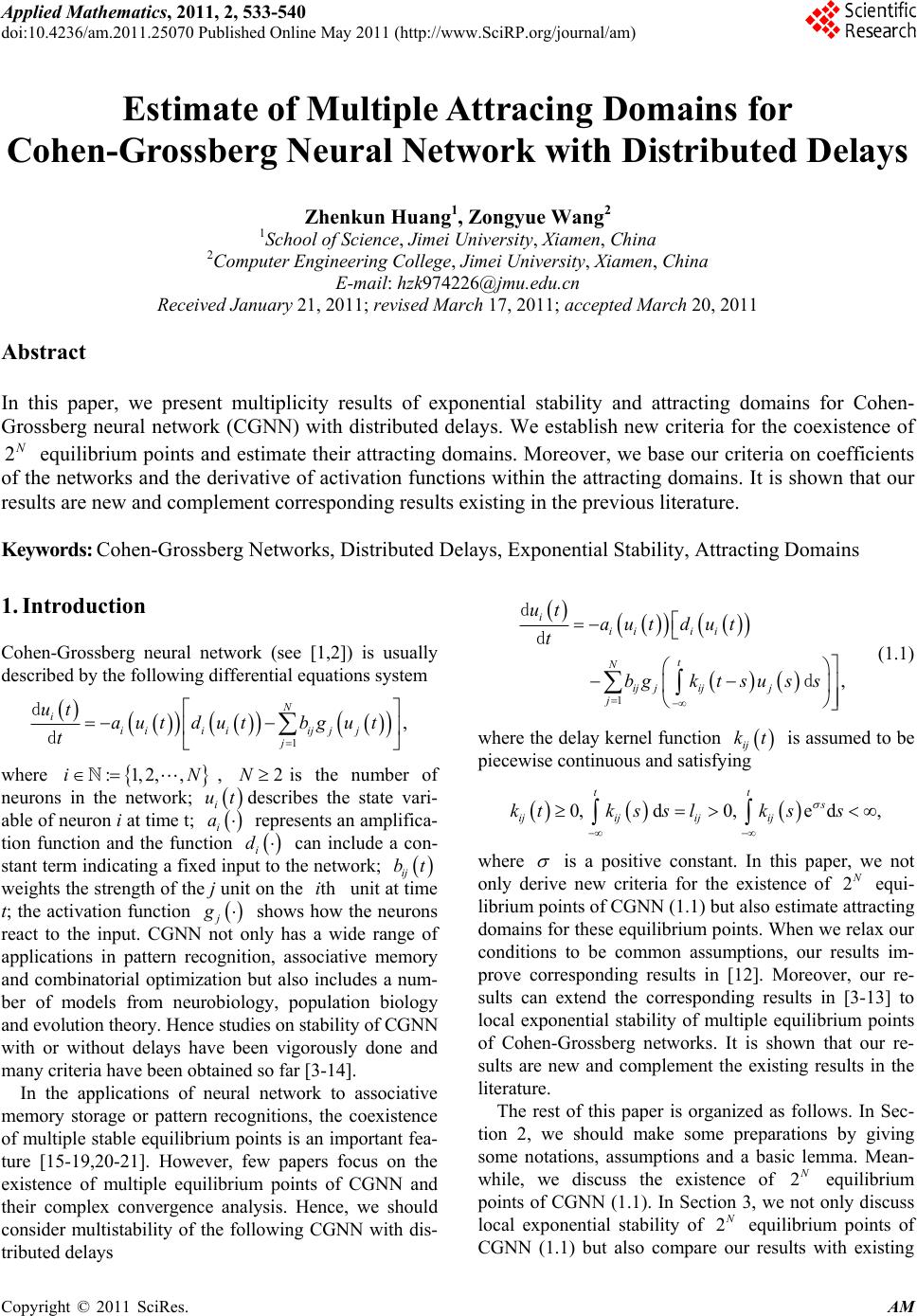 Applied Mathematics, 2011, 2, 533-540 doi:10.4236/am.2011.25070 Published Online May 2011 (http://www.SciRP.org/journal/am) Copyright © 2011 SciRes. AM Estimate of Multiple Attracing Domains for Cohen-Grossberg Neural Network with Distributed Delays Zhenkun Huang1, Zongyue Wang2 1School of Science, Jimei University, Xiamen, China 2Computer Engineering College, Jimei University, Xiamen, China E-mail: hzk974226@jmu.edu.cn Received January 21, 2011; revised March 17, 2011; accepted Marc h 20, 2011 Abstract In this paper, we present multiplicity results of exponential stability and attracting domains for Cohen- Grossberg neural network (CGNN) with distributed delays. We establish new criteria for the coexistence of equilibrium points and estimate their attracting domains. Moreover, we base our criteria on coefficients of the networks and the derivative of activation functions within the attracting domains. It is shown that our results are new and complement corresponding results existing in the previous literature. 2N Keywords: Cohen-Grossberg Networks, Distributed Delays, Exponential Stability, Attracting Domains 1. Introduction Cohen-Grossberg neural network (see [1,2]) is usually described by the following differential equations system 1, N iiiiiijj j j ut autdutbgut t d d where , is the number of neurons in the network; describes the state vari- able of neuron i at time t; i :1,2,,i i a N2N ut represents an amplifica- tion function and the function can include a con- stant term indicating a fixed input to the network; i d ij bt weights the strength of the j unit on the unit at time t; the activation function thi j g shows how the neurons react to the input. CGNN not only has a wide range of applications in pattern recognition, associative memory and combinatorial optimization but also includes a num- ber of models from neurobiology, population biology and evolution theory. Hence studies on stability of CGNN with or without delays have been vigorously done and many criteria have been obtained so far [3-14]. In the applications of neural network to associative memory storage or pattern recognitions, the coexistence of multiple stable equilibrium points is an important fea- ture [15-19,20-21]. However, few papers focus on the existence of multiple equilibrium points of CGNN and their complex convergence analysis. Hence, we should consider multistability of the following CGNN with dis- tributed delays 1, i ut ii ii t N ij jijj j aut dut t bgk t su ss d d (1.1) where the delay kern el function is assumed to be where d ij kt ng piecewise continuous and satisfyi tt 0,d0,e d, s ij ijij ij ktksslks s is a positive constant. In this paper, we not only de new criteria for the existence of 2 rive equi- librium points of CGNN (1.1) but