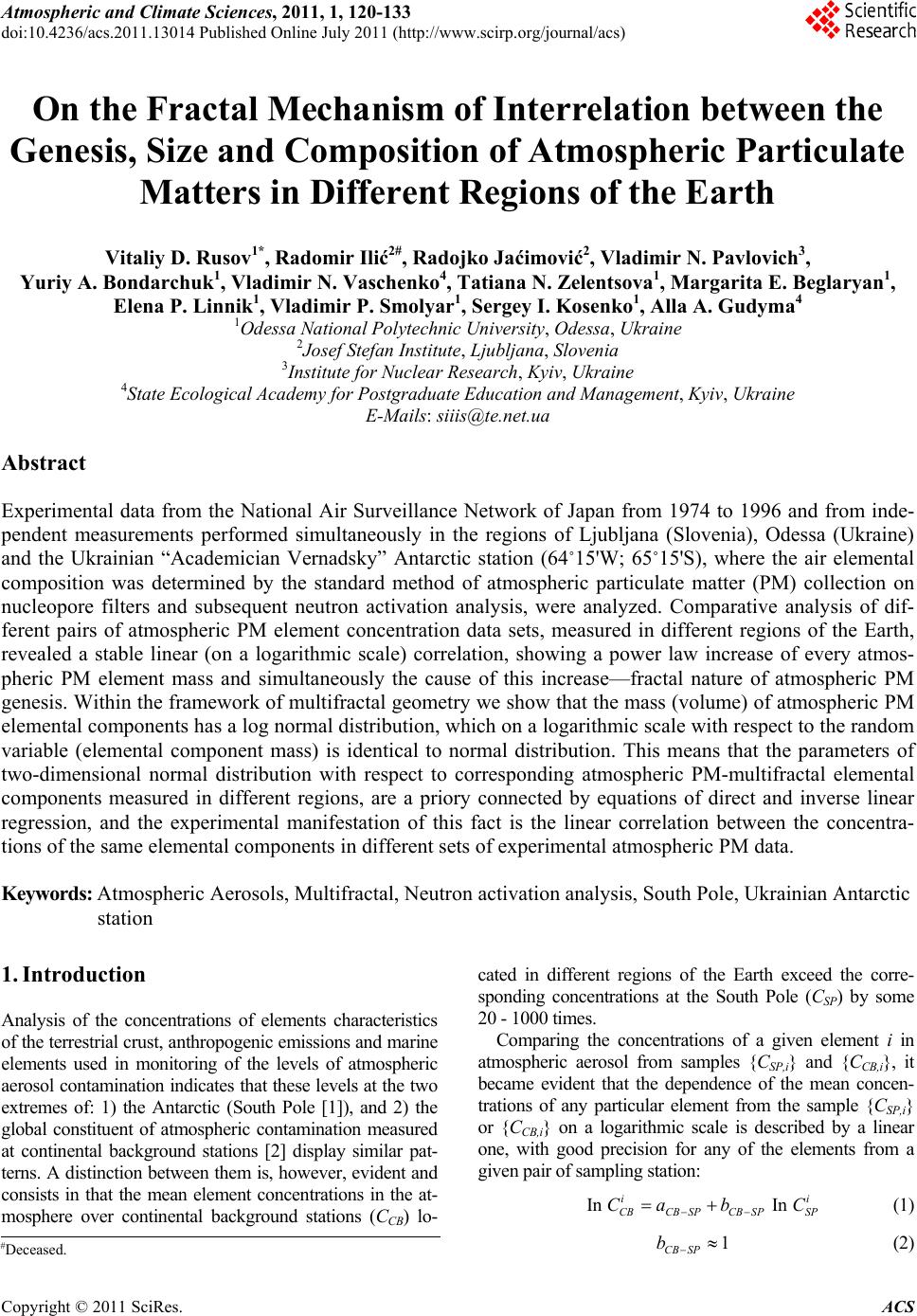 Atmospheric and Climate Sciences, 2011, 1, 120-133 doi:10.4236/acs.2011.13014 Published Online July 2011 (http://www.scirp.org/journal/acs) Copyright © 2011 SciRes. ACS On the Fractal Mechanism of Interrelation between the Genesis, Size and Composition of Atmospheric Particulate Matters in Different Regions of the Earth Vitaliy D. Rusov1*, Radomir Ilić2#, Radojko Jaćimović2, Vladimir N. Pavlovich3, Yuriy A. Bondarchuk1, Vladimir N. Vaschenko4, Tatiana N. Zelentsova1, Margarita E. Beglaryan1, Elena P. Linnik1, Vladimir P. Smolyar1, Sergey I. Kosenko1, Alla A. Gudyma4 1Odessa National Polytechnic University, Odessa, Ukraine 2Josef Stefan Institute, Ljubljana, Slovenia 3Institute for Nuclear Research, Kyiv, Ukraine 4State Ecological Academy for Postgraduate Education and Management, Kyiv, Ukraine E-Mails: siiis@te.net.ua Abstract Experimental data from the National Air Surveillance Network of Japan from 1974 to 1996 and from inde- pendent measurements performed simultaneously in the regions of Ljubljana (Slovenia), Odessa (Ukraine) and the Ukrainian “Academician Vernadsky” Antarctic station (64˚15'W; 65˚15'S), where the air elemental composition was determined by the standard method of atmospheric particulate matter (PM) collection on nucleopore filters and subsequent neutron activation analysis, were analyzed. Comparative analysis of dif- ferent pairs of atmospheric PM element concentration data sets, measured in different regions of the Earth, revealed a stable linear (on a logarithmic scale) correlation, showing a power law increase of every atmos- pheric PM element mass and simultaneously the cause of this increase—fractal nature of atmospheric PM genesis. Within the framework of multifractal geometry we show that the mass (volume) of atmospheric PM elemental components has a log normal distribution, which on a logarithmic scale with respect to the random variable (elemental component mass) is identical to normal distribution. This means that the parameters of two-dimensional normal distribution with respect to corresponding atmospheric PM-multifractal elemental components measured in different regions, are a priory connected by equations of direct and inverse linear regression, and the experimental manifestation of this fact is the linear correlation between the concentra- tions of the same elemental components in different sets of experimental atmospheric PM data. Keywords: Atmospheric Aerosols, Multifractal, Neutron activation analysis, South Pole, Ukrainian Antarctic station 1. Introduction Analysis of the concentrations of elements characteristics of the terrestrial crust, anthropogenic emissions and marine elements used in monitoring of the levels of atmospheric aerosol contamination indicates that these levels at the two extremes of: 1) the Antarctic (South Pole [1]), and 2) the global constituent of atmospheric contamination measured at continental background stations [2] display similar pat- terns. A distinction between them is, however, evident and consists in that the mean element concentrations in the at- mosphere over continental background stations (СCB) lo- cated in different regions of the Earth exceed the corre- sponding concentrations at the South Pole (СSP) by some 20 - 1000 times. Comparing the concentrations of a given element i in atmospheric aerosol from samples {CSP,i} and {CCB,i}, it became evident that the dependence of the mean concen- trations of any particular element from the sample {CSP,i} or {CCB,i} on a logarithmic scale is described by a linear one, with good precision for any of the elements from a given pair of sampling station: In In ii CBCB SPCB SPSP Ca bC (1) 1 CB SP b (2) #Deceased.
