 J. Biomedical Science and Engineering, 2009, 2, 190-199 Published Online June 2009 in SciRes. http://www.scirp.org/journal/jbise JBiSE Descriptively pr obabilisti c relations hip betwee n muta ted primary structure of von Hippel-Lindau protein and its clinical outcome Shao-Min Yan1, Guang Wu2* 1National Engineering Research Center for Non-food Biorefinery, Guangxi Academy of Sciences, 98 Daling Road, Nanning, Guangxi Province, CN-530007, China; 2Computational Mutation Project, DreamSciTech Consulting, 301, Building 12, Nanyou A-zone, Jianna n Road, Shenzhen, Guangdong Province CN-518054, China; *Corresponding author (hongguanglishibahao@yahoo.com), Tel: +86 771 2503 930, Fax: +86 755 2664 8177. Received 5 August 2008; revised 4 January 2009; accepted 7 January 2009. ABSTRACT In this study, we use the cross-impact analysis to build a descriptively probabilistic relationship between mutant von Hippel-Lindau protein and its clinical outcome after quantifying mutant von Hippel-Lindau proteins with the amino-acid distribution probability, then we use the Bayes- ian equation to determine the probability that the von Hippel-Lindau disease occurs under a mutation, and finally we attempt to distinguish the classifications of clinical outcomes as well as the endocrine and nonendocrine neoplasia induced by mutations of von Hippel-Lindau protein. The results show that a patient has 9/10 chance of being von Hippel-Lindau disease when a new mutation occurs in von Hippel- Lindau protein, the possible distinguishing of classifications of clinical outcomes using mod- eling, and the explanation of the endocrine and nonendocrine neoplasia in modeling v iew. Keywor ds: Amino Acid; Bayes’ Law; Cross-Impact Analysis; Distribution Probability; Mutation; Von Hippel-Lin d au Disease 1. INTRODUCTION Perhaps, the first step to study the genotype-phenotype relationship is to determine a protein in relation to a dis- ease, and the second step would be to build a quantitative relationship between mutant protein and its clinical out- come. Then we ma y be in the position to predict the clini- cal outcome based on such a quantitative relati onship, even to predict new functions led by new mutations. Thus, we need the methods, which can quantify a pro- tein sequence as a numeric sequence in order to build a quantitative relationship. In fact, we have various ways to quantify a protein sequence, for example, to use the physicochemical property of amino acid to quantify a protein sequence [1]. Since 1999, we have developed three approaches to quantify each amino acid in a protein as well as a whole protein (for reviews, see [2,3,4]), and our quantifications indeed differ before and after mutation, thus it is possi- ble to use our approaches to build a quantitative rela- tionship between changed primary structure and changed functio n of protein. In 1911 and 1926, von Hippel and Lindau described the von Hippel-Lindau disease [5,6], later on Melmon and Rosen established the notion of the von Hippel- Lindau disease [7], which is an autosomal dominant dis- order characterized by cerebellar, spinal cord, and retinal hemangioblastomas; cysts of the kidney, pancreas, liver, and epididymis; and has an increased frequency of renal cancer (renal cell carcinoma or hypernephroma), pan- creatic cancer, and pheochromocytoma [8,9,10]. The von Hippel-Lindau disease has a birth incidence of about 1 in 36000 and about 20% of cases arise as de novo muta- tions without a family history [11,12]. The von Hippel -Li ndau disease tum or supp ressor gene was identified in 1993 [13], of which mutations are the major cause for developing the von Hippel-Lindau dis- ease. Pathologically relevant is inactivation of the von Hippel-Lindau gene and subsequent loss of the function of the von Hippel-Lindau protein, and Elongin B, C complex [14,15]. The dysfunction of the ubiquitination of hypoxia-inducible factors is an important step in the development of various tumors [15,16,17,18,19]. Also, a recent study elucidated the role of NGF/JunB/ EglN3- related pathways in developmental apoptosis linking to tumourigenesis [2 0]. Clinically the von Hippel-Lindau disease is classified into two types: type I without pheochromocytoma and type II with pheochromocytoma [10,17]. On the other hand, more than 300 different von Hippel-Lindau muta-
 S. M. Yan et al. / J. Biomedical Science and Engineering 2 (2009) 190-199 191 SciRes Copyright © 2009 JBiSE tions have been described at DNA level [21,22,23,24], and more than 100 at protein level. It would be great helpful if we can build a quantitative relationship be- tween von Hippel-Lindau protein mutation and von Hippel-Lindau disease status, that is, the relationship between mutant protein and its clinical outcome. In this study, we build a descriptively quantitative rela- tionship between changed primary structure of mutated von Hippel-Lindau protein and the classification of its clinical outcome, distinguish the classifications of clinical outcomes as well as the endocrine and nonendocrine neo- plasia induced by mutations of von Hippel-Lindau protein. 2. MATERIALS AND METHODS 2.1. Data The human von Hippel-Lindau disease tumor suppr essor with total 132 mutations (accession number P40337; December 4, 2007; Entry version 91) is obtained from UniProtKB/Swiss-Prot entry [25]. Among them, 123 are missense point mutations , 7 deletions and 3 insertions. 