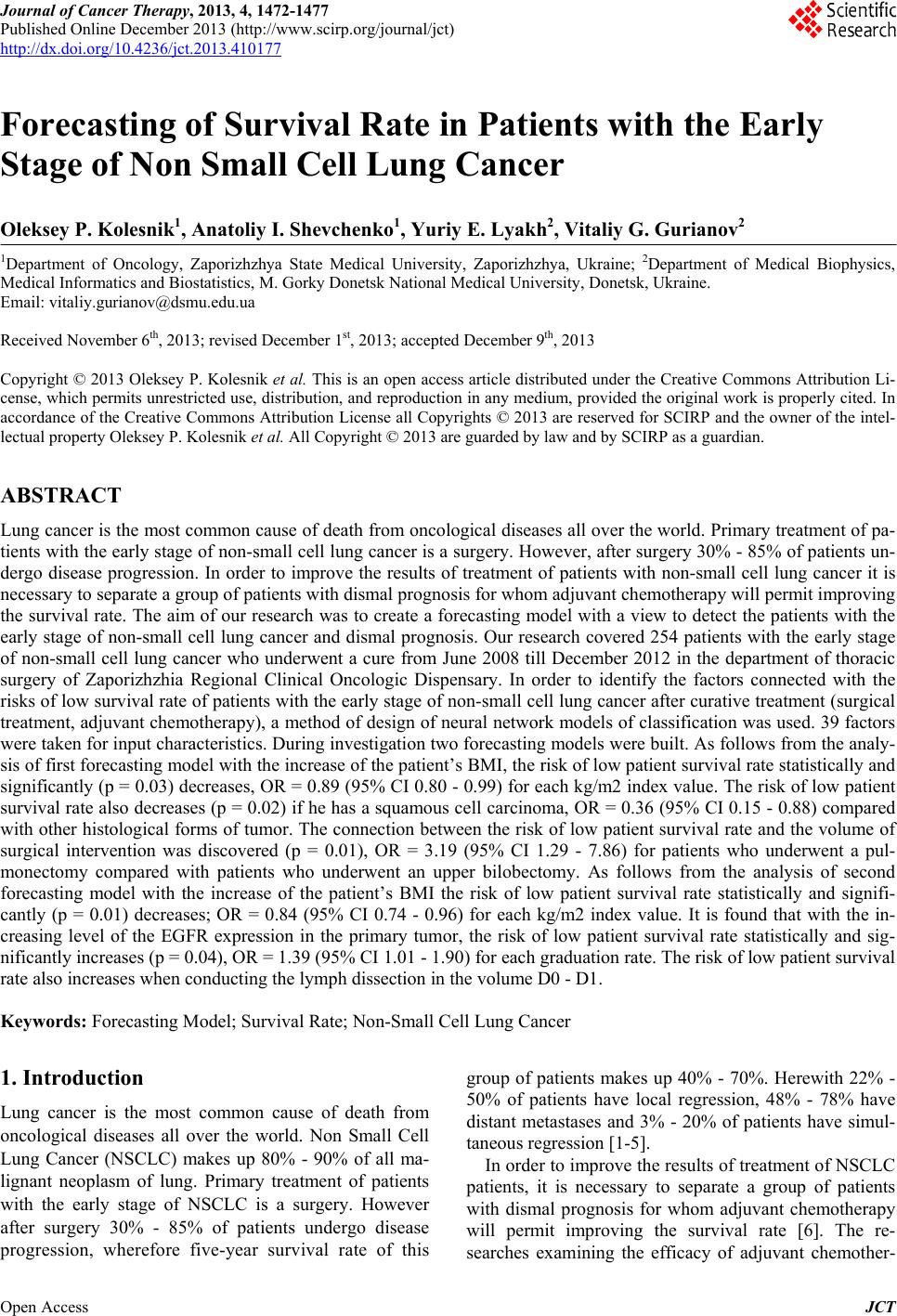 Journal of Cancer Therapy, 2013, 4, 1472-1477 Published Online December 2013 (http://www.scirp.org/journal/jct) http://dx.doi.org/10.4236/jct.2013.410177 Open Access JCT Forecasting of Survival Rate in Patients with the Early Stage of Non Small Cell Lung Cancer Oleksey P. Kolesnik1, Anatoliy I. Shevchenko1, Yuriy E. Lyakh2, Vitaliy G. Gurianov2 1Department of Oncology, Zaporizhzhya State Medical University, Zaporizhzhya, Ukraine; 2Department of Medical Biophysics, Medical Informatics and Biostatistics, M. Gorky Donetsk National Medical University, Donetsk, Ukraine. Email: vitaliy.gurianov@dsmu.edu.ua Received November 6th, 2013; revised December 1st, 2013; accepted December 9th, 2013 Copyright © 2013 Oleksey P. Kolesnik et al. This is an open access article distributed under the Creative Commons Attribution Li- cense, which permits unrestricted use, distribution, and reproduction in any medium, provided the original work is properly cited. In accordance of the Creative Commons Attribution License all Copyrights © 2013 are reserved for SCIRP and the owner of the intel- lectual property Oleksey P. Kolesnik et al. All Copyright © 2013 are guarded by law and by SCIRP as a guardian. ABSTRACT Lung cancer is the most common cause of death from oncological diseases all over the world. Primary treatment of pa- tients with the early stage of non-small cell lung cancer is a surgery. However, after surgery 30% - 85% of patients un- dergo disease progression. In order to improve the results of treatment of patients with non-small cell lung cancer it is necessary to separate a group of patients with dismal prognosis for whom adjuvant chemotherapy will permit improving the survival rate. The aim of our research was to create a forecasting model with a view to detect the patients with the early stage of non-small cell lung cancer and dismal prognosis. Our research covered 254 patients with the early stage of non-small cell lung cancer who underwent a cure from June 2008 till December 2012 in the department of thoracic surgery of Zaporizhzhia Regional Clinical Oncologic Dispensary. In order to identify the factors connected with the risks of low survival rate of patients with the early stage of non-small cell lung cancer after curative treatment (surgical treatment, adjuvant chemotherapy), a method of design of neural network models of classification was used. 39 factors were taken for input characteristics. During investigation two forecasting models were built. As follows from the analy- sis of first forecasting model with the increase of the patient’s BMI, the risk of low patient survival rate statistically and significantly (p = 0.03) decreases, OR = 0.89 (95% CI 0.80 - 0.99) for each kg/m2 index value. The risk of low patient survival rate also decreases (p = 0.02) if he has a squamous cell carcinoma, OR = 0.36 (95% CI 0.15 - 0.88) compared with other histological forms of tumor. The connection between the risk of low patient survival rate and the volume of surgical intervention was discovered (p = 0.01), OR = 3.19 (95% CI 1.29 - 7.86) for patients who underwent a pul- monectomy compared with patients who underwent an upper bilobectomy. As follows from the analysis of second forecasting model with the increase of the patient’s BMI the risk of low patient survival rate statistically and signifi- cantly (p = 0.01) decreases; OR = 0.84 (95% CI 0.74 - 0.96) for each kg/m2 index value. It is found that with the in- creasing level of the EGFR expression in the primary tumor, the