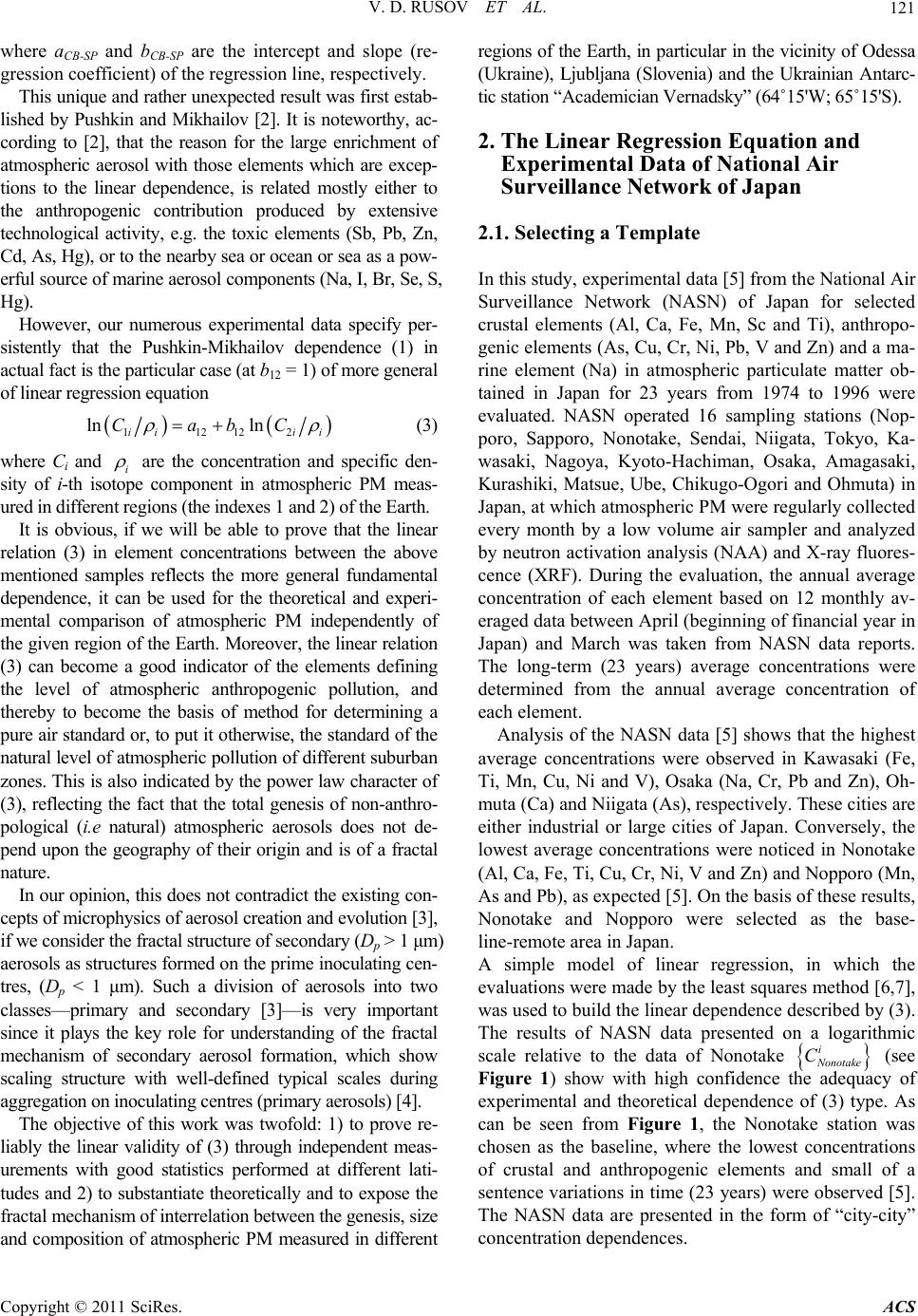 V. D. RUSOV ET AL.121 where аCB-SP and bCB-SP are the intercept and slope (re- gression coefficient) of the regression line, respectively. This unique and rather unexpected result was first estab- lished by Pushkin and Mikhailov [2]. It is noteworthy, ac- cording to [2], that the reason for the large enrichment of atmospheric aerosol with those elements which are excep- tions to the linear dependence, is related mostly either to the anthropogenic contribution produced by extensive technological activity, e.g. the toxic elements (Sb, Pb, Zn, Cd, As, Hg), or to the nearby sea or ocean or sea as a pow- erful source of marine aerosol components (Na, I, Br, Se, S, Hg). However, our numerous experimental data specify per- sistently that the Pushkin-Mikhailov dependence (1) in actual fact is the particular case (at b12 = 1) of more general of linear regression equation 112122 ln ln ii ii CabC (3) where Сi and i are the concentration and specific den- sity of i-th isotope component in atmospheric PM meas- ured in different regions (the indexes 1 and 2) of the Earth. It is obvious, if we will be able to prove that the linear relation (3) in element concentrations between the above mentioned samples reflects the more general fundamental dependence, it can be used for the theoretical and experi- mental comparison of atmospheric PM independently of the given region of the Earth. Moreover, the linear relation (3) can become a good indicator of the elements defining the level of atmospheric anthropogenic pollution, and thereby to become the basis of method for determining a pure air standard or, to put it otherwise, the standard of the natural level of atmospheric pollution of different suburban zones. This is also indicated by the power law character of (3), reflecting the fact that the total genesis of non-anthro- pological (i.e natural) atmospheric aerosols does not de- pend upon the geography of their origin and is of a fractal nature. In our opinion, this does not contradict the existing con- cepts of microphysics of aerosol creation and evolution [3], if we consider the fractal structure of secondary (Dp > 1 μm) aerosols as structures formed on the prime inoculating cen- tres, (Dp < 1 μm). Such a division of aerosols into two classes—primary and secondary [3]—is very important since it plays the key role for understanding of the fractal mechanism of secondary aerosol formation, which show scaling structure with well-defined typical scales during aggregation on inoculating centres (primary aerosols) [4]. The objective of this work was twofold: 1) to prove re- liably the linear validity of (3) through independent meas- urements with good statistics performed at different lati- tudes and 2) to substantiate theoretically and to expose the fractal mechanism of interrelation between the genesis, size and composition of atmospheric PM measured in different regions of the Earth, in particular in the vicinity of Odessa (Ukraine), Ljubljana (Slovenia) and the Ukrainian Antarc- tic station “Academician Vernadsky” (64˚15'W; 65˚15'S). 2. The Linear Regression Equation and Experimental Data of National Air Surveillance Network of Japan 2.1. Selecting a Template In this study, experimental data [5] from the National Air Surveillance Network (NASN) of Japan for selected crustal elements (Al, Ca, Fe, Mn, Sc and Ti), anthropo- genic elements (As, Cu, Cr, Ni, Pb, V and Zn) and a ma- rine element (Na) in atmospheric particulate matter ob- tained in Japan for 23 years from 1974 to 1996 were evaluated. NASN operated 16 sampling stations (Nop- poro, Sapporo, Nonotake, Sendai, Niigata, Tokyo, Ka- wasaki, Nagoya, Kyoto-Hachiman, Osaka, Amagasaki, Kurashiki, Matsue, Ube, Chikugo-Ogori and Ohmuta) in Japan, at which atmospheric PM were regularly collected every month by a low volume air sampler and analyzed by neutron activation analysis (NAA) and X-ray fluores- cence (XRF). During the evaluation, the annual average concentration of each element based on 12 monthly av- eraged data between April (beginning of financial year in Japan) and March was taken from NASN data reports. The long-term (23 years) average concentrations were determined from the annual average concentration of each element. Analysis of the NASN data [5] shows that the highest average concentrations were observed in Kawasaki (Fe, Ti, Mn, Cu, Ni and V), Osaka (Na, Cr, Pb and Zn), Oh- muta (Ca) and Niigata (As), respectively. These cities are either industrial or large cities of Japan. Conversely, the lowest average concentrations were noticed in Nonotake (Al, Ca, Fe, Ti, Cu, Cr, Ni, V and Zn) and Nopporo (Mn, As and Pb), as expected [5]. On the basis of these results, Nonotake and Nopporo were selected as the base- line-remote area in Japan. A simple model of linear regression, in which the evaluations were made by the least squares method [6,7], was used to build the linear dependence described by (3). The results of NASN data presented on a logarithmic scale relative to the data of Nonotake i onotake C (see Figure 1) show with high confidence the adequacy of experimental and theoretical dependence of (3) type. As can be seen from Figure 1, the Nonotake station was chosen as the baseline, where the lowest concentrations of crustal and anthropogenic elements and small of a sentence variations in time (23 years) were observed [5]. The NASN data are presented in the form of “city-city” concentration dependences. Copyright © 2011 SciRes. ACS
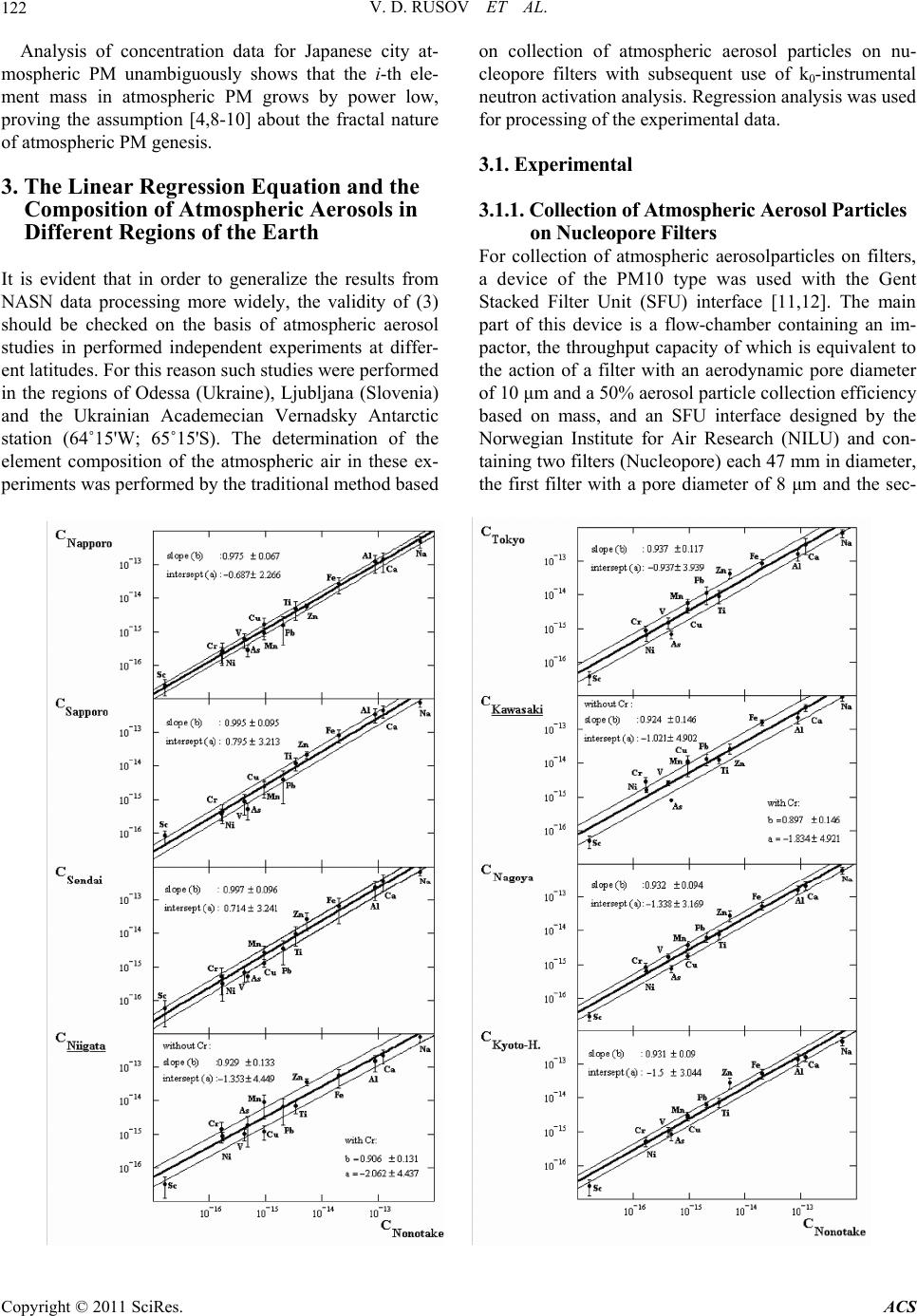 V. D. RUSOV ET AL. Copyright © 2011 SciRes. ACS 122 Analysis of concentration data for Japanese city at- mospheric PM unambiguously shows that the i-th ele- ment mass in atmospheric PM grows by power low, proving the assumption [4,8-10] about the fractal nature of atmospheric PM genesis. 3. The Linear Regression Equation and the Composition of Atmospheric Aerosols in Different Regions of the Earth It is evident that in order to generalize the results from NASN data processing more widely, the validity of (3) should be checked on the basis of atmospheric aerosol studies in performed independent experiments at differ- ent latitudes. For this reason such studies were performed in the regions of Odessa (Ukraine), Ljubljana (Slovenia) and the Ukrainian Academecian Vernadsky Antarctic station (64˚15'W; 65˚15'S). The determination of the element composition of the atmospheric air in these ex- periments was performed by the traditional method based on collection of atmospheric aerosol particles on nu- cleopore filters with subsequent use of k0-instrumental neutron activation analysis. Regression analysis was used for processing of the experimental data. 3.1. Experimental 3.1.1. Collection of Atmospheric Aerosol Particles on Nucleopore Filters For collection of atmospheric aerosolparticles on filters, a device of the PM10 type was used with the Gent Stacked Filter Unit (SFU) interface [11,12]. The main part of this device is a flow-chamber containing an im- pactor, the throughput capacity of which is equivalent to the action of a filter with an aerodynamic pore diameter of 10 μm and a 50% aerosol particle collection efficiency based on mass, and an SFU interface designed by the Norwegian Institute for Air Research (NILU) and con- taining two filters (Nucleopore) each 47 mm in diameter, the first filter with a pore diameter of 8 μm and the sec-
