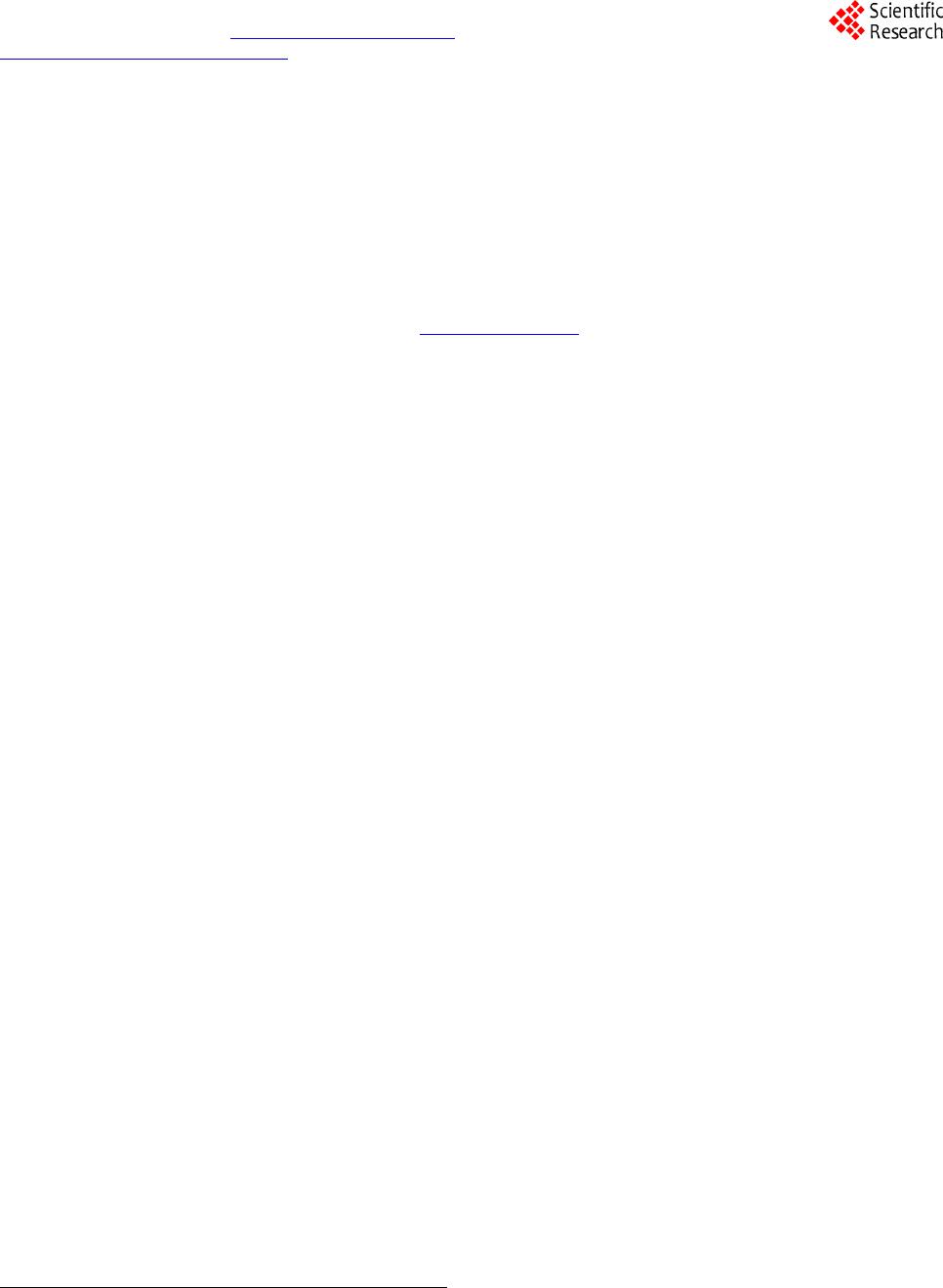 Internationa l Journal of Geosciences, 2014, 5, 27-37 Published Online January 2014 (http://www.scirp.org/journal/ijg) http://dx.doi.org/10.4236/ijg.2014.51005 Remote Sensing Data Application to Monitor Snow Cover Variation and Hydrological Regime in a Poorly Gauged River Catchment—No rthern P ak istan* Samreen Abdul Hakeem, Muhammad Bilal, Arshid Pervez, Adnan Ahmad Tahir# Department of Environmental Sciences, COMSATS Institute of Information Technology, Abbottabad, Pakistan Email: #uaf_adnan@hotmail.fr Received November 16, 2013; revise d December 13, 2013; accepted January 7, 2014 Copyright © 2014 Samreen Abdul Hakeem et al. This i s an open access article di stribu ted under the Creat ive Commons Attribu tion License, which permits unrestricted use, distribution, and reproduction in any medium, provided the original work is properly cited. In accordance of the Creative Commons Attribution License all Copyrights © 2014 are reserved for SCIRP and the owner of the intellectual property Samreen Abdul Hakeem et al. All Copyright © 2014 are guarded by law and by SCIRP as a guardian . ABSTRACT Snow- and glacier-nourished river ba sins located in the Himalaya-Karakoram-Hindukush ( HKH) ranges s upply a significant amount of discharge in River Indus up stream Tarbela Dam. It is, hence, importa nt to comprehend the cryosphere variation and its relationship to the stream flow in these high-altitude river catchments. The MODIS re motely sensed da tabase of snow products wa s chosen to exa mine the a verage annua l snow and gla cier cover (cryosphere) variations in the Shigar River basin (poorly gauged mountainous sub-catchment of the Indus River). Hydrological regime in the area was investigated through monthly database of observed stream fluxes and climate variables (precipitation and mean temperature) for the Shigar River catchment. Analysis indicated the usefulness of remote sensing techniques for estimation of the snow cover variation in the poorly or un-gauged high-elevation catchments of the HKH zone. Results also showed that Shigar River discharge was influenced mainly by the seasonal and annual snow cover area (SCA) variation and the temperature seasonality. Moreover, it is i mportant to uncover s uch inter-relationship of stream flow, climate variables and snow cover in the poo rly gauged high-altitude catchments of Karakoram region for better water resource manage ment and accurate flood hazards predictions at Tarbela. KEYWORDS Shigar River Ba sin; Satellite Remote Sensing; MODIS Snow Products; Water Resources Management; ASTER-GDEM 1. Introduction and Background Pakistan, the world’s sixth largest populated country, is an agro-based economy where the lives of ~60% people (mostly rural inhabitants) are either directly or indirectly affiliated to agriculture. Pakistan is an arid to semi-arid country with rainfall contributing only ~10% to agricul- ture [1]. Agriculture of Pakistan thus depends on irriga- tion system constituted by a gigantic chain of hydraulic structures. The Indus River and its two eastern contribu- tories—Jhelum and Chenab, commit a main share of the water supply to this irrigation system. Reference [2] has shown that the livelihood of the people of Pakistan is strongly dependent on the agriculture, which in turn is relying on the vast irrigation system of the country i.e. Indus Basin Irrigation system. Originating in Tibetan Plateau, the Indus River passes through the towering mountainous ranges of Hindukush-Karakoram-Himalaya (HKH). The catchment area upstream Tarbela reservoir is named as Upper Indus River Basin (UIB) (Figure 1). The catchment area is located in four countries of South Asia namely Pakistan, India, China and Afghanistan, with the largest part in Pakistan. Permanent ice caps in- cluding many of the world’s largest glaciers cover ~11.5 % of the total UIB a rea that make s it largest glacie- rized area out of Polar and Greenland regions [3,4]. Mount ains bring t he main wate r reso urce fo r the Ind us Basin Irrigation System as a consequence of snow- and Remote sensing of sn ow cover va r iation in poorly gauged catchment. OPEN ACCESS IJG
 S. A. HAKEEM ET AL. Figure 1 . Location of the study area and its Global digital elevation model (GDEM). glacier-melt water . The I ndus river channel i n mounta ins of northern-Pakistan contributes more than half the total flow upstream Tarbela Dam (key storage at Indus) (Figure 1). Indus River flow is dispensed by coalition of dir ect runof f from rain fall bot h durin g the winte r and the summer monsoon seasons, and melted outflow from seasonal and permanent snow and ice fields [5]. Different studies (e.g. [6-11]) propose that the snow and glacier melt in the HKH range contributes more than 50% of annua l flow in the Upp er Indus River. Deterioration of snow cover area (SCA) is a globally prevalent phenomenon since past few decades, which is accredited to global warming. The degradation of SCA has an intense influence on stream water availability in the river basins where snow and glacial melt constitute the main component of stream flow in summer. The sta- tus a nd conditio n of cryo sphere is crucial in future water planning; providing more melt water initially on shrink- ing and then reducing it gradually till the glaciers disap- pearance. On the contrary, advancing glaciers may store solid-precipitation, reducing