risk of low patient survival rate statistically and sig- nificantly increases (p = 0.04), OR = 1.39 (95% CI 1.01 - 1.90) for each graduation rate. The risk of low patient survival rate also increases when conducting the lymph dissection in the volume D0 - D1. Keywords: Forecasting Model; Survival Rate; Non-Small Cell Lung Cancer 1. Introduction Lung cancer is the most common cause of death from oncological diseases all over the world. Non Small Cell Lung Cancer (NSCLC) makes up 80% - 90% of all ma- lignant neoplasm of lung. Primary treatment of patients with the early stage of NSCLC is a surgery. However after surgery 30% - 85% of patients undergo disease progression, wherefore five-year survival rate of this group of patients makes up 40% - 70%. Herewith 22% - 50% of patients have local regression, 48% - 78% have distant metastases and 3% - 20% of patients have simul- taneous regression [1-5]. In order to improve the results of treatment of NSCLC patients, it is necessary to separate a group of patients with dismal prognosis for whom adjuvant chemotherapy will permit improving the survival rate [6]. The re- searches examining the efficacy of adjuvant chemother-
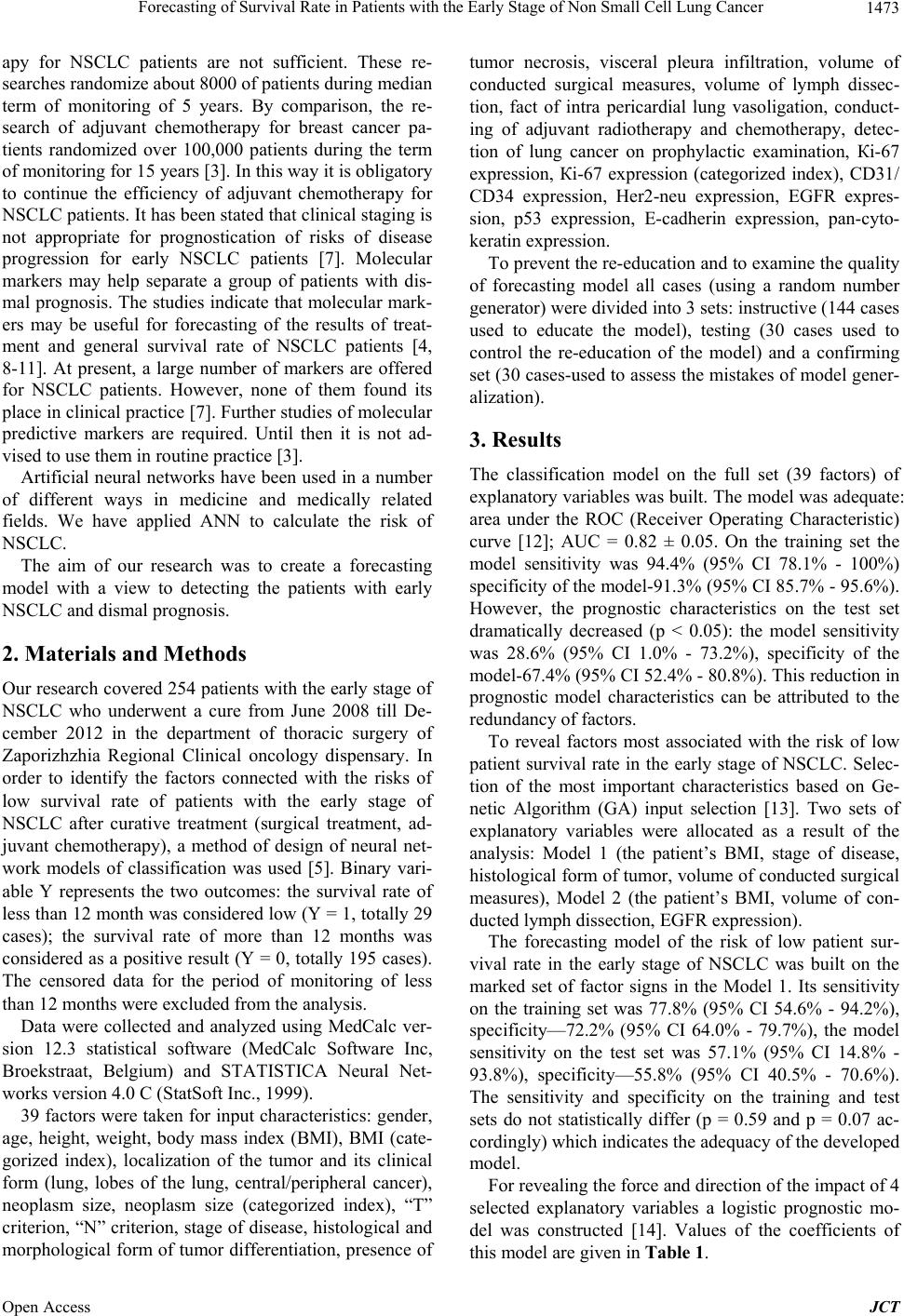 Forecasting of Survival Rate in Patients with the Early Stage of Non Small Cell Lung Cancer 1473 apy for NSCLC patients are not sufficient. These re- searches randomize about 8000 of patients during median term of monitoring of 5 years. By comparison, the re- search of adjuvant chemotherapy for breast cancer pa- tients randomized over 100,000 patients during the term of monitoring for 15 years [3]. In this way it is obligatory to continue the efficiency of adjuvant chemotherapy for NSCLC patients. It has been stated that clinical staging is not appropriate for prognostication of risks of disease progression for early NSCLC patients [7]. Molecular markers may help separate a group of patients with dis- mal prognosis. The studies indicate that molecular mark- ers may be useful for forecasting of the results of treat- ment and general survival rate of NSCLC patients [4, 8-11]. At present, a large number of markers are offered for NSCLC patients. However, none of them found its place in clinical practice [7]. Further studies of molecular predictive markers are required. Until then it is not ad- vised to use them in routine practice [3]. Artificial neural networks have been used in a number of different ways in medicine and medically related fields. We have applied ANN to calculate the risk of NSCLC. The aim of our research was to create a forecasting model with a view to detecting the patients with early NSCLC and dismal prognosis. 2. Materials and Methods Our research covered 254 patients with the early stage of NSCLC who underwent a cure from June 2008 till De- cember 2012 in the department of thoracic surgery of Zaporizhzhia Regional Clinical oncology dispensary. In order to identify the factors connected with the risks of low survival rate of patients with the early stage of NSCLC after curative treatment (surgical treatment, ad- juvant chemotherapy), a method of design of neural net- work models of classification was used [5]. Binary vari- able Y represents the two outcomes: the survival rate of less than 12 month was considered low (Y = 1, totally 29 cases); the survival rate of more than 12 months was considered as a positive result (Y = 0, totally 195 cases). The censored data for the period of monitoring of less than 12 months were excluded from the analysis. Data were collected and analyzed using MedCalc ver- sion 12.3 statistical software (MedCalc Software Inc, Broekstraat, Belgium) and STATISTICA Neural Net- works version 4.0 C (StatSoft Inc., 1999). 