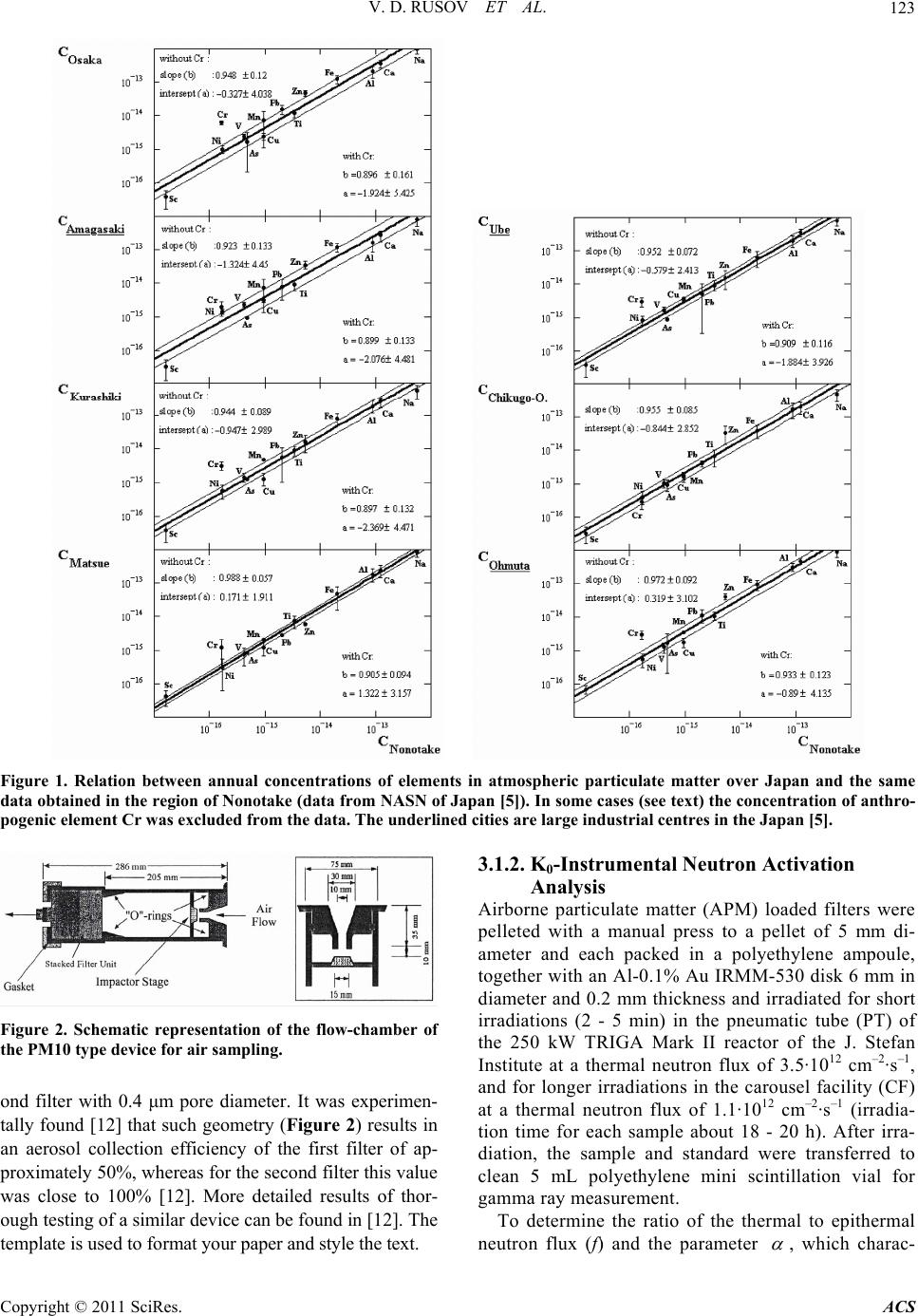 V. D. RUSOV ET AL.123 Figure 1. Relation between annual concentrations of elements in atmospheric particulate matter over Japan and the same data obtained in the region of Nonotake (data from NASN of Japan [5]). In some cases (see text) the concentration of anthro- pogenic element Cr was excluded from the data. The underlined cities are large industrial centres in the Japan [5]. Figure 2. Schematic representation of the flow-chamber of the PM10 type device for air sampling. ond filter with 0.4 μm pore diameter. It was experimen- tally found [12] that such geometry (Figure 2) results in an aerosol collection efficiency of the first filter of ap- proximately 50%, whereas for the second filter this value was close to 100% [12]. More detailed results of thor- ough testing of a similar device can be found in [12]. The template is used to format your paper and style the text. 3.1.2. K0-Instrumental Neutron Activation Analysis Airborne particulate matter (APM) loaded filters were pelleted with a manual press to a pellet of 5 mm di- ameter and each packed in a polyethylene ampoule, together with an Al-0.1% Au IRMM-530 disk 6 mm in diameter and 0.2 mm thickness and irradiated for short irradiations (2 - 5 min) in the pneumatic tube (PT) of the 250 kW TRIGA Mark II reactor of the J. Stefan Institute at a thermal neutron flux of 3.5·1012 cm–2·s–1, and for longer irradiations in the carousel facility (CF) at a thermal neutron flux of 1.1·1012 cm–2·s–1 (irradia- tion time for each sample about 18 - 20 h). After irra- diation, the sample and standard were transferred to clean 5 mL polyethylene mini scintillation vial for gamma ray measurement. To determine the ratio of the thermal to epithermal neutron flux (f) and the parameter , which charac- Copyright © 2011 SciRes. ACS
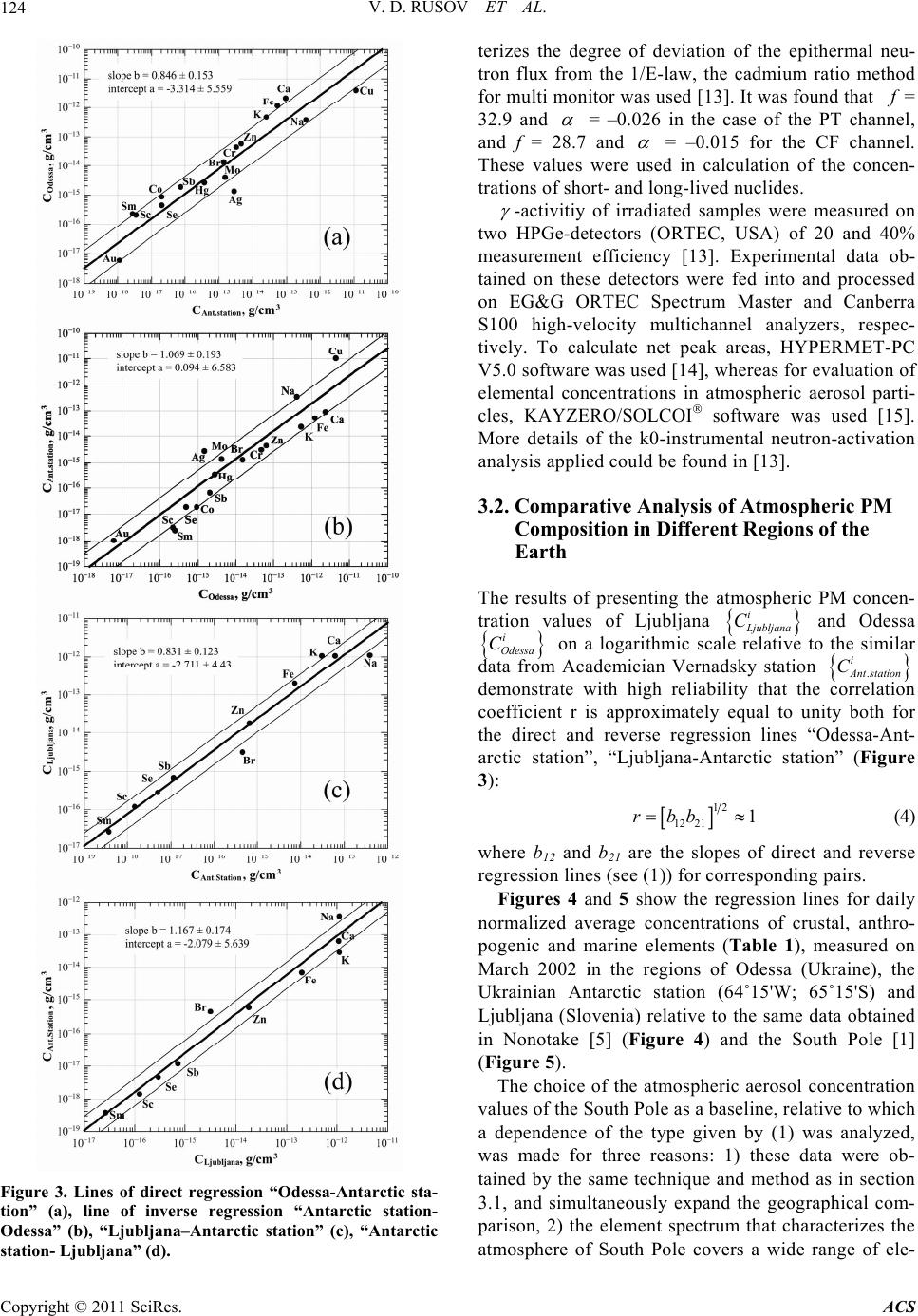 V. D. RUSOV ET AL. 124 Figure 3. Lines of direct regression “Odessa-Antarctic sta- tion” (a), line of inverse regression “Antarctic station- Odessa” (b), “Ljubljana–Antarctic station” (c), “Antarctic station- Ljubljana” (d). terizes the degree of deviation of the epithermal neu- tron flux from the 1/E-law, the cadmium ratio method for multi monitor was used [13]. It was found that = 32.9 and = –0.026 in the case of the РТ channel, and f = 28.7 and = –0.015 for the CF channel. These values were used in calculation of the concen- trations of short- and long-lived nuclides. -activitiy of irradiated samples were measured on two HPGe-detectors (ORTEC, USA) of 20 and 40% measurement efficiency [13]. Experimental data ob- tained on these detectors were fed into and processed on EG&G ORTEC Spectrum Master and Canberra S100 high-velocity multichannel analyzers, respec- tively. To calculate net peak areas, HYPERMET-PC V5.0 software was used [14], whereas for evaluation of elemental concentrations in atmospheric aerosol parti- cles, KAYZERO/SOLCOI software was used [15]. More details of the k0-instrumental neutron-activation analysis applied could be found in [13]. 3.2. Comparative Analysis of Atmospheric PM Composition in Different Regions of the Earth The results of presenting the atmospheric PM concen- tration values of Ljubljana i jubljana C and Odessa i Odessa C on a logarithmic scale relative to the similar data from Academician Vernadsky station . i Ant station C demonstrate with high reliability that the correlation coefficient r is approximately equal to unity both for the direct and reverse regression lines “Odessa-Ant- arctic station”, “Ljubljana-Antarctic station” (Figure 3): 12 12 211rbb (4) where b12 and b21 are the slopes of direct and reverse regression lines (see (1)) for corresponding pairs. Figures 4 and 5 show the regression lines for daily normalized average concentrations of crustal, anthro- pogenic and marine elements (Table 1), measured on March 2002 in the regions of Odessa (Ukraine), the Ukrainian Antarctic station (64˚15'W; 65˚15'S) and Ljubljana (Slovenia) relative to the same data obtained in Nonotake [5] (Figure 4) and the South Pole [1] (Figure 5). The choice of the atmospheric aerosol concentration values of the South Pole as a baseline, relative to which a dependence of the type given by (1) was analyzed, was made for three reasons: 1) these data were ob- tained by the same technique and method as in section 3.1, and simultaneously expand the geographical com- parison, 2) the element spectrum that characterizes the atmosphere of South Pole covers a wide range of ele- Copyright © 2011 SciRes. ACS
 V. D. RUSOV ET AL.125 Figure 4. Relationship between atmospheric PM elemental concentrations measured in theregions Odessa (Ukraine), Ljubljana (Slovenia), Vernadsky station (64˚15'W; 65˚15'S), SouthPole [1] and the same data measured in the region of Nonotake [5]. ments (see Table 1); and 3) the South Pole has the purest atmosphere on Earth, making it a convenient basis for comparative analysis. We present also the monthly normalized average concentrations of atmospheric PM measured over the period 2006-2007 in the region of the Ukrainian Ant- arctic station “Academician Vernadsky” (Figures 6, 7). Comparative analysis of experimental sets of nor- malized concentrations of atmospheric aerosol ele- ments measured in our experiments and independent experiments of the Japanese National Air Surveillance Network (NASN) shows a stable linear (on a logarith mic scale) dependence on different time scales (from average daily to annual). That points to a power law increase of every atmospheric PM element mass (vol- Figure 5. Relationship between atmospheric PM elemental concentrations measured in the regions Odessa (Ukraine), Ljubljana (Slovenia), Vernadsky station (64˚15'W; 65˚15'S), Nonotake [5] and the same data measured in the region of the South Pole [1]. Copyright © 2011 SciRes. ACS
