 American Journal of Industrial and Business Management, 2013, 3, 645-654 Published Online December 2013 (http://www.scirp.org/journal/ajibm) http://dx.doi.org/10.4236/ajibm.2013.38074 Open Access AJIBM 645 Interactions between House Prices, Stock Prices and Monetary Policy—Using Recursive VAR Chun-Chang Lee1, Chih-Min Liang2, Wen-Hui Wu1, Shu-Man You3 1Department of Real Estate Management, National Pingtung Institute of Commerce, Pingtung, Taiwan; 2Department of Public Fi- nance and Tax Administration, National Taipei Institute of College of Business, Taipei, Taiwan; 3Department of Real Estate and Built Environment, Natioanl Taipei University, New Taipei City, Taiwan. Email: lcc@npic.edu.tw, ljimmy.ljimmy@msa.hinet.net, winnie0177@hotmail.com, youshuman@gmail.com Received July 21st, 2013; revised August 21st, 2013; accepted August 29th, 2013 Copyright © 2013 Chun-Chang Lee et al. This is an open access article distributed under the Creative Commons Attribution License, which permits unrestricted use, distribution, and reproduction in any medium, provided the original work is properly cited. In accor- dance of the Creative Commons Attribution License all Copyrights © 2013 are reserved for SCIRP and the owner of the intellectual property Chun-Chang Lee et al. All Copyright © 2013 are guarded by law and by SCIRP as a guardian. ABSTRACT This paper uses a recursive VAR model to analyze the effects of monetary policy shock and the role of house price and stock price shocks. The data come from the first quarter of 1993 to the second quarter of 2010 in Taiwan. There is em- pirical evidence suggesting that house prices and stock prices do not play the role of a transmission mechanism for monetary policy shocks. When house prices increase, stock prices undergo a simultaneous and significant increase, but this effect gradually disappears. As house prices increase, the interest rate is simultaneously and positively affected; this effect is insignificant until after the second quarter. As stock prices increase, house prices are positively affected, which is consistent with the sign of the expected value, but the effect is insignificant. Under increasing stock prices, the inter- est rate simultaneously increases, and this effect is significant during the third and tenth quarters. Keywords: Asset; House Prices; Stock Prices; Monetary Policy; Recursive VAR 1. Introduction The subprime crisis and a series of financial crises in the US highlight the importance of asset prices as a trans- mission mechanism for monetary policy changes. This is primarily due to the central collateral role played by asset prices such as house prices. Asset prices not only affect the capital of financial institutions but also re- sult in changes in overall corporate investment and private consumption patterns, making these a source of macroeconomic fluctuations. Bjørnland and Jacobsen [1] suggested that central banks have managed to keep inflation in check through inflation targeting, yet they have not managed to prevent asset prices from burst- ing and having real negative effects. A report by the International Monetary Fund [2] noted that monetary policy-makers should pay more attention to the overall financial risk caused by the bursting of real property bubbles. Due to the role played by the wealth storage of assets, the impact of asset prices should be carefully assessed in times of disturbance. Asset prices can simultaneously respond to monetary policy and thus become an important shock transmission mechanism [3-5]. Hence, because of their timely response to economic shocks, asset prices may be important indicators of the status of monetary policy. An analysis of the role played by asset prices as a transmission mechanism of monetary policy can help to understand whether monetary policy can effectively achieve its goals. Unlike other assets, houses have the dual role of being a wealth storage mechanism as well as an important durable consumption good. Changes in house prices can affect the wealth of households. According to the Tobin Q theory, when the value of a pledge rises, the rising house price will stimulate housing construction activities . House price shock may affect real output and commodity price, making it an important forward-looking variable. Hence, monetary policymakers will monitor house prices. House prices can affect housing market development, capital flows and prices in other markets. Past research on the role house prices play in the overall economy has often neglected the effect of other asset prices, leading to
