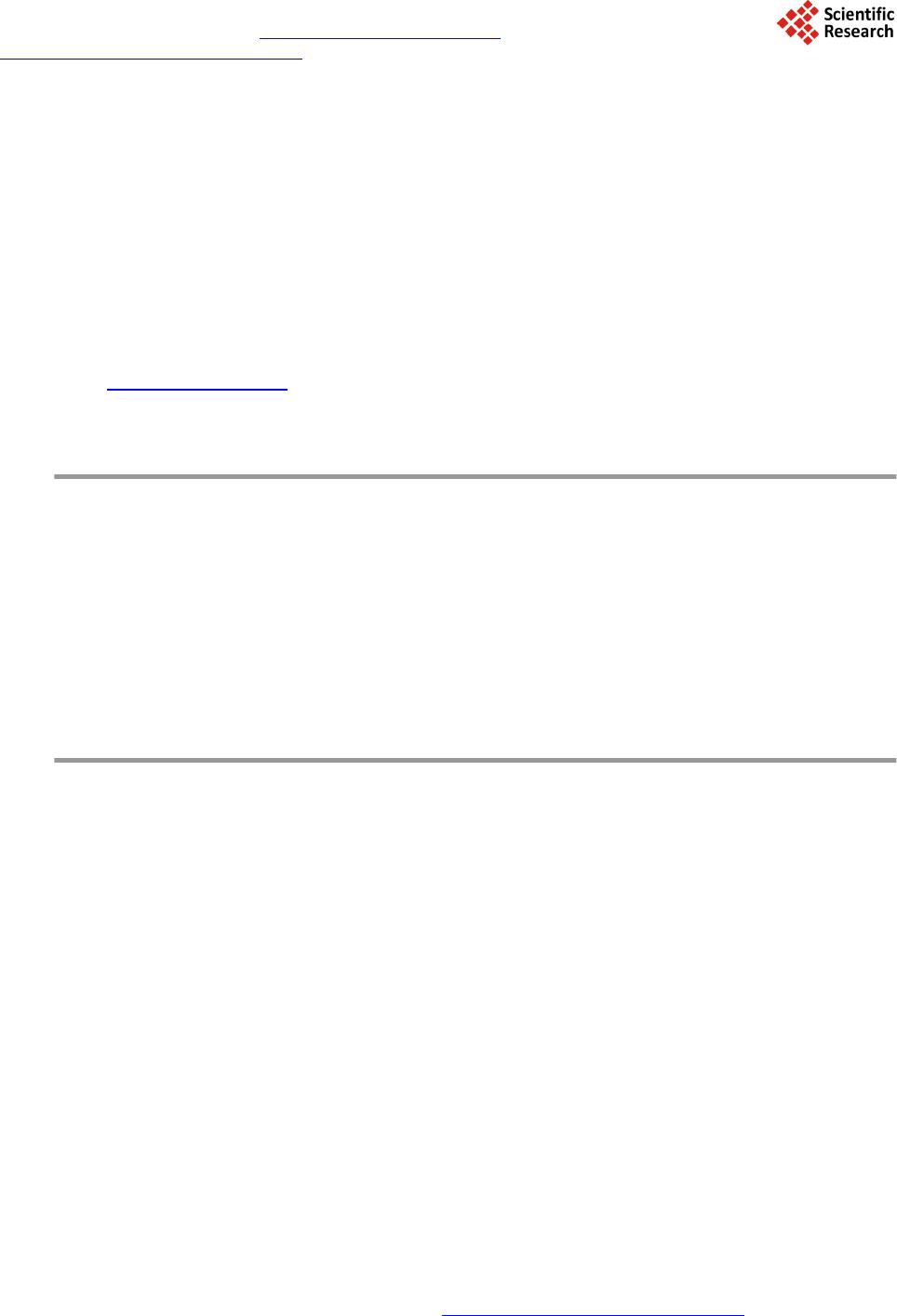 Journal of Applied Mathematics and Physics, 2014, 2, 189-193 Published Online April 2014 in SciRes. http://www.scirp.org/journal/jamp http://dx.doi.org/10.4236/jamp.2014.25023 How to cite this paper: Menshikov, Y.L. (2014) Identification of Mathematical Model Parameters of Stationary Process. Journal of Applied Mathematics and Physics, 2, 189-193. http://dx.doi.org/10.4236/jamp.2014.25023 Identification of Mathematical Model Parameters of Stationary Process Yuri L. Menshikov Department of Mechanics & Mathematics, Dnepropetrovsk University, Gagarina, Dnepropetrovsk, Ukraine Email: Menshikov2003@list .ru Received January 2014 Abstract In work the problem of synthesis of mathematical model of stationary process is examined in de- terministic statement. It is supposed that the amount of measurements by each variable minimally and coincides with number of variable in model. The some possible variants of statement of such problem are considered. The calculations on real measurements were executed for comparison with known methods. Keywords Parameters Identification, Different Statements, Regularization Method 1. Introduction The problem of parameters identification of mathematical models of physical processes in the form of algebraic relations between the initial and final states of the process arises when constructing mathematical models of these processes, in the investigation of optimal or nonstandard regimes of work, etc. [1,2]. If these parameters are considered as constant, it is implicit that the relationship between the initial and the final states of the process varies little during the time of measurement, of constructing a mathematical model and the syntheses of fore- casts (and this change can be ignored). Such physical processes will be called stationary (in the sense they are being repeated). Mathematical models of the same processes may have different structure and different