
portfolios performances are very close to each other with
that of SEMI being the optimal.
These findings suggest that during high volatility pe-
riods, like the credit crunch crisis, the optimal risk meas-
ure selection does not play such a crucial role. Next
finding was relative to the rebalancing frequency of the
portfolios. If a portfolio is never rebalanced, it will
gradually drift from its target asset allocation to higher-
return, higher-risk assets. Compared to the target alloca-
tion, portfolio’s expected return increases, as does its
vulnerability to deviations from the return of the target
asset allocation. Therefore there is a trade-off between
risk of return deviation and expected return deviation.
This trade off accounts for the cost of rebalancing, which
in this study is set to 30 basis points for buy transactions
and 70 basis points for sell transactions. Optimizations
with no transaction costs were run and it was found that
transaction costs did not significantly influence the re-
Table 1. Risk measures.
Risk Measure Portfolio Value Change
Variance 71.32% –22.59%
ES99% 71.89% –22.12%
ES95% 72.55% –21.58%
ES90% 72.11% –21.94%
Semivariance 74.45% –20.03%