 Interactions between House Prices, Stock Prices and Monetary Policy—Using Recursive VAR 646 errors in the empirical results. Case et al. [6] noted that the stock market and housing market have an important effect on macroeconomic activity in a mature economy. Hence, this study will also explore the role of stock price shocks. When estimating the relationships between macro- economic variables, VAR (vector autoregression) analy- sis, including three types of reduced form VAR, re- cursive VAR, and SVAR (structural VAR), is often applied. The reduced form VAR is a VAR model with- out a priori limits that can perform an empirical study without relying on any economic theory. Although it is very convenient for analyzing the short-term dynamic re- lationships between variables, the over-parameterization of results in the VAR model reduce the estimation ef- ficiency of the model, and the long-term relationships between economic variables cannot be explained. Bernanke and Blinder [7] noted that it is highly risky to use the VAR model without limits in structural inference. Each variable of the reduced form VAR model is a linear function composed of the past value of the variable, the past values of other variables, and errors, without con- sidering the current impact in between variables. The regression errors of the reduced form VAR are correlated with the contemporaneous term, making it difficult to identify the structural impact. Comparatively, there is no correlation between the recursive VAR structural errors. As IRF (impulse reaction function) requires no cor- relation between shocks, the recursive VAR model is also frequently used to illustrate the dynamic impact of specific variables on the endogenous variables. When using a recursive VAR model to measure the structural effects caused by policy changes, the ordering of monetary policy variables and other economic va- riables should be conducted. The contemporaneous impact between variables is of recursive form [8-9]. The order of model endogenous variables is subject to the contemporaneous relationship between monetary policy and other economic variables. Compared with SVAR, the identification of recursive VAR is simpler and more straightforward. This paper uses recursive VAR as the model setting approach. Because monetary policy may affect asset prices (stock and house prices) via the interest rate chan- nel, this model illustrates the interactive relationship be- tween asset price and monetary policy. When setting the empirical model, this study also considers the potential impact of asset prices on monetary policy to understand the short-term interaction between monetary policy and asset prices. The proposed model is a traditional closed economy system model, which consists of macroeco- nomic variables for monetary policy and asset prices to explain the effect of changes in monetary policy and the role of house and stock price shocks. 2. Empirical Model Setting First, t is assumed to represent the stationary variable vector in the macroeconomic respect : y 51 ln, ln, ln, ln, tt yGDPCPIHPSTOCKRATE ; the endogenous variables in brackets represent output, con- sumer price index, house prices, stock prices and interest rate, respectively. If the VAR presentation equation of t y is tt Ly , 0 0 , pj j j LL I , and L is an invertible matrix, then it can be written into the equation of the moving average as follows: , t yBL t (1) where t is a 51 vector of reduced form residuals assumed to be identically and independently distributed, tiid 0, , with a positive definite covariance matrix . BL is the 55 convergent matrix polyno- mial in the lag operator L, 0 j j BL BL . Following the literature, the innovations t are assumed to be written as linear combinations of the underlying or- thogonal structure disturbances t e, i.e., tt Se . The VAR can then be written in terms of structural shocks in the following manner: 1 tt yBLSS CLe t (2) where CL BLS. If S is