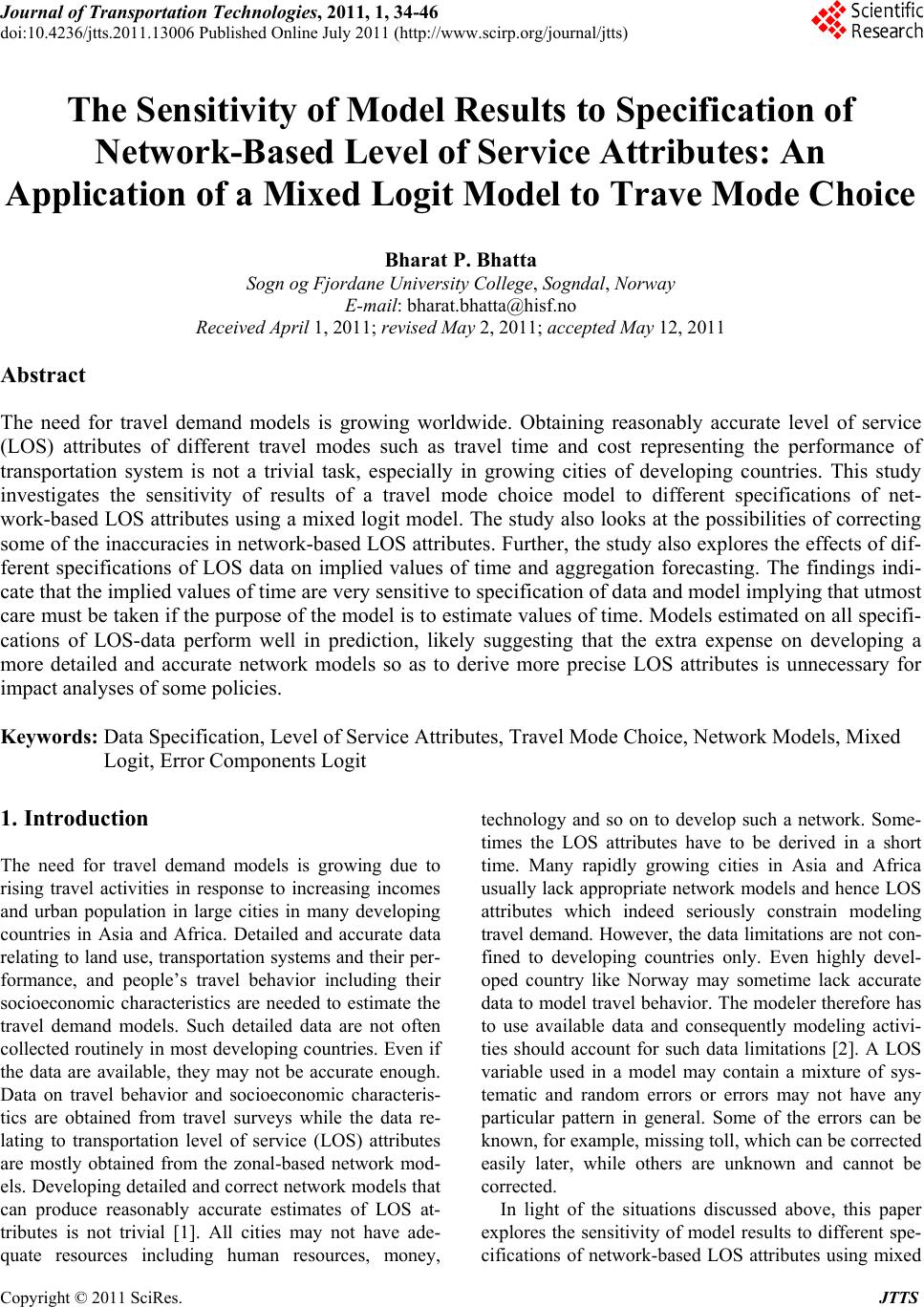 Journal of Transportation Technologies, 2011, 1, 34-46 doi:10.4236/jtts.2011.13006 Published Online July 2011 (http://www.scirp.org/journal/jtts) Copyright © 2011 SciRes. JTTS The Sensitivity of Model Results to Specification of Network-Based Level of Service Attributes: An Application of a Mixed Logit Model to Trave Mode Choice Bharat P. Bhatta Sogn og Fjordane University College, Sogndal, Norway E-mail: bharat.bhatta@hisf.no Received April 1, 2011; revised May 2, 2011; accepted May 12, 2011 Abstract The need for travel demand models is growing worldwide. Obtaining reasonably accurate level of service (LOS) attributes of different travel modes such as travel time and cost representing the performance of transportation system is not a trivial task, especially in growing cities of developing countries. This study investigates the sensitivity of results of a travel mode choice model to different specifications of net- work-based LOS attributes using a mixed logit model. The study also looks at the possibilities of correcting some of the inaccuracies in network-based LOS attributes. Further, the study also explores the effects of dif- ferent specifications of LOS data on implied values of time and aggregation forecasting. The findings indi- cate that the implied values of time are very sensitive to specification of data and model implying that utmost care must be taken if the purpose of the model is to estimate values of time. Models estimated on all specifi- cations of LOS-data perform well in prediction, likely suggesting that the extra expense on developing a more detailed and accurate network models so as to derive more precise LOS attributes is unnecessary for impact analyses of some policies. Keywords: Data Specification, Level of Service Attributes, Travel Mode Choice, Network Models, Mixed Logit, Error Components Logit 1. Introduction The need for travel demand models is growing due to rising travel activities in response to increasing incomes and urban population in large cities in many developing countries in Asia and Africa. Detailed and accurate data relating to land use, transportation systems and their per- formance, and people’s travel behavior including their socioeconomic characteristics are needed to estimate the travel demand models. Such detailed data are not often collected routinely in most developing countries. Even if the data are available, they may not be accurate enough. Data on travel behavior and socioeconomic characteris- tics are obtained from travel surveys while the data re- lating to transportation level of service (LOS) attributes are mostly obtained from the zonal-based network mod- els. Developing detailed and correct network models that can produce reasonably accurate estimates of LOS at- tributes is not trivial [1]. All cities may not have ade- quate resources including human resources, money, technology and so on to develop such a network. Some- times the LOS attributes have to be derived in a short time. Many rapidly growing cities in Asia and Africa usually lack appropriate network models and hence LOS attributes which indeed seriously constrain modeling travel demand. However, the data limitations are not con- fined to developing countries only. Even highly devel- oped country like Norway may sometime lack accurate data to model travel behavior. The modeler therefore has to use available data and consequently modeling activi- ties should account for such data limitations [2]. A LOS variable used in a model may contain a mixture of sys- tematic and random errors or errors may not have any particular pattern in general. Some of the errors can be known, for example, missing toll, which can be corrected easily later, while others are unknown and cannot be corrected. In light of the situations discussed above, this paper explores the sensitivity of model results to different spe- cifications of network-based LOS attributes using mixed
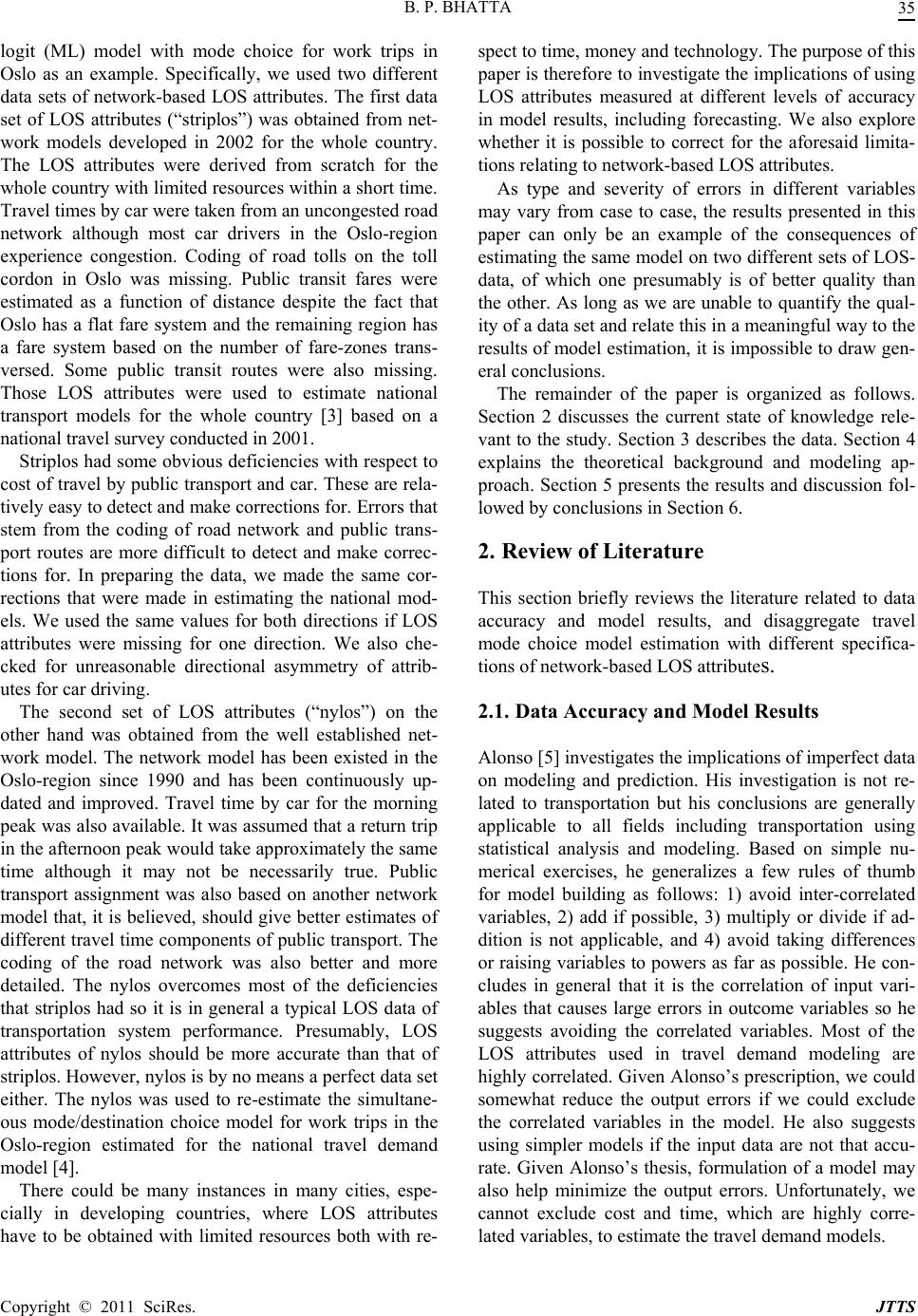 B. P. BHATTA35 logit (ML) model with mode choice for work trips in Oslo as an example. Specifically, we used two different data sets of network-based LOS attributes. The first data set of LOS attributes (“striplos”) was obtained from net- work models developed in 2002 for the whole country. The LOS attributes were derived from scratch for the whole country with limited resources within a short time. Travel times by car were taken from an uncongested road network although most car drivers in the Oslo-region experience congestion. Coding of road tolls on the toll cordon in Oslo was missing. Public transit fares were estimated as a function of distance despite the fact that Oslo has a flat fare system and the remaining region has a fare system based on the number of fare-zones trans- versed. Some public transit routes were also missing. Those LOS attributes were used to estimate national transport models for the whole country [3] based on a national travel survey conducted in 2001. Striplos had some obvious deficiencies with respect to cost of travel by public transport and car. These are rela- tively easy to detect and make corrections for. Errors that stem from the coding of road network and public trans- port routes are more difficult to detect and make correc- tions for. In preparing the data, we made the same cor- rections that were made in estimating the national mod- els. We used the same values for both directions if LOS attributes were missing for one direction. We also che- cked for unreasonable directional asymmetry of attrib- utes for car driving. The second set of LOS attributes (“nylos”) on the other hand was obtained from the well established net- work model. The network model has been existed in the Oslo-region since 1990 and has been continuously up- dated and improved. Travel time by car for the morning peak was also available. It was assumed that a return trip in the afternoon peak would take approximately the same time although it may not be necessarily true. Public transport assignment was also based on another network model that, it is believed, should give better estimates of different travel time components of public transport. The coding of the road network was also better and more detailed. The nylos overcomes most of the deficiencies that striplos had so it is in general a typical LOS data of transportation system performance. Presumably, LOS attributes of nylos should be more accurate than that of striplos. However, nylos is by no means a perfect data set either. The nylos was used to re-estimate the simultane- ous mode/destination choice model for work trips in the Oslo-region estimated for the national travel demand model [4]. There could be many instances in many cities, espe- cially in developing countries, where LOS attributes have to be obtained with limited resources both with re- spect to time, money and technology. The purpose of this paper is therefore to investigate the implications of using LOS attributes measured at different levels of accuracy in model results, including forecasting. We also explore whether it is possible to correct for the aforesaid limita- tions relating to network-based LOS attributes. As type and severity of errors in different variables may vary from case to case, the results presented in this paper can only be an example of the consequences of estimating the same model on two different sets of LOS- data, of which one presumably is of better quality than the other. As long as we are unable to quantify the qual- ity of a data set and relate this in a meaningful way to the results of model estimation, it is impossible to draw gen- eral conclusions. The remainder of the paper is organized as follows. Section 2 discusses the current state of knowledge rele- vant to the study. Section 3 describes the data. Section 4 explains the theoretical background and modeling ap- proach. Section 5 presents the results and discussion fol- lowed by conclusions in Section 6. 