 V. D. RUSOV ET AL. 126 Table 1. Chemical element composition in atmospheric PM. Symbols CSP, CCB, CNonotake denote concentrations at the South Pole, continental background stations and Nono- take-city, respectively. Measured concentrations in the vi- cinity of the Ukrainian Antarctic Station, Odessa and Ljubljana are denoted by CAntarctica, COdessa and CLjubljana. СSP СCB CNono- take САntarctica СОdessa CLjubljana Element (ng/m3) S 49 - - - - - Si - - - - - - Cl 2.4 90 - - - 528.2 Al 0.82 1.2 × 103 237.4- - 1152 Ca 0.49 - 185.893 2230 1630 Fe 0.62 1 × 102 157.653.4 1201 1532 Mg 0.72 - - - - 1264 K 0.68 - - 24.4 502.8 918 Na 3.3 1.4 × 102 538.8361.95 393.8 1046 Pb - 10 23.2- - - Zn 3.3 × 10–2 10 38.34.48 62.6 127.2 Ti 0.1 - 15.4- - - F - - - - - - Br 2.6 4 - 1.34 14.81 9.62 Cu 3 × 10–2 3 8.2011050 4240 - Mn 1.2 × 10–2 3 6.61- - 34.7 Ni - 1 1.48- - - Ba 1.6 × 10–2 - - - - 6.91 V 1.3 × 10–3 1 2.44- - 17.08 I 0.74 - - - - 36.24 Cr 4 × 10–2 0.8 1.143.11 45.2 - Sr 5.2 × 10–2 - - - - - As 3.1 × 10–2 1 2.74- 1.83 4.18 Rb 2 × 10–3 - - - - 0.92 Sb 8 × 10–4 0.5 - 0.07 1.97 4.62 Cd <1.5 × 10–2 0.4 - - - - Mo - - - 1.46 4.08 - Se <0.8 0.3 - 0.02 0.47 1.21 Ce 4 × 10–3 - - - 4.22 2.72 Hg 0.17 0.3 - 0.36 2.75 - W 1.5 × 10–3 - - 0.24 - - La 4.5 × 10–4 - - - 1.79 1.36 Ga <1 × 10–3 - - - - - Co 5 × 10–4 0.1 - 0.02 0.89 0.29 Ag <4 × 10–4 - - 2.74 1.42 - Cs 1 × 10–4 - - - 0.09 - Sc 1.6 × 10–4 5 × 10–2 0.043.36 × 10–3 0.21 0.298 Th 1.4 × 10–4 - - - 0.29 0.060 U - - - - 0.07 - Sm 9 × 10–5 - - 2.68 × 10–3 0.24 0.198 In 5 × 10–5 - - - - - Ta 7 × 10–5 - - - - - Hf 6 × 10–5 - - - - - Yb <0.05 - - - - - Eu 2 × 10–5 - - - - - Au 4 × 10–5 - - 1.07 × 10–3 0.006 - Lu 6.7 × 10–6 - - - - - ume) and simultaneously to the cause of this increase – the fractal nature of atmospheric PM genesis. In other words, stable fulfillment of the equality (4) not only for the experimental data shown in Figures 3- 7, but also for any pairs of the NASN data [5] (see Figure 2) unambiguously indicates that, on the one hand, the model of linear regression is satisfactory and, on the other hand, any of the analyzed samples mi Figure 6. The regression lines for the normalized monthly average concentrations of atmospheric PM measured in the region of the Ukrainian Antarctic station “Academician Vernadsky” measured in August - December 2006 with respect to October 2006. Copyright © 2011 SciRes. ACS
 V. D. RUSOV ET AL.127 Figure 7. The regression lines for the normalized monthly average concentrations of atmospheric PM measured in the region of the Ukrainian Antarctic station “Academician Vernadsky” in January - March 2007 with respect to Octo- ber 2006. which describe the sequence of i-th element partial concentrations in an aerosol, must to obey the Gauss distribution with respect to the random quantity ln pi. Proof of these assertions for multifractal objects is presented below. 4. The Spectrum of Multifractal Dimensions and Log Normal Mass Distribution of Secondary Aerosol Elements A detailed analysis of Figures 1, 3-5, where the linear regressions for different pairs of experimental samples of element concentrations in atmospheric PM measured at various latitudes are shown, allows us to draw a definite conclusion about the multifractal nature of PM. The basis of such a conclusion is the reliably observed linear de- pendence of (3) type between the normalized concentra- tions Сi of the same element i in atmospheric PM in dif- ferent regions of the Earth. Thus, it is necessary to consider the atmospheric PM, which is the multicomponent (with respect to elements) system, as a nonhomogeneous fractal object, i.e., as a multifractal. At the same time, the spectrum of fractal dimensions f and not a single dimension 0 (which is equal to D0 for a homogeneous fractal) is nec- essary for complete description of a nonhomogeneous fractal object. We will show below that the spectrum of fractal dimensions f of multifractal predetermines the log normal type of statistics or, in other words, the log normal type of mass distribution of multifractal i-th component. This is very important, because the represen- tation of mass distribution of atmospheric PM as the log normal distribution is predicted within the framework of the self-preserving theory [20,21] and is confirmed by numerous experiments at the same time [3]. To explain the main idea of derivation we give the ba- sic notions and definitions of the theory of multifractals. Let us consider a fractal object which occupies some bounded region £ of size L in Euclidian space of dimen- sion d. At some stage of its construction let it represents the set of N >> 1 points distributed somehow in this re- gion. We divide this region £ into cubic cells of side << L and volume d . We will take into considera- tion only the occupied cells, where at least one point is. Let i be the number of occupied cell i = 1, 2,···, N , where N is the total number of occupied cells, which depends on the cell size . Then in the case of a regular (homogeneous) fractal, according to the definition of fractal dimensions D, the total number of occupied cells N at quite small ε looks like D L NL . (5) where is the cell size in L units, L is the size of fractal object in ε units. When a fractal is nonhomogeneous a situation becomes more complex, because, as was noted above, a multifrac- tal is characterized by the spectrum of fractal dimensions f , i.e. by the set of probabilities pi, which show the fractional population of cells by which an initial set is covered. The less the cell size is, the less its population. For self-similarity sets the dependence pi on the cell size is the power function 1i iL i pL N i (6) Copyright © 2011 SciRes. ACS