identified, we can derive the MA representation in (2) as is calculated from a reduced form estimation. To identify S, the et’s are normalized so that they all have unit variance. The normalization of BL ecov t implies that SS . Be- cause the model contains five variables, the simultaneous matrix S can identify as many as 15 parameters. There- fore, the matrix S should be added with some restrictions to identify structural disturbances. With a five-variable VAR, we can identify five struc- tural shocks, namely, the output shocks , the consumer price index shocks , house price shocks ln GDP t e ln CPI t e ln P t e, stock price shocks and monetary policy shocks ln STOCK t e P t e. We then order the vector of structural shocks as follows: lnlnln ln ,,, , GDPCPIHPSTOCK MP tttt tt eee eee This paper attempts to establish a recursive VAR model and set constraints for the simultaneous variables, ten of which are set to zero. No variable is assumed to be affected by the variable that follows it in the contempo- raneous term. Hence, in the recursive VAR model, dif- ferences in the ordering of variables will affect the em- Open Access AJIBM
 Interactions between House Prices, Stock Prices and Monetary Policy—Using Recursive VAR 647 pirical results as well as the degree of response to shocks. The variables in the model are ordered as follows: gross domestic product and consumer price index are the proxy macroeconomic variables; asset price is represented by the Sinyi house price index and the weighted average of the stock prices; finally, the weighted average lending interest rate is the proxy variable of monetary policy. The reason for such ordering is illustrated below. This paper bases the limits set on simultaneous vari- ables on past literature. Using the rules on implementing monetary policies set by the Central Bank, Rotemberg and Woodford [10] established a model with the interest rate as a function of the output and inflation. Bjørnland and Jacobsen [1] argued that macroeconomic variables do not simultaneously react to policy variables, while the simultaneous reaction from the macroeconomic envi- ronment to policy variables is allowed for. Hence, the output in the first column and inflation in the second column are ranked higher than interest rate (as shown in Equation (3)). The output represents the aggregate de- mand relationship and inflation stands in for the aggre- gate supply relationship. The two columns represent the equation of commodity market equilibrium [9-10]. The third column is the equation of the house prices. As noted by Bjørnland and Jacobsen [1], house prices contribute greatly to the macroeconomic variables and the interest rate, indicating the importance of including house prices in the model. In other words, the unexpected rise of house prices will promote short-term consumption and output growth, which results in inflation, thereby affecting monetary policy. The fourth column is the equation of stock prices. As noted by Case et al. [6], stock market, macroeconomic factors and the housing market are often correlated in a mature economic system. Regarding stock and housing markets, assets of different types have apparent price spillovers [2], suggesting that booming transactions will result in rising house prices, which trigger stock price fluctuations in times of a booming housing market. In the monetary policy equation represented in the fifth column, the real GDP, CPI, house prices and stock prices are ranked ahead of monetary policy, indicating that the macroeconomic variables have a contemporane- ous effect on the interest rate given the constraints on simultaneous variables and assuming that “monetary policy has no simultaneous impact on economic vari- ables.” Svensson [11] stressed that monetary shocks have no effect on output or inflation in the monetary policy transmission mechanism. However, changes in mac- roeconomy occur simultaneously with monetary policy shifts. Therefore, Bjørnland and Jacobsen [1] established a VAR model in 2010 that ranks output, inflation, and house prices ahead of interest rate. Therefore, we define the model as follows: ln 11 ln 21 22 ln 31 3233 ln 41 424344 51 52535455 0000 000 00 0 GDP tCPI tHP ttSTOCK tMP t Se SS e SSS e SSSS e SSSSS e (3) 3. Data Source and Description First, we describe the empirical data source and the data processing of the variables at their original value levels. We then describe the decomposed sequence diagram by Hodrick-Prescott as well as the unit root test results. 