2. Review of Literature This section briefly reviews the literature related to data accuracy and model results, and disaggregate travel mode choice model estimation with different specifica- tions of network-based LOS attributes. 2.1. Data Accuracy and Model Results Alonso [5] investigates the implications of imperfect data on modeling and prediction. His investigation is not re- lated to transportation but his conclusions are generally applicable to all fields including transportation using statistical analysis and modeling. Based on simple nu- merical exercises, he generalizes a few rules of thumb for model building as follows: 1) avoid inter-correlated variables, 2) add if possible, 3) multiply or divide if ad- dition is not applicable, and 4) avoid taking differences or raising variables to powers as far as possible. He con- cludes in general that it is the correlation of input vari- ables that causes large errors in outcome variables so he suggests avoiding the correlated variables. Most of the LOS attributes used in travel demand modeling are highly correlated. Given Alonso’s prescription, we could somewhat reduce the output errors if we could exclude the correlated variables in the model. He also suggests using simpler models if the input data are not that accu- rate. Given Alonso’s thesis, formulation of a model may also help minimize the output errors. Unfortunately, we cannot exclude cost and time, which are highly corre- lated variables, to estimate the travel demand models. Copyright © 2011 SciRes. JTTS
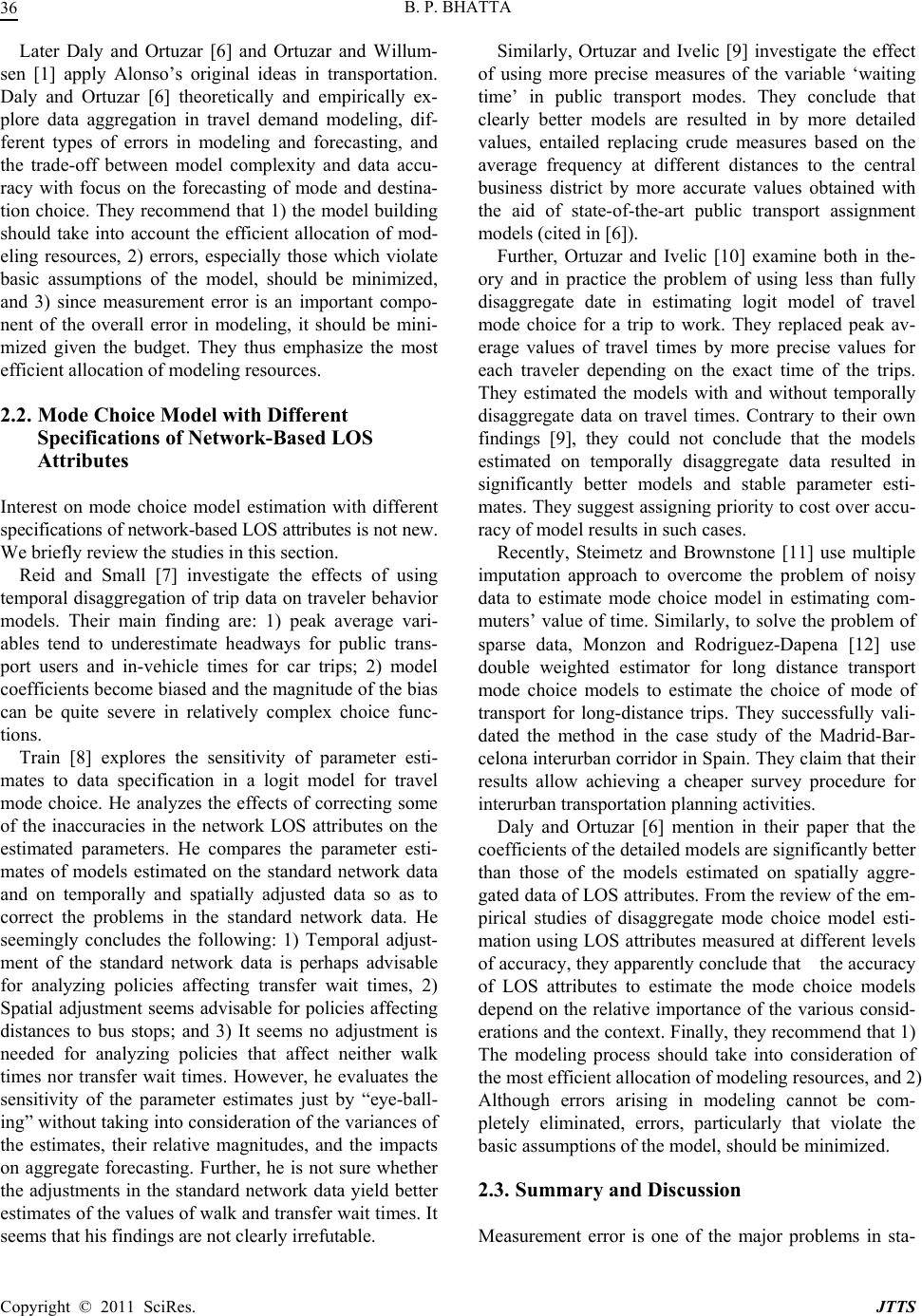 B. P. BHATTA 36 Later Daly and Ortuzar [6] and Ortuzar and Willum- sen [1] apply Alonso’s original ideas in transportation. Daly and Ortuzar [6] theoretically and empirically ex- plore data aggregation in travel demand modeling, dif- ferent types of errors in modeling and forecasting, and the trade-off between model complexity and data accu- racy with focus on the forecasting of mode and destina- tion choice. They recommend that 1) the model building should take into account the efficient allocation of mod- eling resources, 2) errors, especially those which violate basic assumptions of the model, should be minimized, and 3) since measurement error is an important compo- nent of the overall error in modeling, it should be mini- mized given the budget. They thus emphasize the most efficient allocation of modeling resources. 2.2. Mode Choice Model with Different Specifications of Network-Based LOS Attributes Interest on mode choice model estimation with different specifications of network-based LOS attributes is not new. We briefly review the studies in this section. Reid and Small [7] investigate the effects of using temporal disaggregation of trip data on traveler behavior models. Their main finding are: 1) peak average vari- ables tend to underestimate headways for public trans- port users and in-vehicle times for car trips; 2) model coefficients become biased and the magnitude of the bias can be quite severe in relatively complex choice func- tions. Train [8] explores the sensitivity of parameter esti- mates to data specification in a logit model for travel mode choice. He analyzes the effects of correcting some of the inaccuracies in the network LOS attributes on the estimated parameters. He compares the parameter esti- mates of models estimated on the standard network data and on temporally and spatially adjusted data so as to correct the problems in the standard network data. He seemingly concludes the following: 1) Temporal adjust- ment of the standard network data is perhaps advisable for analyzing policies affecting transfer wait times, 2) Spatial adjustment seems advisable for policies affecting distances to bus stops; and 3) It seems no adjustment is needed for analyzing policies that affect neither walk times nor transfer wait times. However, he evaluates the sensitivity of the parameter estimates just by “eye-ball- ing” without taking into consideration of the variances of the estimates, their relative magnitudes, and the impacts on aggregate forecasting. Further, he is not sure whether the adjustments in the standard network data yield better estimates of the values of walk and transfer wait times. It seems that his findings are not clearly irrefutable. Similarly, Ortuzar and Ivelic [9] investigate the effect of using more precise measures of the variable ‘waiting time’ in public transport modes. They conclude that clearly better models are resulted in by more detailed values, entailed replacing crude measures based on the average frequency at different distances to the central business district by more accurate values obtained with the aid of state-of-the-art public transport assignment models (cited in [6]). Further, Ortuzar and Ivelic [10] examine both in the- ory and in practice the problem of using less than fully disaggregate date in estimating logit model of travel mode choice for a trip to work. They replaced peak av- erage values of travel times by more precise values for each traveler depending on the exact time of the trips. They estimated the models with and without temporally disaggregate data on travel times. Contrary to their own findings [9], they could not conclude that the models estimated on temporally disaggregate data resulted in significantly better models and stable parameter esti- mates. They suggest assigning priority to cost over accu- racy of model results in such cases. Recently, Steimetz and Brownstone [11] use multiple imputation approach to overcome the problem of noisy data to estimate mode choice model in estimating com- muters’ value of time. Similarly, to solve the problem of sparse data, Monzon and Rodriguez-Dapena [12] use double weighted estimator for long distance transport mode choice models to estimate the choice of mode of transport for long-distance trips. They successfully vali- dated the method in the case study of the Madrid-Bar- celona interurban corridor in Spain. They claim that their results allow achieving a cheaper survey procedure for interurban transportation planning activities. Daly and Ortuzar [6] mention in their paper that the coefficients of the detailed models are significantly better than those of the models estimated on spatially aggre- gated data of LOS attributes. From the review of the em- pirical studies of disaggregate mode choice model esti- mation using LOS attributes measured at different levels of accuracy, they apparently conclude that the accuracy of LOS attributes to estimate the mode choice models depend on the relative importance of the various consid- erations and the context. Finally, they recommend that 1) The modeling process should take into consideration of the most efficient allocation of modeling resources, and 2) Although errors arising in modeling cannot be com- pletely eliminated, errors, particularly that violate the basic assumptions of the model, should be minimized. 2.3. Summary and Discussion Measurement error is one of the major problems in sta- Copyright © 2011 SciRes. JTTS
