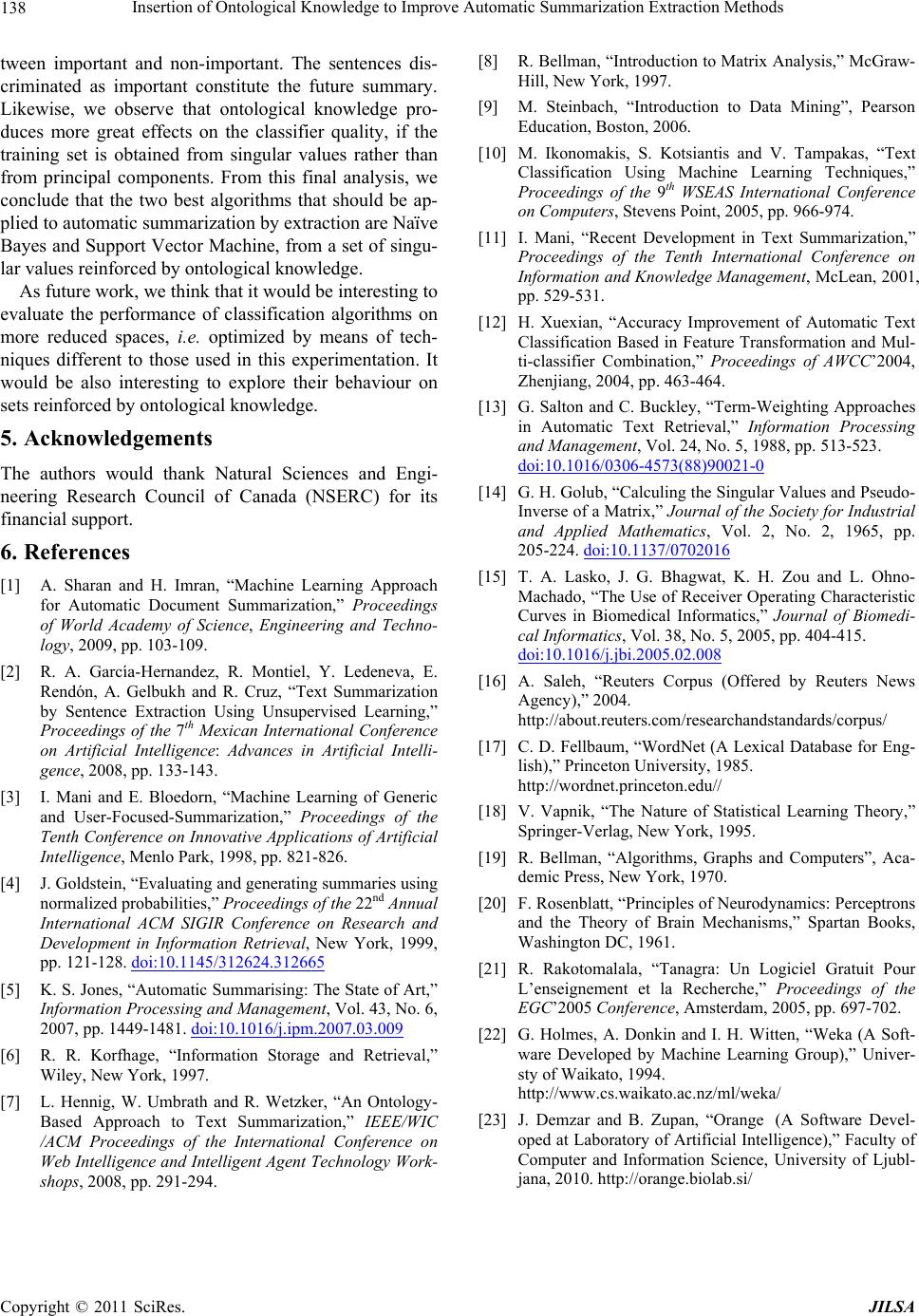
Insertion of Ontological Knowledge to Improve Automatic Summarization Extraction Methods
Copyright © 2011 SciRes. JILSA
138
tween important and non-important. The sentences dis-
criminated as important constitute the future summary.
Likewise, we observe that ontological knowledge pro-
duces more great effects on the classifier quality, if the
training set is obtained from singular values rather than
from principal components. From this final analysis, we
conclude that the two best algorithms that should be ap-
plied to auto matic summarization by extraction are Naïve
Bayes and Support Vector Machine, from a set of singu-
lar values reinforced by ontological kn o wl e d ge.
As future work, we think that it would be interesting to
evaluate the performance of classification algorithms on
more reduced spaces, i.e. optimized by means of tech-
niques different to those used in this experimentation. It
would be also interesting to explore their behaviour on
sets reinforced by ontological knowledge.
5. Acknowledgements
The authors would thank Natural Sciences and Engi-
neering Research Council of Canada (NSERC) for its
financial support.
