Journal of Environmental Protection
Vol.4 No.2(2013), Article ID:28511,13 pages DOI:10.4236/jep.2013.42025
The Impact of Traffic Emission on Air Quality in an Urban Environment
Department of Environmental Sciences, Faculty of Meteorology, Environment & Arid Land Agriculture, King Abdulaziz University, Makkah, Saudi Arabia.
Email: hjeelani@gmail.com
Received November 26th, 2012; revised December 27th, 2012; accepted January 12th, 2013
Keywords: Air Quality; Pollutant Concentration; Field Experiment; Power Plant; Vehicle Traffic
ABSTRACT
Study of the impact of traffic emissions on air quality around the Haram Mosque in Makkah, Saudi Arabia, was conducted experimentally, numerically and statistically. Experimental study was performed to measure existing air quality. Numerical study was done to model the extent of air movement and pollutant dispersion within and around the Haram area. Statistical study was conducted to determine correlation coefficients, auto-correlation and time lags of each pollutant. Pollutant measurements were carried out using an air quality mobile laboratory at three sites. Numerical calculations were made using an ISC-AERMOD dispersion model. Concentrations of traffic emissions including nitrogen dioxide (NO2), sulfur dioxide (SO2), carbon monoxide (CO), ozone (O3) and airborne particulate material under 10 μm diameter (PM10) are presented and analyzed. The calculated concentrations are validated by comparing with observed values at the three sites. The results indicate good agreement between calculated concentrations and observed values, which demonstrate satisfactory model performance. Results show that the Haram area is experiencing high concentrations of dust. High buildings around the Haram Mosque act as flow obstacles. Mean pollutant dispersion was toward the south and southeast during January and June. Highest mean concentrations were observed in January and June.
1. Introduction
The air quality of urban environments has become more important in recent years. Control of air quality affected by traffic emission is vital for human health. Vehicular emissions are one of the major sources of air pollutants in the urban environment.
In recent years, many researchers have investigated pollutant emissions at various locations in Saudi Arabia. [1] found that in Jeddah, the most significant source of air pollution is the automobile. The number of automobiles in Jeddah jumped from less than 30,000 in 1973 to approximately 1,075,000 in 1992. This rate of increase roughly applies to most Saudi cities. Studies on air pollution have been made in Makkah, Saudi Arabia, focusing on the central area near the Haram Mosque and other important religious sites (i.e., Mina and Arafat). These studies show high concentrations of atmospheric air pollutants, in excess of standards. This is attributed to traffic emission during the Hajj season, when about three million people gather in these limited areas [2-10]. Also, there are many studies assessing air quality inside tunnels near the Haram Mosque, which also show very high concentrations surpassing standards [11-13].
Although several studies of pollutant emission around the Haram area in Makkah have been done, there is a lack of air quality data in that area. The objective of this study is to measure existing air quality and model pollutant dispersion within and around Haram, to improve both.
2. Methodology
2.1. Experimental Study
2.1.1. Site Description
Three sites were selected for air quality monitoring, to represent the study area and surroundings. Figure 1 shows locations of monitoring stations at the three sites. Two of these are close to the Haram Mosque. One is located near the Tawheed Hotel, about 400 meters west of the Kabaa, and the other is in front of Gesr Al-Nadwa gate, 200 meters from the north side of the Kabaa. The third site is at the end of the Shameyah area, approximately 600 meters from the north side from the Kabaa. Site selection reflected requirements of the air quality model, prevailing meteorological parameters, and locations of potentially affected sensitive receivers (both human and physical) in the area, for original and future
Figure 1. Locations of the mobile stations around Haram.
building scenarios.
Site selection was also based on traffic density. Station (S1) was chosen at the highest possible traffic density point, whereas station (S2) is in a zone with no traffic allowed. Station (S3) is in a relatively moderate traffic density area, at the border of the Shameyah area. In this way, effects of external sources of pollution, either permanent or temporary, can be measured.