 B. P. BHATTA37 tistical analysis and modeling. It is generally accepted that errors in input data create errors in models, and often those errors can become far more serious in the model than appears in the data. It is also mostly accepted that developing an accurate and detailed network model to derive accurate enough LOS attributes is not that trivial [1]. Daly and Ortuzar [6] therefore emphasize that errors especially those which violate basic assumptions of a model should be minimized. Studies on the problem of disaggregate travel mode choice model estimation using LOS attributes measured at different levels of accuracy are conducted in different situations for specific problems with different assump- tions. Additionally, those studies focus on different vari- ables and do not have consistent findings. Consequently, it is difficult to draw general conclusions. The study in this paper examines the effects of using network LOS attributes measured at different levels of accuracy on relative magnitudes of the coefficients, specifically a value of time implied by travel demand models, and ag- gregate forecasting. The modelers will frequently en- counter the situation dealt in this paper. We generally expect that requirement of accuracy of LOS attributes may depend on the purpose of developing a model to some extent [6,13]. A systematic error in one or more variables may not necessarily result in severe consequences if model applications also use the input data having the same systematic error. The parameter estimates will in most cases be robust to the error. But systematic error may lead to severe consequences if the purpose is to estimate the implied value of travel time savings for different modes. Random measurement errors always bias parameters in an unpredictable way [14]. 3. Data We use data from the Norwegian national travel survey undertaken in 2001 in this study supplemented by a similar travel survey undertaken in the Oslo-region in the same period and the LOS attributes of transportation system obtained from network models. The survey ran- domly selected 20,751 people. The respondents were asked about the socioeconomic characteristics of the household, his/her travel activities including daily travels, long travels, employment, work travel, spouse/cohabitant, household, household access to transport resources, and detailed information about the interviewees. A detailed description of the design and conduct of the survey, characteristics of the sample, and questionnaire adminis- tered can be found in Denstadli, et al. [15]. The travel survey therefore provides the information on actual choice and socioeconomic characteristics of travelers including their households. This study uses a sub-sample for commuting trips to work, hereafter referred to as work trips, in Oslo of the national travel survey. The work trip is defined as a two way movement from home to work and back. Some trips had secondary destinations such as taking/collecting kids to/from kindergarten, shop- ping at grocery, etc on the way to/from work. There are 2,946 such trips in the sub-sample. As a part of data validation, several screening and consistency checks were performed. Some observations were deleted during the data validation process so the final data set had only 2, 876 work tours. The possible alternatives for the population for work trips in the study area consisted of five modes, viz., walking (WK), cycling (CK), car driving (CD), car pas- senger (CP) and public transport (PT) with actual modal shares of 8%, 6%, 52%, 5% and 29% respectively. These five modes serve as the universal choice set. Each indi- vidual traveler may have different choice sets given their own circumstances and constraints. The criteria used for alternative availability when estimating the models on the different specifications of the LOS attributes were of course the same. As mentioned earlier, the two data sets of the network-based LOS attributes, namely, striplos and nylos, were used to study the models in this study. Interzonal trips are sometimes excluded from model estimation since they do not appear on a network in the centroid-to-centroid travel. The exclusion of these trips results in biased sample thereby causing biased parame- ter estimates of model and biased aggregate forecasting [16]. In this analysis, instead of outright deleting the in- trazonal trips, they were included in the estimation. It was assumed that the length of the trip was equal to the length of the centroid connector and low speed for intra- zonal trips by cars since the trips are short and usually stay on local roads. However, public transport was set unavailable for the intrazonal trips. 4. Theoretical Framework and Model Formulation In this section, we present theoretical framework under- lying choice modeling and model formulation. 4.1. Theoretical Framework Choice models based on random utility maximization (RUM) hypothesis are the most widely used tools to examine individual travel behavior [1,17-20]. In a RUM framework of choice modeling, a decision maker facing a mutually exclusive and collective exhaustive set of finite number of alternatives obtains utility from each alternative and chooses the one with the highest utility. But the analyst is not able to observe the utility of alter- Copyright © 2011 SciRes. JTTS
 B. P. BHATTA 38 natives, therefore, decomposes the utility into two parts for analytical purposes: 1) an observable part and 2) an unobservable part. The utility of the alternative i J for the decision maker n, Uin, can therefore be written as: Uin = Vin + εin (1) where Vin and εin represent the observed and unobserved parts of the utility of the alternative i for the decision maker n respectively from the point of view of the ana- lyst. Vin is the systematic or representative utility. The systematic utility is deterministic in the sense that it is broadly a function of a vector of attributes of the alterna- tive, Zin, and a vector of characteristics of the decision maker, Sn, so: Vin = V (Zin, Sn) (2) RUM models of travel demand is a highly researched field where advanced models such as generalized ex- treme value models allowing for advanced nesting struc- tures (cross-nesting and multi-levels and recursive) and models with mixed distributions (e.g. mixed logit) [20-22] are developed. In recent years, advanced discrete choice models, such as models with advanced nesting structures and models based on mixed distributions, are increas- ingly used to allow for flexible substitution patterns, correlation across alternatives and/or random taste het- erogeneity [22]. The mixed logit (ML) model is the most advanced model among choice models. Initially, Boyd and Mellman [23], and Cardell and Dunbar [24] used the ML model in modeling automobile demand. Their mod- els were not truly disaggregate because their dependent variable was market shares rather than individual cus- tomers’ choice and the explanatory variables did not vary over the decision makers. The extremely high cost of estimation (and hence implementing the results) pre- vented the use of ML model for many years after the initial development. The disaggregate ML model has been in the extensive use since the advent of high speed computers, mass storage devices, and simulation. The flexibility of the model and decreased cost of computa- tion have led to the widespread use of ML models in diverse fields, including political science [25], resource economics [26], transportation [27], peace and conflict [28], and business [29], to name only a few. The ML is an intuitive, powerful and practical model that prevents the three limitations of the multinomial logit model by allowing for random taste variation, unre- stricted substitution patterns, and correlation in unob- served factors over time and space. McFadden and Train [30] prove that the ML model is a highly flexible model that can represent any RUM model. In order to realisti- cally represent the individual choice behavior, the ML model has become one of the most widely used models in the field of demand modeling over the years. Since ML is the most advanced and flexible model among dis- crete choice models, we used the ML model of travel mode choice in this paper. 