2.2. Amino-Acid Distribution Probability Among three approaches developed by us, the amino-acid distribution probability is mainly related to the positions of amino acids along the protein, which is suitable for mutation analysis, and we have used this approach in a number of our previous studies [2,3,4,26,27,28,29,30,31,32,33,34,35,36,37, 38,39,40,41,42,43,44]. The quantification is developed along such a thought, for example, how do two amino acids dis- tribute along a protein sequence? Our intuition may suggest that there would be one amino acid in the first half of the sequence and anothe r one in the second half. In fact, there are only three possible distributions, 1) both amino acids are in the first half, 2) one amino acid is in each half and 3) both amino acids are in the second half. Thus, each distribution has the probability of 1 /3. If we do not distinguish either the first half or second half but are simply interested in whether both amino acids are in both halves or in any half, there will be the probability of 1/2 for each distribution. If we are interested in the distribution probability of three amino acids in a protein, we naturally imagine to grouping the protein into three partitions, and our intuition may suggest that each partition contains an amino acid. If we do not distinguish the first, second and third partition, actually there are to tally three types of dis tributions , i.e. 1) each amino acid is in each partition, 2) two amino acids are in a partition and an amino acid is in another partition, and 3) three amino aci ds are in a partition. In this situation, the distribution probability can be calculated according to the statistical mechanics, which classifies the distribution of elementary particles in en- ergy states according to three assumptions of whether distinguishing each particle and energy state, i.e. Max- well-Boltzmann, Fermi-Dirac and Bose-Einstein as- sumptions [45]. We actually use the Maxwell-Boltzmann assumption for computing amino-acid distribution probability, which is equal to !...!! ! 10 n qqq r r n n rrr r !...!! ! 21 [45], where r is the number of amino acids, n is the number of partitions, rn is the number of amino acids in the n-th partition, qn is the number of partitions with the same number of amino acids, and ! is the factorial function. Thus, the distribution probabilities are different for these three types of distributions of three amino acids, say, 0.2222 for 1), 0.6667 for 2) and 0.1111 for 3). Clearly the protein can only adopt one type of distribu- tion for these three amino acids, which is the actual dis- tribution probability. For four amino acids, there are five distributions, 1) each partition contains an amino acid, 2) a partition contains two amino acids and two partitions contain an amino acid each, 3) two partitions contain two amino acids each, 4) a partition contains an amino acid and a partition contains three amino acids, and 5) a partition contains four amino acids. Their distribution probabilities are 0.0938 for 1), 0.5625 for 2), 0.1406 for 3), 0.1875 for 4), and 0.0156 for 5). Furthermore, there are seven distributions for five amino acids, 11 distributions for six amino acids, 15 dis- tributions for seven amino acids, and so on. 2.3. Quantification of Wild-Type von Hippel- Lindau Protein Table 1. Amino acids, their composition and distribution prob- ability in wild-type human von Hippel-Lindau protein. (A, alanine; R, arginine; N, asparagine; D, aspartic acid; C, cys- teine; E, glutamic acid; Q, glutamine; G, glycine; H, histidine; I, isoleucine; L, leucine; K, lysine; M, methionine; F, phenyla- lanine; P, proline; S, serine; T, threonine; W, tryptophan; Y, tyrosine; V, valine.) Amino acid Number Distribution probability A 10 0.0476 R 20 0.0067 N 9 0.1770 D 11 0.1077 C 2 0.5000 E 30 0.0001 Q 8 0.0673 G 18 0.0389 H 5 0.0640 I 6 0.1543 L 20 0.0422 K 3 0.1111 M 3 0.6667 F 5 0.2880 P 19 0.0319 S 11 0.0404 T 7 0.2142 W 3 0.6667 Y 6 0.2315 V 17 0.1280
 192 S. M. Yan et al. / J. Biomedical Science and Engineering 2 (2009) 190-199 SciRes Copyright © 2009 With respect to the wild-type von Hippel-Lindau protein, for example, there are eight glutamines “Q” in von Hip- pel-Lindau protein (Table 1). We may ask how these eight Qs distribute along the von Hippel-Lindau protein? According to the problem of the occupancy of subpopu- lations and partition s [45], the simple way to answer this question is to imagine that we would divide the von Hip- pel-Lindau protein into eight equal partitions, and each partition has about 27 amino acids (213/8=26.625) be- cause the von Hippel-Lindau protein is composed of 213 amino acids, then there would be 22 configurations for all the possible distributions of eight Qs (Table 2). Here, we calculate two distribution probabilities in Ta- ble 2 as example according to the above equation. For eight Qs equally distribute in each partition (the second row in Table 2), we have q0=0, q1=8, . . . q8=0; and r1=1, r2=1, . . . r8=1. Thus, we have the distribution probability, 0.0024 16777216 1 11111111 40320 1111111403201 40320 8 !1!1!1!1!1!1!1!1 !8 !0!0!0!0!0!0!0!8!0 !8 8 Clearly, the von Hippel-Lindau protein can adopt only one distribution pattern, which is that two partitions contain zero