39 factors were taken for input characteristics: gender, age, height, weight, body mass index (BMI), BMI (cate- gorized index), localization of the tumor and its clinical form (lung, lobes of the lung, central/peripheral cancer), neoplasm size, neoplasm size (categorized index), “Т” criterion, “N” criterion, stage of disease, histological and morphological form of tumor differentiation, presence of tumor necrosis, visceral pleura infiltration, volume of conducted surgical measures, volume of lymph dissec- tion, fact of intra pericardial lung vasoligation, conduct- ing of adjuvant radiotherapy and chemotherapy, detec- tion of lung cancer on prophylactic examination, Кі-67 expression, Кі-67 expression (categorized index), CD31/ CD34 expression, Her2-neu expression, EGFR expres- sion, p53 expression, Е-cadherin expression, pan-cyto- keratin expression. To prevent the re-education and to examine the quality of forecasting model all cases (using a random number generator) were divided into 3 sets: instructive (144 cases used to educate the model), testing (30 cases used to control the re-education of the model) and a confirming set (30 cases-used to assess the mistakes of model gener- alization). 3. Results The classification model on the full set (39 factors) of explanatory variables was built. The model was adequate: area under the ROC (Receiver Operating Characteristic) curve [12]; AUC = 0.82 ± 0.05. On the training set the model sensitivity was 94.4% (95% CI 78.1% - 100%) specificity of the model-91.3% (95% CI 85.7% - 95.6%). However, the prognostic characteristics on the test set dramatically decreased (p < 0.05): the model sensitivity was 28.6% (95% CI 1.0% - 73.2%), specificity of the model-67.4% (95% CI 52.4% - 80.8%). This reduction in prognostic model characteristics can be attributed to the redundancy of factors. To reveal factors most associated with the risk of low patient survival rate in the early stage of NSCLC. Selec- tion of the most important characteristics based on Ge- netic Algorithm (GA) input selection [13]. Two sets of explanatory variables were allocated as a result of the analysis: Model 1 (the patient’s BMI, stage of disease, histological form of tumor, volume of conducted surgical measures), Model 2 (the patient’s BMI, volume of con- ducted lymph dissection, EGFR expression). The forecasting model of the risk of low patient sur- vival rate in the early stage of NSCLC was built on the marked set of factor signs in the Model 1. Its sensitivity on the training set was 77.8% (95% CI 54.6% - 94.2%), specificity—72.2% (95% CI 64.0% - 79.7%), the model sensitivity on the test set was 57.1% (95% CI 14.8% - 93.8%), specificity—55.8% (95% CI 40.5% - 70.6%). The sensitivity and specificity on the training and test sets do not statistically differ (p = 0.59 and p = 0.07 ac- cordingly) which indicates the adequacy of the developed model. For revealing the force and direction of the impact of 4 selected explanatory variables a logistic prognostic mo- del was constructed [14]. Values of the coefficients of this model are given in Table 1. Open Access JCT