4.2. Formulating a Mixed Logit Model An ML model includes a flexible random term ηin repre- senting additional unobservable factors, independent of εin, in its utility function [19,21,22,30]. The ML model is thus given by: Uin = Vin+ ηin + εin (3) As evident by the notation, the random terms ηin vary across both alternatives and decision makers. The re- searcher can assume any convenient and/or appropriate distribution for ηin. Consequently, the ML model is very flexible and free from restrictive assumption such as in- dependent of irrelevant attributes. Unfortunately, the ML choice probabilities have no longer closed form due to the presence of ηin in utility function of the model. As a result, we have to estimate the model with the help of some numerical solutions such as numerical integration, numerical approximation or simulation [19]. The choice probability of alternative i for decision maker n with the ML model is the integral of logit choice probability over the assumed distribution of random terms as given by: (|) ()d n inn nn PLif (4) where Ln(i|ηn) is the logit choice probability ofalterna- tive i for decision maker n conditional on ηn: (| ) in n V nn JV j e Li e (5) The ML models are normally derived either to allow flexible substitution patterns across alternatives or to accommodate random taste heterogeneity across decision makers [19,30]. The former approach gives rise to the error components logit (ECL) model and the latter to the random coefficients logit (RCL1) model. One can also develop more advanced model to allow for random taste heterogeneity, inter-alternative correlation, and hetero- scedasticity by combining the ECL-RCL approaches [22]. The ECL model allows some elements of η to be shared across some alternatives which in turn introduces corre- lation between the random terms of these alternatives. The ECL model thus closely resembles the models using a nesting structure such as nested logit that accommo- dates correlation across some of the alternative while it simultaneously accommodates random taste heterogene- ity and heteroscedasticity, which the nested logit model 1“Random coefficients logit” and “random parameters logit” are used interchangeably in literature. Copyright © 2011 SciRes. JTTS
 B. P. BHATTA39 does not [21]. The utility function of the ECL model is specified in such a way that error components (EC) create correla- tions among utilities of different alternatives [19]: Uin = Vin+ γn’zin + εin (6) where γn is a vector of random terms with zero mean and a covariance matrix Σ and zin is a vector of observed variables relating to alternative i. Put succinctly, zin is a vector of binary variables that indicate the EC entering the utility function of alternative i. The ECL model re- duces to the logit model if zin is 0 for all the alternatives meaning that the unobserved parts of the utility are un- correlated across alternatives. Naturally, the choice pro- bability with the ECL model does not have a closed form and thus requires the numerical processes to solve the inte- grals. The ECL model enables to estimate the error com- ponents that measure the relative sensitivity of changes in choice of different alternatives and to accommodate het- eroscedasticity in the unobserved influences on the choice. The choice probability of alternative i for deci- sion maker n with the ECL formulation of the ML is then obtained by integration over the distribution of γn [22]: ' ' 1 (|0,)d inn in jnn jn nn Vz inn n IVz j e P e (7) where ф (γn|0, Σ) is the joint normal density function of the elements in γn. In recent years, there has been a considerable interest in using ECL models in order to accommodate inter- alternative correlation and heteroscedasticity despite high cost of estimation (and hence application) and identifica- tion issues [31]. [21,32] are the two recent and the most notable applications of the ECL model structures to in- vestigate the factors influencing the time of day and mode choice. The ECL model is also applied to analyze the corporate bankruptcy and insolvency risk in Australia [29]. 4.3. Formulating the ECL Model of Mode Choice The ECL model was used to estimate the error compo- nents that measure the relative sensitivity of changes in mode choice and to accommodate heteroscedasticity in the unobserved influences on the mode choice. We ex- plored various possible specifications of the ECL models. Mainly, we focused on two specifications: 1) common unobserved factors between walking (WK) and cycling (CK) (non-motorized modes), and 2) common unob- served factors between car driving (CD) and car passen- ger (CP) (car modes), or common unobserved factors among CD, CP and public transport (PT) (motorized modes). As WK and CK are both non-motorized modes, the hypothesis is that they share unobserved factors that introduce correlation between the utilities of those alter- natives. Similarly, since CD, CP and PT are motorized modes, they presumably share unobserved factors that introduce correlation among the utilities of those alterna- tives. Among those specifications, we chose the one sharing the error components between alternatives be- longing to motorized and non-motorized modes. Based on the discussions above, we formulated the ECL model by adding the error components to the utility function as follows (by suppressing n): Ui = Vi+ σnmt. ζnmt. NMT(i) + σmt. ζmt. MT(i)+ εi (8) where ζnmt and ζmt are random variables drawn inde- pendently from the standard normal distribution, and σnmt and σmt are the standard deviations of the error compo- nents. σnmt and σmt are actually the elements of the vari- ance-covariance matrix capturing the correlation between WK and CK, and CD, CP and PT respectively. In this specification, NMT (i) is a dummy variable with 1 for the alternatives belonging to non-motorized modes and 0 otherwise. This dummy variable thus determines whether the error component relating to the non-motorized modes is included in the utility function of alternative i. Simi- larly, MT (i) is also a dummy variable with 1 for the al- ternatives belonging to the motorized modes and 0 oth- erwise and thus determines whether the error component relating to the motorized modes enter the utility function of alternative i. The utility of an alternative i contains at most one of those two error components. Estimation of this ECL model yields estimates of the parameters of the standard deviations of the error com- ponents (setting their mean to zero) in addition to the coefficients of the variables included in systematic utility functions. The variance of the error components related to nonmotorized modes is estimated by normalizing the variance of the error components of motorized modes to one because we can only identify the sum of the vari- ances in this particular formulation. The relative magni- tude of the variances of the error components associated with the non-motorized and motorized modes provide a measure of the relative sensitivity of these two modes to changes in the major attributes of travel modes such as travel time and cost components. Three types of variables such as characteristics of the journey, characteristics of a traveler and his/her house- holds, and performance of the transportation system as measured by the LOS attributes of different modes are included in systematic utility functions of travel modes [1,13]. Table 1 illustrates names and definitions of the variables including alternative specific constants (ASC) where the first two letters refer to the mode (i.e. utility function) where the variable enters. The models were Copyright © 2011 SciRes. JTTS