Q, five partitions contain one Q and one partition contains three Qs (the fourth row in Tab le 2 ). So we have q0=2, q1=5, q2=0, q3=1, q4=0, q5=0, q6=0, q7=0, q8=0; and r1=0, r2=0, r3=1, r4=1, r5=1, r6=1, r7=1, r8=3, that is, 0.0673 16777216 1 61111111 40320 11111111202 40320 8 !3!1!1!1!1!1!0!0 !8 !0!0!0!0!0!0!1!0!5!2 !8 8 In such a manner, we can quantify each amino acid in wild-type von Hippel-Lindau protein. Thereafter, we can assign these probabilities to each amino acid in the von Hippel-Lindau protein as shown in Figure 1, from which we get the visual sense of how these distribution prob- abilities go along the von Hippel-Lindau protein, and more importantly we can sum up these distribution prob- abilities together for al l 213 amino acids in t he pr ot ei n. us a way to estimate the position of am ino acid in a protein, because there is a standard method for the computation using Maxwell-Bolzmann assumption, which saves us from inventing new computational methods. Moreover, the primary structure is the base for higher - le vel structure, t hus any mutation in primary structure would lead to the change in distribution probability, in higher-level structure, and finally the biological function. This is the biological mean- ing of use of Maxwell- Bolzmann assumption for quantify- Actually, the Maxwell-Bolzmann assumption provides Table 2. All possible distributions of eight glutamines in von Hippel-Lindau protein. (Bold and italic is the real distribution.) Partition 1 Partition 2 Partition 3 Partition 4 Partition 5 Partition 6 Partition 7 Partition 8 Probability 1 1 1 1 1 1 1 1 0.002403 1 1 1 1 1 1 2 0.0673 1 1 1 1 1 3 0.0673 1 1 1 1 4 0.0280 1 1 1 5 5.6076e-3 1 1 6 5.6076e-4 1 7 2.6703e-5 8 4.7684e-7 1 1 1 1 2 2 0.2523 1 1 1 2 3 0.2243 1 1 2 4 0.0421 1 2 5 3.3646e-3 2 6 9.3460e-5 1 1 2 2 2 0.1682 1 2 2 3 0.0841 2 2 4 4.2057e-3 2 2 2 2 0.0105 1 1 3 3 0.0280 2 3 3 5.6076e-3 1 3 4 5.6076e-3 4 4 1.1683e-4 3 5 1.8692e-4 JBiSE
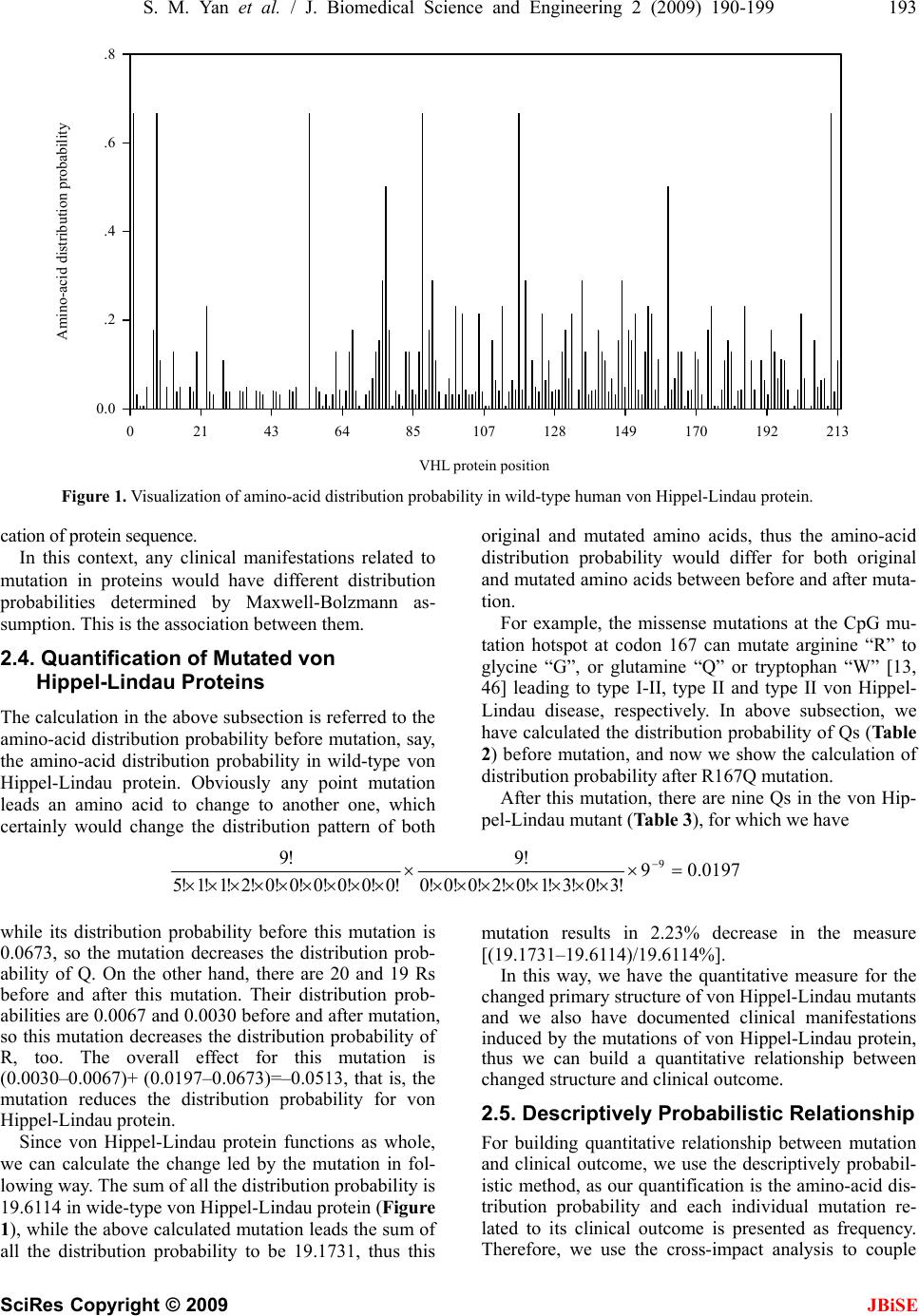 S. M. Yan et al. / J. Biomedical Science and Engineering 2 (2009) 190-199 193 SciRes Copyright © 2009 JBiSE VHL protein position 021436485107 128 149 170 192 213 Amino-acid distribution probability 0.0 .2 .4 .6 .8 VHL protein position Amino-acid distribution probability Figure 1. Visualization of amino-acid distribution probability in wild-type human von Hippel-Lindau protein. cation of protein sequence. In this context, any clinical manifestations related to mutation in proteins would have different distribution probabilities determined by Maxwell-Bolzmann as- sumption. This is the association between them. 2.4. Quantification of Mutated von Hippel-Lindau Proteins The calculation in the abov e subsection is referred to the amino-acid distribution probability before mutation, say, the amino-acid distribution probability in wild-type von Hippel-Lindau protein. Obviously any point mutation leads an amino acid to change to another one, which certainly would change the distribution pattern of both original and mutated amino acids, thus the amino-acid distribution probability would differ for both original and mutated amino acids between before and after muta- tion. For example, the missense mutations at the CpG mu- tation hotspot at codon 167 can mutate arginine “R” to glycine “G”, or glutamine “Q” or tryptophan “W” [13, 46] leading to type I-II, type II and type II von Hippel- Lindau disease, respectively. In above subsection, we have calculated the distribution probab ility of Qs (Table 2) before mutation, and now we show the calculation of distribution probability after R167Q mutation. After this mutation, there are nine Qs in the von Hip- pel-Lindau mutant (Table 3), for which we hav e 0.01979 !3!0!3!1!0!2!0!0!0 !9 !0!0!0!0!0!0!2!1!1!5 !9 9 while its distribution probability before this mutation is 0.0673, so the mutation decreases the distribution prob- ability of Q. On the other hand, there are 20 and 19 Rs before and after this mutation. Their distribution prob- abilities are 0.0067 and 0.0030 b efore an d after mutation, so this mutation decreases the distribution probability of R, too. The overall effect for this mutation is (0.0030–0.0067)+ (0.0197–0.0673)=–0.0513, that is, the mutation reduces the distribution probability for von Hippel-Lindau protein. Since von Hippel-Lindau protein functions as whole, we can calculate the change led by the mutation in fol- lowing way. The su m of all th e d istribution probab ility is 19.6114 in wide-type von Hippel-Lindau protein (Figure 1), while the above calculated mutation leads the sum of mutation results in 2.23% decrease in the measure [(19.1731–19.6114)/19.6114%]. In this way, we have the quan all the distribution probability to be 19.1731, thus this titative measure for the ch elationship anged primary structure o f von Hippel-Li ndau mutant s and we also have documented clinical manifestations induced by the mutations of von Hippel-Lindau protein, thus we can build a quantitative relationship between changed structure and clinical outcome. 2.5. Descriptively Probabilistic R For building quantitative relationship between mutation and clinical outcome, we use the descriptively probabil- istic method, as our quantification is the amino-acid dis- tribution probability and each individual mutation re- lated to its clinical outcome is presented as frequency. Therefore, we use the cross-impact analysis to couple
