Open Journal of Statistics
Vol. 3 No. 1 (2013) , Article ID: 28068 , 5 pages DOI:10.4236/ojs.2013.31006
Strong Law of Large Numbers for a 2-Dimensional Array of Pairwise Negatively Dependent Random Variables
1Department of Mathematics and Computer Science, Chulalongkorn University, Bangkok, Thailand
2Centre of Excellence in Mathematics, CHE, Bangkok, Thailand
Email: nattakarn.c@chula.ac.th
Received October 30, 2012; revised November 30, 2012; accepted December 14, 2012
Keywords: Strong Law of Large Numbers; Negatively Dependent; 2-Dimensional Array of Random Variables
ABSTRACT
In this paper, we obtain the strong law of large numbers for a 2-dimensional array of pairwise negatively dependent random variables which are not required to be identically distributed. We found the sufficient conditions of strong law of large numbers for the difference of random variables which independent and identically distributed conditions are regarded. In this study, we consider the limit as which is stronger than the limit as
when m, n are natural numbers.
1. Introduction and Main Results
|
as |
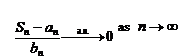
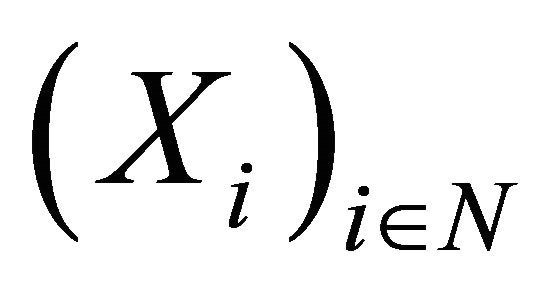
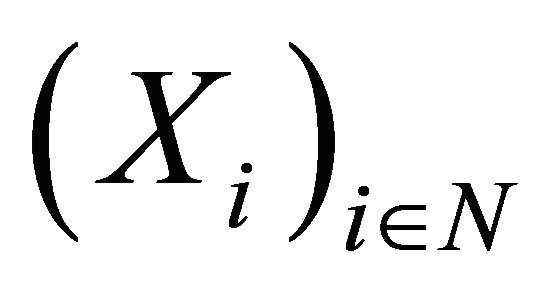
(SLLN) if there exist sequences of real numbers
and such that
as
. where
and the abbreviation a.s. stands for almost surely.
To study the strong law of large numbers, there is a simple question come in mind. When does the sequence satisfy the SLLN? Many conditions of the sequence
have been found for this question. The SLLN are investigated extensively in the literature especially to the case of a sequence of independent random variables (see for examples in [1-3]). After concepts of dependence was introduced, it is interesting to study the SLLN with condition of dependence.
A sequence of random variables is said to be pairwise positively dependent (pairwise PD) if for any
and
,
and it is said to be pairwise negatively dependent (pairwise ND) if for any and
,
Theorem 1.1-1.5 are examples of SLLN for a sequence of pairwise PD and pairwise ND random variables.
Theorem 1.1. (Birkel, [4]) Let be a sequence of pairwise PD random variables with finite variances. Assume 1)
2)
Then as
Theorem 1.2. (Azarnoosh, [5]) Let be a sequence of pairwise ND random variables with finite variances. Assume 1)
2)
Then as
Theorem 1.3. (Nili Sani, Azarnoosh and Bozorgnia, [6]) Let be a positive and increasing sequence such that
as
Let be a sequence of pairwise ND random variables with finite variances such that 1)
2)
Then as
In this work, we study the SLLN for a 2-dimensional array of pairwise ND random variables. We say that
satisfies the SLLN if there exist double sequences of real numbers
and
such that
as
where
In 1998, Kim, Beak and Seo investigated SLLN for a 2-dimensional array of pairwise PD random variables and it was generalized to a case of weighted sum of 2-dimensional array of pairwise PD random variables by Kim, Baek and Han in one year later. The followings are their results.
A double sequence is said to be pairwise positively dependent (pairwise PD) if for any
and
Theorem 1.4. (Kim, Beak and Seo, [7]) Let
be a 2-dimensional array of pairwise PD random variables with finite variances. Assume 1)
2)
Then as
Theorem 1.5. (Kim, Baek and Han, [8]) Let be a 2-dimensional array of positive numbers and
such that
and
as
Let be a 2-dimensional array of pairwise PD random variables with finite variances such that 1)
2)
Then as
where
Observe that, for a double indexed sequence of real number the convergence as
implies the convergence as
. However, a double sequence
where
shows us that the converse is not true in general.
Our goal is to obtain the SLLN for 2-dimensional array of random variables in case of pairwise ND.
A double sequence is said to be pairwise negtively dependent (pairwise ND) if for any
and
The followings are SLLNs for a 2-dimensional array of pairwise ND random variables which are all our results.
Theorem 1.6. Let and
be increasing sequences of positive numbers such that
which
as
and
as
Let be a 2-dimensional array of pairwise ND random variables with finite variances. If there exist real numbers
such that
then for any double sequence such that
for every
as
The next theorem is the SLLN for the difference of random variables which independent and identically distributed conditions are regarded.
Theorem 1.7. Let and
be 2dimensional arrays of random variables on a probability space (Ω, F, P). If
then
as
Corollary 1.8 and Corollary 1.9 follow directly from Theorem 1.6 by choosing and
where
and
with p = q = 4, respectively.