2.1.2. Monitoring Stations
The current status of air quality within the study domain was observed by onsite ground level measurements. Monitoring was done simultaneously at all sites during May. The following parameters of national standards promulgated by the Presidency of Meteorology and Environment (PME) were measured: 1) nitrogen dioxide (NO2), 2) ozone (O3), 3) sulfur dioxide (SO2), 4) hydrogen sulfide (H2S), 4) carbon monoxide (CO), 5) nonmethane volatile organic compounds (NMVOC), and 6) particulates (PM10, PM2.5). Observed data of ground level concentration at the monitoring stations were used to validate simulated values from the air dispersion model.
2.2. Numerical Model
2.2.1. Model Description
The ISC-AERMOD dispersion model (Industrial Source Complex—AMS/EPA Regulatory Model) is an advanced, new generation model developed by the US EPA, and serves as a complete replacement for the ISC3. It is designed to predict pollutant concentration, as well as the extent of deposits over short-range (out to 50 kilometers) dispersion of air pollutant emissions from an industrial source complex. ISC-AERMOD features an integrated Geographical Information System (GIS), as well as intuitive data analysis interface tools that enable modeled objects and results to interact and be displayed alongside a variety of geophysical data.
The ISC-AERMOD is a steady-state plume model, in the sense that it assumes that concentrations over various distances during a modeled hour are governed by the temporally averaged meteorology of that hour. The steady state assumption yields useful results since statistics of concentration distribution are of primary concern, rather than specific concentrations at particular times and locations. ISC-AERMOD has been designed to handle computation of pollutant impacts in both flat and complex terrain within the same modeling framework.
In the stable boundary layer (SBL), concentration distribution is assumed Gaussian in both the vertical and horizontal. In the convective boundary layer (CBL), the horizontal distribution is assumed Gaussian, but the vertical distribution is described with a bi-Gaussian probability density function (pdf). The model also tracks any plume mass that penetrates an elevated stable layer, and allows it to reenter the boundary layer when and if appropriate.
Calculations of the AERMOD model were performed on a rectangular grid for the Haram area. All emission sources, such as traffic as line sources and power plants as point sources, are located on the selected rectangular grid. Coordinates extend a distance of 4 km in the positive direction of x, and a distance of 4 km in the positive direction of y. Grid base elements are squares, with side dimensions 500 m. The uniform square grids include 289 receptors. Figure 2 describes the study grid and locations of example pollutant sources.
2.2.2. Meteorological Data
Meteorological parameter data inputs for this modeling were obtained from the surface weather observatory station at King Abdulaziz International Airport (Figure 1), and were assumed representative of meteorological data for the entire city of Makkah. A detailed analysis of the meteorological data such as ceiling height, wind speed, wind direction, air temperature, total cloud opacity and total cloud amount has been made for the year 2009.
A fair estimate of pollutant dispersion in the atmosphere is based on the frequency distribution of wind direction, as well as wind speed. Figure 3 illustrates the hourly wind rose diagram for 2009. The prevailing wind direction was from the north, and comprised about 41.7% of all hourly wind directions. About 19.8% of the time, wind speed was 1.54 m/s; 14.9% of the time it was 3.09 m/s; during 48% of the time, wind was calm.
Temperature fluctuation around the Haram Mosque in Makkah is great. This is ascribed to the city being affected by thermal stability. The minimum recorded temperature for 2008 was 21˚C in January, and the maximum was 51˚C in July.
Pasquill classifies atmospheric stability according to
Figure 2. Model grids and location.
Figure 3. Hourly wind data for Makah during a year.
six classes. They are: A—extremely unstable, B—moderately unstable, C—slightly unstable, D—neutral, E— slightly stable and F—moderately stable. This classification was compiled according to wind speed, cloud cover and solar insolation, following [14] table. The mixing height is defined as the height above the surface through which relatively vigorous vertical mixing occurs [15], and it is determined using the Holzworth technique [16]. In the present study, meteorological data of wind speed, wind direction, temperature, atmospheric stability, mixing height, and other parameters have been used in the air dispersion model.