6. References
[1] A. Sharan and H. Imran, “Machine Learning Approach
for Automatic Document Summarization,” Proceedings
of World Academy of Science, Engineering and Techno-
logy, 2009, pp. 103-109.
[2] R. A. García-Hernandez, R. Montiel, Y. Ledeneva, E.
Rendón, A. Gelbukh and R. Cruz, “Text Summarization
by Sentence Extraction Using Unsupervised Learning,”
Proceedings of the 7th Mexican International Conference
on Artificial Intelligence: Advances in Artificial Intelli-
gence, 2008, pp. 133-143.
[3] I. Mani and E. Bloedorn, “Machine Learning of Generic
and User-Focused-Summarization,” Proceedings of the
Tenth Conference on Innovative Applications of Artificial
Intelligence, Menlo Park, 1998, pp. 821-826.
[4] J. Goldstein, “Evaluating and generating summaries using
normalized probabilities,” Proceedings of the 22nd Annual
International ACM SIGIR Conference on Research and
Development in Information Retrieval, New York, 1999,
pp. 121-128. doi:10.1145/312624.312665
[5] K. S. Jones, “Automatic Summarising: The State of Art,”
Information Processing and Management, Vol. 43, No. 6,
2007, pp. 1449-1481. doi:10.1016/j.ipm.2007.03.009
[6] R. R. Korfhage, “Information Storage and Retrieval,”
Wiley, New York, 1997.
[7] L. Hennig, W. Umbrath and R. Wetzker, “An Ontology-
Based Approach to Text Summarization,” IEEE/WIC
/ACM Proceedings of the International Conference on
Web Intelligence and Intelligent Agent Technology Work-
shops, 2008, pp. 291-294.
[8] R. Bellman, “Introduction to Matrix Analysis,” McGraw-
Hill, New York, 1997.
[9] M. Steinbach, “Introduction to Data Mining”, Pearson
Education, Boston, 2006.
[10] M. Ikonomakis, S. Kotsiantis and V. Tampakas, “Text
Classification Using Machine Learning Techniques,”
Proceedings of the 9th WSEAS International Conference
on Computers, Stevens Point, 2005, pp. 966-974.
[11] I. Mani, “Recent Development in Text Summarization,”
Proceedings of the Tenth International Conference on
Information and Knowledge Management, McLean, 2001,
pp. 529-531.
[12] H. Xuexian, “Accuracy Improvement of Automatic Text
Classification Based in Feature Transformation and Mul-
ti-classifier Combination,” Proceedings of AWCC’2004,
Zhenjiang, 2004, pp. 463-464.
[13] G. Salton and C. Buckley, “Term-Weighting Approaches
in Automatic Text Retrieval,” Information Processing
and Management, Vol. 24, No. 5, 1988, pp. 513-523.
doi:10.1016/0306-4573(88)90021-0
[14] G. H. Golub, “Calculing the Singular Values and Pseudo-
Inverse of a Matrix,” Journal of the Society for Industrial
and Applied Mathematics, Vol. 2, No. 2, 1965, pp.
205-224. doi:10.1137/0702016
[15] T. A. Lasko, J. G. Bhagwat, K. H. Zou and L. Ohno-
Machado, “The Use of Receiver Operating Characteristic
Curves in Biomedical Informatics,” Journal of Biomedi-
cal Informatics, Vol. 38, No. 5, 2005, pp. 404-415.
doi:10.1016/j.jbi.2005.02.008
[16] A. Saleh, “Reuters Corpus (Offered by Reuters News
Agency),” 2004.
http://about.reuters.com/researchandstandards/corpus/
[17] C. D. Fellbaum, “WordNet (A Lexical Database for Eng-
lish),” Princeton University, 1985.
http://wordnet.princeton.edu//
[18] V. Vapnik, “The Nature of Statistical Learning Theory,”
Springer-Verlag, New York, 1995.
[19] R. Bellman, “Algorithms, Graphs and Computers”, Aca-
demic Press, New York, 1970.
[20] F. Rosenblatt, “Principles of Neurodynamics: Perceptrons
and the Theory of Brain Mechanisms,” Spartan Books,
Washington DC, 1961.
[21] R. Rakotomalala, “Tanagra: Un Logiciel Gratuit Pour
L’enseignement et la Recherche,” Proceedings of the
EGC’2005 Conference, Amsterdam, 2005, pp. 697-702.
[22] G. Holmes, A. Donkin and I. H. Witten, “Weka (A Soft-
ware Developed by Machine Learning Group),” Univer-
sty of Waikato, 1994.
http://www.cs.waikato.ac.nz/ml/weka/
[23] J. Demzar and B. Zupan, “Orange (A Software Devel-
oped at Laboratory of Artificial Intelligence),” Faculty of
Computer and Information Science, University of Ljubl-
jana, 2010. http://orange.biolab.si/