 Forecasting of Survival Rate in Patients with the Early Stage of Non Small Cell Lung Cancer Open Access JCT 1474 Table 1. Coefficients of 4-factor forecasting model of the risk of low patient survival rate. Factor The value of forecasting model coefficients, b ± mLevel of significance difference, p Odds ratio (OR) (95% CI OR) The patient’s BMI −0.12 ± 0.05 0.03 0.89 (0.80 - 0.99) Stage of disease 0.04 ± 0.20 0.82 – Histological form of tumor −1.00 ± 0.45 0.02 0.36 (0.15 - 0.88) Type of conducted surgery 1.16 ± 0.46 0.01 3.19 (1.29 - 7.86) *-statistically significant, p < 0.05. As follows from the analysis (Table 1) with the in- creasing of the patient’s BMI the risk of low patient sur- vival rate statistically significant (p = 0.03) decreases, odds ratio (OR) = 0.89 (95% CI 0.80 - 0.99) for each kg/m2 index value. The risk of low patient survival rate also decreases (p = 0.02) if he has a squamous cell car- cinoma, OR = 0.36 (95% CI 0.15 - 0.88) compared with other histological forms of tumor. The connection be- tween the risk of low patient survival rate and the volume of surgical intervention was discovered (p = 0.01), OR = 3.19 (95% CI 1.29 - 7.86) for patients who underwent a pulmonectomy compared with patients who underwent an upper bilobectomy. Thus, based on this forecasting model, the most fa- vorable prognosis was determined in patients with high BMI, squamous cell lung cancer, conducted surgery in the volume of lobectomy. Accordingly, the unfavorable prognosis was determined in patients with low BMI, non- squamous cell lung cancer, conducted surgery in volume of pulmonectomy. The forecasting model of the risk of low patient sur- vival rate in the early-stage of NSCLC was built on the marked set of factor signs in the Model 2. Its sensitivity on the training set was 66.7% (95% CI 42.2% - 87.1%), specificity—69.8% (95% CI 61.5% - 77.6%). The model sensitivity on the test set was 42.9% (95% CI 6.2% - 85.2%), specificity—67.4% (95% CI 52.4% - 80.8%). The sensitivity and specificity on the training and test sets do not statistically differ (p = 0.53 and p = 0.92 ac- cordingly) which indicates the adequacy of the developed model. For revealing the force and direction of the impact of 3 selected factors a logistic forecasting model was con- structed [14]. Values of the coefficients of this model are given in Table 2. As follows from the analysis (Table 2) with the in- creasing of the patient’s BMI the risk of low patient sur- vival rate statistically significant (p = 0.01) decreases; OR = 0.84 (95% CI 0.74 - 0.96) for each kg/m2 index value. It is found that with the increasing level of EGFR the expression in the primary tumor the risk of low patient survival rate statistically significant increases (p = 0.04), OR = 1.39 (95% CI 1.01 - 1.90) for each graduation rate. The risk of low patient survival rate also increases when conducting the lymph dissection in the volume D0 - D1. That is the high BMI, low level of EGFR expression, and D2 lymph dissection are favorable prognostic factors. Accordingly, the low BMI, high EGFR expression, and D0 - D1 lymph dissection are unfavorable factors. Comparison of ROC curves test has been used to compare the prognostic characteristics of the models Model 1 and Model 2 for the prediction of the risk of low patient survival rate in the early-stage of NSCLC. Figure 1 shows the ROC-curves of the models. The area under the ROC-curve for Model 1: AUC = 0.72 ± 0.05 statistically significant (p < 0.001) differs from 0.5 and indicating the importance of selected vari- ables. The area under the ROC-curve for Model 2: AUC = 0.70 ± 0.05 statistically significant (p < 0.001) differs from 0.5 and indicating the importance of selected vari- ables too. There is no significant difference between two AUC (p = 0.79). Therefore, the conducted analysis shows that the risk