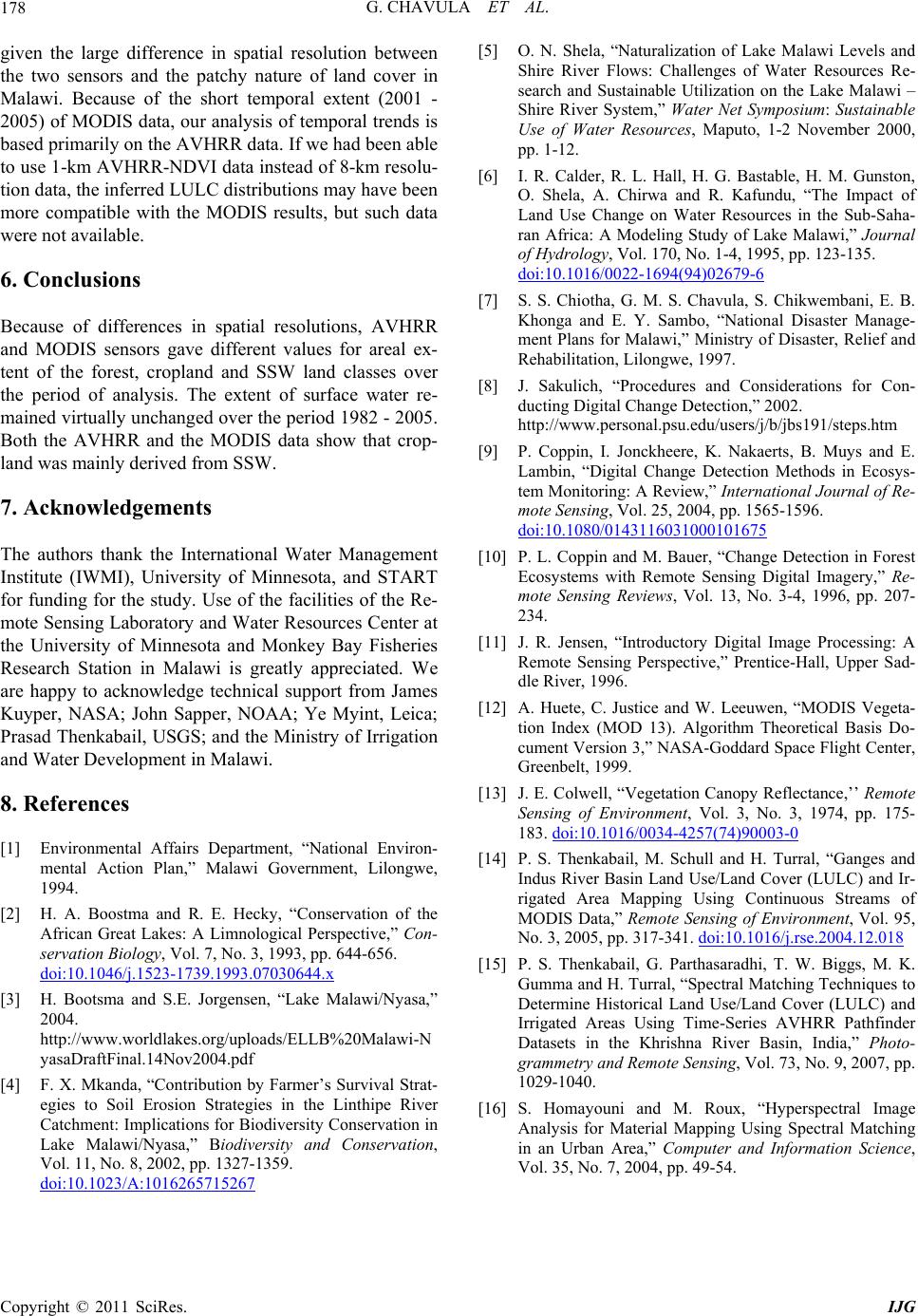
G. CHAVULA ET AL.
Copyright © 2011 SciRes. IJG
178
given the large difference in spatial resolution between
the two sensors and the patchy nature of land cover in
Malawi. Because of the short temporal extent (2001 -
2005) of MODIS data, our analysis of temporal trends is
based primarily on the AVHRR data. If we had been able
to use 1-km AVHRR-NDVI data instead of 8-km resolu-
tion data, the inferred LULC distributions may have been
more compatible with the MODIS results, but such data
were not available.
6. Conclusions
Because of differences in spatial resolutions, AVHRR
and MODIS sensors gave different values for areal ex-
tent of the forest, cropland and SSW land classes over
the period of analysis. The extent of surface water re-
mained virtually unchanged over the period 1982 - 2005.
Both the AVHRR and the MODIS data show that crop-
land was mainly derived from SSW.