summer stream flow and can cause local hazards [1]. In the preceding century a clear effect of climate change has been observed on snow and ice globally [12]. Climatic factors such as precipita- tion and cloud cover also have an effect on the glacial recede but air temperature is aforethought to be the greatest influential. Refer e nce [13] has stated that changes in temperature are likely to govern the glacial contraction even in the regions where precipitation is expected to raise. While accelerated ice cover melting has been proc- lai med fro m the wor ld incl uding t he nei ghbo uring Gr eat- er Himalaya [14], the Karakoram Range shows a subtle situation as highlighted by the IPCC “Intergovernmental Panel on Climate Change” [12]. Central Karakoram is the biggest of those regions where augmentation of glaciers in length and mass is noticed by various re- searchers (e.g. [15-18]). Since mid-1990s, few of the substantia l gla ci er s i n the Ka r a ko ram Ran ge have surged ; 13 glaciers of intermediate size (10 - 20 km in length) and 16 high-altitude tributaries are perceived to be thickening [4,19]. These variations have been investi- gated only in the high alti tude ce ntral Kar akora m regio n. This discrepancy in glacier development indicates a dif- ferent climate pattern in Karakoram fro m in the Greater Himalaya [20]. Different climatic regimes influence the UIB: the monsoon rainfall dominates the Southern Hi- malayan sub-catchments of UIBand snow and ice abla- tion i s the ma in sour ce of r unoff fr o m Kar a kor a m re gions of UIB [21]. Therefore, the Indus River can be very de- OPEN ACCESS IJG
S. A. HAKEEM ET AL. licate to the climate change in HKH region which, if comes true, will significantly increase or decrease the Indus River discharge in future. Snow cover has a momentous part in the system of Earth’s climate. But the actua l amount of precipitatio n in the form of snow received at high-altitudes is poorly gauged because of well known catching errors (e.g. stro ng wi nds at highe r ele vati ons) or even un -gauge d d ue to the lack of observation stations in these remote areas. Shiga r Rive r basi n (Figure 1) in HKH r egion i san exa m- ple of inadequately gauged catchments, thus posing key concerns in flow forecast from snow and glacier melt. Reference [1] has reported that few measures from ac- cumulation pits above 4000-meters above sea level (m.a.s.l.) in UIB varied from 1000-mm to more than 3000-mm annual precipitation, conditional upon the lo- cation. Mapping snow-cover area and snow volume as explained by [22] is another way to estimate the solid precipitation. Satellite remote sensing can be a very helpful tool to map and examine snow and glacier cover variation in such remote and inaccessible areas [23] and predict the seasonal flow from snowmelt. The use of re- motely sensed satellite data for the mapping of snow- cover extent has a long history reaching back until the 1960s [22]. Reference [22] shows that the satellite re- mote sensing data can efficiently map snow-cover cha- racteristics and snow mass in both high temporal and spatial resolutions. Reference [24] coupled the satellite remote sensing technique with ground observations to analyse successfully the influence of global warming on the Himalayan cryosphere. The management of Tarbela reservoir (Figure 1) cal- culates largely on the summer influx conferred by the snow- and glacier-nourished tributaries of Indus River located in the high-altitudes of HKH range. It is, for this reason, crucial to estimate the seasonal snow cover in high-altitude sno w-fed catchments using remotely sensed satellite data for various ai ms such as hydro meteorology, flood predictions and water resource management. Aforementioned study is one of the few to look into the annual cryosphere (snow and ice cover) change using the remotely sensed MODIS snow cover data (MOD10A2) in a snow- and glacier-fed River basin (Karakoram re- gion). The main research contents of this paper are to inves tigat e the: 1) Average annual snow and glacier cover variation in a high-altitude river catchment area situated in the HKH region using MODIS remote sensing snow pr oduct ; 2) Dependence of River flow on annual cryosphere varia tio n in t he s tud y are a usi ng satel lit e da ta and gr ound observations; Correlation of River flow, climatic variables (mean temperature and precipitation) and snow cover variation in the study area. 2. Study Area The geographical area taken into account for this study is Shigar River basin (Figure 1) (one of the UIB sub-cat- chments) situated in the northern areas (Karakoram re- gion) of Pakistan. Some key characteristics of this river basin are presented in Ta b l e 1. Shigar River basin ranges fro m 74.5˚ - 76.5˚E in Lon- gitude, and 35.2˚ - 36.38˚N in Latitude. The catchment area of Shigar River is ~7000-km2 at S higa r Bri dge (flow gauge point of Shigar River) (Figure 1), as calculated from the Advanced Spaceborne Thermal Emission and Reflection Radiometer-Global Digital Elevation Model (ASTER-GDEM) i n this study. Its entire catchment is situated within Pakistan and is signi fica nt for eva luati ng the influe nce o f climate change within the Karakoram Range of Pakistan. The peak ele- vation in this catchment area is reached by K2-Mountain (Figure 1) with 8611-m.a.s.l. and the lowest point is at Shigar Bridge at 2187-m.a.s.l (Figure 1). The mean ele- vation of the catchment is ~4705-m.a.s.l. (calculated from the hypsometry using ASTER–GDEM) (Figure 2) and ~35% of the area is over 5000-m.a.s.l. (calculated- from the hypsometry using ASTER–GDEM) (Figure 2). The major proportion (~80%, surface area of ~13,000- km2) of total Karakoram glaciers is located within Pakis- tan [25]. The most important are the Biafo (~63-km long) and Baltoro (~60-km long) (Figure 3(a)) situated in the Shigar Ri ver basin. The hydrological regime in the Shigar River basin is, to some extent, influenced by monsoon while the snow and glacier meltin g contrib ute s the maj or pr opor tion. The Shigar River has a mean yearly flow of ~206 m3/s (i. e . 