also estimate atacting domains for these equilibrium points. When we relax our conditions to be common assumptions, our results im- prove corresponding results in [12]. Moreover, our re- sults can extend the corresponding results in [3-13] to local exponential stability of multiple equilibrium points of Cohen-Grossberg networks. It is shown that our re- sults are new and complement the existing results in the literature. The rest tr of this paper is organized as follows. In Sec- tion 2, we should make some preparations by giving some notations, assumptions and a basic lemma. Mean- while, we discuss the existence of 2 equilibrium points of CGNN (1.1). In Section 3, we only discuss local exponential stability of 2not equilibrium points of CGNN (1.1) but also compareur results with existing o
 Z. K. HUANG ET AL. 534 . Coexistence of Equilibrium Points this paper, we denote by ones in the literature. In Section 4, two examples are given to illustrate the new results. Finally, concluding re- marks are given in Section 5. 2 In ,0 ,N C pings from the set of all continuous and bounded map ,0 to N equipped with p-norm 1 pp defined by 0 1sup , N p i s i s Where 12 ,,, ,0, NC , For any given ,0 , C, we denote by ;ut the solutio with us s all n of CGNN (1.1) for ,0s . Given any 0, we define ;s u us ; for all ,0 , s then ;,0, uC . Throughout this paper we always assume that 1 S inedFo ,. For each and there r each i, i a is a continuous function def on . Meil assume that 01 0av anwhe, we iii v 2 S exist i, , i d i a, , i 2 i gC constants † i d suc that h † 0,lim, 0limsup 0, 0, 0, ii iii v ii ii vv ii i ddvd gvg gv gvgv gv vgvdvv v where i and l networks [5 2 iiii bl ark 2.1. limvi dv . Hopfield-type neu Rem For ra ,8-10], we have 1 i av, ii dv dv, tanh i vv, where 0 i d is at. y, w that dvd, 0 i dv , constanObviousle can check ii lim tanh1 vv , '' 1 sup1 limv tanhvv 0, tanh 0v , nce, 2 tanh '' 0vv. He tanh v tanh v ' 1 and T S W a consta 2 Se hold. say nt vector 1,, N ) if for each i, uuu is an eq Consider uilibrium point of CGNN (1.1 N 1 iiijjj j dut bgut iiiiii vdvbgut where . Then it follows ma 2 Assund the following assumption v, i Lem .1.me 12 SS a 1, Aiii Hd bi sup iii v l gv i hold. For each , there exist only twpoints and with o 1i v 2i v12 0 ii vv such that Fv 0 ii and 2 sgn 0, iii Fvv v 1 v v where v a 1,2.vv nd , i Proof. We get from A and t 1 H 2 S tha 0 i 00 0 iii iii Fdblg and †† lim 0 ii v iiiiii Fvb gdl dv as . It follows from that v 2 S 0 i vF v which implies that i v is strictly increasing on ,0 ore, there 0 andly decreasing on . exist only two points 1i v and v with 12ii v is strict 0, Theref 2iv such that 0 ii Fv and n 0,v vv 12iii sgFv v and 2.,1, i vv v where The proof is com- plete. It follows from 1 A H0 for each i ii b that . Now, we considwier the follong