 194 S. M. Yan et al. / J. Biomedical Science and Engineering 2 (2009) 190-199 SciRes Copyright © 2009 JBiSE Partition I II III IV V VI VII VIIIIX Table 3. Distribution pattern of glutamines before and after mutation at position 167 in von Hippel-Lindau protein. Befor e mutation0 0 1 11 1 1 3 - After mutation 0 0 0 20 1 3 0 3 em [35,47,48,49,50,51,52,53], because the amino-acid tical n is based on permutation, and can be nted as mean±SD for normal distribu- CUSSION obability in s on the re- la th distribution probability either increases or decreases af- ter mutation, which is a 2-possibilty event, and the clinical outcome either occurs or does not occur after mutation, which is a yes-and-no event. Thereafter, we can use the Bayesian equation to calculate the probabil- ity of occurrence of clinical outcome under a mutation. 2.6. Classification of Clinical Outcomes It is extremely challenging how to use a mathema modeling to distinguish the clinical outcomes with re- spect to mutant von Hippel-Lindau protein because of the variety of clinical outcomes. In an effort towards solving this problem, we employ our second quantifica- tion, amino-acid pair pred ictability, whose relational an d applications have been published intensively (for re- views, see [2,3,4]). This quantificatio calculated in the following way. For example, there are 30 glutamic acids “E” and 20 Rs in von Hippel- Lindau protein, the predicted frequency of amino-acid pair ER would be 3 (30/21320/212212=2.817), while we do find three ERs in the protein, so the amino- acid pair ER is predictable. Still, the predicted frequency of EE would be 4 (30/21329/212212=4.085), but actually the EE appears nine times in reality. This is the case that the actual frequenc y is larger than its predicte d one. In this manner, we can quantify a protein sequence according to the percentage of how many amino-acid pairs are predict- able among all the amino-acid pairs in given protein as well as its mutants. For instance, the predictable portion of amino-acid pairs is 27.54% in wild-type von Hip- pel-Lindau protein and 31.88% in its P25L mutant. 2.7. Statistics The data are prese tion or median with interquatile range for non-normal distribution. The Kruskal-Wallis one-way ANOVA and Chi-square are used for statistical inference, and P < 0.05 is considered significant. 3. RESULTS AND DIS After computing amino-acid distribution pr wild-type von Hippel-Lindau protein and in its 132 mu- tants, we have 132 changed amino-acid distribution probabilities. Firstly, we can use the cross-impact analy- sis to build a quantitative relationship between the in- crease/decrease of distribution probability after muta- tions and the clinical diag nosis, because the cross-impact analysis is particularly suited for two relevant events coupled together [35 ,47,48,49,50,51,52,53]. Figure 2 displays the cross-impact analysi tionship between changed primary structure and von Hippel-Lindau disease. At the level of amino-acid dis- tribution probability, P(2) and Pare the decreased and increased probabilities ind ucy mutations, and 53 and 79 mutations result in the distribution probability decreased and increased, respectively. At the level of clinical diagnosis: 1) 2 ed b 2|1P is the impact probability (conditional probabilitt the von Hippel-Lindau disease is diagnosed under the condition of increased distribution probability, and 70 mutations have such an effect. 2) y) tha 2|1P is the impact probability that other disease is sed under the condition of increased distribution probability, and 9 mutations work in such a manner. 3) P(1|2) is the impact probability that the von Hippel-Lindau disease is diagnosed under the condition of decreased distribution probability, and 44 mutations play such a role. 4) diagno 2|1P is the impact probability that other disease is ded under the condition of decreased distribution probability, and 9 mutations fall into this category. At the level of combined events, we can see the combined results of changed structure and von Hippel-Lindau disease. Ta bl e 4 lists the calculate iagnos d probabilities with respect to Figure 2, from which several interesting points can be drawn. 1) As 2P is larger than P(2), a mutation has a larger chance of ireasing the distribution probability in von Hippel-Lindau mutant. 2) As nc P much lar- ger than 2|1 is 2|1P, a mutation that ins the distribu- tion probabas about n ine ten th chan ce o f b eing vo n Hippel-Lindau disease. 3) As P(1|2) is much larger than crease ility h 2|1P, a mutation that decreases the distribution lity has much larger chance of being von Hippel- Lindau disease. probabi able 4. Computed probabilities in reference to the cross-im-T pact analysis in Figure 2. P(2)=53/132=0.4015 2P=1–P(2)=1–0.4015=0.5985=79/132 2=70/79=0.8861 |1P 2|1P=1– 2|1P=1–0.8861=0.1139=9/79 P(1|2)==0.83044/532 2|1P=1–P(1|2)=1–0.8302=0.1698=9/53 21P= 2|1P× 2P=70/79×79/132=0.5303=70/132 21P= 2|1P× 2P P(12)= |2)×P=44 =9/79×79/132=0.0682=9/132 P(1 (2)/53×53/132=0.3333=44/132 21P= 2|1P×P(2)=9/53×53/132=0.0682=9/132