 V. D. RUSOV ET AL. 128 where i is a certain exponent which, generally speak- ing, is different for the different cells i. It is obvious, that for a regular (homogeneous) fractal all the indexes i in (6) are identical and equal to the fractal dimension D. We now pass on to probability distribution of the dif- ferent values i Let nd is the probability what αi is in the interval , +d . In other words, nd is the relative number of the cells i, which have the same measure pi as i in the interval , +d . According to (5), this number is propor- tional to the total number of cells N for a monofractal, since all of i are identical and equal to the fractal dimension D. However, this is not true for a multifractal. The differ- ent values of i occur with probability characterized by the different (depending on ) values of the exponent f , and this probability inherently corresponds to a spectrum of fractal dimensions of the homogeneous sub- sets £ of initial set £: f L n . (7) Thus, from here a term “multifractal” becomes clear. It can be understood as a hierarchical joining of the differ- ent but homogeneous fractal subsets £ of initial set £, and each of these subsets has the own value of fractal dimension f . Now we show how the function f predetermines the log normal kind of mass (volume) distribution of the multifractal i-th component. To ease further description we represent expression (8) in the following equivalent form: exp lnnfL . (8) It is not hard to show [19] that the single-mode func- tion f can be approximated by a parabola near its maximum at the value 0 . 2 0 fD 0 (9) where the curvature of parabola 0 00 0 () 1 222() q f DD (10) is determined by the second derivative of function f at a point 0 . Due to a convexity of the function f it is obvious, that the magnitude in square brackets must be always positive. The fact, that the last summand 0q D in these brackets is numerically small and it can be ne- glected, will be grounded below. At the large L the distribution n (8) with an allowance for (9) takes on the form 2 0 0 00 ln ()~exp ln4() L nDL D . (11) Then, taking into account (5), we obtain from (11) the distribution function of random variable pi 0 0 2 0 0 exp ~ln 1ln ln 4ln 1 Di i D Nnp pp pN (12) which with consideration of normalization takes on the final form 2 2 2 11 exp 2 2 1 expln 2 i i Pp p (13) where 0 2 0 0 ln 1 1n , =2 pp (14) This is the so-called log normal (relative) mass pi dis- tribution. It is possible to present the first moments of such a kind of distribution for random variable pi in the following form: 00 0 23 223 0 3 exp 1 2 Ds ppN L (15) 00 00 22 22 322 varexp2exp 4exp 3 1 DD p LL (16) At the same time, it is easy to show that the distribu- tion (13) for the random variable ln pi has the classical Gaussian form 2 2 2 1 1n 2 1 exp1n 1n 2 Pp pp (17) where the first moments of this distribution for the ran- dom variable ln pi look like 2 00 1n 21npD L (18) 2 00 var1n 21npaD L (19) Thus, according to the known theorem of multidimen- sional normal distribution shape [22], a normal law of plane distribution for the two-dimensional random vari- able (p1i, p2i) will be written down as 12 2 12 22 2 1 1n ,1n 21 1 exp 2 21 ii pp p r uv ruv r (20) Copyright © 2011 SciRes. ACS
 V. D. RUSOV ET AL.129 where 112 2 12 1n 1n 1n 1n , (21) iiii pp pp v = cov(ln p1i, ln p2i)/σ1 σ2 is the correlation coefficient between ln p1i and ln p2i. , by virtue of well-known linear correlation theo- rem [6,22] it is easy to show that ln p1i and ln p2i are con- nected by the linear correlation dependen two-dimensional random variable (ln p1i, ln p2i) is nor- m r Then ce, if the ally distributed. This means that the parameters of two-dimensional normal distribution of the random val- ues p1i and p2i for the i-th component in one aerosol parti- cle, which are measured in different regions of the Earth (the indexes 1 and 2), are connected by the equations of direct linear regression: 1 112 2 2 1n 1n 1n 1n i iii i i pprp p (21) and inverse linear regression 2 22 1n 1n i ii pp 11 1 1n 1n ii i r pp (22) where I = 1,···,Np is the component number. Taking into consideration that we measure experimen- i of the i-th component in the unit volume of atmosphere, the partial concentration mi of the i-th component in one aerosol particle in different regions of the Earth (the indexes 1 and 2) tally the total concentration С measured looks lik 111122 , iii i mCnmCn (23) where n1 and n2 are the number of inoculating centers, whose role play the primary aerosols (Dp < 1 μm). Here it is necessary to make important digression con- cerning the choice of quantitative measure for description of fractal structures. According to Feder [18] tion of appropriate probabilities correspo ure of pr , determina- nding to the chosen measure is the main difficulty. In other words, if choice of measure determines the search proced obabilities pi, which describe the increment of the chosen measure for given level of resolution , then the probabilities themselves predetermine, in its turn, the proper method of their measurement. So, general strategy of quantitative description of fractal objects, in general case, should contain the following direct or reverse pro- cedure: the choice of measure—the set of appropriate probabilities—the measuring method of these probabili- ties. We choose the reverse procedure. So long as in the present work the averaged masses of elemental compo- nents of atmospheric PM-multifractal are measured, the geometrical probabilities, which can be constructed by experimental data for some fixed , have the practically unambiguous form: // // iii i ii ii ii mi C p constmC (24) where ρi is the specific gravity of the i-th component of secondary aerosol. Since the random nature of atmospheric PM formation is a priori determined by the random process component diffusion-limited aggregation (DLA), the so-called harmonic measure [18] to describe quantita- volution of possible growth of cluster PM ster. Both these magnitudes, Np and N, ch of multi- we used tively a stochastic surface inhomogeneity or, more pre- cisely, to study an e diameter. In practice, a harmonic measure is estimated in the fol- lowing way. Because the perimeter of clusters, which form due to DLA, is proportional to their mass, the num- ber of knots Np on the perimeter, i.e., the number of pos- sible growing-points, is proportional to the number of cells N in a clu ange according to the power law (5) depending on the cluster diameter L. From here it follows that all the knots Np, which belong to the perimeter of such clusters, have a nonzero probability what a randomly wandering particle will turn out in them, i.e., they are the carriers of har- monic measure , dL Mq 1 , 0,( ) ,,() pd N q dL i i d LL L Mqp L dq Zq dq (26) where , qL al q is the generalized statistical sum in the interv , q is the index of mass, at which a mure does not become zeeas ro or infinity at L . at in 0 L obvious, thIt is such a form the harmonic measure is described by the full index sequence , which de- epending on. At t lated in the usual way, but using thian parti- cl q e “Brown termines according to what power law the probabilities pi change d Lhe same time, the spectrum of fractal dimensions for the harmonic measure is calcu- es-probes” of fixed diameter for study of possible growth of the cluster diameter L. From (26) iows that in this case the generalized statistical sum , t foll Zq can be represented in the form. 1 , ~ p N q q LiL i Zqp (25) As is known from numerical simulation of a harmonic measure, when the DLA cluster surface is y the large number of randomly wandering particles, theaks of “high” asperities in su probed b e p ch a fractal aggregate have Copyright © 2011 SciRes. ACS
