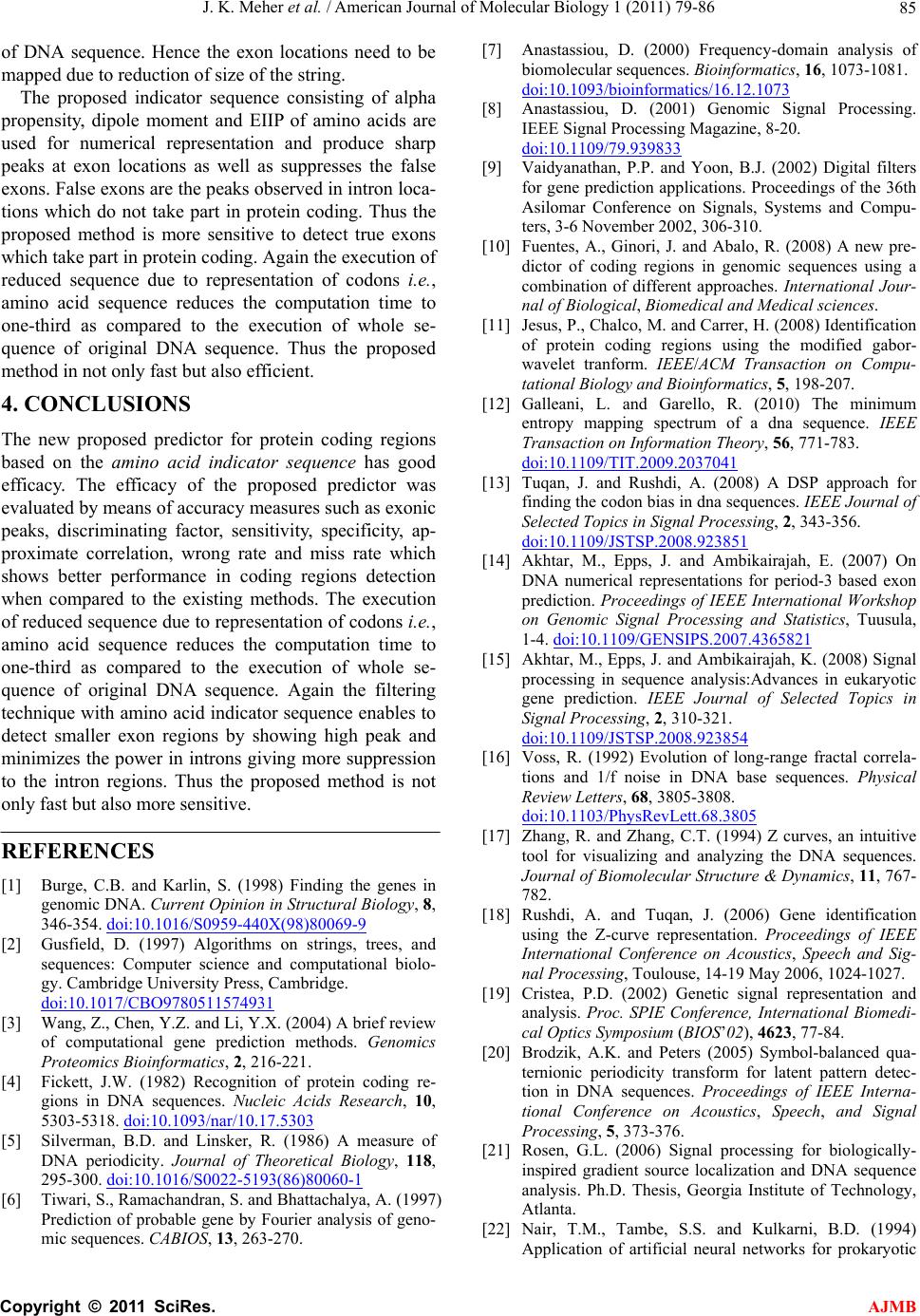
J. K. Meher et al. / American Journal of Molecular Biology 1 (2011) 79-86 85
of DNA sequence. Hence the exon locations need to be
mapped due to reduction of size of the string.
The proposed indicator sequence consisting of alpha
propensity, dipole moment and EIIP of amino acids are
used for numerical representation and produce sharp
peaks at exon locations as well as suppresses the false
exons. False exons are the peaks observed in intron loca-
tions which do not take part in protein coding. Thus the
proposed method is more sensitive to detect true exons
which take part in protein coding. Again the execution of
reduced sequence due to representation of codons i.e.,
amino acid sequence reduces the computation time to
one-third as compared to the execution of whole se-
quence of original DNA sequence. Thus the proposed
method in not only fast but also efficient.
4. CONCLUSIONS
The new proposed predictor for protein coding regions
based on the amino acid indicator sequence has good
efficacy. The efficacy of the proposed predictor was
evaluated by means of accuracy measures such as exonic
peaks, discriminating factor, sensitivity, specificity, ap-
proximate correlation, wrong rate and miss rate which
shows better performance in coding regions detection
when compared to the existing methods. The execution
of reduced sequence due to representation of codons i.e.,
amino acid sequence reduces the computation time to
one-third as compared to the execution of whole se-
quence of original DNA sequence. Again the filtering
technique with amino acid indicator sequence enables to
detect smaller exon regions by showing high peak and
minimizes the power in introns giving more suppression
to the intron regions. Thus the proposed method is not
only fast but also more sensitive.