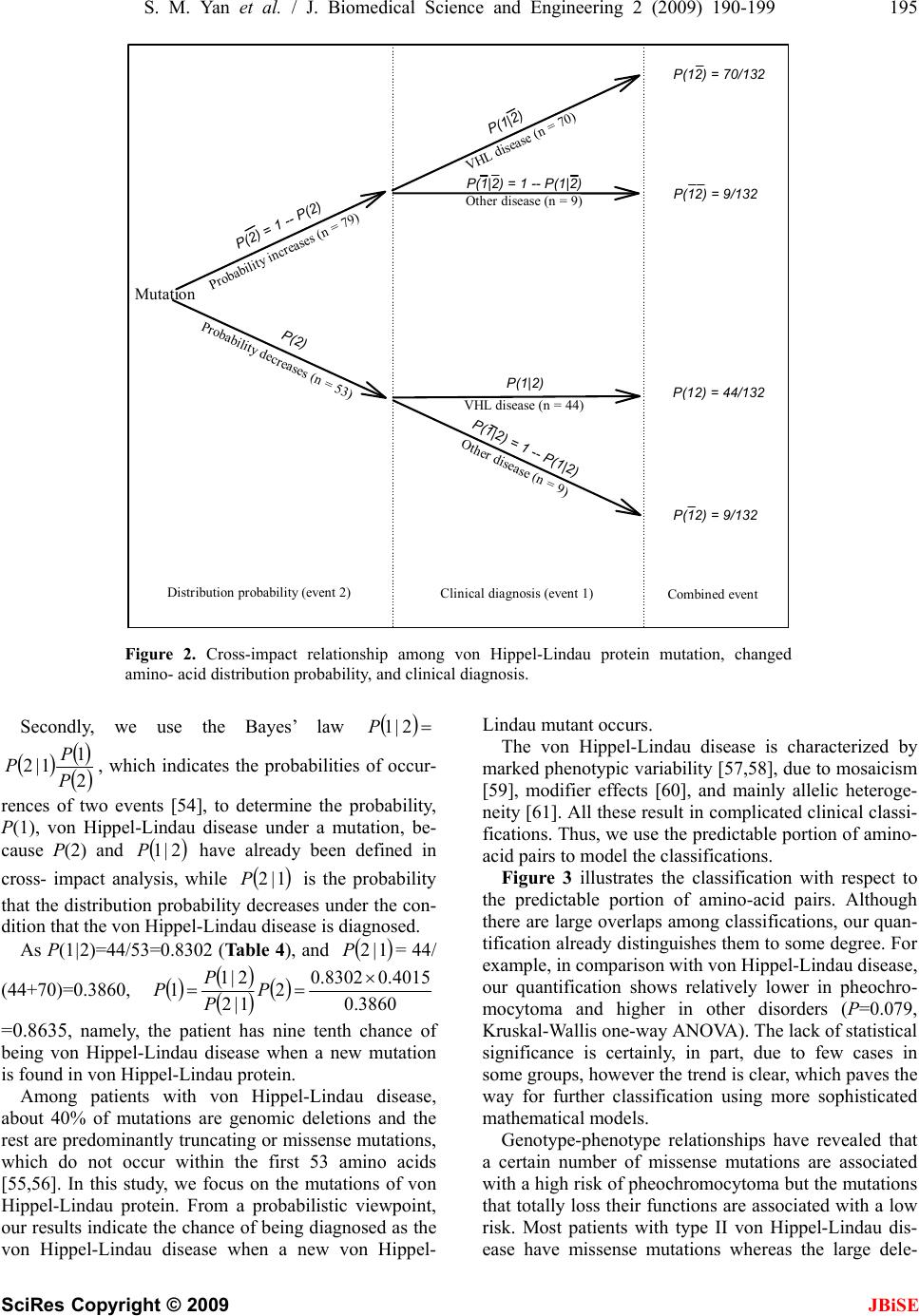 S. M. Yan et al. / J. Biomedical Science and Engineering 2 (2009) 190-199 195 SciRes Copyright © 2009 Mutation Probability increases (n = 79) Probability decreases (n = 53) P(2) P(2) = 1 -- P(2) Di s t ribut ion probabil it y (eve nt 2)Clinical diagnosis (event 1)Combined event Other d i sea se ( n = 9 ) VHL disease (n = 44) VHL disease (n = 70) Other diseas P(12) = 70/132 JBiSE e (n = 9) P(12) = 9/132 P(1|2) P(1|2) P(1|2) = 1 -- P(1|2) P(1|2) = 1 -- P(1| P(12) = 44/132 P(12) = 9/132 2) Figure 2. Cross-impact relationship among von Hippel-Lindau protein mutation, changed amino- acid distribution probability, and clinical diagnosis. Secondly, wse the Bayes’ law e u 2|1P 2 1 1|2 P P P, whindicates the probabilities o nces of two ev [54], to determine the p (1), von Hippelndau disease under a mu ause P(2) and have already been ross- imwhile is the p at the distributrobability con- ition that the voippel-Lindau disease is diagnosed. As P(1|2)=44/0.8302 (Table 4), and = 44/ 4+70)=0.3860, ich ents -Li 1P ion p n H 53= f occur- robability, tation, be- defined in robability re P c2| pact analysis, 1|2P c th decreases under the d 1|2P 3860.0 .08302 4015.0 2 1|2 2|1 1 (4 P P P 0.8635, namely, the patient has nine tenth chance of eing von Hippel-Lindau disease when a new mutation found in von Hippel-Lindau protein. Among patients with von Hippel-Lindau disease, bout 40% of mutations are genomic deletions and the st are predominantly truncating or missense mutations, hich do not occur within the first 53 amino acids 5,56]. In this study, we focus on the mutations of von ippel-Lindau protein. From a probabilistic viewpo ur results indicate the chance of being diagnos von Hippel-Lindau disease when a new von Hippel- Lindau mutant occurs. The von Hippel-Lindau disease is characterized by marked phenotypic variability [5 7,58], due to mosaicism [59], modifier effects [60], and mainly allelic heteroge- neity [61]. All these result in complicated clinical classi- fications. Thus, we use the predictable portion of amino- acid pairs to model the classifications. Figure 3 illustrates the classification with respect to the predictable portion of amino-acid pairs. Although there are large overlaps among classifications, our quan- tification already disting uishes them to some degr ee. For example, in comparison with von Hippel - Lindau disease, our quantification shows relatively lower in pheochro- mocytoma and higher in other disorders (P=0.079, Kruskal-Wallis one-w ay ANOVA). The lack of statistical significance is certainly, in part, due to few cases in some groups, however the trend is clear, which paves the way for further classification using more sophisticated mathematical models. Genotype-phenotype relationships have revealed that a certain number of missense mutations are associated with a high risk of pheochromocyto ma but the mutatio ns eir functions are associated with a low ts with type II von Hippel-Lindau dis- ease have missense mutations whereas the large dele- P = b is a re w [5int, ed as the that totally loss th risk. Most patien H o
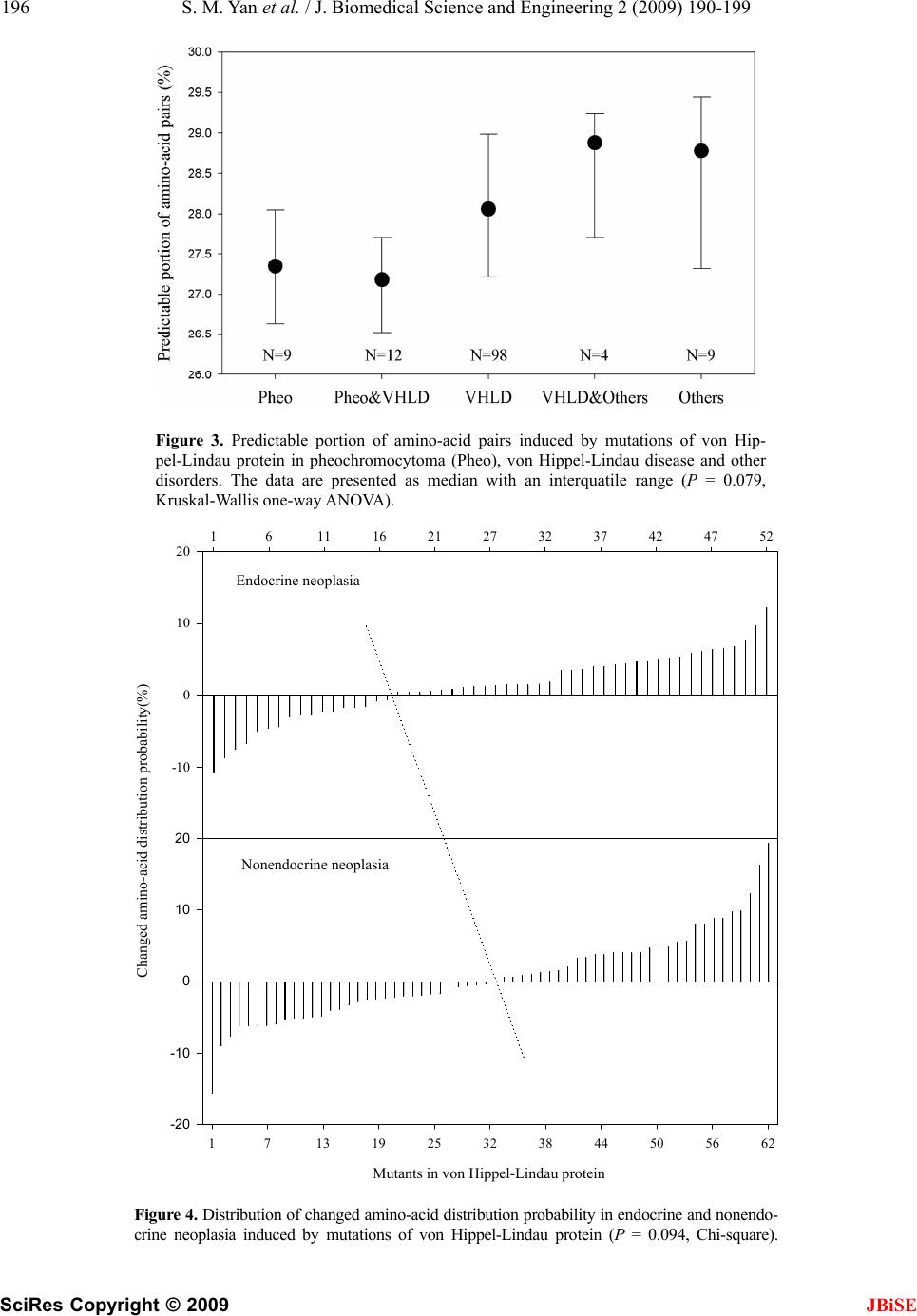 196 S. M. Yan et al. / J. Biomedical Science and Engineering 2 (2009) 190-199 SciRes Copyright © 2009 JBiSE pairs induced by mutations of von Hip- eo), von Hippel-Lindau disease and other with an interquatile range (P = 0.079, Figure 3. Predictable portion of amino-a pel-Lindau protein in pheochromocytoma disorders. The data are presented as med Kruskal-Wallis one-way ANOVA). cid (Ph ian nendo- 161116 21 27 32 3742 47 52 Figure 4. Distribution of changed amino-acid d crine neoplasia induced by mutations of von istribution probability in endocrine and no Hippel-Lindau protein (P = 0.094, Chi-square). Changed amino-acid distribution probability (%) -10 0 10 20 Endocrine neoplasia Endocrine neoplasia Mutants in von Hippel-Linda u protein 1713 19 25 32 38 44 50 56 62 Changed amino-acid distribution probability(%) 10 20 Nonendocrine neoplasia Nonendocrine neopla sia -20 -10 0 Mutants in von Hippel-Lindau protein
 S. M. Yan et al. / J. Biomedical Science and Engineering 2 (2009) 190-199 197 SciRes Copyright © 2009 JBiSE ype I ribution probability (upper panel), h might provide the much clearer pattern, l viewpoint, one could consider t is different. Without suc t a theoretical study finds ical assays. Our ap- tions and truncating mutations predominate in t families [11,19,62,63]. Many missense mutations caus- ing a type I phenotype are involved in the core hydro- phobic residues and were predicted to disrupt protein structure, whereas type II phenotype missense mutations nvolved in substitutiare ions at a surface amino acid that does not cause a total loss of function [64,65]. Figure 4 displays the distribution of changed amino- acid distribution probability in endocrine neoplasia (pheochromocytoma, type II von Hippel-Lindau disease) and nonendocrine neoplasia (type I von Hippel-Lindau disease). As can be seen, the mutations that led to the endocrine neoplasia have the trend to increase the amino-acid dist whereas the mutations that led to nonendocrine neopla- sia have the effect to either increase or decrease the amino-acid distribution probability (lower panel). The difference between two panels is mainly considered from view of symmetry. As the x-axis is related to the number of von Hippel-Lindau mutations, this figure would be different when more mutations would be found in future, whic although we did not find the statistical difference be- tween