degree сompliance of reality. Linear mathematical models are the most popular, since any smooth function in a small neighborhood of parameters change is well approximated by a linear dependence. The number of variables of state, which are characterized the investigated process, may be different from one to infinity. However, any number of variables of the mathematical model can not be assumed accurate. Usually the number of variables in the model depends on the degree of their influence on the process under study and on the capabilities of the measuring equipment, which are measured by these variables. In practice, the identification of parameters of mathematical models based on measurements of the character- istics selected a priori mathematical model, the overwhelming majority of currently represent methods that use statistical characteristics of the measured values [3-5]. These methods are successfully used in the study of the average characteristics of the physical process, as well as to build a long-term (average) forecast. However, if
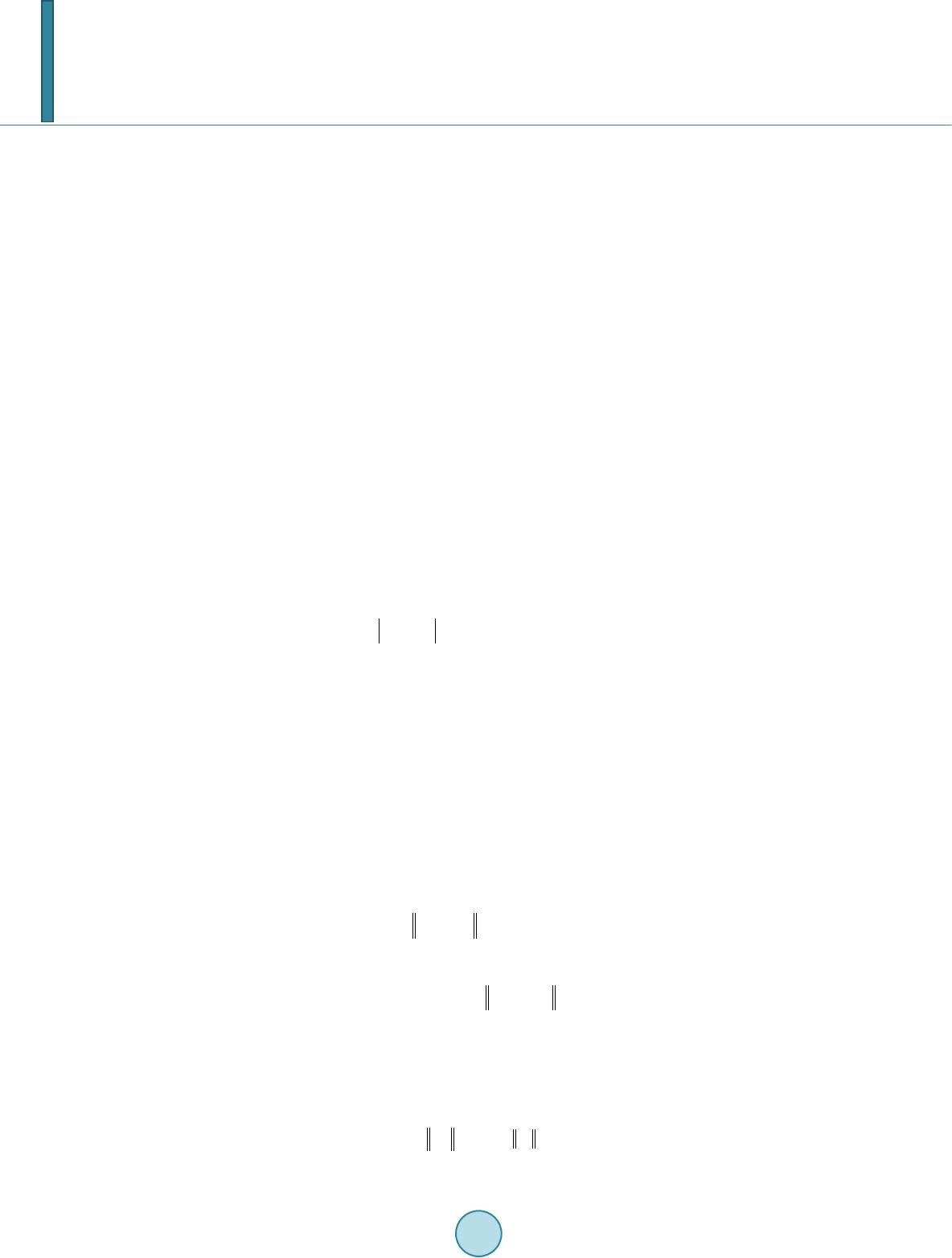 Y. L. Menshikov the identification problems are considered in a stochastic statement, then the class of stationary physical proc- esses should be expanded to include the class of possible physical processes in which the relation between the initial and final states of the process can be variable by averaging of the raw data. Forecast which obtained with help the averaged mathematical model will have only the averaged character. This quality of the forecast does not always satisfy the requirements of practice. Furthermore, in these methods it is assumed that a mathematical model of communication between the aver- aged characteristics of the process is linear. This assumption is not founded. In this paper the different approach to the problem of parameters identification of mathematical models of sta- tionary processes is suggested. This method is suitable for the forecast using a small number of measurements (number of measurements of the characteristics of the process is assumed to be the number of these characteris- tics) [6]. In this approach, the measurement error has interval type and its value is known [7]. 