REFERENCES
[1] Burge, C.B. and Karlin, S. (1998) Finding the genes in
genomic DNA. Current Opinion in Structural Biology, 8,
346-354. doi:10.1016/S0959-440X(98)80069-9
[2] Gusfield, D. (1997) Algorithms on strings, trees, and
sequences: Computer science and computational biolo-
gy. Cambridge University Press, Cambridge.
doi:10.1017/CBO9780511574931
[3] Wang, Z., Chen, Y.Z. and Li, Y.X. (2004) A brief review
of computational gene prediction methods. Genomics
Proteomics Bioinformatics, 2, 216-221.
[4] Fickett, J.W. (1982) Recognition of protein coding re-
gions in DNA sequences. Nucleic Acids Research, 10,
5303-5318. doi:10.1093/nar/10.17.5303
[5] Silverman, B.D. and Linsker, R. (1986) A measure of
DNA periodicity. Journal of Theoretical Biology, 118,
295-300. doi:10.1016/S0022-5193(86)80060-1
[6] Tiwari, S., Ramachandran, S. and Bhattachalya, A. (1997)
Prediction of probable gene by Fourier analysis of geno-
mic sequences. CABIOS, 13, 263-270.
[7] Anastassiou, D. (2000) Frequency-domain analysis of
biomolecular sequences. Bioinformatics, 16, 1073-1081.
doi:10.1093/bioinformatics/16.12.1073
[8] Anastassiou, D. (2001) Genomic Signal Processing.
IEEE Signal Processing Magazine, 8-20.
doi:10.1109/79.939833
[9] Vaidyanathan, P.P. and Yoon, B.J. (2002) Digital filters
for gene prediction applications. Proceedings of the 36th
Asilomar Conference on Signals, Systems and Compu-
ters, 3-6 November 2002, 306-310.
[10] Fuentes, A., Ginori, J. and Abalo, R. (2008) A new pre-
dictor of coding regions in genomic sequences using a
combination of different approaches. International Jour-
nal of Biological, Biomedical and Medical sciences.
[11] Jesus, P., Chalco, M. and Carrer, H. (2008) Identification
of protein coding regions using the modified gabor-
wavelet tranform. IEEE/ACM Transaction on Compu-
tational Biology and Bioinformatics, 5, 198-207.
[12] Galleani, L. and Garello, R. (2010) The minimum
entropy mapping spectrum of a dna sequence. IEEE
Transaction on Information Theory, 56, 771-783.
doi:10.1109/TIT.2009.2037041
[13] Tuqan, J. and Rushdi, A. (2008) A DSP approach for
finding the codon bias in dna sequences. IEEE Journal of
Selected Topics in Signal Processing, 2, 343-356.
doi:10.1109/JSTSP.2008.923851
[14] Akhtar, M., Epps, J. and Ambikairajah, E. (2007) On
DNA numerical representations for period-3 based exon
prediction. Proceedings of IEEE International Workshop
on Genomic Signal Processing and Statistics, Tuusula,
1-4. doi:10.1109/GENSIPS.2007.4365821
[15] Akhtar, M., Epps, J. and Ambikairajah, K. (2008) Signal
processing in sequence analysis:Advances in eukaryotic
gene prediction. IEEE Journal of Selected Topics in
Signal Processing, 2, 310-321.
doi:10.1109/JSTSP.2008.923854
[16] Voss, R. (1992) Evolution of long-range fractal correla-
tions and 1/f noise in DNA base sequences. Physical
Review Letters, 68, 3805-3808.
doi:10.1103/PhysRevLett.68.3805
[17] Zhang, R. and Zhang, C.T. (1994) Z curves, an intuitive
tool for visualizing and analyzing the DNA sequences.
Journal of Biomolecular Structure & Dynamics, 11, 767-
782.
[18] Rushdi, A. and Tuqan, J. (2006) Gene identification
using the Z-curve representation. Proceedings of IEEE
International Conference on Acoustics, Speech and Sig-
nal Processing, Toulouse, 14-19 May 2006, 1024-1027.
[19] Cristea, P.D. (2002) Genetic signal representation and
analysis. Proc. SPIE Conference, International Biomedi-
cal Optics Symposium (BIOS’02), 4623, 77-84.
[20] Brodzik, A.K. and Peters (2005) Symbol-balanced qua-
ternionic periodicity transform for latent pattern detec-
tion in DNA sequences. Proceedings of IEEE Interna-
tional Conference on Acoustics, Speech, and Signal
Processing, 5, 373-376.
[21] Rosen, G.L. (2006) Signal processing for biologically-
inspired gradient source localization and DNA sequence
analysis. Ph.D. Thesis, Georgia Institute of Technology,
Atlanta.
[22] Nair, T.M., Tambe, S.S. and Kulkarni, B.D. (1994)
Application of artificial neural networks for prokaryotic
C
opyright © 2011 SciRes. AJMB