two panels (P=0.094, Chi-square) now. From a theoreticao No. calculate the distribution probability of all 19 potential types of mutations at each position of von Hippel-Lindau protein, and then find the link between mutations and clinical outcomes. However, the amount of computation is huge because it would be equal to 2.36910272 muta- tions (19213), which is not only beyond the capacity of any computers, but also beyond the capacity for com- parison. Actually, we really know that each position does not have 19 types of potential mutations, because this mutation process is gov erned by the tran slation probab il- ity between RNA codon and mutated amino acids [66, 67,68]. On the other hand, our study is focused on the documented data rather than the simulated data. In this study, we use a single valu e, the sum of all dis- tribution probability to represent the normal von Hippel- Lindau protein and its mutated proteins, respectively, because there is no other way to use a single value dy- namically to represent a protein, namely, the value is different when a proteinh a measure, we cannot model a protein dynamically with its mutations. To the best of knowledge, currently it is only the accession number that can represent a protein uniquely, however it has nothing to do with the protein itself, i.e. composition, length, function, etc. In general, one would hope to verify this type of study against the real-life cases, which is possible in future although it would deal with a large-scale collaboration because this type of diseases is not frequently seen in clinical settings, for example, the von Hippel-Lindau disease has a birth incid ence of abou t 1 in 36 000 [11,12]. It will take years to verify wha with fast-speed computational technique. Even, we can- not verify all the theoretical studies, for example, we cannot create another earth without global warming. The implications of this study include two aspects. 1) relationship between changed primary structTheure and changed function is very meaningful, because it provides the dynamic rather than static relationship between mu- tant protein and its function. This can furthermore pro- vide us the basis for building a dynamic model to predict the new function in mutant proteins. Nevertheless, we need to quantify the proteins in order to build a dynamic model and this study is doing in su ch a way. 2) Fro m the clinical viewpoint, the classification of von Hippel- Lindau disease as well as many mutation related diseases needs a considerable amount of clin proach can provide a probabilistic estimate for disease classification after determining which amino acid has mutated, because the primary structure of protein is the base for its high-level structure and function. 4. ACKNOWLEDGEMENTS This study was partly supported by Guangxi Science Foundation (No. 0537012-G and 0991080), and Guangxi Academy of Sciences (project 9YJ17SW07). 0 REFERENCES [1] K. C. Chou, (2004) Structure bioinformatics and its im- pact to biomedical science, Curr. Med. Chem, 11, 2105-2134. [2] G. Wu and S. Yan, (2002) Randomness in the primary structure of protein: Methods and implications, Mol. Biol. Today, 3, 55-69. [3] G. Wu and S. Yan, (2006) Mutation trend of hemaggluti- nin of influenza A virus: A review from computational mutation viewpoint, Acta Pharmacol. Sin., 27, 513-526. [4] G. Wu and S. Yan, (2008) Lecture notes on computational mutation, Nova Science Publishers, New York, 2008. [5] Von Hippel, (1911) Die anatomische Grund lage der von mir beschriebenen ‘sehr seltenen Erkrankung der Netz- haut’, Graefes. Arch. Ophthalmol., 79, 350-377. [6] A. Lindau, (1926) Studien uber kleinhirncysten, bau, pathogenese und bezoejimgem zur angiomatosis retinae, Acta Pathol. Microbiol. Scand., Suppl 1, 1-128. [7] K. L. Melmon and S. W. Rosen, (1964) Lindau’s disease, Am. J. Med., 36, 595-617. [8] V. V. Michels, (1988) Investigative studies in von Hip- pel-Lindau disease, Neurofibromatosis, 1, 159-163. [9] H. P. Neumann, (1987) Basic criteria for clinical diagno- sis and genetic counselling in von Hippel-Lindau syn- drome, Vasa, 16, 220-226. [10] R. R. Lonser, G. M. Glenn, M. Walther, E. Y. Chew, S. K. Libutti, W. M. Linehan, and E. H. Oldfield, (2003) von Hippel-Lindau disease, Lancet, 361, 2059-2067. [11] E. R. Maher, A. R. Webster, F. M. Richards, J. S. Green, P. A. Crossey, S. J. Payne, and A. T. Moore, (1996) Phe- notypic expression in von Hippel-Lindau disease: Corre-
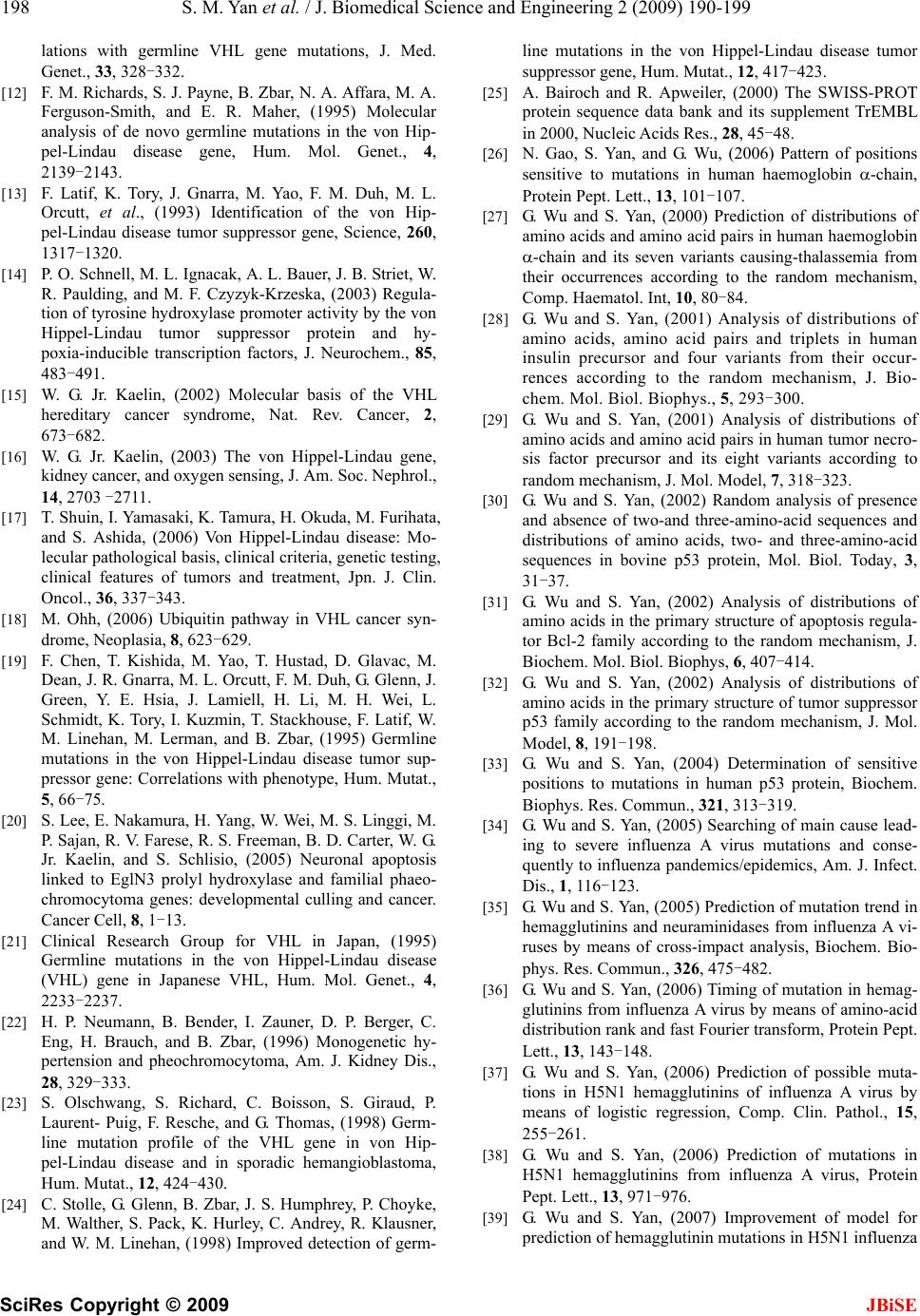 198 S. M. Yan et al. / J. Biomedical Science and Engineering 2 (2009) 190-199 SciRes Copyright © 2009 JBiSE Mol. Genet., 4, . ity by the von Kaelin, (2002) Molecular basis of the VHL er, and oxygen sensing, J. Am. Soc. Nephrol., gical basis, clinical criteria, genetic testing, . Tory, I. Kuzmin, T. Stackhouse, F. Latif, W. lations with phenotype, Hum. Mutat., mas, (1998) Germ- von Hip- von Hippel-Lindau disease tumor suppressor gene, Hum. Mutat., 12, 417-423. 