Corollary 1.8. Let and
be increasing sequences of positive numbers such that
which
as
and
as
Let be a 2-dimensional array of pairwise ND random variables with finite variances. If there exist
such that
then for any
as
Corollary 1.9. Let be a 2-dimensional array of pairwise ND random variables with finite variances. If
then
as
2. Auxiliary Results
In this section, we present some materials which will be used in obtaining the SLLN’s in the next section.
Proposition 2.1. (Móricz, [9]) Let be a double sequence of positive numbers such that for all
and as
Let be a double sequence of real numbers. Assume that 1)
2) for every
and
for every
Then
as
The following proposition is a Borel-Cantelli lemma for a sequence of double indexed events Proposition 2.2. Let be a double sequence of events on a probability space
Then
where
Proof. Let be such that
First note that
where denote the greatest integer smaller than or equal
and hence
Therefore and
This completes the proof. □
3. Proof of Main Results
Proof of Theorem 1.6
Let and define
and
Clearly, f and g are increasing whose facts
and
which imply that
and
.
Let be given. By using the fact that
for
([10], p. 313), we have
From this fact and Chebyshev’s inequality, we have
For each let
and and
. Since
and
, we have
and
. From this facts and (3.1), we have
Since and
, we have
and
.
From this facts and (3.2) together with our assumption 2), we have
By Proposition 2.2 with
we have and this hold for every
By using the same idea with Theorem 4.2.2 ([11], p. 77), we can prove that
as
Proof of Theorem 1.7
Let By Proposition 2.2, we have
For every we will show that
(3.3)
for every,
(3.4)
and for every
(3.5)
From (3.3), (3.4) and (3.5), we can apply Proposition 2.1 with that
as We here note that
as
implies
as
. Hence
as
To prove (3.3), (3.4) and (3.5), let Then there exists
such that for
(3.6)
Thus for each and
are different only finitely many terms. This implies that (3.3) holds.
For fixed we can find a large
such that (3.6) holds for all
which means that there are only finitely many different terms of
and
So for fixed
.
Similarly, for fixed
Now (3.4) and (3.5) are now proved and this ends the proof.
Remark 3.1. In case of m fixed and by considering the limit as
we also obtain the corresponding results for a case of 1-dimensional pairwise ND random variables.
4. Example
Example 4.1 A box contains pq balls of p different colors and q different sizes in each color. Pick 2 balls randomly.
Let and
be a random variable indicating the presence of a ball of the ith color and the jth size such that
For let
be a random variable defined by
Proof. By a direct calculation, we have’s are pairwise ND random variables, i.e. for
that
and
Note that
and
Hence,
By applying Theorem 1.6, for any double sequence such that
for every m
, we have
as
5. Acknowledgements
The authors would like to thank referees for valuable comments and suggestions which have helped improving our work. The first author gives an appreciation and thanks to the Institute for the Promotion of Teaching Science and Technology for financial support.
REFERENCES
- S. Csörgő, K. Tandori and V. Totik, “On the Strong Law of Large Numbers for Pairwise Independent Random Variables,” Acta Mathematica Hungarica, Vol. 42, No. 3- 4, 1983, pp. 319-330. doi:10.1007/BF01956779
- N. Etemadi, “An Elementary Proof of the Strong Law of Large Numbers,” Zeitschrift für Wahrscheinlichkeitstheorie und Verwandte Gebiete, Vol. 55, No. 1, 1981, pp. 119-122.
- R. G. Laha and V. K. Rohatgi, “Probability Theory,” John Wiley & Sons, Hoboken, 1979.
- T. Birkel, “A Note on the Strong Law of Large Numbers for Positively Dependent Random Variables,” Statistics & Probability Letters, Vol. 7, No. 1, 1989, pp. 17-20. doi:10.1016/0167-7152(88)90080-6
- H. A. Azarnoosh, “On the Law of Large Numbers for Negatively Dependent Random Variables,” Pakistan Journal of Statistics, Vol. 19, No. 1, 2003, pp. 15-23.
- H. R. Nili Sani, H. A. Azarnoosh and A. Bozorgnia, “The Strong Law of Large Numbers for Pairwise Negatively Dependent Random Variables,” Iranian Journal of Science & Technology, Vol. 28, No. A2, 2004, pp. 211-217.
- T. S. Kim, H. Y. Beak and H. Y. Seo, “On Strong Laws of Large Numbers for 2-Dimensional Positively Dependent Random Variables,” Bulletin of the Korean Mathematical Society, Vol. 35, No. 4, 1998, pp. 709-718.
- T. S. Kim, H. Y. Beak and K. H. Han, “On the Almost Sure Convergence of Weighted Sums of 2-Dimensional Arrays of Positive Dependent Random Variables,” Communications of the Korean Mathematical Society, Vol. 14, No. 4, 1999, pp. 797-804.
- F. Móricz, “The Kronecker Lemmas for Multiple Series and Some Applications,” Acta Mathematica Academiae Scientiarum Hungaricae, Vol. 37, No. 1-3, 1981, pp. 39- 50. doi:10.1007/BF01904871
- N. Ebrahimi and M. Ghosh, “Multivariate Negative Dependence,” Communications in Statistics—Theory and Methods, Vol. A10, No. 4, 1981, pp. 307-337.
- K. L. Chung, “A Course in Probability Theory,” Academic Press, London, 2001.