 B. P. BHATTA Copyright © 2011 SciRes. JTTS 40 Table 1. Variable (including ASCs) definitions. Variable Definition CK_00 ASC for CK alternative CD_00 ASC for CD alternative CP_00 ASC for CP alternative PT_00 ASC for PT alternative WK_dist Walking distance to get to work CK_dist Cycling distance to get to work CD_time Generic travel time by different travel modes GA_cost Generic travel cost by different travel modes PT_wktm Access/egress time to get to PT PT_invht Invehicle time with PT (in minutes) PT_wait Waiting time for PT PT_xfers Number of transfers to get to the work place with PT CP_female 1 if the traveler is female, 0 otherwise CD_parkgod 1 if guaranteed free parking at work , 0 otherwise CD_parkfair 1 if fair parking possibility at work, 0 otherwise CK_winter 1 if the trip was made during winter, 0 otherwise CD_soj 1 if the trip involves a secondary destination, 0 other- wise CD-dbfem 1 if good car access in the household, 0 otherwise CP_time Car time by CP PT_female 1 if the traveler is female, 0 otherwise CD_tmrush Car time for the trip made during peak to Oslo coded and estimated in BIOGEME [33] using 1,000 random draws. A set of “reasonable models” were for- mulated (and reformulated) and estimated (and re-esti- mated) based on a priori-consideration. The systematic process of model building led to the final specification (Table 3) based on goodness-of-fit measures, statistical tests and informal tests. In addition to the ECL model of mode choice esti- mated on nylos, four ECL models on different specifica- tions of striplos with identical specification of systematic utility function were estimated as follows: · Model 1 (base case): The first model was estimated on the LOS attributes at “face value” except the correc- tion for missing direction and unreasonable asymmetry in LOS attributes. · Model 2: In the second model, public transport fares in Oslo were corrected, but the fares in the rest of the region and between Oslo and the rest of the region were not adjusted. · Model 3: In the third model, missing toll in Oslo was corrected for in addition to the correction made in the Model 2. · Model 4: In the fourth model, an attempt was made to account for congestion in Oslo in addition to the cor- rection made in the Model 3 since striplos did not have separate LOS values for peak and off-peak hours. Re- spondents had reported when the trip was taken and this enabled us to adjust for congestion by using a variable where driving time interacts with a dummy for peak travel, i.e., instead of higher driving times during peak hours, the model had additional coefficient for driving time. This variable was added to the utility of car driving in the model First the differences in LOS attributes between striplos and nylos are discussed. Then estimation results of the models based on statistical significance of coefficients, goodness-of-fit measures such as final log-likelihood, log-likelihood ratio index (2 ) and adjusted log-like- lihood ratio index (2 ), expected signs of the coeffi- ents and relative magnitudes of the coefficients within each model are compared. The implied value of time (VOT), the trade-off between travel time and travel cost, Table 2. Differences in LOS attributes. Attribute Mean diff. Var. Minm. Maxm. Mean diff. /Mean nylos R2 of linear regn2 CD_time –5.35 41.79 –51.24 20.36 –0.151 0.937 CD_cost –5.83 47.64 –44.30 16.17 –0.144 0.979 PT_wktm –2.83 629.18 –240.98 129.73 –0.080 0.205 PT_invht –2.85 224.36 –121.42 87.16 –0.064 0.835 PT_wait 6.65 256.21 –62.50 165.00 0.392 0.515 PT_xfers 0.44 1.02 –3.03 4.00 0.641 0.432 PT_fare 11.68 781.32 –55.20 163.20 0.301 0.605 WK_dost –1.40 6.95 –31.64 13.85 –0.051 0.991 PT_fare_c 8.81 706.42 –55.20 163.20 0.227 0.611 CD_cost_c –1.84 12.86 –44.30 17.59 –0.046 0.993 2Striplos = a + b Nylos + u, where a, b, u, striplos, and nylos are intercept, coefficient, error term, the values of the respective attributes of striplos and nylos respectively. This may not be theoretically meaningful relation. But the purpose was to have some indication of the extent of importance of systematic and random components between the respective LOS attributes.
 B. P. BHATTA Copyright © 2011 SciRes. JTTS 41 was chosen as a measure of relative magnitude of coeffi- ents within a model. Additionally, market shares of dif- rent travel modes and aggregate direct and cross elastic- ies under different policy scenarios are compared. 5. Results and Discussion This section presents and discusses the results regarding differences in LOS attributes, estimation results of mod- s, aggregate elasticity and aggregate forecasting. 5.1. Differences in LOS Attributes First, the actual differences and degree of correlation between the corresponding LOS attributes of the two data sets were examined. Table 2 summarizes statistics on difference between striplos and nylos. As expected for the base case, nylos gave, on average, higher values for car time and car cost. After correction for road tolls, the mean and standard deviation of the difference became much smaller for car cost (CD_cost_c). The remaining difference can mainly be attributed to differences in driving distance caused by differences in coding of the road network. But correction for public transport fare (PT_fare_c) did not help much to reduce the difference. The mean differences were smaller both in absolute and relative terms with access/egress time (PT_wktm) and invehicle time of public transport (PT_invht), but the variance was much greater, especially for PT_wktm. The striplos had, on average, higher values and the differ- ences were relatively big for waiting time (PT_wait) and number of transfers (PT_xfers) of public transport. This probably reflects a mixture of coding and different as- signment algorithms. The distance based function used to estimate public transport fares for striplos obviously overestimated the fares significantly and a relatively large difference persisted even after the correction for the fares pertaining to internal trips in Oslo. The difference between the LOS attributes in the two data sets is a mixture of systematic differences in the mean values and a “random” component. The extent of the random component varies between the attributes and is reflected in the ratio between standard deviation and mean value of the differences and in R2 (given in the last column) if we run a linear regression between the re- spective attributes in the two data sets. The random com- ponent is the most important for PT_wktm, PT_wait and PT_xfers based on the R2. 