2. Statement of Problem We shall present the problem of synthesis of linear mathematical model with variables rela- tively for number of measurements n, as a problem of the solution of system [6]: , (1) where the operator is determined as follows 2 312231 ( ,,,,) pnnn n A q qqzzqzqzqze − =++ + e is the unit vector of dimension n, is unknown vector of parameters of the mathematical model of the proc- ess. As the measurements of variables are received experimentally it is assumed that each mea- surement has some error the maximal size of which is known: ,1, 1,2,,,, ex ij iji qqj nin δ −≤≤≤ = (2) where is exact measurements of variable . The similar information of measurement errors, as a rule, is known a priori. The statistical characteristics of errors of measurements are unknown. Let us denoted vector as vector from space ( 1) .... nnn nnn RRR RR − ⊕⊕⊕ ⊕= : 212 3131 ( ,.,,,..,,..,,..,) Tnn nnn p qqqqqq= where is Euclidean vector space, is sign of transposition. Each vector can accept meanings in some closed area Di⊂Rn by virtue of inequalities (2). Vectors p can accept meanings in some closed area ( 1) 234 .... nn n DDD DDR − =⊕⊕⊕⊕ ⊂ . The certain operator Ap associ- ates with each vector p from area D. The class of operators {Ap} = KA will correspond to the set . Shall we rewrite (1) as , (3) where 11 1 11 11 ; ;;, nnex ex uquUR zZRuuu δδδ δ =∈=∈=−≤ is exact right part of (3); u δ 1 – u1ex ≤ δ 1; . is the norm of a vector in Euclidean space Rn. Let us consider now the set of the solutions of the Equation (3) with the fixed operator Ap ∈ KA: . The set is limited if and unlimited if . Any vector z from set is the good mathematical model of process so this