3. Results and Discussion
3.1. Model Validation
Results of the air quality model were validated using the three mobile stations (S1), (S2), and (S3) near the Haram area, as indicated previously in Figure 1. Stations monitored the various pollutants during the final weeks of May 2009. A comparison between monitored and model data is shown in Table 1.
Figure 4 shows a graphic comparison between monitored and modeled data. CO predictions for the three stations (3234, 4792, 1269 µg/m3) were generally close to monitored values (3406, 4206, 549 g/m3). The results indicate good agreement between calculated concentrations and observed values, which demonstrate satisfactory model performance. NOX predictions at stations (S2) and (S3) (172 and 109 µg/m3, respectively) were higher than measurements (65 and 108 µg/m3, respectively). Since the area was experiencing temporary heavy construction that was not included in the prediction model, the stations recorded higher concentrations of PM10 and PM2.5 particulates than corresponding modeled values.
3.2. Experimental Results
During the monitored days, several observations were made. Station (S1) is near the intersection of Jabal Al Kabaa Street, where heavy vehicle traffic was observed.
Table 1. Comparison of monitored and modeled data during May.
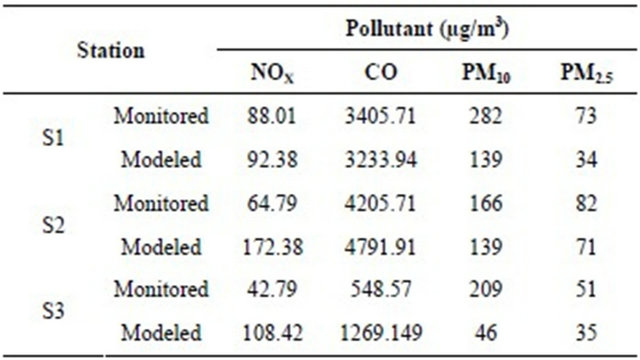
Figure 4. Modeled vs. measured concentrations.
There was also heavy construction at the Jabal Omar project (100 meters west of the site), where there was intense activity certain days of the week. This activity generated large amounts of airborne dust and particulates, as seen from monitoring data.
Average station data for the various pollutants during May 2009 are shown in Table 2. In general, Station (S1) showed the highest concentrations for all pollutants. Average NOX was 53.53 ppb, twice that at station (S3). SO2 and H2S were 3 - 4 times greater than at the other two stations, and non-methane hydrocarbon (NMHC) was also much greater. In general, causes of high pollution at station (S1) can be attributed to the following sources: 1) Heavy traffic, including standing taxi cabs near the station; 2) heavy construction activities at Jabal Omar; and 3) the temporary bus station near the area.
Automobile and truck traffic are permanent sources of air pollution in the area. An extensive car count was conducted at seven locations around the study area. The hourly average car and truck count on the street near station (S1) was 3102 cars and 282 trucks. The relatively greater concentrations from Monday through Friday were due to this traffic. Hourly average monitored data from the stations during study days are presented in Figures 5-7.
Maximum measured hourly and daily average concen
Table 2. Averaged data for all stations.

Figure 5. Station (S1) hourly averaged data May.
Figure 6. Station (S1) hourly averaged data May
Figure 7. Station (S1) hourly averaged data May.
trations of pollutants are shown in Table 3. All measured concentrations were converted to mg/m3 for comparison with standards. Comparisons for each pollutant from the three stations show that SO2 concentration at all stations were not higher than standards. The highest concentration was at station (S1), with a 1-hour average of 129 µg/m3, and 24-hour average of 85 µg/m3. Hydrogen sulfide did not exceed any 1-hour standard, at any station. However, station (S1) recorded one high 24-hour average concentration of H2S, on Saturday, 30 May 2009. The average reading was 33.17 ppb or 46 µg/m3, which exceeds the 40 µg/m3 standard of many countries, including Saudi Arabia and Royal Commission Environmental Regulations. Station (S1) reported only one high hourly average concentration of NO2—131.4 ppb or 247 µg/m3. Although this concentration exceeds the conservative value used by EPA-Kuwait (188 µg/m3), it does not surpass the 660 µg/m3 standard approved by Saudi Arabia and the Royal Commission Environmental Regulations. The maximum 24-hour average concentration of NO2 at all stations did not violate any standard. The levels of ozone and CO at the stations also did not exceed any standard. Particulate concentrations, however, exceeded all standards nearly 35% of the time, every day. Station (S1) reported 75 violations, during which PM10 topped 300 µg/m3. Station (S2) reported 28 violations for PM10, which is about 13% of the total number of days. For the 24-hour average standard, PM10 concentration surpassed the 150 g/m3 standard on nearly 90% of total days at station (S1). PM2.5 at the same station exceeded the standard of 65 µg/m3. In conclusion, the area around Haram is currently experiencing high airborne particulate matter concentrations, which must be dealt with carefully. This is a serious consideration during the coming construction in the Haram Mosque area.