additional assumption: 21,1,2, N Aii ijj HFvbg i 1, jj i in 2 A H, it is easy for us to get that Take 1 10, ijj vbgi 1, ii jj i F N ( 2.1) Due to i Fv as L the continuity of v,emma 2.1 and i v, there exists a unique with † v1i 11ii v † v suc h that † 2 N ii v 1, † 22 1, 0, 0, forall, ijj jji N iijjii jji F bg vbg vvv (2.2) Take 2 in 2 A H ere exists a unique ,by similar argument, we de- rive that t with h† v2i22ii † vv such that † 11, † 11 1, 0, 0, forall,1 ii ijj jji N ii ijjii jji Fv bg N vbg vvv (2.3) Let 12 11 11 , ii NN iijj iij jj v dbgvdbg j Due to the monotonicity of i d, it is easy for us to Copyright © 2011 SciRes. AM
 Z. K. HUANG ET AL.535 ch 2 Feck that †† 11 2 0 ii i vv vv or each i i . , de- fine 1 i . Hence, wcon- struc †† 112 2 ,, , iii ii vv vv 2 t 2 e can subsets 1 12 2N N , where 12 ,,, N with i1, 2 , i e : . following theoremWith these notations, whe have t Theorem 2.1. Under the assumptions 12 SS AA and 12 HH, there exist at least 2 e point.1). Proof. For each , quilibrium N s of CGNN (1 12 ,, with 1, 2 i , i, we define , N12 ,, FF F h : tWi F by 1 iii j Fd u 12 ,,, T N uu u , jjijj glu 1N b re whe , we have . It is obvioforu us that any u 12 , iii vv u for each ich i . For ea , i,s for us to fur discussion. Case I: If 1 i there ar e two case rthe , †, then from i.e., 1ii uv 1 A H and (2.2) we get 1 1 1 1, N ii jjij j N iii iiij d bglu dbglv If 2 i † ij j ji bg † (2.4) C 11ii v ij Fu ase II: , i.e. , uv† 2ii , then from 1 A H and (2.3) we get 1 1 1 1, N ii jjij j N iiiiii j bglu dbglv i ii F †† 22ii v ij Hence ijj ji bg (2.5) Fu d u, that is y Brouwer's Fu theory, f for or eall . Bfixed point ach u , ist at least one u such that there ex u u. Therefore, there exist at least 2 equilibriu CGNN (1.1). T he pro of is compe. Next we should make some preparations for the com- in m points of let g section. For each i, we define the following subsets of ,0 ,C as 1 2 ,0 , i N i N 1 2 † † i i ,0 , Csv Csv we have construct 2 Hence, subsets 1 1 HH 2 2,0 N, N HC . GiveHn any , N12 ,, with 1, 2 i , we defi subsets for each ne semi-close i , 1 †. i Ov v 1 11 i i v For any , we12 12 N N OOOO get ,0 . nd st ssume s for all ility aimation of Attracting Domains Th that assumptions 3. Stab E eorem 3.1. A 12 SS an 12 AA HH d hold. For each , if , then ; t uH for all t . Proof. Fix 12 ,,, N . Fo any r , we that should prove ; t uH for all t. For each i , we onlye 2 i consider the cas , i.e., i † 2i v for all ,0 . s rt that, fo sufficien- all We asser any tly sm † 22 0vv , theion ii solut ; itu † 2i v hold0t. If this is not true, there ex- ists a * t s for all such that 0 i ut † 2 i v , * i ut 0 and i ut † 2 i v for * ,tt . Due to 1 from CG A H, (2.3) and thetonicity ofrivNN mono i g, we dee (1.1) that * d i ut t † 2 † 21 † 2 †† 22 1 1 d 0 ii tN ii iiijij j j ii N