5.2. Estimation Results Since the models were formulated and reformulated in a number of ways during the model building process, a substantial body of empirical results was generated. However, this section analyzes the results of the final models with the best specification based on iterative process of model building. Table 3 summarizes the estimation results of the ECL models on both the data sets. The estimation results of the ECL model yielded the parameters for the standard deviations of the error components in addition to the co- efficients of the variables included in the systematic util- ity functions of the models. The variance of the error components related to nonmotorized modes was esti- mated by normalizing the variance of the error compo- nents of motorized modes to one because we can only identify the sum of the variances in this particular for- mulation. Contrary to the hypothesis, σnmt was not statis- tically significant in all the models, likely indicating that there is no significant common unobserved factors and heteroscedasticity across the alternatives. The estimation results of the “base case” on striplos looked reasonably good. All the coefficients were statis- tically significant and had the expected signs according to theory and the previous results except the number of transfers of public transport (PT_xfers). PT_xfers was significant, but had the wrong sign. It was also the case in the estimation of the national model that used the whole sample (Madslien, et al., 2005). As a result, the number of transfers was not included in the final model. But we included it in this study in order to compare the effects of different corrections to LOS attributes. The implied value of time (VOT) of car driver seemed low (Table 4). Without any further improvement of the LOS- data, this model might have been re-estimated without PT transfers in the model if the purpose of the study is not to estimate the VOTs and the transfer vari- able is not needed in the analysis. We tried this and the results were good enough based on signs, significance and relative values of the coefficients, and goodness-of- fit measures. In the second model on striplos, we used the corrected PT fare for the trips within Oslo instead of the fare esti- mated from travel distance. However, the distance based fare was still used for other combinations of origin-des- tination although the actual fare system was based on ‘fare zones’ and the number of fare zones tranversed. All the coefficients, except PT_xfers, were significant with expected signs and reasonable magnitudes. PT_xfers had still the wrong sign but significant at lower confidence level. The VOTs in this estimation came close to ‘official values’ used in cost benefit analyses in Norway. Sur- prisingly, the model gave a poorer fit as measured by the value of the log-likelihood function at maximum. In Model 3, introducing both corrected public trans- port fares and road tolls resulted in a further drop of odel fit! The VOTs slightly decreased compared to the m
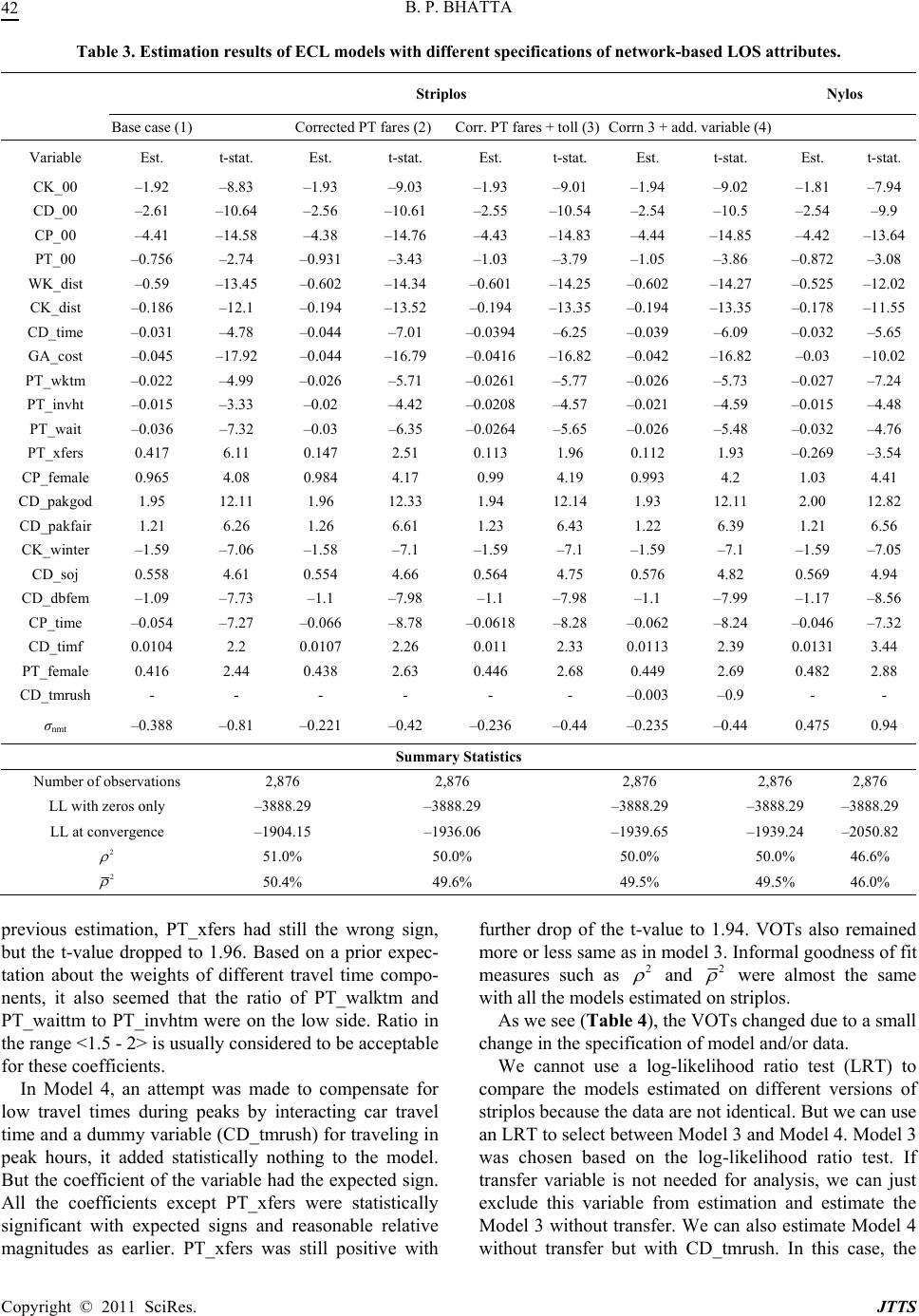 B. P. BHATTA Copyright © 2011 SciRes. JTTS 42 Table 3. Estimation results of ECL models with different specifications of network-based LOS attributes. Striplos Nylos Base case (1) Corrected PT fares (2) Corr. PT fares + toll (3)Corrn 3 + add. variable (4) Variable Est. t-stat. Est. t-stat. Est. t-stat. Est. t-stat. Est. t-stat. CK_00 –1.92 –8.83 –1.93 –9.03 –1.93 –9.01 –1.94 –9.02 –1.81 –7.94 CD_00 –2.61 –10.64 –2.56 –10.61 –2.55 –10.54 –2.54 –10.5 –2.54 –9.9 CP_00 –4.41 –14.58 –4.38 –14.76 –4.43 –14.83 –4.44 –14.85 –4.42 –13.64 PT_00 –0.756 –2.74 –0.931 –3.43 –1.03 –3.79 –1.05 –3.86 –0.872 –3.08 WK_dist –0.59 –13.45 –0.602 –14.34 –0.601 –14.25 –0.602 –14.27 –0.525 –12.02 CK_dist –0.186 –12.1 –0.194 –13.52 –0.194 –13.35 –0.194 –13.35 –0.178 –11.55 CD_time –0.031 –4.78 –0.044 –7.01 –0.0394 –6.25 –0.039 –6.09 –0.032 –5.65 GA_cost –0.045 –17.92 –0.044 –16.79 –0.0416 –16.82 –0.042 –16.82 –0.03 –10.02 PT_wktm –0.022 –4.99 –0.026 –5.71 –0.0261 –5.77 –0.026 –5.73 –0.027 –7.24 PT_invht –0.015 –3.33 –0.02 –4.42 –0.0208 –4.57 –0.021 –4.59 –0.015 –4.48 PT_wait –0.036 –7.32 –0.03 –6.35 –0.0264 –5.65 –0.026 –5.48 –0.032 –4.76 PT_xfers 0.417 6.11 0.147 2.51 0.113 1.96 0.112 1.93 –0.269 –3.54 CP_female 0.965 4.08 0.984 4.17 0.99 4.19 0.993 4.2 1.03 4.41 CD_pakgod 1.95 12.11 1.96 12.33 1.94 12.14 1.93 12.11 2.00 12.82 CD_pakfair 1.21 6.26 1.26 6.61 1.23 6.43 1.22 6.39 1.21 6.56 CK_winter –1.59 –7.06 –1.58 –7.1 –1.59 –7.1 –1.59 –7.1 –1.59 –7.05 CD_soj 0.558 4.61 0.554 4.66 0.564 4.75 0.576 4.82 0.569 4.94 CD_dbfem –1.09 –7.73 –1.1 –7.98 –1.1 –7.98 –1.1 –7.99 –1.17 –8.56 CP_time –0.054 –7.27 –0.066 –8.78 –0.0618 –8.28 –0.062 –8.24 –0.046 –7.32 CD_timf 0.0104 2.2 0.0107 2.26 0.011 2.33 0.0113 2.39 0.0131 3.44 PT_female 0.416 2.44 0.438 2.63 0.446 2.68 0.449 2.69 0.482 2.88 CD_tmrush - - - - - - –0.003 –0.9 - - σnmt –0.388 –0.81 –0.221 –0.42 –0.236 –0.44 –0.235 –0.44 0.475 0.94 Summary Statistics Number of observations 2,876 2,876 2,876 2,876 2,876 LL with zeros only –3888.29 –3888.29 –3888.29 –3888.29 –3888.29 LL at convergence –1904.15 –1936.06 –1939.65 –1939.24 –2050.82 2 51.0% 50.0% 50.0% 50.0% 46.6% 2 50.4% 49.6% 49.5% 49.5% 46.0% previous estimation, PT_xfers had still the wrong sign, but the t-value dropped to 1.96. Based on a prior expec- tation about the weights of different travel time compo- nents, it also seemed that the ratio of PT_walktm and PT_waittm to PT_invhtm were on the low side. Ratio in the range <1.5 - 2> is usually considered to be acceptable for these coefficients. In Model 4, an attempt was made to compensate for low travel times during peaks by interacting car travel time and a dummy variable (CD_tmrush) for traveling in peak hours, it added statistically nothing to the model. But the coefficient of the variable had the expected sign. All the coefficients except PT_xfers were statistically significant with expected signs and reasonable relative magnitudes as earlier. PT_xfers was still positive with further drop of the t-value to 1.94. VOTs also remained more or less same as in model 3. Informal goodness of fit measures such as 2 and 2 were almost the same with all the models estimated on striplos. As we see (Table 4 ), the VOTs changed due to a small change in the specification of model and/or data. We cannot use a log-likelihood ratio test (LRT) to compare the models estimated on different versions of striplos because the data are not identical. But we can use an LRT to select between Model 3 and Model 4. Model 3 was chosen based on the log-likelihood ratio test. If transfer variable is not needed for analysis, we can just exclude this variable from estimation and estimate the Model 3 without transfer. We can also estimate Model 4 without transfer but with CD_tmrush. In this case, the
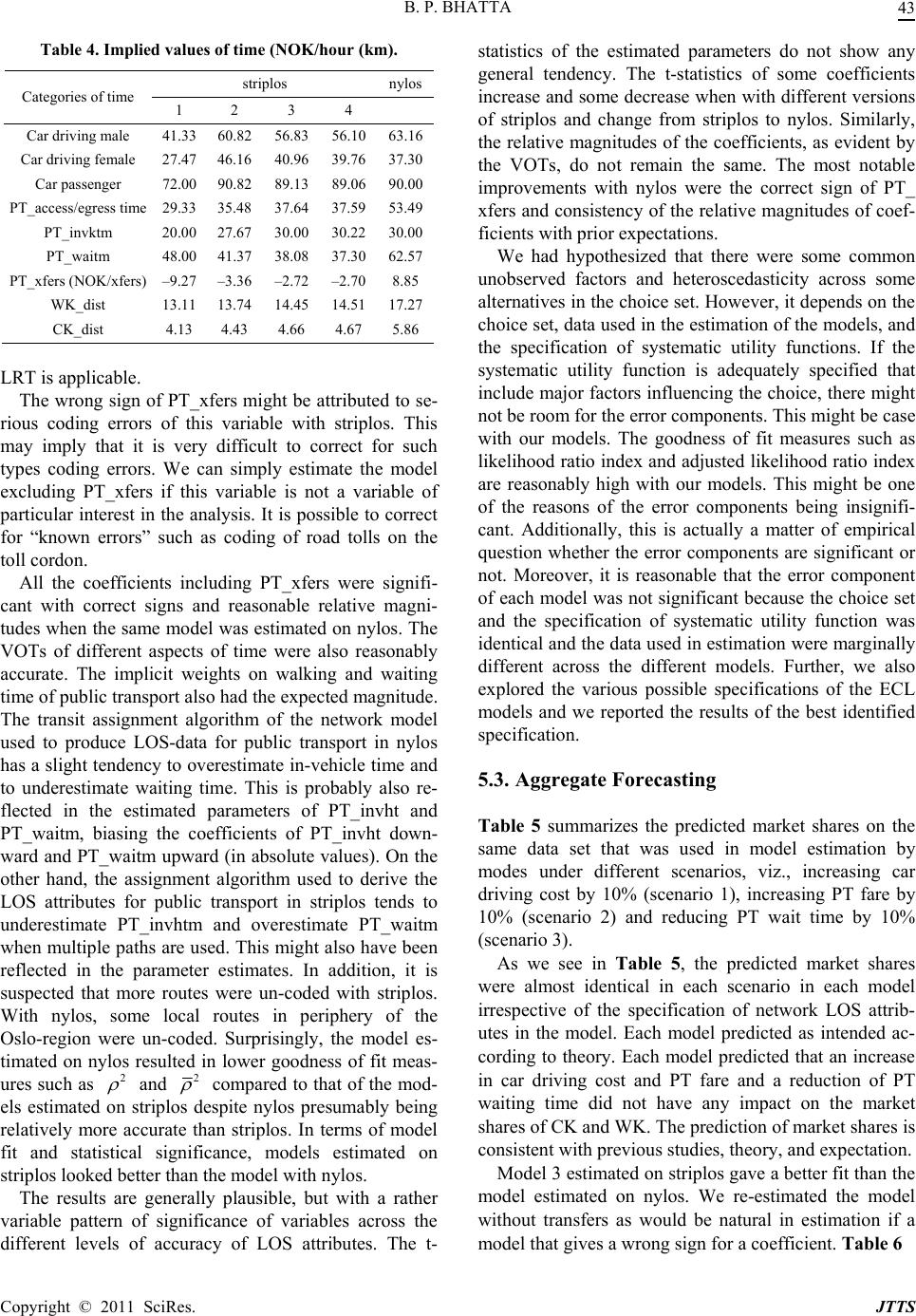 B. P. BHATTA43 Table 4. Implied values of time (NOK/hour (km). striplos nylos Categories of time 1 2 3 4 Car driving male 41.33 60.82 56.83 56.10 63.16 Car driving female 27.47 46.16 40.96 39.7637.30 Car passenger 72.00 90.82 89.13 89.0690.00 PT_access/egress time 29.33 35.48 37.64 37.59 53.49 PT_invktm 20.00 27.67 30.00 30.2230.00 PT_waitm 48.00 41.37 38.08 37.30 62.57 PT_xfers (NOK/xfers) –9.27 –3.36 –2.72 –2.708.85 WK_dist 13.11 13.74 14.45 14.5117.27 CK_dist 4.13 4.43 4.66 4.67 5.86 LRT is applicable. The wrong sign of PT_xfers might be attributed to se- rious coding errors of this variable with striplos. This may imply that it is very difficult to correct for such types coding errors. We can simply estimate the model excluding PT_xfers if this variable is not a variable of particular interest in the analysis. It is possible to correct for “known errors” such as coding of road tolls on the toll cordon. All the coefficients including PT_xfers were signifi- cant with correct signs and reasonable relative magni- tudes when the same model was estimated on nylos. The VOTs of different aspects of time were also reasonably accurate. The implicit weights on walking and waiting time of public transport also had the expected magnitude. The transit assignment algorithm of the network model used to produce LOS-data for public transport in nylos has a slight tendency