7. Acknowledgements
The authors thank the International Water Management
Institute (IWMI), University of Minnesota, and START
for funding for the study. Use of the facilities of the Re-
mote Sensing Laboratory and Water Resources Center at
the University of Minnesota and Monkey Bay Fisheries
Research Station in Malawi is greatly appreciated. We
are happy to acknowledge technical support from James
Kuyper, NASA; John Sapper, NOAA; Ye Myint, Leica;
Prasad Thenkabail, USGS; and the Ministry of Irrigation
and Water Development in Malawi.
8. References
[1] Environmental Affairs Department, “National Environ-
mental Action Plan,” Malawi Government, Lilongwe,
1994.
[2] H. A. Boostma and R. E. Hecky, “Conservation of the
African Great Lakes: A Limnological Perspective,” Con-
servation Biology, Vol. 7, No. 3, 1993, pp. 644-656.
doi:10.1046/j.1523-1739.1993.07030644.x
[3] H. Bootsma and S.E. Jorgensen, “Lake Malawi/Nyasa,”
2004.
http://www.worldlakes.org/uploads/ELLB%20Malawi-N
yasaDraftFinal.14Nov2004.pdf
[4] F. X. Mkanda, “Contribution by Farmer’s Survival Strat-
egies to Soil Erosion Strategies in the Linthipe River
Catchment: Implications for Biodiversity Conservation in
Lake Malawi/Nyasa,” Biodiversity and Conservation,
Vol. 11, No. 8, 2002, pp. 1327-1359.
doi:10.1023/A:1016265715267
[5] O. N. Shela, “Naturalization of Lake Malawi Levels and
Shire River Flows: Challenges of Water Resources Re-
search and Sustainable Utilization on the Lake Malawi –
Shire River System,” Water Net Symposium: Sustainable
Use of Water Resources, Maputo, 1-2 November 2000,
pp. 1-12.
[6] I. R. Calder, R. L. Hall, H. G. Bastable, H. M. Gunston,
O. Shela, A. Chirwa and R. Kafundu, “The Impact of
Land Use Change on Water Resources in the Sub-Saha-
ran Africa: A Modeling Study of Lake Malawi,” Journal
of Hydrology, Vol. 170, No. 1-4, 1995, pp. 123-135.
doi:10.1016/0022-1694(94)02679-6
[7] S. S. Chiotha, G. M. S. Chavula, S. Chikwembani, E. B.
Khonga and E. Y. Sambo, “National Disaster Manage-
ment Plans for Malawi,” Ministry of Disaster, Relief and
Rehabilitation, Lilongwe, 1997.
[8] J. Sakulich, “Procedures and Considerations for Con-
ducting Digital Change Detection,” 2002.
http://www.personal.psu.edu/users/j/b/jbs191/steps.htm
[9] P. Coppin, I. Jonckheere, K. Nakaerts, B. Muys and E.
Lambin, “Digital Change Detection Methods in Ecosys-
tem Monitoring: A Review,” International Journal of Re-
mote Sensing, Vol. 25, 2004, pp. 1565-1596.
doi:10.1080/0143116031000101675
[10] P. L. Coppin and M. Bauer, “Change Detection in Forest
Ecosystems with Remote Sensing Digital Imagery,” Re-
mote Sensing Reviews, Vol. 13, No. 3-4, 1996, pp. 207-
234.
[11] J. R. Jensen, “Introductory Digital Image Processing: A
Remote Sensing Perspective,” Prentice-Hall, Upper Sad-
dle River, 1996.
[12] A. Huete, C. Justice and W. Leeuwen, “MODIS Vegeta-
tion Index (MOD 13). Algorithm Theoretical Basis Do-
cument Version 3,” NASA-Goddard Space Flight Center,
Greenbelt, 1999.
[13] J. E. Colwell, “Vegetation Canopy Reflectance,’’ Remote
Sensing of Environment, Vol. 3, No. 3, 1974, pp. 175-
183. doi:10.1016/0034-4257(74)90003-0
[14] P. S. Thenkabail, M. Schull and H. Turral, “Ganges and
Indus River Basin Land Use/Land Cover (LULC) and Ir-
rigated Area Mapping Using Continuous Streams of
MODIS Data,” Remote Sensing of Environment, Vol. 95,
No. 3, 2005, pp. 317-341. doi:10.1016/j.rse.2004.12.018
[15] P. S. Thenkabail, G. Parthasaradhi, T. W. Biggs, M. K.
Gumma and H. Turral, “Spectral Matching Techniques to
Determine Historical Land Use/Land Cover (LULC) and
Irrigated Areas Using Time-Series AVHRR Pathfinder
Datasets in the Khrishna River Basin, India,” Photo-
grammetry and Remote Sensing, Vol. 73, No. 9, 2007, pp.
1029-1040.
[16] S. Homayouni and M. Roux, “Hyperspectral Image
Analysis for Material Mapping Using Spectral Matching
in an Urban Area,” Computer and Information Science,
Vol. 35, No. 7, 2004, pp. 49-54.