iiiiiiijj j N iiij j j av dv gktsussbg av dv glvbg av bg †† 22 ii ii ii b b Fv d (3.1) which leads to a contradiction. Since the choice of is arbitrary, for , if † i each i 2 i v for all ,0s , then † 2 ; ii ut v holds for all t. When 1 i 0 , similar aent can ormed to show tif rgumbe perf hat i† 1 i v for all ,0s , n the ; ii ut † 1 v holds for all 0t. Hence, for any , we have that ; for 0. The lete. ition 3.1. Let t uHall t proof is comp Defin , N u u be aneuilib- rium point of CGN 12 ,,uu .1) and q N (1 ,0 , Yu u C be a neighborhood of . If there exist 0 and Copyright © 2011 SciRes. AM
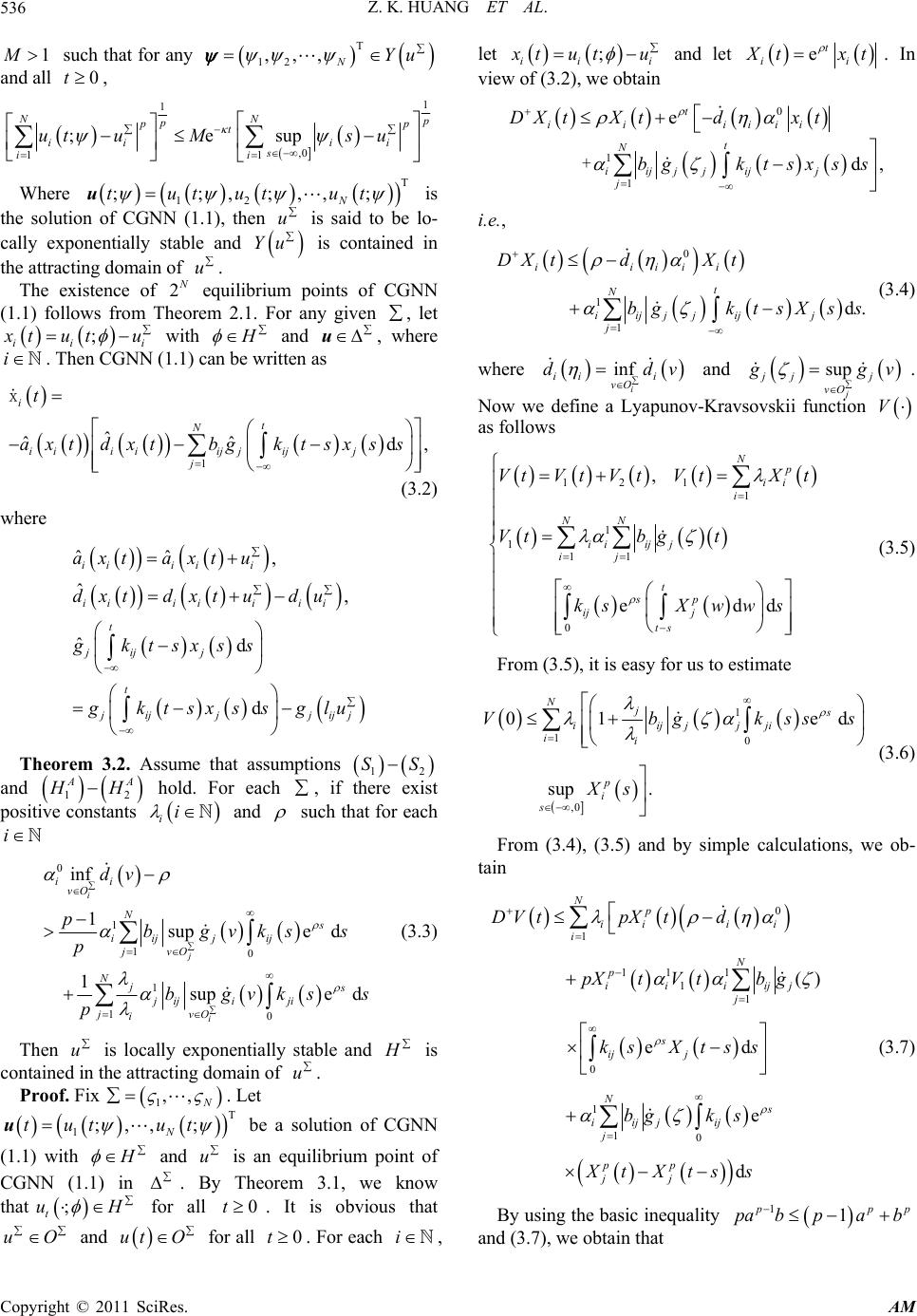 Z. K. HUANG ET AL. 536 1 such that for a TYu ny 12 ,,, N and all 0t, 1 1 NN ,0 11 sup;e p pp tii s ii uts u ii u M Where is the solu lo- cally exponentn the attracting dom istence of equilibrium points of CG or T 12 ;;,;,,; N tututut u tion of CGNN (1.1), then u is said to be ially stable and Yu is contained i ain of u. N The exNN (1.1) follows from Theorem 2.1. Fany given 2 , let ; ii i tut u with and u, where i. Then CGNN (1.1) bcane written where as 1 ˆ d, i N iiiijjij j t xt dxtbgktss x ˆ ˆt ij axs (3.2) ˆˆ , ˆ, ˆd d iiiii iiiii ii t jij j t ijjjijj axtaxtu dxtdxtudu gktsxss ktsxssglu Theorem 3.2. Assume that assumptions 12 SS , if there exist and hold. For each and 12 AA HH positive constants ii such that for each i 0 1 1 i Ns p 10 1 10 inf supe d 1supe d j i ii vO iijj ij jvO Njs jij iji jvO i dv bgvkss p bgvkss p (3.3) Then is locally exponentially stable and u is containehe attracting domain of Proof. Fix d in tu. 1,, T . Let be a solution of CGNN 1;,, ; N tutut u (1.1) with and u is an equilibrium point of CGin . By TheoreNN (1.1) m 3.1, we know that ; t uH for all t uO and ut O for 0. It is obvious that 0t. For each iall , let ; ii i t uut and let et ii txt . In view of (3.2), we obtain 0 1 e , t ii ii t jj j tx t g k 1d ii N iijijj DX tXd btsx ss + i.e., 0 1 1d. iiiii t N iijjjij j j DX tdX t bgk tsXss (3.4) where inf i ii i vO dd v and sup j jj j vO gv sovskii function . Now v-Kravwe define a Lyapuno V as follows 1 1 i NN ij Vt t Vtbg t 1211 11 0 , edd Np ii iiij j t sp ij j ts VtVtVXt ksX wws (3.5) From (3.5), it is easy for us to estimate sup . p i Xs 1 10 ,0 01 ed Njs iijjjji ii s Vbgksss (3.6) From (3.4), (3.5) and by simple calculations, we ob- tain 0 1 11 1() N p pX tVtb g 11 0 1 10 ed e d Np iii i i iiiijj j s ij j Ns iijj ij j pp jj DV tpXtd ks Xtss bgk s Xt Xts s (3.7) By using the basic inequality 11 pp pa bpab and (3.7), we obtain that Copyright © 2011 SciRes. AM