930-mm of water depth equivalent) gauged at Shigar Bridge (Figure 1), as calculated from the 15-years (1985-2001) flow record of the SWHP-WAPDA in this study. The governing sustenance for the glacier systems of the Karakoram Range is provided by the Westerlies precipitation system during winter [26]. There is one AWS (Automatic Weather Station) (Figure 1) installed in the Shigar basin and the other climate station (CS) is installed at Skardu (Figure 1) outside the catchment boundary of Shigar near the catchment outlet. The cli- mate data used in this study is the averaged data of both AWS and CS. The mean total annual precipitation is ~216-mm at Skardu CS and ~405-mm at Shigar AWS according to the available data in the catchment. Being at higher-altitude (~3500-m.a.s.l. as estimated by super- posing the AWS on GDEM—exact value of altitude for AWS should be verified), Shigar AWS receives more precipitation than Skardu CS (~2200-m.a.s.l.). However, the present total annual precipitation records at both cli- mate stations are not illustrative of the runoff at the Shi- gar River outlet because of the climate data scarcity at altitudes above 4000-m and under-estimation of winter OPEN ACCESS IJG
 S. A. HAKEEM ET AL. Table 1. Key characterist ics of the study area (Shigar River basin). Catchment name Shigar River flow gaugin g station Shigar Bridge Latitude 35˚25'12"N Longitude 75˚42'36"E Elevation of river gauging station. ~2 187-m Drainage area (com puted from ASTER GDEM in this study). ~7000-km2 Glacier-cover ed area. ~2120-km2 (Calculated using, “World Glacier Inventory” data in this study) ~2774-km2 [1] ~2240-km2 (a) Glacier c o v er perc entage. 30% - 39% (Estimated from di f f er ent s ources mentioned a bove e. g. W G I , [1], Campbell) Mean eleva tion (com puted from ASTER GDEM in this study). ~4705-m Area above 5000-m (computed from ASTER GDEM in thi s study). ~2460-km2, ~35 % Meteorol ogical station data used in this study. 1 (managed by PMD) – Skardu (~2210-m) 1 (managed by WAPDA) – Shiga r AWS (~3500-m, estimated by supe r-posing the AW S on GDEM) – exact value of altitude for AWS shoul d be verified aCampbell JG (2004) Inventory of Glaciers, Glacial Lakes and the Identification of Potential Glacial Lake Outburst Floods (GLOFs) Affected by Global Warmin g in the Mount ains of Indi a, Paki stan and Chin a/Tib et Auton om ous Region, Fina l rep ort for APN pr oject 2004-03-CMY-Campbe ll. Kath mandu , Nepal: International Centre for Integrated Mountain Development; Kobe, Japan: Asia-Pacific Network for Globa l Change Research. Figure 2 . Hypsometric cu rve and the area u nder 500 -m elevation b and of the Shigar R iver basin. OPEN ACCESS IJG
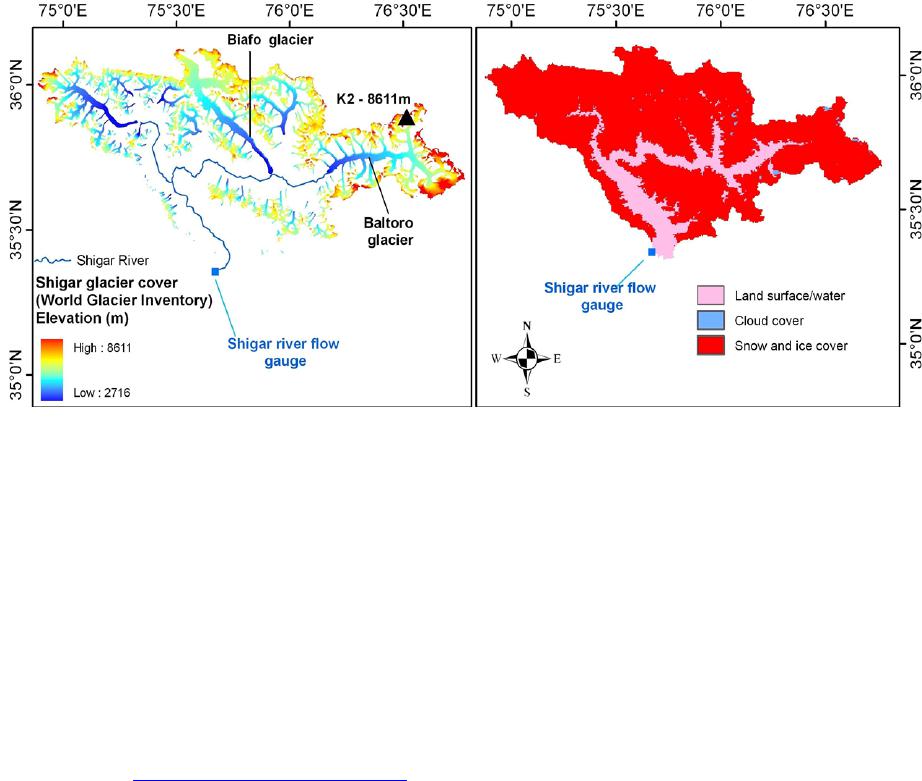 S. A. HAKEEM ET AL. Figure 3. (a) GDEM showing glacier cover area using WGI (World Glacier Inventory) data and (b) An example of MODIS snow cover image for the S higar River basin. precipitation (snow) in this basin and may also be asso- ciated to the well-known gauging errors (wind induced, wetting loss, trace precipitation, blowing and drifting snow, and systematic mechanical errors etc.) in high mountain environment described by many authors, e.g. [27-29]. 3. Data Sets and Treat m ent In order to accomplish the study objectives, two types of data sets were obtained: 1) Satellite Remote Sensing Data (downloaded from the NASA web site http://reverb.echo.nasa.gov/reverb). ● ASTER–GDEM . ● MODIS MOD10A2 snow product. 2) Hydro-meteorological Data (Stream flow, mean temperature and precipitation). 3.1. Satellite Remote Sensing Data The satellite remote sensing data were obtained from two sources to be used for two different purposes. Advanced Spaceborne Thermal Emission and Reflection Radiome- ter (ASTER)-Global Digital Elevation Model (GDEM) data was employed for sketching out the catchment area (Shigar Basin) (Figure 1) and calculating the hypso- metric curve (area-elevation) (Figure 2). The ASTER– GDEM based on ASTER images is handy for high- latitude and steep mountainous areas not attained by SRTM3 [30]. It is set up on ASTER images and has a resolution of 30-m × 30-m. It was referenced to WGS84/ EGM96 coordinate system. The downloaded ASTER tiles were mosaicked and treated through a variety of steps using standard ARCMAP techniques (©1995-2012 ESRI) to draw the Shigar River catchment region. AS- TER-GDEM was also treated to estimate the hypsomet- ric-curve and the catchment area in every 500-m eleva- tion band (Figure 2). World Glacier Inventory (WGI) data [31] was used along with ASTER–GDEM to extract the glacier cover (Figure 3(a)) from the catchment area. Moderate Resolution Imaging Spectroradiometer MO- DIS/Terra Snow Cover 8-Day L3 Global 500-m Grid (MOD10A2) snow products for 