vector after action of the op- erator Ap coincides with the given vector q1 with accuracy of measurement . For choice of particular model from set it is necessary to use additional conditions. If such conditions are absent then it is possible to accept as the solution (3) the element for which the equality is carried out [6]: . (4)
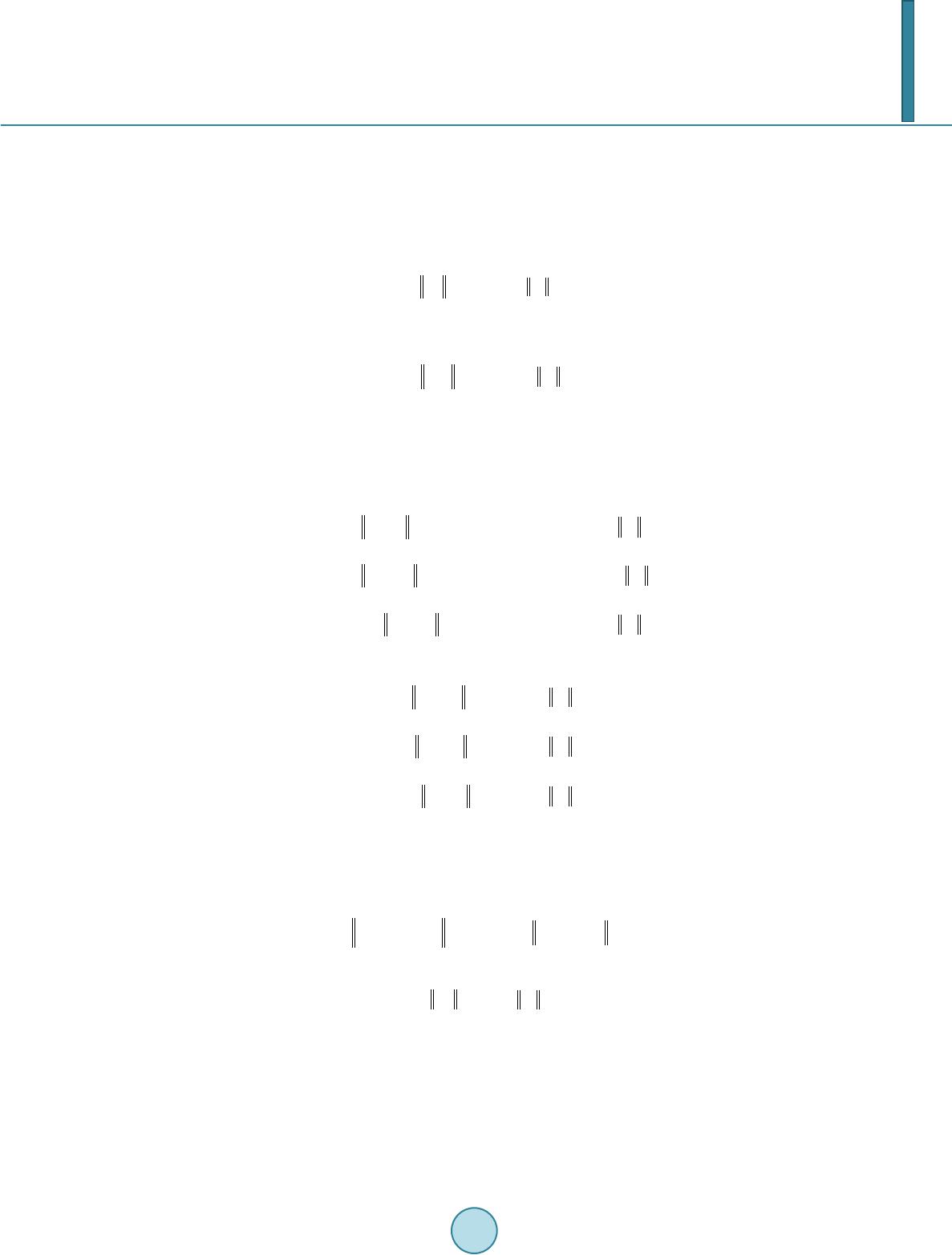 Y. L. Menshikov The vector is possible to interpret as a maximum steady element to the change of the factors not taken into account (most stable part), as the influence of these factors will increase norm of a vector [8]. Such a property of the solution is especially important if one takes into account that the vector further will be used for forecasting real processes (parameter q1). Consider now the set . Let us consider an extreme problem . (5) The vector z*∈Q* is an estimation from below of possible solutions of the Equation (3). The statement of the following extreme problem is possible also: , 1 22 * sup sup inf p zQ pD zz δ ∈ ∈ = (6) The vector has the greatest norm among the solutions of a problem of synthesis on sets Qδ1,p. Models can be used for short-term forecasting of change of variable q1 as on the one hand models are received by a rapid way and on the other hand these models are steadiest to the change of the fac- tors not taken into account. Except (5), (6) it is possible to examine the following statements of problems: 223 311, 1 22 0,0,..,1 infinf ...infsupinf nn p nn qDq DqDzQ qD zz δ −− ∈∈ ∈∈ ∈ = (7) 223 3, 1 11 22 0,0,...,1,1 infinf ...supsupinf p n nnn qDq DzQ qD qD zz δ −− ∈∈ ∈ ∈∈ = (8) , 1 221 1 22 0,1,...,1,1 sup ...supsupinf p n nnn zQ qDqD qD zz δ −− ∈ ∈ ∈∈ = (9) In some cases it is expedient to consider the following problems of identification of parameters: 0,0,...,0 , 1 22 0,0,...,0 inf p zQ zz δ ∈ = , (10) 0,0,...,1 , 1 22 0,0,...,1 inf p zQ zz δ ∈ = , (11) 1,1,...,1 , 1 22 1,1,...,1 inf p zQ zz δ ∈ = , (12) where vector