 V. D. RUSOV ET AL. 130 greater possibilities than the peaks of “lo if possible growing-points on the perime m w” asperities. So, ter of our aerosol ultifractal to renumber by the index 1, , iN the set of probabilities 1 ii p (26) composed of the probabilities of (25) type will emulate the possible set of interaction cross-sections between Brownian particle and atmospheric PM-m sur- face, which consist ultifractal s of the Np groups of identical atoms distributed on the surface. Each of these groups charac- terizes the i-th elemental component in the one atmos- pheric PM. A situation is intensified by the fact that by virtue of (17) each of the independent components obeys the Gauss distribution, as is known [22], belong to the class of infi- nitely divisible distributions or, more specifically, to the class of so-called α-stable distributions. This means that although the Gauss distribution has different parameters (the average 1n ii p and variance 2 i = var(ln pi) for each of components, the final distribution is the Gauss distribution too, but with the parameters ln p i и 22 i . From here it follows that the pa- rameters of the two-dimensional normal distribution of all corresponding components in the plane p1, p2 are con- nected by the equations of direct linear regression: 1 11 2 2 1n 1n 1n 1n pprp p (27) and inverse linear regression: 2 2 22 11 1 1n 1n 1n 1n ppr pp (28) So, validity of the assumption (28) for the s aerosol or, that is the same, validity of the application of uantitative description of mul- taneous consideration of (28) and equation of dir regression (29) will result in an equation identical to the eq econdary harmonic measure for the q aerosol stochastic fractal surface, will be proven if si ect linear uation of direct linear regression (3). Therefore, taking into account (28) we write down, for example, the equation of direct linear regression or, in other words, the condition of linear correlation between the samples of i-th component concentrations 1 ln/ i i C and 2 ln/ i i C in an atmospheric aerosol measured in different places (indexes 1 and 2): 12 112122 1 1 12 2 2 1 lnln , 1ln , p ii ii N ii i b p ii i CabC C br N C 1 12 p N a (31) It is obvious, that this equation completely coincides with the equation of linear regression (3), but is theoreti- cally obtained on basis of the Gauss distribution of the random magnitude ln pi and not in an empirical wa Physical interpretation of the intersept a12 is evident from the expression (29), whereas meaning of the regres- sion coefficient b12 becomes clear, according to (19) and (2 y. 9), from the following expression: 12 12 111 12 222 var(ln )ln() var(ln )ln() pL br r pL (29) where L1 and L2 are the average sizes of separate atmos- pheric PM-multifractals typical for the atmosphere of investigated regions (indexes 1 and 2) of the Earth, 1 and 2 are the cell sizes into which the correspond atmc PM-multifractals are divided. Below we give a computational procedure for identification of the generalized fractal dimension Dq ing ospheri algorithm spectra and function f . It is obvious, that such a problem can be solved by the following redundant system of nonlinear equations of (15), (16), (18) and (19) type: 00 00 ) var( )1pL L 00 2(23)2(2 (23) ln DD pDL ln . (30) 2 00 2 00 ln2) ln var(ln)2() ln pDL pDL ( , (31) where is the cubic cell fixed size, into which the bounded region £ of size L in Euclidian space of dimen- sion d is divided. To solve the system of Equations (33) and (34) with respect to the variables 0, D0 and , lly the it is necessary and sufficient to measure experimi nents of the concentration sample Сi in the unit volume s l n enta -th compo- of atmopheric air (see section 3) and size distribution of atmospheric PM for determination of the average size L. It will be recaled that from the physical standpoint so-called the box counting dimension D0, the entropy dimension D1 and the correlation dimesion D2 are the most interesting in the spectrum of the generalized fractal dimensions Dq corresponding to different multifractal inhomogeneities. Within the framework of the notions and definitions of multifractal theory mentioned above we decribe below the simple procedure for finding of spec- trum of the generalized fractal dimensions Dq taking into account the solution of the system of Equations (33) and (34). From (27) it follows that in our case a multifractal is characterized by the nonlinear function q of mo- ments q 0 ln(, ) () limln L L Zq q . (32) Copyright © 2011 SciRes. ACS
 V. D. RUSOV ET AL.131 ies some bounded region £ of “running” size L (so th As well as before we consider a fractal object, wich occup at 0 L ) in Euclidian space of dimension d. Then spectrum of the generalized fractal dimensDq char- acterizing ions the multifractal statistical inhomogeneity (the distribution of points in the region £) is det relation ermined by the () 1 q q Dq , (33) where (q – 1) is the numerical factor, which normalizes the function q so that the equality Dq = d is fulfilled for a set of constant density in the d-dimensional Euclid- ian space. Further, we are interested in the known in theory of multifractal connection between the mass index q and the multifractal function f by which the spec- trum of generalized fractal dimD is determ ensions qined ) 1()(()) 11 q Dq aqfaq qq . (34) It is obvious, t (q hat in our case, when the sample pi is experimentally determined and the cell size L is numerically evaluated (by the system of Equations (33) and (34)), the spectrum of generalized fractal dimensions Dq (36) can be obtained by the expression for the mass index (35): q 1 ln () 1( 1)ln i i q p N q p q Dqq . (35) Finally, joint using of the Legendre