10-years (2000- 2009) were utilized to estimate the snow cover variation in the study area. The monthly average values of SCA were estimated to analyse its relationship with hydro-meteo- rological variables. MODIS Snow products (MOD10A2) contain data fields for maximum snow cover extent over an 8-day composite time, projected with the WGS 1984 UTM ZONE 43N projection system. MOD10A2 is con- stituted of 1200-km × 1200-km tiles of 500-m resolution data gridded in a sinusoidal map projection. MODIS cryosphere data is established on a snow-mapping algo- rithm that procures a Normalized Difference Snow Index (NDSI) and other criterion tests [32]. MODIS snow cover products have been extensively exploited to reckon the snow cover area (to couple it with hydrological models for flow simulation and forecasting) by various researchers (e.g. [33-37]) in numerous geo- graphical areas of the world. Reference [38] correlated the accuracy of MODIS, NOAA and IRS Data and illu- strated that MODIS data could be more effective for SCA evaluation, which is an important factor for snow- melt runoff modelling. Many researchers (e.g. [39-41]) evaluated MODIS/Terra daily snow-cover and meteoro- logical product through comparability with ground inves- tigations and found that the misclassified fractions of MOD10A1 were in general lesser at high elevations than at low elevations. Reference [41] observed the snow- OPEN ACCESS IJG
S. A. HAKEEM ET AL. mapping correlation coefficient value of ~95% between seasonal MODIS daily snow maps and in-situ observa- tions under clear-sky conditions. Reference [42] ex- plained that the snow products derived from EOS- MODIS, AMSR-E and QSCAT data have beenharmo- nised into a solo, worldwide, diurnal, user-fri endly p rod- uct via a latest developed Air Force Weather Agency (AFWA)/NASA Snow Algorithm (ANSA). A higher ef- ficiency is also suggested for outlining snow-cover area with the MODIS product than with the AMSR product upon cloud-free MODIS images availability. Reference [42] used MODIS snow product as the default for esti- mati ng snow co ver for thi s previ ously me ntioned reason. The passive microwave product AMSR was preferred barely in those domains where MODIS data were not suitable due to clouds and darkness [42]. The snow-mapping algorithm differentiates pixels as snow, ice lakes, cloud, water, land or other. Snow extent is the variable of prime significance in this data set. The temporal search of snow cover through MOD10A2 was carried out through images downloaded from the above- mentionedNASA website. The study area (Shigar basin) was then extracted by mask function from the treated MODIS snow cover images (Figure 3(b)) using ARC- MAP (©1995-2012 ESRI). Snow cover area was esti- mated from the cloud free images. If the cloud cover ex- ceeded 15% on a specific date then the image was ex- cluded from the time serie s. The sno w cover o n this dat e was then estimated by linearly interpolating between the previous and next cloud free image. 3.2. Hydro-Meteorological Data The hydrological and meteorological data sets were ob- tained from WAPDA (Water and Power Development Authority) and Pakistan Meteorological Department (PMD), respectively. Surface Water Hydrology Project of Water and Power Development Authority (SWHP- WAPDA) mostly do stream flow assessment in Pakistan. PMD-CS has quite a long data series for climate va- riables while WAPDA–AWS are installed in 1995 and thus have shorter data series. The monthly average stream flow was estimated from the available data of ~15-yrs (1985-2001) in order to compr e he nd t he c o rr el at io n a mo n g str eam flo w a nd snow cover area of Shigar Basin. Theavailable data on climatic variables including temperature (min. and max) and pre- cipitation was averaged for the entire period of ~55-yrs (1952-2007) for PMD–CS and ~11-yrs (1996-2006) for WAPD A–AWS to get the monthly average values. The Pearson Product Moment correlation [43], Spearman Rank Order correlation [44] and Kendall’s rank correla- tion [45,46] tests were performed (with a significance level of p = 5%) to evaluate the relationship between monthly stream flow, monthly snow cover variation and climate variables ( monthly mean temperat ure and monthly total precipitation) to scrutinize the core regulating co n- stituents of runoff from t he Sh igar River basin. 4. Results and Discussion 4.1. Snow Cover Variation in the Shigar River Ba si n In the present study, the Snow Cover Area (SCA) for Shiga r B asin was esti mate d th ro ugh MODI S i mage s over the 10-years period (2000-2009). The images were treated through ArcGIS software (©1995-2012 ESRI) and SCA percentage was calculated for each 8-days im- age. This S CA was t he n ave r age d t o mont hl y ti me ste p to get the mean monthly values of SCA variation. MODIS images showing intra-annual SCA variation and its val- ues in the Shigar River basin are presented in Figure 4. The average SCA varies from maximum in winter (De- cember-January) to minimum in summer (July-August) (Figure 4). The snow accumulation begins in the months of September-October and the snow-covered area reached to maximum (~88%) in January (Figure 4). T he maximum snowmelt (minimum s now-covered area ~10%) was observed in the month of August (Figure 4). This huge variation of SCA from ~88% to 10% can be re- flected by high discharges in the Shigar River during snowmelt season because