of low patient survival rate with the early-stage of NSCLC can obtain adequate prediction based on the analysis of patient’s BMI, histological structure of the tumor, volume of conducted surgery or patient’s BMI, volume of conducted lymph dissection, and the level of EGFR expression. In both models the patient’s BMI is an important prognostic parameter. Moreover, the survival rate worsens with a decrease of this parameter and patients with low BMI have unfavorable prognosis. Except BMI, the his- tological structure of tumor has the important prognostic value. In our study the non-squamous form of the tumor was prognostic unfavorable. Perhaps this is due to the fact that this version includes malignant neoplasms of glan- dular structure, mixt-forms and undifferentiated tumors. Another important factor in prediction of disease outcome in patients with the early-stage of NSCLC is the stage of the disease. This fact is not new but confirms the correct selection of explanatory variables and the proper staging in the performance of the study. It is marked that the prognosis deteriorates for examined patients with in- creasing stage. The type of conducted operative interfere- ence and the volume of executed lymph dissection
 Forecasting of Survival Rate in Patients with the Early Stage of Non Small Cell Lung Cancer 1475 Table 2. Coefficients of 3-factor forecasting model of the risk of low patient survival rate. Factor The value of forecasting model coefficients, b ± m Level of significance difference, p Odds ratio (OR) (95% CI OR) The patient’s BMI –0.17 ± 0.07 0.01* 0.84 (0.74 - 0.96) The volume of conducted lymph dissection –1.05 ± 0.60 0.08 – EGFR expression 0.33 ± 0.16 0.04* 1.39 (1.01 - 1.90) *-statistically significant, p < 0.05. Figure 1. ROC-curves of the models of the risk of low patient survival rate: Mod el 1 (1), Mod el 2 ( 2), –“O” marked optimal values of sensitivity and specificity of the model. showed the important prognostic value. In patients who underwent the pneumonectomy the deterioration of sur- vival rate was revealed. Perhaps, due to the large spread of tumor at the time of the intervention. At the same time the greater volume of lymph dissection significantly improves the survival rate of patients with the early stage of NSCLC that is possible due to the removal of micro metastases and more correct staging of disease. Of the examined mo- lecular markers the EGFR expression was prognostic significant. This marker indicates the presence of epi- dermal growth factor on the membrane of tumor cells. The higher number of receptors-the less favorable prognosis of patients examined. This is because of the fact that the work of this receptor is associated with many important processes occurring in the cell, including the increase of proliferation, apoptosis, cell adhesion, increase of metas- tatic potential of the tumor. Using these prognostic models it is possible to select patients with a poor prognosis for conducting the chemotherapy (PCT). 4. Discussion A number of studies are devoted to find the prognostic factors [4,8-11]. The analyzed clinical, morphological, genetic factors to predict the survival rate of patients with NSCLC were done. To predict the survival rate Leonar- dus and coauthors (2010) used a model that included the following clinical factors: sex, age, smoking, function of external breathing, comorbidities, stage, type of resection, histology. It is shown that the forecasting model ade- quately predicts 1, 2 year survival rate [15]. The follow- ing clinical factors were also