to overestimate in-vehicle time and to underestimate waiting time. This is probably also re- flected in the estimated parameters of PT_invht and PT_waitm, biasing the coefficients of PT_invht down- ward and PT_waitm upward (in absolute values). On the other hand, the assignment algorithm used to derive the LOS attributes for public transport in striplos tends to underestimate PT_invhtm and overestimate PT_waitm when multiple paths are used. This might also have been reflected in the parameter estimates. In addition, it is suspected that more routes were un-coded with striplos. With nylos, some local routes in periphery of the Oslo-region were un-coded. Surprisingly, the model es- timated on nylos resulted in lower goodness of fit meas- ures such as 2 and 2 compared to that of the mod- els estimated on striplos despite nylos presumably being relatively more accurate than striplos. In terms of model fit and statistical significance, models estimated on striplos looked better than the model with nylos. The results are generally plausible, but with a rather variable pattern of significance of variables across the different levels of accuracy of LOS attributes. The t- statistics of the estimated parameters do not show any general tendency. The t-statistics of some coefficients increase and some decrease when with different versions of striplos and change from striplos to nylos. Similarly, the relative magnitudes of the coefficients, as evident by the VOTs, do not remain the same. The most notable improvements with nylos were the correct sign of PT_ xfers and consistency of the relative magnitudes of coef- ficients with prior expectations. We had hypothesized that there were some common unobserved factors and heteroscedasticity across some alternatives in the choice set. However, it depends on the choice set, data used in the estimation of the models, and the specification of systematic utility functions. If the systematic utility function is adequately specified that include major factors influencing the choice, there might not be room for the error components. This might be case with our models. The goodness of fit measures such as likelihood ratio index and adjusted likelihood ratio index are reasonably high with our models. This might be one of the reasons of the error components being insignifi- cant. Additionally, this is actually a matter of empirical question whether the error components are significant or not. Moreover, it is reasonable that the error component of each model was not significant because the choice set and the specification of systematic utility function was identical and the data used in estimation were marginally different across the different models. Further, we also explored the various possible specifications of the ECL models and we reported the results of the best identified specification. 5.3. Aggregate Forecasting Table 5 summarizes the predicted market shares on the same data set that was used in model estimation by modes under different scenarios, viz., increasing car driving cost by 10% (scenario 1), increasing PT fare by 10% (scenario 2) and reducing PT wait time by 10% (scenario 3). As we see in Table 5, the predicted market shares were almost identical in each scenario in each model irrespective of the specification of network LOS attrib- utes in the model. Each model predicted as intended ac- cording to theory. Each model predicted that an increase in car driving cost and PT fare and a reduction of PT waiting time did not have any impact on the market shares of CK and WK. The prediction of market shares is consistent with previous studies, theory, and expectation. Model 3 estimated on striplos gave a better fit than the model estimated on nylos. We re-estimated the model without transfers as would be natural in estimation if a model that gives a wrong sign for a coefficient. Table 6 Copyright © 2011 SciRes. JTTS
 B. P. BHATTA 44 Table 5. Predicted market shares by modes in different sce- narios. Travel modes WKCK CD CP PT Actual market shares 8.26.3 51.6 4.9 29.0 Predicted market shares Model 1_S 8.36.5 49.8 5.3 30.1 Model 2_S 8.26.5 49.9 5.3 30.1 Scenario 1 Model 3_S 8.26.5 49.7 5.3 30.2 Model 4_S 8.26.5 49.7 5.3 30.2 Nylos 8.26.4 49.9 5.1 30.3 Model 1_S 8.36.6 52.9 5.2 27.0 Model 2_S 8.36.5 52.8 5.2 27.3 Scenario 2 Model 3_S 8.36.5 52.7 5.1 27.3 Model 4_S 8.36.5 52.7 5.1 27.3 Nylos 8.36.5 52.6 5.1 27.5 Model 1_S 8.26.3 51.1 4.8 29.7 Model 2_S 8.26.3 51.1 4.8 29.6 Scenario 3 Model 3_S 8.26.3 51.2 4.8 29.6 Model 4_S 8.26.3 51.2 4.8 29.5 Nylos 8.26.3 51.1 4.8 29.6 Table 6. Implied demand elasticities—simulated on sample. Direct Cross CD PT Striplos 3 without xfers –0.32 0.46 Scenario 1 Nylos –0.31 0.48 PT CD Striplos 3 without xfers –0.60 0.22 Scenario 2 Nylos –0.54 0.20 Striplos 3 without xfers –0.17 0.06 Scenario 3 Nylos –0.19 0.08 presents implied direct and cross- elasticities respectively with the models estimated on both nylos and striplos. Both the models yielded direct and cross elasticities as expected according to theory, i.e., negative direct elastic- ity and positive cross elasticity for the attributes consid- ered. Both the direct and cross elasticities are inelastic and just the opposite in scenario 2. The cross elasticities of CD cost were significantly higher than the own elas- ticities with scenario 1. The implied demand elasticities were almost similar in each scenario. The main differ- ence was a moderately lower both direct and cross elas- ticities of PT waiting time of the model estimated on striplos. The models should thus give the similar conclu- sions for policy purposes. 6. Summary and Conclusions The need for travel demand models is growing world- wide. Obtaining reasonably accurate LOS attributes of transportation system for different travel modes, the ma- jor factors shaping the travel demand, is not a trivial task. The objective of the paper was therefore to investigate the effects of using LOS attributes measured at different levels of accuracy on the results of disaggregate travel mode choice models. The case study in this paper is an example of what might happen practically when we cor- rect for ‘known errors’ in the data set or switch to the data set with better quality. The sensitivity of model re- sults including goodness of fit measures, VOTs and ag- gregate forecasting were compared by estimating ECL models for travel mode choice on the two data sets of LOS attributes. The difference between the LOS attrib- utes in the two data sets was a mixture of systematic dif- ferences in the mean values and a random component. The extent of the random component varied between the attributes. Striplos yielded generally better fit and reasonably satisfactory models statistically. But number of transfers had wrong sign and VOTs were generally low without any correction. The correction helped to get VOTs of reasonable magnitudes. Model estimated on nylos on the other hand had all the significant coefficients with cor- rect sings including number of transfers, the reasonable relative magnitude of coefficients of public transport travel components and reasonably plausible VOT esti- mates except slightly less model fit compared to the model on striplos. Models estimated on both striplos and nylos gave almost similar aggregate forecasting and ag- gregate elasticities on the same data set used in estima- tion. During the model building process, it was also ob- served that the VOTs changed significantly due to a small change in the specification of model and data im- plying that utmost care must be taken for specification of data and model if the purpose of the study is to estimate VOTs. The lack of peak hour driving time in striplos appeared less important for parameter estimates than it was ini- tially expected. This may not hold true in general since having a model that accounts for congestion correctly ought to give better results in an urban setting. All the models predicted well implying that specifica- tion of LOS attributes matters less for prediction as long as the predictions are done with the same data. The re- quirement of data accuracy depends on the purpose of developing a model since the model with relatively in- accurate data also predicts reasonably well. Measuring data as accurately as possible is presumably more im- portant if the purpose of the study is to estimate VOTs. 7. Acknowledgements I am thankful to Odd I. Larsen, John K. Dagsvik and Copyright © 2011 SciRes. JTTS