the large snow surface area may contain the higher depths too. This is supported by the study of [26] conducted on the neighbouringHunza basin. This study [26] explains that the Central Karako- ram region undergoes a snow cover change of about 40% - 70% where SCA is 70% - 80% in the winter (snow ac- cumulation period), whereas, SCA is 30% - 40% in the summer (snowmelt period). Thus these snow- and glacier-fed sub-catchments of Upper Indus River Basin (UIB) make this huge catch- ment dependent on the snow and glacier melt water. This result also proves the suitability of remote sensing snow products for the snow cover mapping in the un-gauged snow- and glacier-fed high-altitude river basins. Even if the ground observation stations are installed in these riv- er basins; these are not able to catch the exact amounts of winter solid precipitations due to previously mentioned gauging errors (like strong winds) at high-altitude s. It is not possible to regularly monitor these high-altitude re- mote areas, due to severe climate conditions to cope-with, for the researchers. Therefore, the remote-sensing satel- lite snow images can be the best solution in these in-ac- cessible regions (during certain seasons) to monitor the SCA on regular basis even in the severe climatic condi- tions. The quality of these satellite snow cover datasets is al- ready affirmed by many studies so these may be used for OPEN ACCESS IJG
 S. A. HAKEEM ET AL. Figure 4. MODIS snow cover images showing intra-annual variation of snow cover for the period of 2000-2009 in Shigar River basin. the snow mapping of larger areas like Upper Indus River Basin. 4.2. Dependence of Shigar River Discharge on the Snow Cover Variation The correlation coefficient values showing the relation- ship of mean monthly Shigar river discharge and mean monthly SCA are presented in Figure 5 and Table 2. High stream flow was observed from July to September when the snow cover area was at its minimum (i.e. in summer during the snow melt period) (Figure 5). A si g- nificant (p = 0.05) inverse correlation was observed be- tween SCA and river discharge with r = −0.87 for Pear- son product moment correlation test and r = −0.95 for Spearman rank order correlation test (Figure 5 and Ta- ble 2). Kendall’s rank correlation test indicated a coeffi- cient value of r = −0.82 (p = 0.05) (Table 2). All these values were significant with a significance level of p = 5%. Data reflect that January to April is the low flow period when the snow accumulates, and it begins to in- crease gradually when the snowmelt starts in the spring season. The peak river discharge is witnessed at mini- mum S CA in the months of J uly to Sep tember where the glacial melt and monsoon rainfall over laps the sno w melt. This peak discharge is also the result of ~65% drop in the SCA from April (~78.3%) to July (~13.6%) that gradu- ally increased the river discharge. Assessing the winter snow accumulatio n may be helpful to pred ict the follow- ing spring and summer season inflows in the Shigar Riv- er discharge. Inter-relationship of river discharge, SCA, mean tem- perature and precipitation for the Shigar catchment area is presented in the next section. 4.3. Inter-Relationship o f SCA, River Discharge and Climate Variables (Mean Temperature and Precipitation) All the available data of SCA, river discharge and cli- mate variables (mean temperature and monthly precipita- tion) was analysed to determine the inter-relationship between all these variables in the study area. Three dif- ferent correlation tests (Pearson, Spearman and Kendall) were applied with a significance level of p = 0.05 (Table 2). Correlation of SCA and mean Temperature: A strong inver se co rrela tion wa s found bet ween aver aged monthl y SCA and averaged monthly mean temperature. The cor- relation coefficient values of r = −0.82, −0.78 and −0.64 were found for Pearson, Spearman and Kendall’s corre- lation tests (Ta ble 2), respectively. All these values were OPEN ACCESS IJG
 S. A. HAKEEM ET AL. Figure 5. Relationship of mean snow cover area, mean River discharge, mean tempe rature and total precipitation ( monthly time step) in the Shigar River basin. Table 2. Correlation coefficient values between monthly values of Snow cover area (SC A), River disc harge (Q ), Pre- cipit atio n (P) an d Mean t emper ature ( Tmean) in the S higar River basin. Variables Kendall’s rank correlation Pearson Correlation Spearman correlation Q vs SCA −0.82 −0.87 −0.95 Q vs P 0.54 0.63 0.66 Q vs Tmean 0.70 0.65 0.85 SCA vs Tme an −0.64 −0.78 −0.82 All the correlation coefficient values in this table are significant at p = 0.05. significant with a significance level of p = 0 .05. The co r- relation graph indicated an inverse relationship between the mean temperature and SCA as shown in Figure 5, where an increase in monthly temperature leads to a re- duced SCA and more snow melt and vice versa. The sno w cover is a t maximu m in t he l ow te mper at ure months from December until March, when the accumula- tion of snow is dominant over ablation that becomes prominent in the following warmer months June to Sep- tember causing a marked decrease in the SCA. Minimum SCA is observed at higher mean temperatures in the months of July and August where the snow melt is at peak as discussed earlier. Correlation of Shigar River discharge with Climatic Variables (mean temperature and precipitation): Figure 