3.3. Statistical Results
We did a statistical study of the various pollutants, at the same station and between stations. Table 4 shows correlation coefficients of the pollutants, with wind speed (Ws), temperature (Temp) and relative humidity (RH), at the same station. The statistical results indicate very high correlation between O3 and Temp, about 64%. Particulates PM10 and PM2.5 are highly correlated with Ws, Temp and RH, from 42% - 58% for station (S1). High negative correlation was observed between NOX and WS at (S2), and between O3 and RH at station (S3), about −56%.
Another useful analysis produced measures of autocorrelation for each pollutant during one day. This showed whether pollutant concentration had a repeatable pattern each day. Figure 8 shows 24-hour lag autocorre-
Table 3. The maximum hourly and daily average concentrations of pollutants of the stations converted to (μg/m3).
Table 4. Show the correlation coefficients of the pollutants.
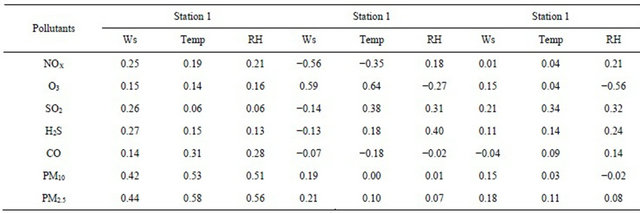
Figure 8. StationsS1, S2 & S3 24-hour lag auto-correlations.
lations of the pollutants at each station. The meteorological parameters are nearly repeatable every 24 hours at all stations. Temperature is 69% - 74% repeatable, and relative humidity 45% - 71% repeatable. Wind speed is 52% - 59% repeatable at stations (S1) and (S2), but was very random (8%) at station (S3). This owes to the location of station (S3), which is surrounded by high buildings. It was also shown that the wind rose for station (S3) does not follow the prevailing wind direction in the city of Makkah (north, northwest). Stations (S1) and (S2) had high particulate concentrations. Autocorrelation was about 30% - 50%. Daily data in the Appendix show repeated high concentrations every day, rising in the afternoon and falling around 8:00 pm. Ozone at stations (S2) and (S3) was 54% - 61% repeatable every day, but was somewhat random (25%) at station (S1). All other pollutants had small autocorrelations, except for CO at station (S2), which had 57% daily repeatability.
The relationship between the stations at different time lags was also examined. If pollutant concentration was changing at one station, this indicated how the other stations would read the temporal change. Table 5 lists the maximum correlation coefficient of pollutant concentrations between stations after a lag time in hours. Figures 9-13 show each pollutant as recorded at the three stations, and their temporal correlations. Notable findings of this inter-correlation study indicate that air temperature is almost 100% correlated between stations, meaning that a temperature change is recorded by stations equally. Relative humidities at stations (S2) and (S3) are strongly correlated, at 90% at zero-lag. There was a correlation of 53% - 62% at 6-hr lag between station (S1) and the other two. This may indicate that a humidity change at the (S1) location would influence areas to the east at this rate, owing to activities in the S1 area. Particulate readings are
Figure 9. Inter-correlations between stations for NOX.
Figure 10. Inter-correlations between stations for O3.
Figure 11. Inter-correlations between stations for SO2.