5] A. Bairoch and R. Apweiler, (2000) The SWISS-PROT protein sequence data bank and its supplement TrEMBL cid pairs in human haemoglobin alysis of presence Analysis of distributions of -198. lations with germline VHL gene mutations, J. Med. Genet., 33, 328-332. [12] F. M. Richards, S. J. Payne, B. Zbar, N. A. Affara, M. A. Ferguson-Smith, and E. R. Maher, (1995) Molecular analysis of de novo germline mutations in the von Hip- pel-Lindau disease gene, Hum. 2139-2143. [13] F. Latif, K. Tory, J. Gnarra, M. Yao, F. M. Duh, M. L. Orcutt, et al., (1993) Identification of the von Hip- pel-Lindau disease tumor suppressor gene, Science, 260, 1317-1320 [14] P. O. Schnell, M. L. Ignacak, A. L. Bauer, J. B. Striet, W. R. Paulding, and M. F. Czyzyk-Krzeska, (2003) Regula- tion of tyrosine hydroxylase promoter activ [28] Hippel-Lindau tumor suppressor protein and hy- poxia-inducible transcription factors, J. Neurochem., 85, 483-491. [15] W. G. Jr. hereditary cancer syndrome, Nat. Rev. Cancer, 2, 673-682. [16] W. G. Jr. Kaelin, (2003) The von Hippel-Lindau gene, kidney canc 14, 2703 -2711. [17] T. Shuin, I. Yamasaki, K. Tamura, H. Okuda, M. Furihata, and S. Ashida, (2006) Von Hippel-Lindau disease: Mo- lecular patholo and a clinical features of tumors and treatment, Jpn. J. Clin. Oncol., 36, 337-343. [18] M. Ohh, (2006) Ubiquitin pathway in VHL cancer syn- drome, Neoplasia, 8, 623-629. [19] F. Chen, T. Kishida, M. Yao, T. Hustad, D. Glavac, M. Dean, J. R. Gnarra, M. L. Orcutt, F. M. Duh, G. Glenn, J. Green, Y. E. Hsia, J. Lamiell, H. Li, M. H. Wei, L. Schmidt, K M. Linehan, M. Lerman, and B. Zbar, (1995) Germline mutations in the von Hippel-Lindau disease tumor sup- pressor gene: Corre 5, 66-75. [20] S. Lee, E. Nakamura, H. Yang, W. Wei, M. S. Linggi, M. P. Sajan, R. V. Farese, R. S. Freeman, B. D. Carter, W. G. Jr. Kaelin, and S. Schlisio, (2005) Neuronal apoptosis linked to EglN3 prolyl hydroxylase and familial phaeo- chromocytoma genes: developmental culling and cancer. Cancer Cell, 8, 1-13. [21] Clinical Research Group for VHL in Japan, (1995) Germline mutations in the von Hippel-Lindau disease (VHL) gene in Japanese VHL, Hum. Mol. Genet., 4, 2233-2237. [22] H. P. Neumann, B. Bender, I. Zauner, D. P. Berger, C. Eng, H. Brauch, and B. Zbar, (1996) Monogenetic hy- pertension and pheochromocytoma, Am. J. Kidney Dis., 28, 329-333. [23] S. Olschwang, S. Richard, C. Boisson, S. Giraud, P. Laurent- Puig, F. Resche, and G. Tho line mutation profile of the VHL gene in pel-Lindau disease and in sporadic hemangioblastoma, Hum. Mutat., 12, 424-430. [24] C. Stolle, G. Glenn, B. Zbar, J. S. Humphrey, P. Choyke, M. Walther, S. Pack, K. Hurley, C. Andrey, R. Klausner, and W. M. Linehan, (1998) Improved detection of germ- line mutations in the [2 in 2000, Nucleic Acids Res., 28, 45-48. [26] N. Gao, S. Yan, and G. Wu, (2006) Pattern of positions sensitive to mutations in human haemoglobin -chain, Protein Pept. Lett., 13, 101-107. [27] G. Wu and S. Yan, (2000) Prediction of distributions of amino acids and amino a -chain and its seven variants causing-thalassemia from their occurrences according to the random mechanism, Comp. Haematol. Int, 10, 80-84. G. Wu and S. Yan, (2001) Analysis of distributions of amino acids, amino acid pairs and triplets in human insulin precursor and four variants from their occur- rences according to the random mechanism, J. Bio- chem. Mol. Biol. Biophys., 5, 293-300. [29] G. Wu and S. Yan, (2001) Analysis of distributions of amino acids and amino acid pairs in human tumor necro- sis factor precursor and its eight variants according to random mechanism, J. Mol. Model, 7, 318-323. [30] G. Wu and S. Yan, (2002) Random an bsence of two-and three-amino-acid sequences and distributions of amino acids, two- and three-amino-acid sequences in bovine p53 protein, Mol. Biol. Today, 3, 31-37. [31] G. Wu and S. Yan, (2002) amino acids in the primary structure of apoptosis regula- tor Bcl-2 family according to the random mechanism, J. Biochem. Mol. Biol. Biophys, 6, 407-414. [32] G. Wu and S. Yan, (2002) Analysis of distributions of amino acids in the primary structure of tumor suppressor p53 family according to the random mechanism, J. Mol. Model, 8, 191 [33] G. Wu and S. Yan, (2004) Determination of sensitive positions to mutations in human p53 protein, Biochem. Biophys. Res. Commun., 321, 313-319. [34] G. Wu and S. Yan, (2005) Searching of main cause lead- ing to severe influenza A virus mutations and conse- quently to influenza pandemics/epidemics, Am. J. Infect. Dis., 1, 116-123. [35] G. Wu and S. Yan, (2005) Prediction of mutation trend in hemagglutinins and neuraminidases from influenza A vi- ruses by means of cross-impact analysis, Biochem. Bio- phys. Res. Commun., 326, 475-482. G. Wu and S. Yan, (2006) Timing [36] of mutation in hemag- glutinins from influenza A virus by means of amino-acid distribution rank and fast Fourier transform, Protein Pept. Lett., 13, 143-148. [37] G. Wu and S. Yan, (2006) Prediction of possible muta- tions in H5N1 hemagglutinins of influenza A virus by means of logistic regression, Comp. Clin. Pathol., 15, 255-261. [38] G. Wu and S. Yan, (2006) Prediction of mutations in H5N1 hemagglutinins from influenza A virus, Protein Pept. Lett., 13, 971-976. [39] G. Wu and S. Yan, (2007) Improvement of model for prediction of hemagglutinin mutations in H5N1 influenza