5 and Table 2 showed the correlation coefficient values between Shigar River discharge and mean temperature of the catchment area observed at Skardu and Shigar cli- mate stations (Figure 1). The significant (p = 0.05) correlation coefficient val- ues of r = 0.85, 0.65 and 0.70 were found for Pearson, Spearman and Kendall’s correlation tests (Table 2), re- spectively. The river discharge and mean temperature correlation curve reflected a direct correlation in the temperature and river discharge in the study area (Figure 5). The river flow starts increasing as the spring season starts and mean temperature tends to increase. The flow gets its peak in July when the mean temperature is at maximum of the year. The low flow periods are observed during low mean temperature periods and the flow is at minimum in the months of December and January when the mean temperature is below freezing point (Figure 5). The increase in mean temperature resulted in a coinci- dent increase in the snow and glacial melt, which re- flected in an increased river flow where the peak was observed at the maximum temperature in the month of J uly (Figure 5) in the study area. The hydrograph of the Shigar River basin was pre- sented in Figure 5 with the precipitation in the form of OPEN ACCESS IJG
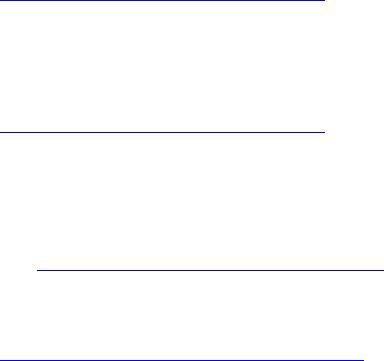 S. A. HAKEEM ET AL. inverted bars and river discharge shown in black solid- line curve. The peak discharge event occurred in the month of July, whereas, the maximum precipitation was rec eived in the mon th o f Aug ust ( Figure 5). This may be due to the accumulation of precipitation at high-altitudes in the form of snowfall in August, which melts later in spring. Table 2 presented the correlation coefficient values between Shigar River discharge and monthly precipita- tion of the catchment area observed at Skardu CS and Shigar AWS (Figure 1). The correlation coefficient val- ues of r = 0.66, 0.63 and 0.54 were found for Pearson, Spearman and Kendall’s correlation tests, respectively. All these values were significant with a significance level of p = 0.05. The peak river discharge and the maximum average rainfall do not coincide which indicates some other controlling factor for Shigar River discharge rather than precipitatio n. The co mparative amount of rainfall i n the months of July and August (peak monsoon) was higher than the other months of the year but yet it was much lower than the mean monthly discharge during these months. Reference [6] stated that as a component of the entire yearly discharge, the catchments of western Himalaya, Karakoram and Hindukush range (e.g. Indus River catchment) are sustained up to 50% by the snow- melt. The correlation values between SCA, stream flow and mean temperature are higher than those between the stream flo w and p r e c ipitation. The above correlation results indicate that the Shigar River flow is affected by the snow cover variation and temperature seasonality rather than by the monthly pre- cipitation. 5. Conclusions Located in the high-altitudes of Karakoram, the Shigar river discharge confides primarily on the amount of snow stored at high altitudes and energy influx, indirectly al- lied to mean temperature. This is proposing that the data estimation methods for climate variables should be up- graded and the remote sensing techniques should be adopted in un-gauged high-altitude catchments to under- stand t he snow co ve r var ia tion a nd r uno f f co nt ro l fa cto r s, further efficaciously. Analyzing the results obtained in this s tudy, can concl ude the follo wing statements: ● Remotely sensed MODIS snow products are useful to estimate the snow cover variation in the poorly- gauged/un-gauged high-altitude catchments of the Karakoram region. These are also useful at times of high snowfall during winter season that subsequently limits the accessibility in such high altitude remote areas. ● Shigar river discharge is largely controlled by the seasonal and annual snow cover area (SCA) variation that is directl y linked to the temperature seasonality. ● Temperature variation strongly affects the snow cover change in the study area that subsequently affects the river discharge. Precipitation does not put forth pro- found effect in the river discharge in comparison of SCA and mean temperature. A continuous monitoring of the snow cover dynamics is required on the high-altitude catchments of the Upper Indus r egio n e .g. Gi lgit , H unza , Shiga r a nd Sh yok. The se are chiefly snow- and glacier- nourished catchments that inject a hefty amount of discharge to the Indus River. The composite of satellite snow cover data and ground data (e.g. snow-pits and AWS) or information generated by s no wme lt -runoff models may be a pertinentscheme to overwhelm the poor gauging of precipitation records at these high-altitudes. Eventually, presentinvestigation may aid to better understand and, enhance the integrated water resource management at the Tarbela reservoir. Ackno wledgements Thanking to WAPDA “Water and Power Development Authority” and the PMD “Pakistan Meteorological De- partment” for providing the hydro-meteorological data. Authors wish also to thank Muhammad Yasir for his valuable comments during manuscript formatting. REFERENCES [1] D. Bocchiola, G. Diolaiuti, A. Soncini, C. Mihalcea, C. D’Agata, C. M a yer, A. Lambrecht, R. Rosso and C . S mi- raglia, “Prediction of Future Hydrological