transformation d dq , (36) () d fq dq , (37) which sets direct algorithm for transition from the vari- ables , qq to the variables , f , and oximate analytical expression (38) for the funct the apprion Dq mble to determi multion akes it possi fractal functi ne an expression for the f . ider thNoonse sp of the box counting dimension D0 and the One of goals of this consideration is the validation of as- 10), whe de sformn sets, which sets transi- tio w we will cecial case of search entropy dimension D1. sumption of smallness of the magnitude in the ex- pression (hich was used for tion of log normal distribution of the random magnitude pi (13). It is easy to show that combined using of (9) and the inverse Legendre tranatio 0q D rivat n from the variables , f to the variables , qq , gives the following dependence of q on the moments q: 00 0 () 2()qqD . (38) Substituting (41) and (9) into (37), we obtain the ap- proximate expression for the spectrum of generalized fractal dimensions Dq (q = 0.1) depending on 0 and D0: 2 1() q DqDqD q 00 00 1 (39) Thus, we cam write down the expressi co . ons for the box unting dimension D0 and the entropy dimension D1 depending on 0 and D0 DD, (40) 00 10 1 () (()) lim 2 1 q qaq faq DD q0 . (41) Here it is necessary to make a few remarks. It will be recalled that 1q f = D 1 is the value of fractal di- mension of that subset of the region £, which makes a most contribue stattion to th virtue of is equal t l size istical su However, bynormalizing cal sum (36)o unity at q = 1 and does not de- pend on the cel m (36) at q = 1. condition the statisti- , on which the region £ i Thus, this moion also is of order unity. There- fo s divided. st contribut ation p re, in this case (and only in this case!) the probabilities of cell occupiL (6) are inversely propor- tional to the total number of cells () f L n , i.e., the condition f is fulfilled. So, the parameters of system of the Equations (33)and (34) obtained by the expression (9) can not in essence contain information aut the generalized fractal dimen- sions Dq for absolute value of the moments q greater than unity (i.e., q 1). Secondly, it is easy that the expression (39) for the entropy dimension D1 does no concrete type of th bo to show t depend on e function f , but is determined by its properties, for example, by symmetry f , 1f and convexity 0f . The geometrical method for determination of the entropy dimension D1 shown in Figure 8 is simultaneously the geometrical proof of assertion (44). Thirdly, the expressions for the entropy dimension D1 obtained by parabolic approximation of the function f and geometricalod (Figure 8) are equivalent. This means that the magnitude 0 D in the expression al to zero. Thus, ption of smallness of the magnitude 0q D meth u our q assum(10) is eq in the expression (10) is mathe- matically valid. In the end, we note that the knowledge of generalized fractal dimensions Dq, the correlation dimension D2 and 100 2DD Copyright © 2011 SciRes. ACS
 V. D. RUSOV ET AL. 132 Figure 8. Geometrical method for determination of the en- tropy dimension D1, which leads to the obvious equality. especially D1, which describes an information loss rate during multifractal dynamic evolution, plays the key role for an understanding of the mechanism of secondary aerosol formation, since makes it possible to simulate a scaling structure of an atmospheric PM with well-defined ay that the magnitude D gives an information necessary for asured i cales (from average daily ints to a power law increase of every ment mass (volume) and simultane- usly to the cause of this increase - the fractal nature of -multifractal elemental co fractal structure is realized, and how their fractal di typical scales. Returning to the initial problem of distribu- tion of points over the fractal set £, it is possible to s 1 determination of point location in some cell, while the correlation dimension D2 determines the probability what a distance between the two randomly chosen points is less than L. In other words, when the relative cell size tends to zero 0 L , these magnitudes are anticorrelated, i.e. the entropy D1 decreases, while the multifractal cor- relation function D2 increases. 5. Conclusions Comparative analysis of different pairs of experimental normalized concentration values of atmospheric PM ele- ments men different regions of the Earth shows a stable linear (on a logarithmic scale) correlation (r = 1) dependence on different time s to annual). That po atmospheric PM ele o the genesis of atmospheric PM. Within the framework of multifractal geometry it is shown that the mass (volume) distribution of the atmos- pheric PM elemental components is a log normal distri- bution, which on the logarithmic scale with respect to the random variable (elemental component mass) is identical to the normal distribution. This means that the parameters of the two-dimensional normal distribution with respect to corresponding atmospheric PM mponents, which are measured in different regions, are a priory connected by equations of direct and inverse lin- ear regression, and the experimental manifestation of this fact is the linear (on a logarithmic scale) correlation be- tween the concentrations of the same elemental compo- nents in different sets of experimental atmospheric PM data. We would like to note here that a degree of our under- standing of the mechanism of atmospheric PM formation, which due to aggregation on inoculating centres (primary aerosols (Dp 1m)) show a scaling structure with well-defined typical scales, can be described by the known phrase: “…we do not know till now why clusters become fractals, however we begin to understand how their mension is related to the physical process” [23]. This made it possible to show that the spectrum of fractal di- mensions of multifractal, which is a multicomponent (by elements) aerosol, always predetermines the log normal type of statistics or, in other words, the log normal type of mass (volume) distribution of the i-th component of at- mospheric PM. It is theoretically shown, how solving the system of nonlinear equations composed of the first moments (the average and variance) of a log normal and normal distri- butions, it is possible to determine the multifractal func- tion f and spectrum of fractal dimensions Dq for separate averaged atmospheric PM, which are the global characteristics of genesis of atmospheric PM and does not depend on the local place of registration (measurement). in We should note here that the results of this work allow an approach to formulation of the very important problem of aerosol dynamics and its implications for global aero- sol climatology, which is connected with the global at- mospheric circulation and the life cycle of troposphere aerosols [3,21]. It is known that absorption by the Earth's solar short-wave radiation at the given point is not com- pensated by outgoing long-wave radiation, although the tegral heat balance is constant. This constant is sup- ported by transfer of excess tropical heat energy to high-latitude regions by the aid of natural oceanic and atmospheric transport, which provides the stable heat regime of the Earth. It is evident that using data about elemental and dispersed atmospheric PM composition in different regions of the Earth which are “broader-based” than today, one can create the map of latitude atmospheric PM mass and size distribution. This would allow an analysis of the interconnection between processes of ocean-atmosphere circulation and atmospheric PM gene- sis through the surprising ability of atmospheric PM for long range transfer, in spite of its short “lifetime” (about Copyright © 2011 SciRes. ACS
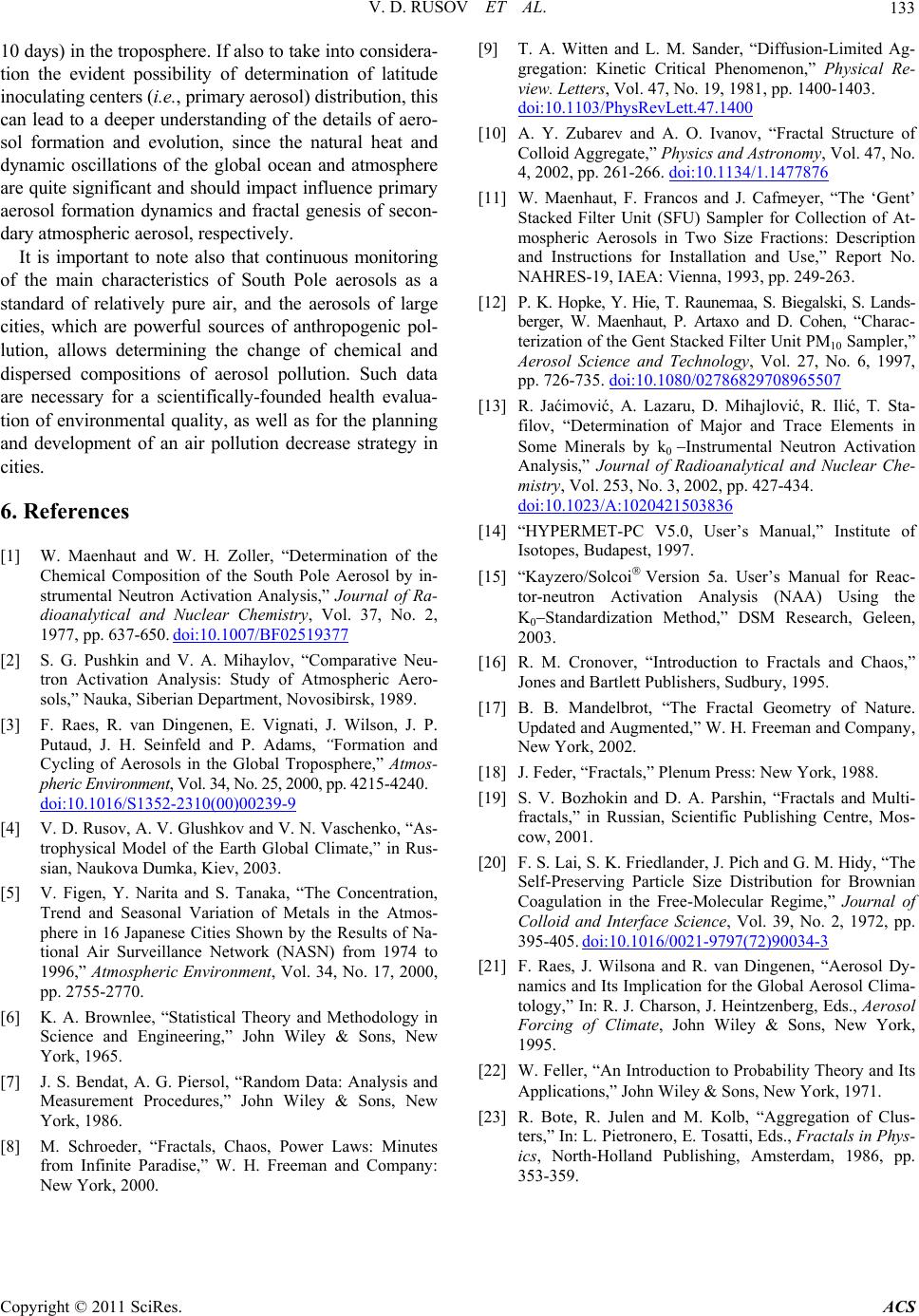 V. D. RUSOV ET AL. Copyright © 2011 SciRes. ACS 133 s for the plan an 10 days) in the troposphere. If also to take into considera- tion the evident possibility of determination of latitude inoculating centers (i.e., primary aerosol) distribution, this can lead to a deeper understanding of the details of aero- sol formation and evolution, since the natural heat and dynamic oscillations of the global ocean and atmosphere are quite significant and should impact influence primary aerosol formation dynamics and fractal genesis of secon- dary atmospheric aerosol, respectively. It is important to note also that continuous monitoring of the main characteristics of South Pole aerosols as a standard of relatively pure air, and the aerosols of large cities, which are powerful sources of anthropogenic pol- lution, allows determining the change of chemical and dispersed compositions of aerosol pollution. Such data are necessary for a scientifically-founded health evalua- tion of environmental quality, as well aning d development of an air pollution decrease strategy in cities. 6. References [1] W. Maenhaut and W. H. Zoller, “Determination of the Chemical Composition of the South Pole Aerosol by in- strumental Neutron Activation Analysis,” Journal of Ra- dioanalytical and Nuclear Chemistry, Vol. 37, No. 2, 1977, pp. 637-650. doi:10.1007/BF02519377 [2] S. G. Pushkin and V. A. Mihaylov, “Comparative Neu- n Analysis: Study of Atmospheric Aero- iberian Department, Novosibirsk, 1989. tron Activatio sols,” Nauka, S [3] F. Raes, R. van Dingenen, E. Vignati, J. Wilson, J. P. Putaud, J. H. Seinfeld and P. Adams, “Formation and Cycling of Aerosols in the Global Troposphere,” Atmos- pheric Environment, Vol. 34, No. 25, 2000, pp. 4215-4240. doi:10.1016/S1352-2310(00)00239-9 [4] V. D. Rusov, A. V. Glushkov and V. N. Vaschenko, “As- trophysical Model of the Earth Global Climate,” in Rus- sian, Naukova Dumka, Kiev, 2003. V. Figen, Y. Narita and S. Tanaka, “The Concentratio[5] n, Trend and Seasonal Variation of Metals in the Atmos- phere in 16 Japanese Cities Shown by the Results of Na- tional Air Surveillance Network (NASN) from 1974 to 1996,” Atmospheric Environment, Vol. 34, No. 17, 2000, pp. 2755-2770. [6] K. A. Brownlee, “Statistical Theory and Methodology in Science and Engineering,” John Wiley & Sons, New York, 1965. [7] J. S. Bendat, A. G. Piersol, “Random Data: Analysis and Measurement Procedures,” John Wiley & Sons, New York, 1986. [8] M. Schroeder, “Fractals, Chaos, Power Laws: Minutes from Infinite Paradise,” W. H. Freeman and Company: New York, 2000. [9] T. A. Witten and L. M. Sander, “Diffusion-Limited Ag- gregation: Kinetic Critical Phenomenon,” Physical Re- view. Letters, Vol. 47, No. 19, 1981, pp. 1400-1403. doi:10.1103/PhysRevLett.47.1400 [10] A. Y. Zubarev and A. O. Ivanov, “Fractal Structure of Colloid Aggregate,” Physics and Astronomy, Vol. 47, No. 4, 2002, pp. 261-266. doi:10.1134/1.1477876 [11] W. Maenhaut, F. Francos and J. Cafmeyer, “The ‘Gent’ Stacked Filter Unit (SFU) Sampler for Collection of mospheric Aerosols in Two Size F At- ractions: Description i, S. Lands- and Instructions for Installation and Use,” Report No. NAHRES-19, IAEA: Vienna, 1993, pp. 249-263. [12] P. K. Hopke, Y. Hie, T. Raunemaa, S. Biegalsk berger, W. Maenhaut, P. Artaxo and D. Cohen, “Charac- terization of the Gent Stacked Filter Unit PM10 Sampler,” Aerosol Science and Technology, Vol. 27, No. 6, 1997, pp. 726-735. doi:10.1080/02786829708965507 [13] R. Jaćimović, A. Lazaru, D. Mihajlović, R. Ilić, T. Sta- filov, “Determination of Major and Trace Elements in Some Minerals by k0 Instrumental Neutron Activation Analysis,” Journal of Radioanalytical and Nuclear Che- mistry, Vol. 253, No. 3, 2002, pp. 427-434. doi:10.1023/A:1020421503836 [14] “HYPERMET-PC V5.0, User’s Manual,” Institute of Isotopes, Budapest, 1997. [15] “Kayzero/ Solcoi Version 5a. User’s Manual for Reac- tor-neutron Activation Analysis (NAA) Using the K0Standardization Method,” DSM Resea 2003. rch, Geleen, rs, Sudbury, 1995. r, “Fractals,” Plenum Press: New York, 1988. ntre, Mos- rticle Size Distribution for Brownian l of [16] R. M. Cronover, “Introduction to Fractals and Chaos,” Jones and Bartlett Publishe [17] B. B. Mandelbrot, “The Fractal Geometry of Nature. Updated and Augmented,” W. H. Freeman and Company, New York, 2002. [18] J. Fede [19] S. V. Bozhokin and D. A. Parshin, “Fractals and Multi- fractals,” in Russian, Scientific Publishing Ce cow, 2001. [20] F. S. Lai, S. K. Friedlander, J. Pich and G. M. Hidy, “The Self-Preserving Pa Coagulation in the Free-Molecular Regime,” Journa Colloid and Interface Science, Vol. 39, No. 2, 1972, pp. 395-405. doi:10.1016/0021-9797(72)90034-3 [21] F. Raes, J. Wilsona and R. van Dingenen, “Aerosol Dy- ory and Its 9. namics and Its Implication for the Global Aerosol Clima- tology,” In: R. J. Charson, J. Heintzenberg, Eds., Aerosol Forcing of Climate, John Wiley & Sons, New York, 1995. [22] W. Feller, “An Introduction to Probability The Applications,” John Wiley Sons, New York, 1971. [23] R. Bote, R. Julen and M. Kolb, “Aggregation of Clus- ters,” In: L. Pietronero, E. Tosatti, Eds., Fractals in Phys- ics, North-Holland Publishing, Amsterdam, 1986, pp. 353-35
|