analyzed in our study: sex, age, height, weight, body mass index (BMI), BMI (cate- gorized index), localization of the tumor and its clinical form (lung, lobes of the lung, central/peripheral cancer), neoplasm size, neoplasm size (categorized index), “T” criterion, “N” criterion, stage of disease. However, only the BMI was prognostic significant. Harpole and coau- thors (1995) showed in their study of 1928 patients that in the prognostic correlation of 16 analyzed factors (sex, hemoptysis, cough, chest pain, age, smoking, dyspnea, type of surgical treatment, histology, tumor size, differ- entiation, vascular invasion, Her-2/neu, p53, Ki-67 ex- pression), the most important are the Her-2/neu status, tumor size more than 3 cm, vascular invasion, p53 status, low differentiation of the tumor [9]. We also investigated the prognostic significance of such molecular factors as histological form and tumor differentiation, Her-2/neu, p53, Ki-67 expression. None of these prognostic factors in the forecasting models is important. Perhaps, it is be- cause of a different set of factors analyzed. In another study 60 morphological factors in 300 patients with ad- vanced NSCLC were examined before the first-line chemotherapy. Prediction and prognostic markers are as follows: general status of patients, extra pulmonary dis- tant metastases, type of chemotherapy, number of leuko- cytes in the blood, level of albumin CYFRA 21-1, nu- cleosome, Ca125, Ca15-3 and Ca72-4 [10]. In our study 39 prognostic markers were investigated in 254 patients. It is noted that the independent prognostic factors in the forecasting model are: the BMI, histological type of tu- mor, stage, type of surgery which had been conducted. The possible alternative combination of factors for pre- dicting the survival rate is as follows: the BMI, volume of lymph dissection, EGFR expression. Another important factor in the opinion of Park and coauthors (2011) is the presence of necrosis in the pri- mary tumor. It is noted that the tumor necrosis is an in- Open Access JCT
 Forecasting of Survival Rate in Patients with the Early Stage of Non Small Cell Lung Cancer 1476 dependent prognostic factor. Thus, the five-year survival rate of patients with absence of necrosis was 94.8% and 86.2% in patients with necrosis (p = 0.04). Five-year recurrence-free survival rate in the absence of necrosis was 92.1% and in patients with tumor necrosis–78.9% (p = 0.016) [4]. In our study the tumor invasion in visceral pleura was investigated (except the presence of necrosis in the primary tumor). None of these factors was associ- ated with survival rate of patients. One of the most important properties of the tumor is proliferation controlled by a group of cyclines. In their study (2001) Dosaka-Akita H. and coauthors noted the different prognostic role of cycline D1 and cycline E. The cycline E correlated with the index of proliferation (measured by Ki-67). The cycline E correlated with the survival rate [16]. According to the data of Lu and coau- thors (2004) DAPK methylation and the low IL-10 ex- pression are markers of the negative prognosis for pa- tients [8]. Rubio L. and coauthors (2005) investigated the prognostic role of clinical factors such as factor 8 (mi- crovascular density), VEGF, iNOS, p53, p21. The sig- nificant prognostic factors were: tumor size (p = 0.0063), angiogenesis (p = 0.0271) and p21 (p = 0.0478). The authors indicate that the developed prognostic Cox- model allows more careful identifying of the patients with a high risk of disease progression [17]. Among the molecular markers the following markers were studied for constructing the prognostic model: erb-b2, р53, bcl2, Ki-67, Cd-44. The multivariate analy- sis shows the prognostic role of the following factors: apoptosis (p53), angiogenesis (factor 8), growth factors (erb-b2), adhesion (Cd-44) and the regulation of the cell cycle (recessive gene retinoblastoma) [2,14]. At the same time Hilbe and coauthors (2003) in the study of the prognostic role of growth factors (EGFR or c-erbb-1, c-erbb-2 and c-erbb-3), metastases inhibition parameters (Cd82), markers of proliferation (Ki67, p120) and mark- ers of apoptosis (p53, bcl-2) did not reveal any relation to the survival rate of any marker. However, when combin- ing the factors and survival analysis of patients with high expression of two or three factors (c-erbb-3, p53, and micro vascular density) noted significant deterioration of survival rate [6]. In our research, among the investigated molecular markers were: Ki-67 expression, Ki-67 ex- pression (categorized index), CD31/CD34 expression, Her2-neu expression, EGFR expression, p53 expression, E-kadherinu expression, expression of pan-cytokeratin. However, only the EGFR expression in the Model 2 plays an important prognostic role. The use of the PCR reaction allows analyzing the prognostic significance of various genes. Thus, Raz and coauthors (2008) analyzed the genes Wnt3a, Erbb3, LSK and Rnd3. All the patients were divided into two groups: patients with high and low risk of disease progression. Five-year survival rate had 41% of the patients with the high risk progression and 62% of the patients with the low risk of it [7]. 5. Conclusion In connection with the uncertainty of the “ideal” predic- tion factors and their combinations, further large studies are necessary to be done [8,14]. Some authors have shown that the use of markers combinations is more beneficial and prognostic than the use of one of them [16]. Therefore, the models developed by us include a num- ber of significant predicting factors that can be used to predict unfavorable survival rate for patients with the early-stage of NSCLC which will help identify patients for further treatment after the operation. REFERENCES [1] D. T. Chen, Y.-L. Hsu, W. J. Fulp, et al., “Prognostic and Predictive Value of a Malignancy-Risk Gene Signature in Early-Stage Non-Small Cell Lung Cancer,” Journal of the National Cancer Institute, Vol. 103, No. 24, 2011, pp. 1859-1870. http://dx.doi.org/10.1093/jnci/djr420 [2] T. A. D’Amico, M. Massey, J. E. Herndon, et al., “A Biologic Risk Model for Stage I Lung Cancer: Immuno- histochemical Analysis of 408 Patients with the Use of Ten Molecular Markers,” The Journal of Thoracic and Cardiovascular Surgery, Vol. 117, No. 4, 1999, pp. 736- 743. http://dx.doi.org/10.1016/S0022-5223(99)70294-1 [3] K. Konopa, “Do We Have Markers to Select Patients for Adjuvant Therapies of Non-Small-Cell Lung Cancer?” Annals of Oncology, Vol. 21, No. 7, 2010, pp. 199-202. [4] S. Y. Park, H. S. Lee, H. J. Jang, et al., “Tumor Necrosis as a Prognostic Factor for Stage IA Non-Small Cell Lung Cancer,” The Annals of Thoracic Surgery, Vol. 91, No. 6, 2011, pp. 1668-1673. http://dx.doi.org/10.1016/j.athoracsur.2010.12.028 [5] B. A. Williams, H. Sugimura, Ch. Endo, et al., “Predict- ing Postrecurrence Survival Among Completely Resected Nonsmall-Cell Lung Cancer Patients,” The Annals of Thoracic Surgery, Vol. 81, No. 3, 2006, pp. 1021-1027. http://dx.doi.org/10.1016/j.athoracsur.2005.09.020 [6] W. Hilbe, S. Dirnhofer, F. Oberwasserlechner, et al., “Im- munohistochemical Typing of Non-Small Cell Lung Cancer on Cryostat Sections: Correlation with Clinical Parameters and Prognosis,” Journal of Clinical Pathology, Vol. 56, No. 10, 2003, pp. 736-741. http://dx.doi.org/10.1136/jcp.56.10.736 [7] D. J. Raz, M. R. Ray, J. Y. Kim, et al., “A Multigene Assay Is Prognostic of Survival in Patients with Early- Stage Lung Adenocarcinoma,” Clinical Cancer Research, Vol. 14, No. 17, 2008, pp. 5565-5570. http://dx.doi.org/10.1158/1078-0432.CCR-08-0544 [8] C. Lu, J.