has the minimal possible size of all components of vector p, has the minimal possible size of components and has the maximal size of ; ... ; vector has the maxim- al possible size of all components of vector p. It is possible to consider the following extreme problem 11 1 * 22 inf sup opt apA pl pa pzQ AK A zuAzu δδ δ ∈∈ −= − (13) where za is the solution of extreme problem . (14) Let’s called solution as more plausible mathematical model. Use of such model with the purpose of the forecast allows to receive the characteristic q1 with the least max- imal deviation from experiment with possible variations of variables q2, q3, ... , qn within the given errors. 3. Methods of Solution and Test Calculations For solution of above extreme problem (4) is used Tikhonov regularization method [9]. Extreme problem (4) is
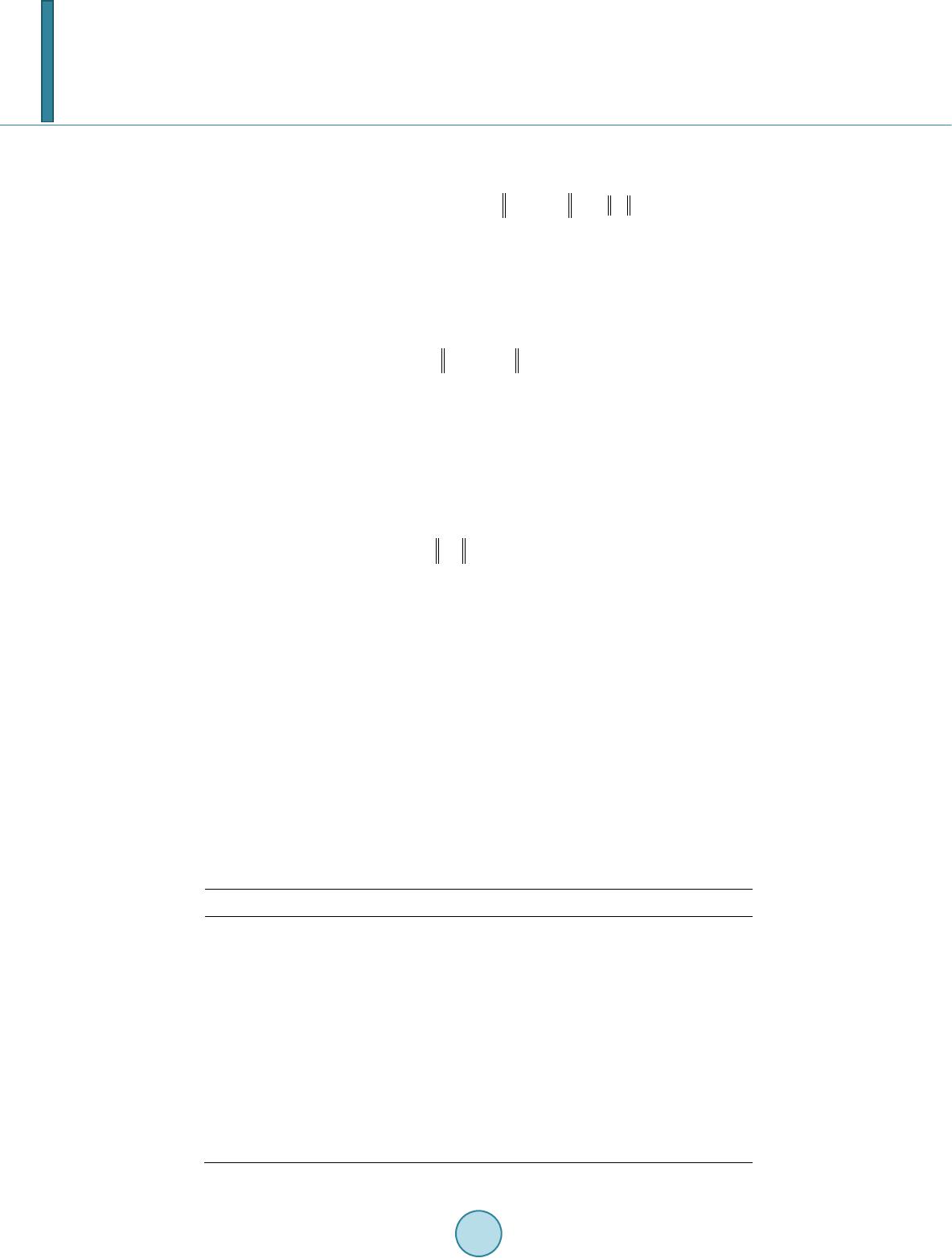 Y. L. Menshikov replaced by the equivalent problem of minimizing the smoothing functional for more efficient use of numerical methods [9]: 11 22 [, ,]. pp MzA uAzuz α δδ α = −+ (15) Euler equation for the functional (5) has the form: (16) where is conjugate operator to . Regularization parameter was obtained by discrepancy method [9]: where is the vector for which the minimum of the functional (16) on the set of possible solutions for a fixed operator . As initial data for the test calculations were selected economic characteristics of development of Ukraine in the period from 1999-2008 (Table 1). In Table 1, the following notations: q1 is debt of state administration (billion UAH), q2 is Ukraine’s GDP at constant prices (billion UAH), q3 is Ukraine’s GDP in current prices (billion UAH), q4 is Inflation, average consumer prices (percent changes), q5 is the unemployment rate (percentage of total manpower), q6 is population (million), q7 is government revenues (billion UAH), q8 is government expenditure (billion UAH). The size of error in the initial data is taken equal to 0.05 . The ultimate goal of parameters identification in this case is the construction of a mathematical model suitable for constructing a stable forecasting the selected stationary process and checking for its adequacy. For this pur- pose, the data are selected from the Table 1 for 1999 to 2006 and is performed the solution of (6). Regulariza- tion parameter is obtained by discrepancy method: [9]. As a result of calculations the mathematical model of the process was obtained: 12 3456 0.080.41 0.320.40.5qq qqqq=+ +−+− (17) To test the adequacy of the results were made retrospective calculations of the size of government debt for 2007 and 2008. In the first case, we used data from a column at 2007 Table 1 (2nd-8th rows), and in the second case, we used the data from column in 2008 Table 1 (2nd-8th rows). Calculations showed the following results: ; Table 1. Economic indicators of Ukraine in the period of 1999-2008. qi 1999 2000 2001 2002 2003 2004 2005 2006 2007 2008 q1 79.6 77.0 74.6 75.7 78.5 85.4 78.2 80.6 88.7 189.4 q2 406.0 429.7 469.1 493.5 540.7 606.1 622.5 668.0 720.7 735.9 q3 130.4 170.1 204.2 225.8 267.3 345.1 441.4 544.1 720.7 948.1 q4 22.78 28.3 12.0 0.74 5.21 9.04 13.6 9.06 12.8 25.2 q5 11.1 11.5 10.8 9.6 9.1 8.6 7.2 6.8 6.3 6.4 q6 49.1 48.7 48.2 47.8 47.4 47.1 46.7 46.5 46.2 46.0 q7 41.6 56.8 68.4 81.3 101.5 128.1 184.6 235.2 301.6 419.7 q8 34.8 62.4 74.6 85.4 103.9 143.3 194.7 242.7 315.9 449.3
 Y. L. Menshikov According Table 1, for 1999 to 2006 the linear mathematical model of the same process by the method of least squares (MLS) was obtained [4]. Then similar calculation of government debt for 2007 and 2008 for Ukraine was made. In this case as in the first case, we used data from the column in 2007 Table 1 (2nd-8th rows), and in the second case, we used data from the column in 2008 Table 1 (2nd -8th rows). The results of calculations by method of MLS showed the following results: ; . Comparison of calculations