Table 5. Maximum inter-correlations between stations for different pollutants at shown lag times.
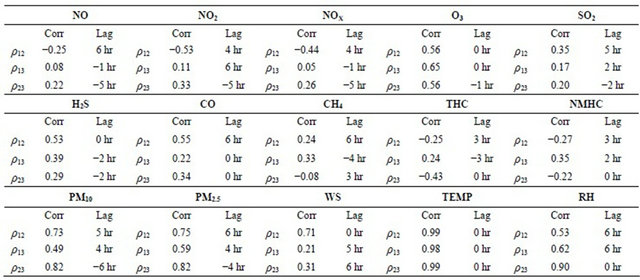
Figure 12. Inter-correlations between stations for CO.
Figure 13. Inter-correlations between stations for PM10.
very interesting. We expected the main source to be near station (S1), because of the construction in the area and traffic at the nearby intersection. The statistical study shows that there is high correlation (73%) between S1 and S2 for PM10, and 75% for PM2.5, at a lag of 5 - 6 hours. This means that it takes some time for particulates to disperse eastward to station (S2) near the Haram building. A similar argument can be made regarding the correlation between stations (S1) and (S3). For PM10, the correlation is 49% at a lag of 4 hours, and 59% at the same lag time for PM2.5. The final noticeable high correlation is for ozone. Correlations between the three stations range from 56% - 65% at zero-lag time, which confirms that ozone is a product of photochemical reactions in the atmosphere. Other pollutants show relatively low correlations for the different stations.
3.4. Numerical Results
Pollutant dispersion from traffic was modeled for each 1-hour averaging period over four months—January (winter), May (spring), June (summer) and September (fall). The Haram Mosque area is in the city of Makkah, which has a hot, dry climate. It contains large, uninhabited desert areas, with temperatures varying between 40˚C and 50˚C for at least 6 - 9 months of the year. Therefore, thermal stratification stability affects contaminant diffusion within the atmospheric boundary layer. Changing wind direction also affects pollutant dispersion.
Counter lines of pollutant concentrations for CO, NOX, VOC, PM10 and PM2.5 during January, May, June and September are shown in Figures 14-17. In January and
Figure 14. Contour lines for CO, NOX & PM10 concentrations during January.
Figure 15. Contour lines for CO, NOX & PM10 concentrations during May.
Figure 16. Contour lines for CO, NOX & PM10 concentrations during June.
Figure 17. Contour lines for CO, NOX & PM10 concentrations during September.
Table 6. Statistical analysis of pollutant concentration (μg/m3).
June, pollutant dispersion was toward the south and southeast. This is because the mean wind direction is north and sometimes northwest. The high buildings in the Haram area create an obstacle flow. Therefore, observed dispersion toward the north and northwest is caused by inverse flow from these high buildings.
In May, the mean wind direction is north and southwest. Therefore, pollutant dispersion was toward the south and northeast. In September, the mean wind direction is north and northwest. Therefore, pollutant dispersion was toward the south and southeast. Regarding the effect of thermal stability, pollutant dispersion in stable atmospheric conditions is greater than that for neutral and unstable conditions, as shown for January. However, observed pollutant dispersion in this month was greater than that in the other months. On the other hand, pollutant concentrations for unstable atmospheric conditions are greater than those for neutral and stable conditions, as shown for May and June. Table 6 describes the statistical analysis of pollutant concentrations for CO, NOX, VOC, and PM10 during January, May, June and September. The highest mean concentration was observed during January and June, whereas the lowest mean concentration was during June and September.
4. Conclusion
We conducted careful investigation of pollutant transport and dispersion from traffic emissions around the Haram Mosque area, using experimental monitoring and a numerical model. Results indicate that stations (S1) and (S2) had high particulate concentrations. Autocorrelation was about 30% - 50%. There was very high correlation between O3 and Temp, about 64%. Particulates PM10 and PM2.5 were highly correlated with WS, Temp and RH, from 42% - 58% for station (S1). High negative correlation was observed between NOX and WS at station S2, and between O3 and RH at station (S3), about −56%. The area is experiencing high concentrations of dust, and high buildings there act as flow obstacles. Mean pollutant dispersion was toward the south and southeast during January and June. Highest mean concentrations were observed during January and June, and lowest mean concentrations during June and September.