Regimes in Poorly Gauged High Altitude Basins: The Case Study of the Upper Indus, Pakistan,” Hydrology and Earth System Sciences (HE SS), Vol. 15, No. 7, 2011, pp. 2059-2075. http://dx.doi.org/10.5194/hess-15-2059-2011 [2] D. R. Archer, N. Forsythe, H. J. Fowler and S. M. Shah, “Sustainability of Water Resources Management in the Indus Basin under Changing Climatic and socio Eco- nomic Conditions,” Hydrology and Earth System Scien- ces (HESS), Vol. 14, No. 8, 2010, pp. 1669-1680. http://dx.doi.org/10.5194/hess-14-1669-2010 [3] K. Hewitt, “Hazards of Melting as an Option,” Upper Indus Glacier s I & II, 2001. [4] K. Hewitt, “Tributary Glacier Surges: An Exceptional Concentration at Panmah Glacier , Karakor am Himalaya,” Journal of Glaciology, Vol. 53, No. 181, 2007, pp. 181- 188. http://dx.doi.org/10.3189/172756507782202829 [5] D. Archer, “Contrasting Hydrological Regimes in the Upper Indus Basin ,” Journal of Hydrology, Vol. 274, No. 1-4, 2003, pp. 198-210. http://dx.doi.org/10.1016/S0022-1694(02)00414-6 [6] B. Bookhagen and D. W. Burbank, “Toward a Complete Himalayan Hydrological Budget: Spatiotemporal Distri- bution of Snowmelt and Rainfall and Their Impact on River Disch arge,” Journal of Geophysical R esearch, Vo l. 115, No. F3, 2010, Article ID: F03019. [7] W. W. Immerzeel, L. P. H. van Beek and M. F. P. Bier- OPEN ACCESS IJG
 S. A. HAKEEM ET AL. kens, “Climate Change Will Affect the Asian Water Towe r s ,” Science, Vol. 328, No. 5984, 2010, pp. 1382- 1385. http://dx.doi.org/10.1126/science.1183188 [8] K. Hewitt, C. P. Wake, G. J. Young and C. David, “Hy- drological Investigation at Biafo Glacier, Karakoram Range, Himalaya; an Important Source of Water for the Indus River,” Annals of Glaciology, Vo l. 13, 1989, pp. 103-108. [9] C. P. Wake, “Glaciochemical Investigations as a Tool to Determin e the Spat ial Vari ation of Snow Accumulation in the Central Karakoram, Northern Pakistan,” Annals of Glaciology, Vol. 13, 1989, pp. 279-284. [10] G. J. Young and K. Hewitt, “Hydrology Research in the Upper Indus Basin, Karakoram Himala ya, Pakistan,” Hy- drology of Mountanous Areas, Czechoslovakia, 1990, pp. 139-152. [11] H. Liniger, R. Weingartner and M. Grosj ean, “Mountains of the Worl d : Water towers for the 21st Century,” Berne, 1998. [12] IPCC, “Climate Change 2007: Synthesis Report. Contri- bution of Working Groups I, II and III to the Fourth As- sessment Report of the Intergovernmental Panel on Cli- mate Chan ge [Core Writ ing Team, R. K. Pachauri a nd A. Reisin ger, Ed s .] ,” Intergovernmental Panel on Climate Change (IPCC), Geneva, 2007. [13] M. Sivapal an, K. Takeuchi, S . W. F ran ks, V. K . Gupt a, H. Karambiri, V. Lak shmi , X. Li ang, J. J. McDonnell, E. M . Mendiondo, P. E. O’Connell, T. Oki, J. W. Pomeroy, D. Schert zer , S. Uhlenbrook and E. Zehe, “IAHS Decade on Predictions in Ungauged Basins (PUB), 2003 - 2012: Shap- ing an Exciting Future for the Hydrological Sciences,” Hydrological Sciences Journal, Vol. 48, No. 6, 2003, pp. 857-880. http://dx.doi.org/10.1623/hysj.48.6.857.51421 [14] E. Bert hier, Y. Arn aud, R. K uma r, S . Ahmad , P . Wagn on and P. Chevallier, “Remote Sensing Estimates of Glacier Mass Balances in the Himachal Pradesh (Western Hima- laya, India),” Remote Sensing of Environment, Vol . 108, No. 3, 2007, pp. 32 7-338. http://dx.doi.org/10.1016/j.rse.2006.11.017 [15] J. Gardelle, E. B er thier and Y. Arnaud, “Sli ght M ass Gain of Karakora m Glaciers in the Early Twenty-First Century,” Nature Geoscience, Vol. 5, No. 5, 2012, pp. 322-325. http://dx.doi.org/10.1038/ngeo1450 [16] J. Gardelle, E. Berthier, Y. Arnaud and A. Kääb, “Re- gion-Wide Glacier Mass Balances over the Pamir-Kara- koram-Himalaya during 1999-2011,” The Cryosphere, Vol. 7, No. 4, 2013, pp. 1263-1286. [17] A. Kääb, E. Ber th ier, C. Nuth , J. Gardelle and Y. Arnaud, “Contrasting Patterns of Earl y Twent y-First-Century Gla- cier Mass Change in the Himalayas,” Nature, Vol. 488, No. 7412, 2012, pp. 495-498. http://dx.doi.org/10.1038/nature11324 [18] T. Ya o, L. Thompson, W. Y ang, W. Yu , Y. Gao , X. Gu o, X. Yang, K. Duan, H. Zhao, B. Xu, J. Pu, A. Lu, Y. Xiang, D. B. Kattel and D. Joswiak, “Different Glacier Status with Atmospheric Circulations in Tibetan Plateau and Surroundings,” Nature Climate Change, V o l. 2, No. 9, 2012, pp. 1-5. [19] K. Hewitt, “The Kar akora m Ano mal y? Glacier Exp ansi on and the Elevation Effect, Karakoram Himalaya,” Moun- tain Resear ch and Development, Vol. 25, No. 4, 2005, pp. 332-340. http://dx.doi.org/10.1659/0276-4741(2005)025[0332:TK AGEA]2.0.CO ; 2 [20] H. J. Fowler and D. R. Archer, “Conflicting Signals of Climatic Change in the Upper Indus Basin,” Journal of Climate, Vol. 19, No. 17, 2006, pp. 4276-4293. http://dx.doi.org/10.1175/JCLI3860.1 [21] C. Mayer, A. Lambrecht, C. Mihalcea, M. Belò, G. Di- olaiuti, C. Smiraglia and F. Bashir, “Analysis of Glacial Meltwater in Bagrot Valley, Kar akoram,” Mountain Re- search and Development, Vol. 30, No. 2, 2010, pp. 169- 177. http://dx.doi.org/10.1659/MRD-JOURNAL-D-09-00043. 