results showed that suggested method gives more adequate mathematical model of process. Choice of the certain mathematical model is being determined of the specificity of a concrete problem and fi- nal goal of use of mathematical model. However the best mathematical model for the forecast can not be deter- mined a priori [8]. 4. Conclusion The offered approach to a problem of parameters identification of mathematical models of stationary processes allows expanding a class of the possible solutions (linear mathematical models) up to maximal possible. Some variants of statement of a problem of identification of parameters are considered. The calculations of test exam- ples were obtained. References [1] Grop, D. (1979) Methods of Identification of Syste ms. World, Moscow. [2] Seidg, A.P. and Мelsa, Dj.L. (1974) Identification of control systems. Science, Мoscow. [3] Malenvo, E. (1975) Statistics Methods in Econometrics. Statistics, Мoscow. [4] Douguerty, К. (1999) Introduce to Econometric. INFRA-М, М., XIV. [5] Wit, E. and McClure, J. (2004) Statistics for Microarrays: Design, Analysis, and Inference. 5th Edition, John Wiley & Sons Ltd., Chichester. http://dx.doi.org/10.1002/0470011084 [6] Menshikov, Yu.L. (2006) The Fast Identification of Parameters. Proc. 11th Int. Conf. Mathematical Methods in Elec- tromagnetic Theory, MMET’06, Kharkiv, Ukraine, 167-169. [7] Polajk, B.Т. and Nazin, S.А. (2006) Evaluation of Parameters in Linear Many-Dimensional Systems with Interval Un- certainty. Problems of Control and Informatics, Kiev. Ukraine, 1-2, 103-115. [8] Menshikov, Yu.L. and Nakonecnhij, A.G. (2003) Principle of the Maximal Stability in Inverse Problems with a Mini- mum of the a Priori Information. Proc. of Inter. Conf. Problems of Decision making under Uncertainties (PDMU- 2003), Kiev-Alushta, 8-12 Se ptember 2003, 80-82. [9] Tikhonov, A.N. and Arsenin, V.Ya. (1979) Methods of Solutions of Incorrect Problems. Science, Moscow.
|