-Ch. Soria, X. Tang, et al., “Prognostic Factors in Resected Stage I Non-Small-Cell Lung Cancer: A Multi- variate Analysis of Six Molecular Markers,” Journal of Clinical Oncology, Vol.22, No. 22, 2004, pp. 4575-4583. Open Access JCT
 Forecasting of Survival Rate in Patients with the Early Stage of Non Small Cell Lung Cancer Open Access JCT 1477 http://dx.doi.org/10.1200/JCO.2004.01.091 [9] D. H. Harpole, Jr. J. E. Herndon, W. G. Wolfe, et al., “A Prognostic Model of Recurrence and Death in Stage I Non-Small Cell Lung Cancer Utilizing Presentation, His- topathology, and Oncoprotein Expression,” Cancer Re- search, Vol. 55, No. 1, 1995, pp. 51-56. [10] S. Holdenrieder, D. Nagel, V. Heinemann, et al., “Predic- tive and Prognostic Biomarker Models in Advanced Lung Cancer,” Journal of Clinical Oncology, Vol. 26, No. 15S, 2008, p. 19010. [11] A. Lopez-Encuentra, Lopez-Rios, E. Conde et al., “Com- posite Anatomical-Clinical-Molecular Prognostic Model in Nonsmall Cell Lung Cancer,” European Respiratory Journal, Vol. 31, No. 7, 2011, pp. 136-142. http://dx.doi.org/10.1183/09031936.00028610 [12] A. Petrie, C. Sabin, “Medical Statistics at a Glance,” In: Petrie A., Sabin C., Eds., Medical Statistics at a Glance, 2nd Edition, Blackwell Publishing, Malden, 2005. [13] S. Haykin, “Neural networks. A Comprehensive Founda- tion,” In: Haykin Simon, Ed., Neural Networks. A Com- prehensive Foundation, 2nd Edition, Prentice Hall, Upper Saddle River, 1999. [14] C. Q. Zhu, W. Shih, C. H Ling and M. S. Tsao, “Immu- nohistochemical Markers of Prognosis in Non-Small Cell Lung Cancer: A Review and Proposal for a Multiphase Approach to Marker Evaluation,” Journal of Clinical Pa- thology, Vol. 59, No. 8, 2006, pp. 790-800. http://dx.doi.org/10.1136/jcp.2005.031351 [15] L. R. Leonardus, van der Pijl, O. Birim, et al., “Validation of a Prognostic Model to Predict Survival after Non- Small-Cell Lung Cancer Surgery,” European Journal of Cardio-Thoracic Surgery, Vol. 38, No. 5, 2010, pp. 615- 620. http://dx.doi.org/10.1016/j.ejcts.2010.03.028 [16] H. Dosaka-Akita, F. Hommura, T. Mishina, et al., “A Risk-Stratification Model of Non-Small Cell Lung Can- cers Using Cyclin E, Ki-67, and rasp21: Different Roles of G1 Cyclins in Cell Proliferation and Prognosis,” Can- cer Research, Vol. 61, 2001, pp. 2500-2504. [17] L. Rubio, F. J. Vera-Sempere, J. A. Lopez-Guerrero, et al., “A Risk Model for Non-Small Cell Lung Cancer Using Clinicopathological Variables, Angiogenesis and Onco- protein Expression,” Anticancer Research, Vol. 25, No. 1B, 2005, pp. 497-504. Abbreviations ANN—Artificial Neural Network AUC—Area under the Curve BMI—Body Mass Index CI—Confidence Interval CYFRA—Serum Cytokeratin Fragment DAPK—Death-Associated Protein Kinase EGFR—Epidermal Growth Factor Receptor GA—Genetic Algorithm IL—Interleukin iNOS—Inducible Nitric Oxide Synthase NSCLC—Non-Small Cell Lung Cancer OR—Odds Ratio PCR—Polymerase Chain Reaction PCT—Prognosis for Conducting the Chemotherapy ROC—Receiver Operating Characteristic VEGF—Vascular Endothelial Growth Factor
|