1 [22] A. J. Dietz, C. Kuenzer, U. Gessner and S. Dech, “Re- mote Sensing of Snow—A Review of Avai lab le Met hod s,” International Journal of Remote Sensing, Vol. 33, No. 13, 2011, pp. 4094-4134. http://dx.doi.org/10.1080/01431161.2011.640964 [23] P. Sirguey, R. Mathieu and Y. Arnaud, “Subpixel Moni- toring of the Seasonal Snow Cover with MODIS at 250-m Spatial Resolution in the Southern Alps of New Zealand: Methodology and Accuracy Assessment,” Remote Sens- ing of Envi r o nm e nt , Vol. 113, No. 1, 2009, pp. 160-181. http://dx.doi.org/10.1016/j.rse.2008.09.008 [24] A. V. Kulkarni, B. P. Rathore, S. K. Singh and I. M. Ba- huguna, “Understanding Changes in the Himalayan Cry- osphere Using Remote Sensing Techniques,” Intern a- tional Journal of Remote Sensing, Vol. 32, No. 3, 2011, pp. 601-615. http://dx.doi.org/10.1080/01431161.2010.517802 [25] J. H. Mercer, “Glaciers of the Karakoram,” In: W. O. Field, Ed., Mountain Glaciers of the Northern Hemis- phere, CRREL, Hano ver New Hampshire, 1975, pp. 371- 409. [26] A. A. Tahir, P. Chevallier, Y. Arnaud and B. Ahmad, “Snow Cover Dynamics and Hydrological Regi me of the Hunza River Basin, Karakoram Range, Northern Pakis- tan,” Hydrology and Earth System Sciences (HESS), Vol. 15, No. 7, 2011, pp. 22 75-2290. http://dx.doi.org/10.5194/hess-15-2275-2011 [27] B. Sevruk, “Correction of Precipitation Measurements,” Workshop on the Correction of Precipitation Measure- ments, Zurich, 1985, pp. 13-23. [28] B. Sevruk, “Reliability of precipitation Measurement,” International Workshop on Precipitation Measurement, 1989, pp. 13-19. [29] E. J. Førland, P. Allerup, B. Dahlström, E. Elomaa , T. Jónsson, H. Madsen, J. Perälä, P. Ri ssanen, H . V ed in and F. Vejen, “Manual for Operational Correction of Nordic Precipitation Data,” DNMI, Oslo, 1996. [30] METI and NASA, “ASTER Global Digital Elevation Model (GDEM),” 2009. http://www.ersdac.or.jp/GDEM/E/2.html [31] NSIDC, “World Glacier Inventory. Compiled and Made OPEN ACCESS IJG
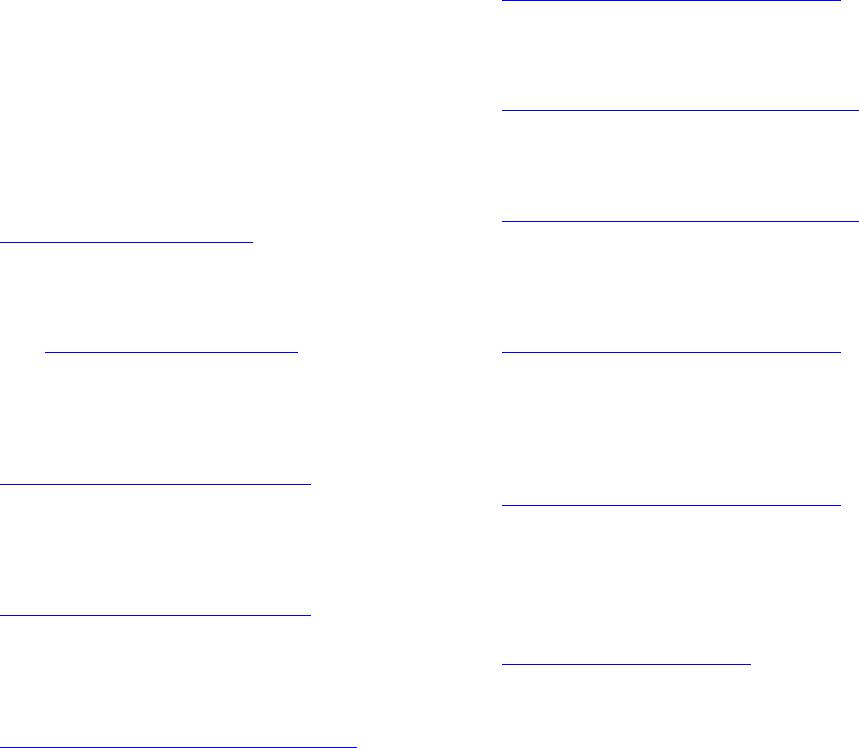 S. A. HAKEEM ET AL. Available by the World Glacier Monitoring Service, Zu- rich, Switzerland, and the National Snow and Ice Data Center, ” Boulder CO, U.S.A., National Snow and Ice Data Center, 1989, updated 2012. [32] D. Hall, G. Riggs and V. Salomonson, “MODIS/Terra Snow Cover 8-Day L3 Global 500m Grid V005,” March 2000 to December 2009, National Snow and Ice Data Center, Boulder, Colorado, Digital media, 2006, updated weekly. [33] E. P. Maurer, J. D. Rhoads, R. O. Dubayah and D. P. Lettenmaier, “Evaluation of the Snow-Covered Area Dat a Product from MODIS,” Hydrol ogical Pro cesses, Vol. 17, No. 1, 2003, pp. 59-71. http://dx.doi.org/10.1002/hyp.1193 [34] S. Lee, A. G. Klein and T. M. Over, “A Comparison of MODIS and NOHRSC Snow-Cover Products for Simu- lating Streamflow Using the Snowmelt Runoff Model,” Hydrol ogi cal P ro cesses, Vol. 19, No. 15, 2005, pp. 2951- 2972. http://dx.doi.org/10.1002/hyp.5810 [35] A. E. Tekeli, Z. Akyürek, A. ArdaSorman, A. Sensoy and A. ÜnalSorman, “Using MODIS Snow Cover Maps in Modeling Snowmelt Runoff Process in the Eastern Part of Turkey,” Remote Sensing of Environment, Vol. 97, No. 2, 2005, pp . 216-230. http://dx.doi.org/10.1016/j.rse.2005.03.013 [36] W. W. I mmerzeel, P. Droogers, S. M. de Jong and M. F. P. Bier kens, “Large-Scale Monitoring of Snow Cover and Runoff Simulation in Himalayan River Basins Using Remote Sensing,” Remote Sensing of Environment, Vol. 113, No. 1, 2009, pp. 40-49. http://dx.doi.org/10.1016/j.rse.2008.08.010 [37] Z. Akyurek, D. K. Hall, G. A. Riggs and A. Sensoy, “Eva- luating the Utility of the ANSA Blended Snow Cover Product in the Mountains of Eastern Turkey,” Interna- tional Journal of Remote Sensing, Vol. 31, No. 14, 2010, pp. 3727 -3744. http://dx.doi.org/10.1080/01431161.2010.483484 [38] S. K. Jain, A. Goswami and A. K. Saraf, “Accuracy As- sess ment o f MODIS, NOAA and IRS Data in Snow Cover Mapping under Himalayan Conditions,” International Journal of Remote Sensing, Vol. 29, No. 20, 2008, pp. 5863-5878. http://dx.doi.org/10.1080/01431160801908129 [39] Q. Tang, M. Durand, D. P. Lettenmaier and Y. Hong, “Satellite-Based Observations of Hydrological Processes,” International Journal of Remote Sensing, Vol. 31, No. 14, 2010, pp . 3661-3667. http://dx.doi.org/10.1080/01431161.2010.483496 [40] Q. Tang and D. P. Lettenmaier, “Use of Satellite Snow-Cover Data for Streamflow Prediction in the Feather River Basin , Califor nia,” International Journal of Remote Sensing, Vol. 31, No. 14, 2010, pp. 374 5-3762. http://dx.doi.org/10.1080/01431161.2010.483493 [41] X. Huang, T. Liang, X. Zhang and Z. Guo, “Validation of MODIS Snow Cover Products Using Landsat and Ground Measurements during the 2001-2005 Snow Seasons over Northern Xinjiang, China,” International Journal of Re- mote Sensing, Vol. 32 , No. 1, 2011, pp. 133-152. http://dx.doi.org/10.1080/01431160903439924 [42] J. L. Foster, D. K. Hall, J. B. Eylander, G. A. Riggs, S. V. Nghiem, M. Tedesco, E. Kim, P. M. Montesano, R. E. J. Kelly, K. A. Casey and B. Choudhury, “A Blended Global Snow Product Using Visible, Passive Microwave and Scatterometer Satellite Data,” International Journal of Remote Sensing, Vol. 32, No. 5, 2011, pp. 1371-1395. http://dx.doi.org/10.1080/01431160903548013 [43] J. L. Rodgers and W. A. Nicewander, “Thirteen Ways to Look at the Correlation Coefficient,” The American Sta- tistician, Vol. 42, No. 1, 1988, pp. 59-66. [44] C. Spearman, “The Proof and Measurement of Associa- tion between Two Things,” American Journal of Psy- chology, Vol. 15, No. 1, 1904, pp. 72-101. http://dx.doi.org/10.2307/1412159 [45] M. G. Kendall, “Rank Correlation Measures,” Charles Gr iff i n, L ondo n, 19 75 . [46] M. G. Kendall and J. D. Gibbons, “Rank Correlation Me- thods,” Edward Arnold, London, 1990. OPEN ACCESS IJG
|