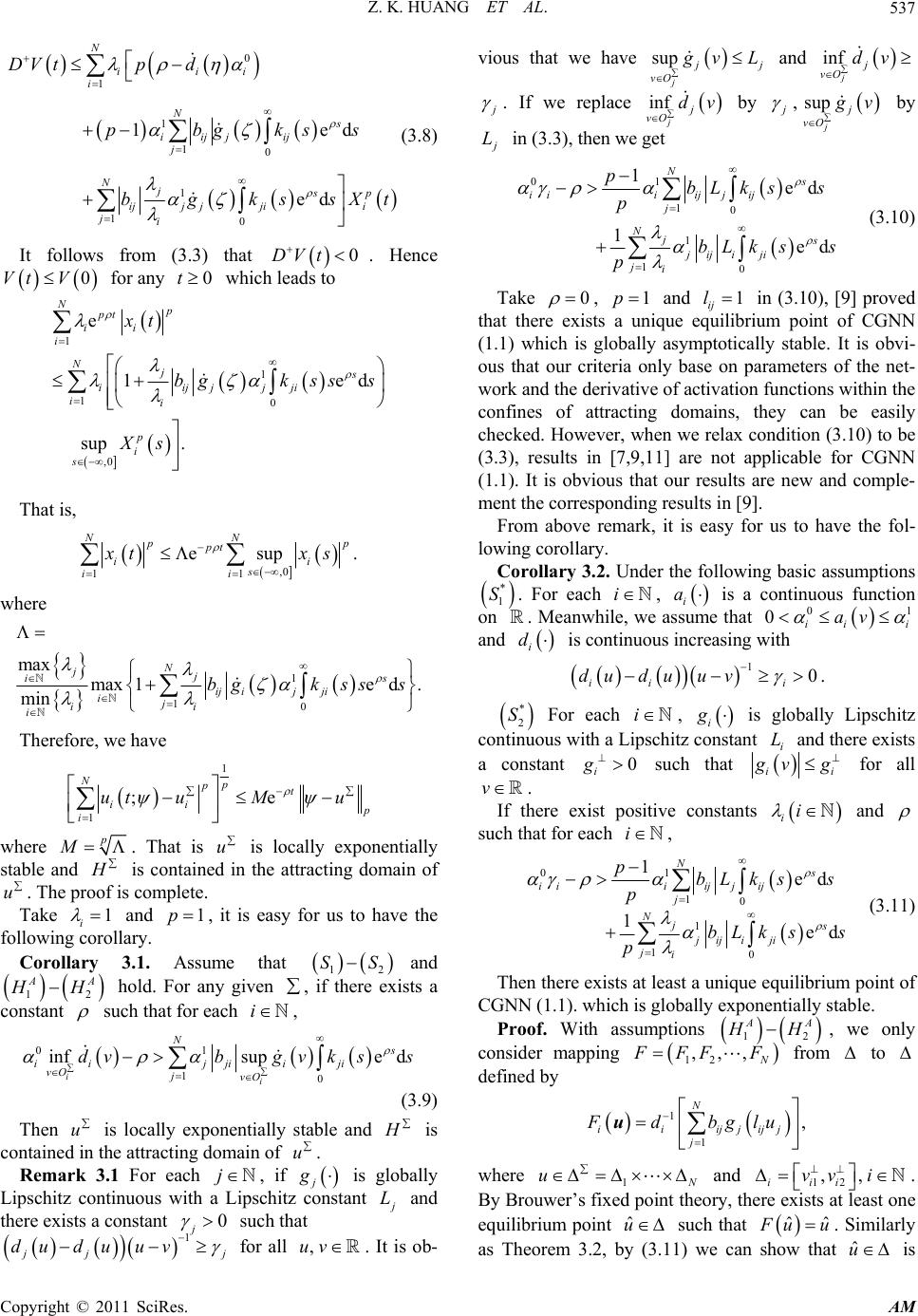 Z. K. HUANG ET AL.537 p i Xt 0 1 1 10 1 10 1ed ed N iii i Ns iijj ij j Njs ijj jji ji DV tpd pbgkss bgkss (3.8) It follows from (3.3) that . Hence for any 0DV t which leads to 0Vt V0t 1 1 1 1 Nj ii j ii bg 0 ,0 e ed sup . p pt ii i s jjji p i s xt k sss Xs That is, N ,0 11 esup NN . p pt ii s ii xt xs where 1 10 max max 1ed. min N jjs iij ijji ij ii i bgk sss Therefore, we have 1 1;e Np pt ii i ut uMu where p M. That is u is locally exponentially st able and 1 is containe the attracting domain of omplete. Take d in u. The proof is c i and is easy for us tve the following crollary. llary 3.1. Assume that 1p, ito ha o Coro 12 SS , if there exand A hold. For any given ists a A 12 HH constant such that for each i, 01 10 infsupe d ii Ns ii jjiiji vO jvO dvbgvk ss (3.9) Then is locally exponentially stable and u is containehe attracting domain of Rem 1 For each d in t ark 3.u. j Li, if is tz continu g jglobally Lipschious with apschitz constant L and there exists nstant 0 j a co such that 1 jj du du uv for all ,uv. It is ob- us that we have sup j vio j vO vL and inf jj vO dv . If we replace inf dv by jj vO , sup j j vO v by L in (3.3), then we get 01 1N p 10 1 10 ed 1ed s ii iijjij j Njs jij iji ji bL k ss p bLk ss p (3.) 10 Take 0 , 1p and 1 ij l in (3.10), [9] ved that there exists a unique equilibriumpro point the e of acon s with confines of attracting dom can en we relax co (3.10) to be (3.3), results in [7,9,11] are not applicable for CGNN (1. resunm me sults in [9]. is easy for us to have the fol- lowing corollary. Corolla ry 3.2. Under the following basic assumptions of CGNN (1.1) which is globally asymptotically stable. It is obvi- ous that our criteria only base on parameters of net- work and the deriv ativtivation functiin the ains, theybe easily checked. However, whndition 1). It is obvious that ourlts are ew and cople- nt the corresponding re From above remark, it * 1 S. For each i , i a eis a continuou that s function on . Meanwhile, we assum 01 0ii av i and i d is csing ontinuous increawith 10 iii u u du dv . * 2 S For each i , i g is globally Lipschitz continuous with a Lipschitz constant i L and there exists a constant 0 i g such that ii vg for all v . If there exist positive constants ii and such that for each i , 01 1N iiiijjij pbLk 10 j N p (3.11) 1 ed 1 s js s s hequilibrium point o Nly stable. W , we only consider mapping 10 ed ji j iji ji bLk ss p T en there exists at least a unique f CGN (1.1). which is globally expone ntial Proof. ith assumptions AA HH 12 ,,, 12 FFF from to defined by 1 1, N iijj j Fdbglu iijj u where 1 u and , ii i . By Brouwer’s fixed ory, ther equilibrium point 12 , i vv e exists at least onepoint the ˆ u such that ˆˆ uu. Similarly n show that ˆ u as Thewe caorem 3.2, by (3.11) is Copyright © 2011 SciRes. AM