3.1. Data Source This paper sources 70 batches of quarterly empirical data from the period of the first quarter in 1993 to the second quarter in 2010. The five variables of the empirical model include: the natural logarithm of the real GDP after quarterly adjustment, the natural logarithm of CPI after quarterly adjustment, the natural logarithm of the Sinyi house price index, the natural logarithm of the weighted average stock prices and the weighted average lending interest rate. The real GDP and CPI data are taken from the Taiwan Economic Journal (TEJ) Database; the weighted average stock price index data are taken from the Taiwan Stock Exchange; and the weighted average lending interest data are based on the published data of the Central Bank. The data on house prices in this paper is represented by the Sinyi house price index. The index is compiled using hedonic pricing theory to remove problems of heterogeneity (excluding biased samples such as aged houses). The Sinyi house price index covers mainly the market of old and middle-aged houses (including apart- ment buildings, buildings with elevators and excluding pre-sale houses). The index is a relatively credible house price index based on publicly available information. The variables and the data source used in this study are shown in Table 1. 3.2. Data Processing Before conducting the empirical study, we use the unit root test approach to test whether the variables included in the analysis are stationary to avoid the problem of “spurious regression.” This study uses the ADF and KPSS approaches to test whether the time series is stationary. If the time series is not stationary, the stationary test will be conducted after Hodrick-Prescott decomposition. The Hodrick-Prescott decomposition can decompose the non-stationary time series data into stationary and non-stationary parts. The empirical study of this paper uses the stationary part after the Hodrick- Open Access AJIBM
 Interactions between House Prices, Stock Prices and Monetary Policy—Using Recursive VAR 648 Prescott decomposition as the data for the empirical analysis. According to the original level sequence for the vari- ables shown in Figure 1 and the unit root test results of the original levels shown in Table 2, the original se- quences of lnGDP, lnCPI, lnHP all have unit roots indi- cating a non-stationary time series. Hence, we conduct the unit root test after the Hodrick-Prescott decomposi- tion. According to the results from the unit root test con- ducted after Hodrick-Prescott decomposition and shown in Tab le 3, both the ADF test and the KPSS test confirm that real GDP, CPI and Sinyi house price index data be- come stationary time series data after the Hodrick-Pres- cott decomposition. 4. Data Source and Description In time series data, the choice of a lagging period is closely related to stability. More lagging periods in the model will result in more parameters to be estimated and fewer degrees of freedom. Hence, there should be a bal- ance between lagging periods and degrees of freedom. The lag order of the model is determined using LR (like- lihood ratio), AIC (Akaike Information Criterion), SC (Schwarz Criterion), Hannan-Quinn Information Criteria, and FPE (Final Prediction Error Criterion) for model reductions. The tests suggested that one lag is acceptable. We therefore set the VAR model lag as 1 for estimation. Table 1. Variable and data description. Variable Description Data Source lnGDP Natural logarithm of real GDP TEJ Database lnCPI Natural logarithm of CPI TEJ Database lnHP Natural logarithm of Sinyi house price index Sinyi Real Estate Agency lnSTOCK Natural logarithm of weighted average stock price index Taiwan Stock Exchange corporation RATE Weighted average lending interest rate Central Bank of Taiwan Table 2. Unit root test of the original levels of the variables. Variables ADF KPSS lnGDP −1.12 1.10*** lnCPI −2.80* 1.01*** lnHP 0.56 0.38* lnSTOCK −3.35** 0.17 “***”, “**”, “*”represent significance at the 1%, 5% and 