 B. P. BHATTA45 anonymous reviewers for their help and compliments on earlier draft of this work. The author is fully responsible for any errors and omissions. 8. References [1] J. D. Ortúzar and L.G. Willumsen, “Modeling Transport,” 3rd Edition, John Wiley & Sons Ltd, England, 2001. [2] J. Walker, L. Jieping, S. Sirinivasan and D. Bolduc, “Travel Demand Models in the Developing World: Cor- recting for Measurement Errors,” TRB 87th Annual Meeting Compendium of Papers DVD, Washington D.C., 13-17 January 2008. [3] A. Madslien, J. Rekdal and O. I. Larsen, “Development of Regional Models for Passenger Transport in Norway,” (in Norwegian with English Summary), Report 766/2005, Institute of Transport Economics, Oslo, Norway, 1 July, 2005. [4] J. Rekdal, and O. I. Larsen, “Regional Modell for Oslo- området: Dokumentasjon av Utviklinsarbeid og Teknisk Innføring i Anvendelse (RTM23+),” (in Norwegian with English summary), Rapport, Møreforsking Molde, Nor- way, 16-18 July 2008. [5] W. Alonso, “Predicting Best with Imperfect Data,” Jour- nal of the American Planning Association, Vol. 34, No. 4, 1968, pp. 248-255. doi:10.1080/01944366808977813 [6] A. Daly and J. D. Ortuzar, “Forecasting and Data Aggre- gation: Theory and Practice,” Traffic Engineering and Control, Vol. 31, No. 12, 1990, pp. 632-643. [7] F. A. Reid, and K. Small, “Effects of Temporal Disag- gregation of Trip Data on Traveler Behavior Models,” Transportation Research Forum Annual Meeting, MIT, Mass., 1976. [8] K. Train, “The Sensitivity of Parameter Estimates to Data Specification in Mode Choice Models,” Transportation, Vol. 7, 1978, pp. 301-309. doi:10.1007/BF00165499 [9] J. D. Ortúzar, and A. Ivelic, “Efectos de la Desagregación Temporal de Variables de Servicio en la Especificación y Estabilidad de Funciones de Demanda,” Actas del IV Congreo Panamerican de Ingenieria de Transito y Trans- porte, Santiago, Chile, December, (in Spanish), 1986. [10] J. D. Ortúzar, and A. Ivelic, “Effects of More Accurately Measured Level of Service Variables on the Specification and Stability of Mode Choice Models,” Proceedings of Seminar C held at the PTRC summer annual meeting, University of Bath, England, 7-11 September, PTRC E- ducation and Research Ltd, 1987. [11] S.S.C Steimetz and D. Brownstone, “Estimating Com- muters’ Value of Time with Noisy Data: A Multiple im- putation Approach,” Transportation Research B, Vol. 39, 2005, pp. 865-889. doi:10.1016/j.trb.2004.11.001 [12] A. Monzon and A. Rodriguez-Dapena, “Choice of Mode of Transport for Long-Distance Trips: Solving the Prob- lem of Sparse Data,” Transportation Research A, Vol. 40, 2006. 587-601. doi:10.1016/j.tra.2005.11.007 [13] B. P. Bhatta, “Discrete Choice Analysis with Applica- tions to Travel Demand,” Ph.D. thesis, Molde University College, Molde, Norway, 2009. [14] B. P. Bhatta and O. I. Larsen, “Errors in Variables in Multinomial Choice Modeling: A Simulation Study Ap- plied to a Multinomial Logit model of Travel Mode Choice,” Transport Policy, Vol. 18, 2011, pp. 326-335. doi:10.1016/j.tranpol.2010.10.002 [15] J. M. Denstadli, R. Hjorthol, A. Rideng and J. I. Lian, “Den Norske Befolkningens Reiser,” (in Norwegian with English summary) TØI rapport 637/2003, Institute of Transport Economics, Oslo, 2003. [16] B. P. Bhatta and O. I. Larsen, “Are Intrazonal Trips Ig- norable?” Transport Policy, Vol. 18, 2011, pp. 13-22. doi:10.1016/j.tranpol.2010.04.004 [17] M. Ben-Akiva and S. R. Lerman, “Discrete Choice Analysis: Theory and Application to Travel Demand,” MIT Press, Cambridge, Mass. 1985. [18] K. Train, “Qualitative Choice Analysis: Theory, Econo- metrics and Application to Automobile Demand,” MIT Press, Cambridge, Mass., 1986. [19] K. Train, “Discrete Choice Methods with Simulation,” Cambridge University Press, Cambridge, UK. 2003. doi:10.1017/CBO9780511753930 [20] D. McFadden, “Disaggregate Behavioral Travel De- mand’s RUM Side: A 30-Year Perspective,” Paper pre- sented at Conference on the International Association of Travel Behavior Research, July 2, Brisbane, Australia, 2000. [21] S. Hess, J. W. Polak, A. Daly and G. Hyman, “Flexible Substitution Patterns in Models of Mode and Time of Day Choice: New Evidence from the UK and the Nether- lands,” Transportation, Vol. 34, 2007, pp. 213-238. doi:10.1007/s11116-006-0011-7 [22] S. Hess, “Advanced Discrete Choice models with Appli- cations to Transport Demand,” Ph.D. thesis. Center for Transport Studies, Imperial College London, 2005. [23] J. H. Boyd and R. E. Mellman, “The Effect of Fuel Economy Standards on the U.S. Automotive Market: A Hedonic Demand Analysis,” Transportation Research A, Vol. 14, 1980, pp. 367-378. doi:10.1016/0191-2607(80)90055-2 [24] N. S. Cardell and F. C. Dunbar, “Measuring the Societal Impacts of Automobile Downsizing,” Transportation Research A, Vol. 14, 1980, pp. 423-434. doi:10.1016/0191-2607(80)90060-6 [25] G. Glasgow, “Mixed Logit Models for Multiparty Elec- tions,” Political Analysis, Vol. 9, 2001, pp. 116-136. [26] J. A. Herriges and D. J. Phaneuf, “Inducing Patterns of Correlation and Substitution in Repeated Logit Models of Recreation Demand,” American Journal of Agricultural Economics, Vol. 84, 2002, pp. 1076-1090. doi:10.1111/1467-8276.00055 [27] S. Hess and J. W. Polak, “Accounting for Random Taste Heterogeneity in Airport-Choice Modeling,” Transporta- tion Research Record, Vol. 1915, 2005, pp. 36-43. doi:10.3141/1915-05 Copyright © 2011 SciRes. JTTS
 B. P. BHATTA Copyright © 2011 SciRes. JTTS 46 [28] C. P. Barros and I. Proenca, “Mixed Logit Estimation of Radical Islamic Terrorism in Europe and North America: A Comparative Study,” Journal of Conflict Resolution, Vol. 49, 2005. 298-314. doi:10.1177/0022002704272829 [29] D. Hensher, J. Steward and W. H. Greene, “An Error Component Analysis of Corporate Bankruptcy and In- solvency Risk in Australia,” Economic Record, Vol. 83, 2007, pp. 86-103. doi:10.1111/j.1475-4932.2007.00378.x [30] D. McFadden and K. Train, “The mixed MNL Models for Discrete Response,” Journal of Applied Econometrics, Vol. 15, 2000, pp. 447-470. doi:10.1002/1099-1255(200009/10)15:5<447::AID-JAE5 70>3.0.CO;2-1 [31] J. Walker, M. Ben-Akiva and D. Bolduc, “Identification of Parameters in Normal Error Components Logit Mix- ture Models,” Journal of Applied Econometrics, Vol. 22, 2007, pp. 1095-1125. doi:10.1002/jae.971 [32] G. de Jong, A. Daly, M. Pieters , C. Vellay, M. A. Brad- ley and F. Hofman, “A Model for Time of Day and Mode Choice Using Error components Logit,” Transportation Research E, Vol. 39, 2003, pp. 245-268. doi:10.1016/S1366-5545(02)00037-6 [33] M. Bierlaire, “BIOGEME: A Free Package for the Esti- mation of Discrete Choice Models,” Proceedings of the 3rd Swiss Transportation Research Conference, Ascona, Switzerland, March 2003. (http://biogeme.epfl.ch)
|