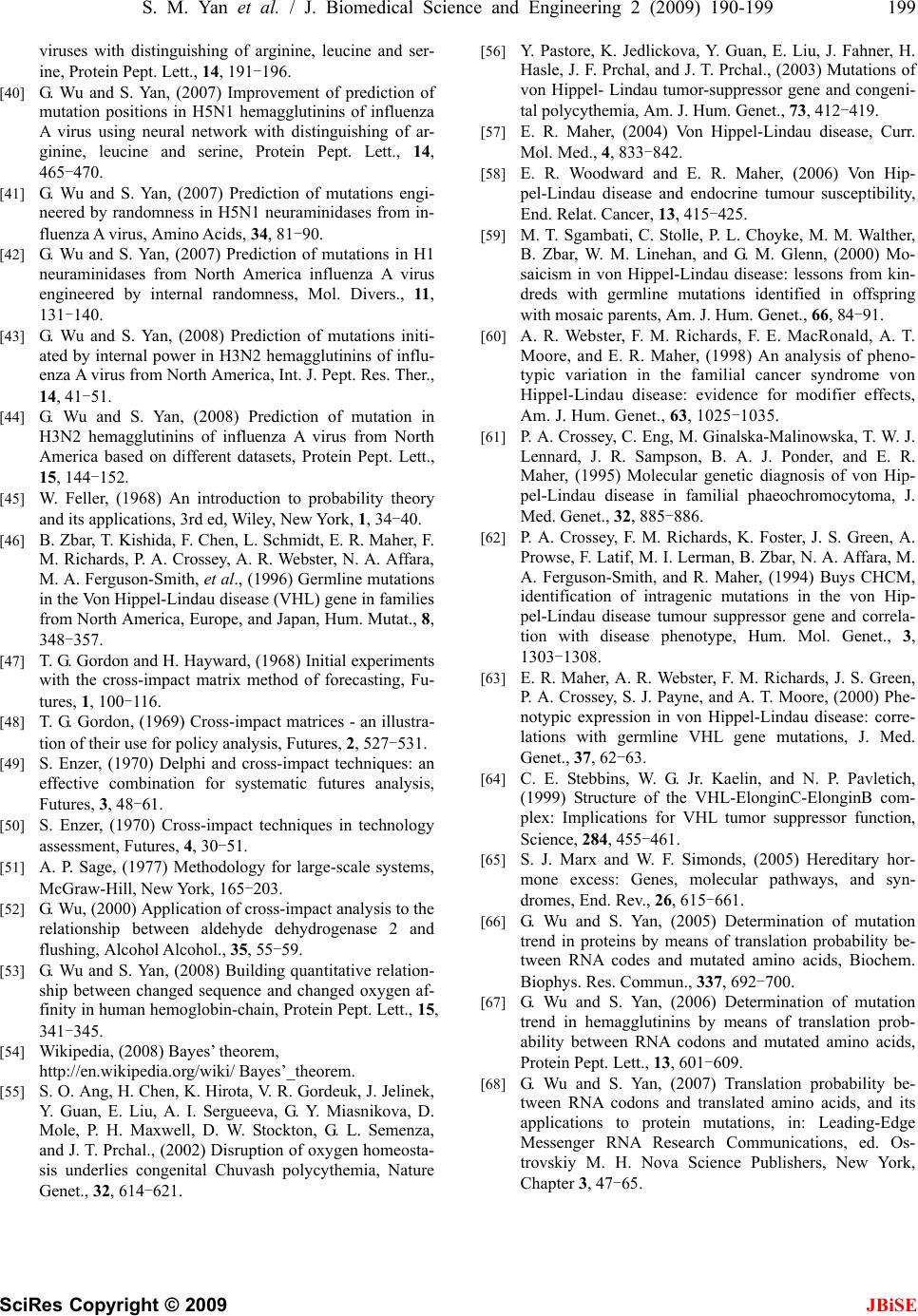 S. M. Yan et al. / J. Biomedical Science and Engineering 2 (2009) 190-199 199 SciRes Copyright © 2009 guishing of arginine, leucine and ser- ine, Protein Pept. Lett., 14, 191-196. 0] G. Wu and S. Yan, (2007) Improvement of prediction of mutation positions in H5N1 hemagglutinins of influenza A virus using neural network with distinguishing of ar- ginine, leucine and serine, Protein Pept. Lett., 14, 465-470. 1] G. Wu and S. Yan, (2007) Prediction of mutations engi- neered by randomness in H5N1 neuraminidases from in- fluenza A virus, Amino Acids, 34, 81-90. 2] G. Wu and S. Yan, (2007) Prediction of mutations in H1 neuraminidases from North America influenza A virus engineered by internal randomness, Mol. Divers., 11, 131-140. [43] G. Wu and S. Yan, (2008) Prediction of mutations initi- ated by internal power in H3N2 hemagglutinins of influ- enza A virus from North America, Int. J. Pept. Res. Ther., 14, 41-51. [44] G. Wu and S. Yan, (2008) Prediction of mutation in H3N2 hemagglutinins of influenza A virus from North America based on different datasets, Protein Pept. Lett., 15, 144-152. [45] W. Feller, (1968) An introduction to probability theory and its applications, 3rd ed, Wiley, New York, 1, 34-40. [46] B. Zbar, T. Kishida, F. Chen, L. Schmidt, E. R. Maher, F. M. Richards, P. A. Crossey, A. R. Webster, N. A. Affara, M. A. Ferguson-Smith, et al., (1996) Germline mutations in the Von Hippel-Lindau disease (VHL) gene in families from North America, Europe, and Japan, Hum. Mutat., 8, 348-357. [47] T. G. Gordon and H. Hayward, (1968) Initial experiments with the cross-impact matrix method of forecasting, Fu- tures, 1, 100-116. [48] T. G. Gordon, (1969) Cross-impact matrices - an illustra- tion of their use for policy analysis, Futures, 2, 527-531. [49] S. Enzer, (1970) Delphi and cross-impact techniques: an effective combination for systematic futures analysis, Futures, 3, 48-61. [50] S. Enzer, (1970) Cross-impact techniques in technology assessment, Futures, 4, 30-51. [51] A. P. Sage, (1977) Methodology for large-scale systems, McGraw-Hill, New York, 165-203. [52] G. Wu, (2000) Application of cross-impact analysis to the relationship between aldehyde dehydrogenase 2 and flushing, Alcohol Alcohol., 35, 55-59. [53] G. Wu and S. Yan, (2008) Building quantitative relation- ship between changed sequence and changed oxygen af- finity in human hemoglobin-chain, Protein Pept. Lett., 15, 341-345. [54] Wikipedia, (2008) Bayes’ theorem, http://en.wikipedia.org/wiki/ Bayes’_theorem. [55] S. O. Ang, H. Chen, K. Hirota, V. R. Gordeuk, J. Jelinek, Y. Guan, E. Liu, A. I. Sergueeva, G. Y. Miasnikova, D. Mole, P. H. Maxwell, D. W. Stockton, G. L. Semenza, and J. T. Prchal., (2002) Disruption of oxygen homeosta- sis underlies congenital Chuvash polycythemia, Nature Genet., 32, 614-621. [56] Y. Pastore, K. Jedlickova, Y. Guan, E. Liu, J. Fahner, H. Hasle, J. F. Prchal, and J. T. Prchal., (2003) Mutations of von Hippel- Lindau tumor-suppressor gene and congeni- tal polycythe mia, Am. J. Hum. Gene t. , 73, 412 -419. [57] E. R. Maher, (2004) Von Hippel-Lindau disease, Curr. Mol. Med., 4, 833-842. [58] E. R. Woodward and E. R. Maher, (2006) Von Hip- pel-Lindau disease and endocrine tumour susceptibility, End. Relat. Cancer, 13, 415-425. [59] M. T. Sgambati, C. Stolle, P. L. Choyke, M. M. Walther, B. Zbar, W. M. Linehan, and G. M. Glenn, (2000) Mo- saicism in von Hippel-Lindau disease: lessons from kin- dreds with germline mutations identified in offspring with mosaic parents, Am. J. Hum. Genet., 66, 84-91. [60] A. R. Webster, F. M. Richards, F. E. MacRonald, A. T. Moore, and E. R. Maher, (1998) An analysis of pheno- typic variation in the familial cancer syndrome von Hippel-Lindau disease: evidence for modifier effects, Am. J. Hum. Genet., 63, 1025-1035. [61] P. A. Crossey, C. Eng, M. Ginalska-Malinowska, T. W. J. Lennard, J. R. Sampson, B. A. J. Ponder, and E. R. Maher, (1995) Molecular genetic diagnosis of von Hip- pel-Lindau disease in familial phaeochromocytoma, J. Med. Genet., 32, 885-886. [62] P. A. Crossey, F. M. Richards, K. Foster, J. S. Green, A. Prowse, F. Latif, M. I. Lerman, B. Zbar, N. A. Affara, M. A. Ferguson-Smith, and R. Maher, (1994) Buys CHCM, identification of intragenic mutations in the von Hip- pel-Lindau disease tumour suppressor gene and correla- tion with disease phenotype, Hum. Mol. Genet., 3, 1303-1308. [63] E. R. Maher, A. R. Webster, F. M. Richards, J. S. Green, P. A. Crossey, S. J. Payne, and A. T. Moore, (2000) Phe- notypic expression in von Hippel-Lindau disease: corre- lations with germline VHL gene mutations, J. Med. Genet., 37, 62-63. [64] C. E. Stebbins, W. G. Jr. Kaelin, and N. P. Pavletich, (1999) Structure of the VHL-ElonginC-ElonginB com- plex: Implications for VHL tumor suppressor function, Science, 284, 455-461. [65] S. J. Marx and W. F. Simonds, (2005) Hereditary hor- mone excess: Genes, molecular pathways, and syn- dromes, End. Rev., 26, 615-661. [66] G. Wu and S. Yan, (2005) Determination of mutation trend in proteins by means of translation probability be- tween RNA codes and mutated amino acids, Biochem. Biophys. Res. Commun., 337, 692-700. [67] G. Wu and S. Yan, (2006) Determination of mutation trend in hemagglutinins by means of translation prob- ability between RNA codons and mutated amino acids, Protein Pept. Lett., 13, 601-609. [68] G. Wu and S. Yan, (2007) Translation probability be- tween RNA codons and translated amino acids, and its applications to protein mutations, in: Leading-Edge Messenger RNA Research Communications, ed. Os- trovskiy M. H. Nova Science Publishers, New York, Chapter 3, 47-65. JBiSE viruses with distin [4 [4 [4
|