10% levels, re- spectively; the ADF testing rejects the null assumption of unit root; and KPSS testing rejects the null assumption of no unit root. Table 3. Unit root test results after Hodrick-Prescott de- composition. Variables ADF KPSS ln GDP −4.53*** 0.04 ln CPI −2.97** 0.08 ln P −4.44*** 0.08 “***”, “**”, “*” represent significance at the 1%, 5% and 10% levels, re- spectively; the ADF testing rejects the null assumption of no unit root; and KPSS testing rejects the null assumption of unit root. 4.1. Effects of Monetary Policy Shocks By the analysis of IRF as set by the theoretical model, we can explore whether a change of one standard deviation of a certain variable has a positive, negative, continuous or sporadic effect on other variables. The IRF of a stable model should be close to 0. Figures 2-4 illustrate the IRFs of different structural shocks on the variables. As past studies have indicated, contractionary mone- tary policy shocks often affect output and inflation. An unexpected rise in interest rates, though temporary, re- sults in lowered output and inflation [1]. Figure 2(a) illustrates that, given an increase of one standard devia- tion (approximately 0.13%) in the interest rate, the GDP responds positively, at first, then negatively, after the sixth quarter. The effect, however, is not significant. The results suggest that the expected contractionary monetary policy shocks will have a negative effect on employment and salary. According to a study by Elbourne and de Haan [10], among EU countries, interest rate shocks have a negative impact on industrial production in all coun- tries except Italy, and the effect has not been significant in most cases. These findings are consistent with the em- pirical outcomes of this study. Figure 2(b) illustrates that after one standard deviation increase in interest rate, CPI unexpectedly responds positively, but the effect is not significant. Goux and Cordahi [12] used the recursive VAR to discuss the transmission mechanism of inter- national monetary policy and found that CPI and short- term interest rate are positively, though not significantly, correlated. The contractionary monetary policy does not result in a lowering of the CPI but in an increase in commodity prices, and this phenomenon is generally known as the price puzzle. This puzzle may be explained by a cost channel of interest rate, where an increase in firms borrowing costs is offset by an increase in prices [11,13]. As shown in Figure 2(c), after an increase of one standard deviation in the interest rate, house prices re- spond positively in the first four quarters, but the effect is not significant. One possible reason for this might be the fact that construction companies transfer part of the cost o consumers to trigger an increase in house prices in the t Open Access AJIBM
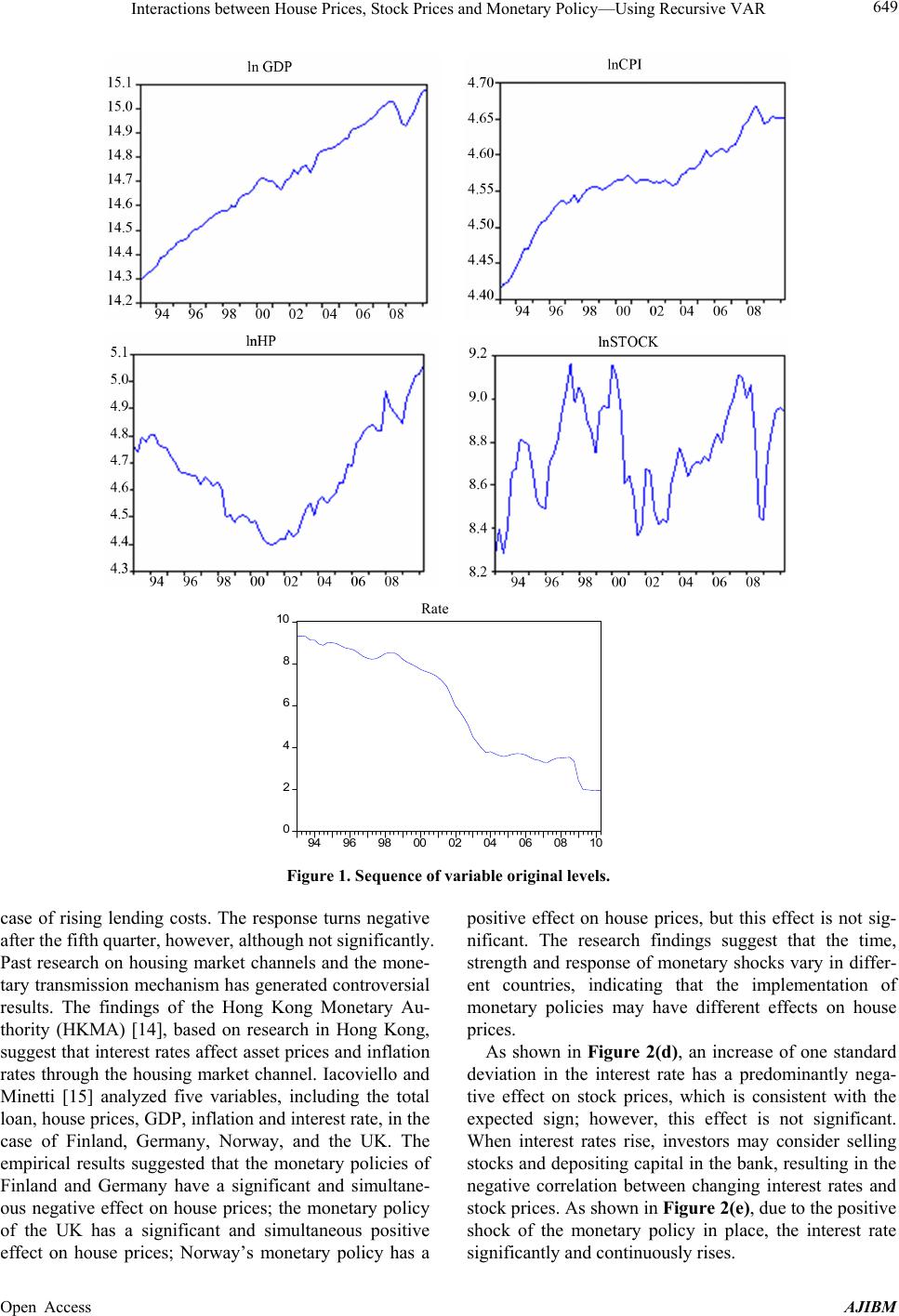 Interactions between House Prices, Stock Prices and Monetary Policy—Using Recursive VAR Open Access AJIBM 649 0 2 4 6 8 10 94 96 98 00 02 04 06 08 10 Rate Figure 1. Sequence of variable original levels. case of rising lending costs. The response turns negative after the fifth quarter, however, although not significantly. Past research on housing market channels and the mone- tary transmission mechanism has generated controversial results. The findings of the Hong Kong Monetary Au- thority (HKMA) [14], based on research in Hong Kong, suggest that interest rates affect asset prices and inflation rates through the housing market channel. Iacoviello and Minetti [15] analyzed five variables, including the total loan, house prices, GDP, inflation and interest rate, in the case of Finland, Germany, Norway, and the UK. The empirical results suggested that the monetary policies of Finland and Germany have a significant and simultane- ous negative effect on house prices; the monetary policy of the UK has a significant and simultaneous positive effect on house prices; Norway’s monetary policy has a positive effect on house prices, but this effect is not sig- nificant. The research findings suggest that the time, strength and response of monetary shocks vary in differ- ent countries, indicating that the implementation of monetary policies may have different effects on house prices. As shown in Figure 2(d), an increase of one standard deviation in the interest rate has a predominantly nega- tive effect on stock prices, which is consistent with the expected sign; however, this effect is not significant. When interest rates rise, investors may consider selling stocks and depositing capital in the bank, resulting in the negative correlation between changing interest rates and stock prices. As shown in Figure 2(e), due to the positive shock of the monetary policy in place, the interest rate significantly and continuously rises.