REFERENCES
- H. A. Jeelani, “Social and Meteorological Factors Control Air Pollution in Jeddah City, Saudi Arabia,” Ph.D. Thesis, University of East Anglia, Norwich, 1996
- Al-A. A. M. S. Abu-Alghat, “Study of the Air Pollution in Makkah City and the Holy Places,” Third Report Submitted to Hajj Research Center, Aum-Alqura University, Makkah, 1992.
- M. I. Badwi and M. A. Al-Hosary, “Study of Air Quality at Central Area near the Holy Mosque, Makkah City,” Final Report Submitted to Hajj Research Center, Makkah, 1993.
- Y. A. Al-Thumali, “Air Pollution Study of Mina and Arafaat Areas during Hajj Season 1996 with Concentration on Suspended Particulates and Some Gases,” Master’s Thesis, Department of Environmental Sciences, King Abdulaziz University, Jeddah, 1998.
- A. A. Yacob, “Air Quality Inside the Holy Mosque (Chemical and Microbial Content) ,” Final Report Submitted to Institute of the Custodian of the Two Holy Mosque, Aum Alqura University, Makkah, 2000.
- H. A. Al-Jeelani and M. H. Ramadhan, “Assessment of Air Quality in Mina and Makkah City during Hajj Season,” First Year Report Submitted to the Institute of Research and Consultations (Arabic Text), King Abdulaziz University, Jeddah, 2004.
- H. A. Al-Jeelani and M. H. Ramadhan, “Assessment of Air Quality in Mina and Makkah City during Hajj Season,” Final Year Report Submitted to the Institute of Research and Consultations (Arabic Text), King Abdulaziz University, Jeddah, 2005.
- H. A. Al-Jeelani, “Assessment of Air Quality in Muna valley during Hajj Season 1424 H,” Journal for Environmental Sciences, Vol. 7, No. 1, 2009.
- H. A. Al-Jeelani, “Air Quality Assessment at Al-Taneem Area in the Holy Makkah City, Saudi Arabia,” Environmental Monitoring and Assessment, Vol. 156, No. 1-4, 2009, pp. 211-222. doi:10.1007/s10661-008-0475-3
- H. A. Al-Jeelani, “Evaluation of Air Quality in the Holy Makkah during Hajj Season 1425 H,” Journal of Applied Sciences Research, Vol. 5, No. 1, 2009, pp. 115-121.
- A. Al-Sawas, “Air Quality of inside King Fahad Tunnel during the Month of Ramadhan,” Final Report Submitted to Hajj Research Center, Aum-Alqura University, Makkah, 1995.
- H. A. Al-Jeelani, “Effects of Traffic on Air Pollutants Concentration inside Souk Alsagheer Tunnel during the Month of Ramadhan,” Final Report Submitted to Hajj Research Center, Aum-Alqura University, Makkah, 1998.
- A. S. Al-Raddadi, “Study the Air Quality inside the Souk Alsagheer Tunnel,” First Report Submitted to Hajj Research Center, Aum Alqura University, Makkah, 1996.
- D. B. Turner, “Workbook of Atmospheric Dispersion Estimates,” Lewis, Washington DC, 1969.
- N. Manju, R. Balakrishnan and N. Mani, “Assimilative Capacity and Pollutant Dispersion Studies for the Industrial Zone of Manali,” Atmospheric Environment, Vol. 36, No. 21, 2002, pp. 3461-3471. doi:10.1016/S1352-2310(02)00306-0
- G. C. Holzworth, “Mixing Depths, Wind Speed and Air Pollution Potential for Selected Locations in the United States,” Journal of Applied Meteorology, Vol. 6, No. 6, 1967, pp. 1039-1044. doi:10.1175/1520-0450(1967)006<1039:MDWSAA>2.0.CO;2