 Z. K. HUANG ET AL. Copyright © 2011 SciRes. AM 538 the unique equilibrium point of CGNN (1.1) which is globally exponentially stable. The proof is complete. Remark 3.2. Take 11 1 22211 12 21 11 2212 21 1222 1,1.4, 3.1 ,6 0.125e ,0.25e , e,tanh , 3.6742, 3.1458, 10. ss s dut ut dututb ks ks ks ksgvgvv bbb , 1122 aut aut and . When 1 ij l1,2p , 0 Corollary 3.2 leads to Theorem 3.2 and Theorem 3.3 in [17]. It is obvious that Theorem 3.2-3.5 in [9] are only ou vior of C 4. Examples Consider the following Cohen-Grossberg networks with ted delays r special cases of Corollary 3.2. Furthermore, our re- sults can extend the existence of multiple equilibrium points and their attracting behaohen-Grossberg networks to [3-12]. distribu 12 2 122 1 222 22 21 1 d N j t N j bg kt su ss ut autdu t t b d d (4.1) 1 211 22 2222 d d t t N ut gktsuss bgksu ss der 111 11 11 1111 1d t N j ut adut t bgktsuss d d 1j Example 4.1 Consi Since 112 2ii i bL bL and (i Li are defined in Co 3.2), most resultrollarys reorted in [7,9-11] could not applicable for CGNN (4.1) even though we take de- lay kernels into consideration. It is obvious that p 12 SS hold and we have 1122 112112 12 00 112 12 d1, 2d1 1.46,3.1 10, 1 llkss llkss 2 vvgvFvvg gg v From some computations, we get 11 1.3565v , 12 v1.3565 , 21 1.19v , 22 1.19v such that 0 ii Fv , hence 1 3.3 2 11 Fv 1, 5 22 v , where 44 and 1 4.6168 F . From (2.2)-( we2.3), ††† .8190 1.81901.91vvv can estimate † 111221 22 1 .9v It is easy for us to get 12 0.0999, 0.0086gv gv . 1) satisfied our assumptions inTherefore, CGNN (4. Theorem 3.2 and assumptions 12 AA HH hold. Let 1,1 16p and 12 1 e calcula- check tolds. By Theorem 3.2, there exist only four locally exponentially stable equilib- rium points of CGNN (4.1) located in . Moreover, their attracting domains can be estimated b . From som h y tions, we can hat (3.3) 1,1 2 1212 1,2 2 1212 2,1 2 121 2 2,2 2 1212 ,,0 ,1.1819,1.9 ,,0 ,1.1819,1.9 ,,0 ,1.1819,1.9 ,,0 ,1.1819,1.9 HC ss HC ss HC ss HCss Example 4.2 Consider We can estimate that So, ' † 11 1 ' † 22 2 '' '' 111 '' '' 212 1.381.4 0.02tanh1.4, 3.083.10.02tanh3.1, 0.02tanh0tanh , 0.02tanh0tanh . ddv vd ddv vd vd vvvbv vd vvvbv 112 2 11 11 22 22 11 11122122 12 12 211222 12 1, 1.4 0.02tanh, 3.1 0.02tanh, 6,e , e tanh ,, 8 3.6724, 3.6724, 10, 3.1458 s s aut aut dut utut dututut b ksksksks gvgvvk s bbbb 12 SS 11 1.37 hold. Similarly as Example 4.1, we get v , 12 1.37v , , 21 1.21v22 1.21v such that ii Fv 0 , hence 11 Fv 13.372 and
 Z. K. HUANG ET AL.539 , where 22 14.633Fv 1, 2 . † 1.91v ptions ere exist From (2.2)-(2 .3), sum and 3.2, th CGNN we can estimate †† 11 12 1.85vv We can check that as (3.3) hold. By Theorem equilibrium points of † 21 22 1.851.91v 12 AA HH only four stable (4.1) located in . Moreover, their attracting domains can be esti- mated as Example 4.1. 5. Concluding Remarks In this paper, some new c istence of 2N equilibrium also given for each e results are new and co [7,9-11]. Furthermore, o ding ones reported in [2 ity of multiple equilibrium 6. Acknowledgements This work was support Pr ding of Jimei Univ ersity ( gram Foundation fo Research Talents of Fujian JA10198). 