 Interactions between House Prices, Stock Prices and Monetary Policy—Using Recursive VAR 650 Figure 2. Response to a monetary policy shock. The empirical results from the monetary policy shocks analysis do not support a transmission role for house and stock prices. A possible reason for this might be low in- terest rates. During the period from the first quarter of 1993 to the second quarter of 2010, the average interest rate was 3.5%, and it decreased to less than 3% after the fourth quarter of 2001. Such low interest rates have no significant impact on investment and consumption lend- ing costs, making monetary policy ineffective. Secondly, the sources of capital in investment and consumption may be channels other than the banking system. Lai [16] also uses the recursive VAR model to discuss possible transmission channels for Taiwan’s monetary policy. The empirical results suggest that, except for its effect on housing investment, contractionary monetary policies have no effect on consumption costs for durable goods, such as machinery and equipment for enterprises, imply- ing that enterprises may have other capital source chan- nels. Hence, a possible reason for the inability of house prices and stock prices to transmit monetary policy shocks is low interest rates and alternative capital source hannels. c Open Access AJIBM
 Interactions between House Prices, Stock Prices and Monetary Policy—Using Recursive VAR 651 Figure 3. Response to a house price shock. As the variance decomposition shown in Table 4 sug- gests, the monetary policy shock has very low explana- tory power on changes in house and stock prices as the interest rates in Taiwan have been relatively low for a long time. Extremely low lending costs make interest rates an insignificant factor in housing and stock invest- ment. Thus the role of stock and housing markets as a transmission mechanism for monetary policy shocks can- not be determined. 4.2. The Role of House Price Shocks Drawing from the analysis of the effects of monetary policy shock, the discussion of the effect of house price shock on the relevant variables is organized as follows. As shown in Figures 3(a) and (b), an increase of one standard deviation (an approximately 0.029 unit) in house price, generates a positive but not significant change in GDP and CPI [17,18]. As shown in Figure 3(c), an increase of one standard deviation in house price generates a simultaneous, sig- nificant, and positive increase in stock prices, reaching a peak value of an approximately 0.05 unit1 in the third quarter. After the fifth quarter, this effect gradually dis- appears. As expected, changes in house prices positively Table 4. Variance decomposition. Contributions from monetary policy shocks to ln GDP, ln CPI, ln HP and ln STOCK. Term lnGDP lnCPI lnHP LnSTOCK 1 0.000 0.000 0.000 0.000 2 0.001 0.002 0.000 5.92E-07 3 0.001 0.006 0.000 6.73E-05 4 0.001 0.014 0.000 0.000 5 0.001 0.022 0.000 0.001 12 0.011 0.059 0.006 0.027 24 0.029 0.098 0.014 0.076 affect the contemporaneous stock prices, confirming that different types of assets exhibit price spillovers [2]. As shown in Figure 3(d), an increase of one standard deviation in house price results in a positive but not sig- nificant change in the interest rate. This positive effect appears after the second quarter and is significant until the eleventh quarter. The relevant literature on this phe- nomenon has noted that the effect of house prices on interest rate is positive (see [5,9]). Our research findings are consistent with Bjørnland and Jacobsen [1], confirm- ng that house price shocks can respond simultaneously i 1Elasticity is 0.05/0.029 = 1.724. Open Access AJIBM