7. References petitive Ne Systems, Man and C [2] S. Grossberg, “N Mechanisms and Archit 622-634. Grossberg Neur riterived for the coex- poidomain is quilibrium point. It is shown that our mplemprevious results in ur rextend correspon- 2-23] to local exponential stabil- poof neural network. byation for Young ersity, Scientific Research Fun- ZQ201 and Training Pro- r Distinguung Scholars and ucation (JA10184, Formation and l Me Stora EE Transactio onlinearal Networks: Principles, ecturNeural NetworksV y hav nd TheVol. 15, No. 6, 2007, pp. doi:10.1016/j.simpat.2006.12.003 Stability of Cohen- al Networks,” etworks, Vol. 15, Delays,” Journal of Mathematical Analysis and Applica- tions, Vol. 296, No. 2, 2004, pp. 665-685. doi:10.1016/j.jmaa.2004.04.039 [7] X. F. Liao, C. G. Li and K.-W. Wong, “Criteria for Ex- ponential Stability of Cohen-Grossberg Neural Net- works,” Neural Networks, Vol. 17, No. 10, 2004, pp. 1401-1414. doi:10.1016/j.neunet.2004.08.007 [8] C. X. Huang and L. H. Huang, “Dynamics of a Class of Cohen-Grossberg Neural Networks with Time-Varying Delays,” Nonlinear Analysis: Real World Applications, Vol. 8, No. 1, 2007, pp. 40-52. doi:10.1016/j.nonrwa.2005.04.008 en-Grossberg Neural Net- elays,” Applied Mathematics and Computation, Vol. 160, No. 1, 2005, pp. 93-110. un and L. Wan, “Global Exponential Stability and ions of Cohen-Grossberg Neural Networks usly Distributed Delays,” Physica D, Vol. 208, No. 1-2, 2005, pp. 1-20. 6/S0167-2789(02)00544-4 , No. 10, 2006, pp. 6.07.006 l Academy of Sci- . 3088-3092. 17 a are deri nts and attracting ent the sults can e ints the Found 0003) ished Yo Higher Ed Paralle orks,” IE s, Vol. Neur es,” ory, onential Neural N ed ofessors of Jimei Univ do [1] M. Cohen and S. Grossberg, “Absolute Stability and Global Patternmoryge by Comural Netwns on ybernetic 13, No. 5, 1983, pp. 815-825. , ol. 1, [15] J. Hopfield, “Neurons with Graded Response have Col- lective Computational Properties like Those of Two State Neurons,” Proceedings of the Nationa ences, USA, Vol. 81, No. 10, 1984, pp No. 1, 1988, pp. 17-61. doi:10.1016/0893-6080(88)90021-4 [3] Z. K. Huang and Y. H. Xia, “Exponential p-Stabilitof Second Order Cohen-Grossberg Neural Networks with Transmission Delays and Learning Beior,” Simulation Modelling Practice a [4] L. Wang and X. Zou, “Exp No. 3, 2002, pp. 415-422. doi:10.1016/S0893-6080(02)00025-4 [5] T. Chen and L. Rong, “Robust Global Exponential Sta- bility of Cohen-Grossberg Neural Networks with Time Delays,” IEEE Transactions on Neural Networks, Vol. 15, No. 1, 2004, pp. 203-205. doi:10.1109/TNN.2003.822974 [6] J. D. Cao and J. L. Liang, “Boundedness and Stability for Cohen-Grossberg Neural Network with Time-Var ying [9] L. Wang, “Stability of Coh works with Distributed D doi:10.1016/j.amc.2003.09.014 [10] X. F. Liao and C. D. Li, “Global Attractivity of Cohen- Grossberg Model with Finite and Infinite Delays,” Jour- nal of Mathematical Analysis and Applications, Vol. 315, No. 1, 2006, pp. 244-262. doi:10.1016/j.jmaa.2005.04.076 11] J. H. S[Periodic Solut with Continuo i:10.1016/j.physd.2005.05.009 [12] L. Wang and X. F. Zou, “Harmless Delays in Cohen- Grossberg Neural Networks,” Physica D: Nonlinear Phenomena, Vol. 170, No. 2, 2002, pp. 162-173. doi:10.101 [13] K. N. Lu, D. Y. Xu and Z. C. Yang, “Global Attraction and Stability for Cohen-Grossberg Neural Networks with Delays,” Neural Networks, Vol. 19 1538-1549. doi:10.1016/j.neunet.200 [14] Z. K. Huang, X. H. Wang and Y. H. Xia, “Exponential Stability of Impulsive Cohen-Grossberg Networks with Distributed Delays,” International Journal of Circuit Theory and Applications, Vol. 36, No. 3, 2008, pp. 345-365. doi:10.1002/cta.424 doi:10.1073/pnas.81.10.3088 [16] C. Y. Cheng, K. H. Lin and C. W. Shih, “Multistability in Recurrent Neural Networks,” SIAM Journal on Applied Mathematics, Vol. 66, No. 4, 2006, pp. 1301-1320. doi:10.1137/050632440 [17] Z. Zeng, D. S. Huang and Z. Wang, “Memory Pattern Analysis of Cellular Neural Networks,” Physics Letters A, Vol. 342, No. 1-2, 2005, pp. 114-128. doi:10.1016/j.physleta.2005.05.0 [18] C. Y. Cheng, K. H. Lin and C. W. Shih, “Multistability and Convergence in Delayed Neural Networks,” Physica D, Vol. 225, No. 1, 2007, pp. 61-74. doi:10.1016/j.physd.2006.10.003 [19] L. P. Shayer and S. A. Campbell, “Stability, Bifurcation Copyright © 2011 SciRes. AM
 Z. K. HUANG ET AL. Copyright © 2011 SciRes. AM 540 0, pp. 673-700. and l. 57, No. 8, 2010, pp eural 0, 2010, pp. 1643-1655. s, Vol. 15, No. 7, 3-6080(02)00039-4 7-53. 7-7 and Multistability in a System of Two Coupled Neurons with Multiple Time Delays,” SIAM Journal on Applied Mathematics, Vol. 61, No. 2, 200 doi:10.1137/S0036139998344015 [20] Z. K. Huang, Q. K. Song and C. H. Feng, “Multistability in Networks with Self-Excitation and High-Order Synap- tic Connectivity,” IEEE Transactions on Circuits Systems-I: Regular Papers, Vo . [2 2144-215. doi:10.1109/TCSI.2009.2037401 [21] Z. K. Huang, X. H. Wang and C. H. Feng, “Multiperi- odicity of Periodically Oscillated Discrete-Time Neural Networks with Transient Excitatory Self-Connections and Sigmoidal Nonlinearities,” IEEE Transactions on N Networks, Vol. 21, No. 1 doi:10.1109/TNN.2010.2067225 [22] Y. M. Chen, “Global Stability of Neural Networks with Distributed Delays,” Neural Network 2002, pp. 867-871. doi:10.1016/S089 3] H. Y. Zhao, “Global Asymptotic Stability of Hopfield Neural Network involving Distributed Delays,” Neural Networks, Vol. 17, No. 1, 2004, pp. 4 doi:10.1016/S0893-6080(03)0007
|