 Interactions between House Prices, Stock Prices and Monetary Policy—Using Recursive VAR 652 Figure 4. Response to a stock price shock. to monetary policy changes in Sweden and Britain. In Norway, the initial effect on house prices is insignificant, but after two quarters, house prices increase 10 basis points. The strength and timing of the response thereafter varies from one country to another, indicating that hous- ing may play a different role in monetary policy setting. Bjørnland and Jacobsen [1] noted that the house price shock can result in changes in the interest rate within one year. The results in this paper confirm these results. The variance decomposition as illustrated in Table 5 suggests that, the house price shock explains approxi- mately 11% - 18% of the variance in stock price and ap- proximately 6% - 21% of the variance in interest rate after the second period. The explained variance in stock price and interest rate gradually increases. House price shock explains approximately 5% of the variance in GDP and CPI after the 12th period. These results demonstrate the importance of house price shock to stock prices and the interest rate. 4.3. The Role of Stock Price Shocks As shown in Figure 4(a), an increase of one standard deviation (an approximately 0.1 unit) in stock price, re- sults in a simultaneous and significant increase in GDP, Table 5. Variance decomposition. Contri butions from house prices shocks to ln GDP, ln CPI, ln STOCK, and RATE. Period lnGDP lnCPI lnSTOCK RATE 1 0.000 0.000 5.379 3.235 2 0.795 0.111 11.693 6.326 3 2.148 0.516 15.469 9.280 4 3.411 1.307 17.418 11.795 5 4.245 2.378 18.286 13.825 12 4.721 5.843 18.233 19.705 24 4.799 5.844 18.214 21.357 reaching a peak value of 0.004 unit2 after the fourth quarter of stock price shock and gradually disappearing. As shown in Figure 4(b), after an increase of one stan- dard deviation in stock price, CPI increases significantly in the fifth quarter, and the period of significant increase lasts for four quarters, from the fifth to the ninth quarter. As shown in Figure 4(c), an increase of one standard deviation in stock price results in an increase in house price, but this effect is not significant. As shown in Fig- 2Elasticity = 0.004/0.1 = 0.04. Open Access AJIBM
 Interactions between House Prices, Stock Prices and Monetary Policy—Using Recursive VAR 653 ure 4(d), an increase of one standard deviation in stock price results in a simultaneous, significant increase in the interest rate from the third to the tenth quarter, reaching a peak value of approximately 0.15 units in the ninth quar- ter after the shock. From the perspective of point estima- tion, the effect seems to be permanent, but it becomes insignificant after the tenth quarter. The variance decomposition illustrated in Table 6 suggests that the variance in GDP, CPI, house price and interest rate attributable to the stock price shock is low at first but gradually increases with time. The ranking by explanatory variance is interest rate, GDP, CPI and house price, indicating that stock price shocks have a significant long-term impact on interest rate, GDP and CPI. 5. Conclusions and Suggestions This study uses a recursive VAR model to analyze the effect of monetary policy shocks and the role of house price and stock price shocks. The empirical results suggest that contractionary monetary policy shock has no significant impact on GDP, CPI, house prices and stock prices. The role of house prices and stock prices as a transmission mechanism for monetary policy shock cannot be confirmed. The findings on the effect of house price changes suggest that stock prices will be simultaneously and positively affected when house prices increase but that this effect gradually disappears after the fifth quarter. Increases in house prices result in simultaneous increases in the interest rate, and this effect becomes significant after the second quarter and lasts until the eleventh quarter. These results are consistent with Bjørnland and Jacobsen [1]. The results from the stock price shock analysis suggest that GDP significantly increases with increases in stock price, but this effect lasts only until the fifth quarter. Increases in stock prices positively and significantly affect CPI. An effect lasts for four quarters from the fifth to the ninth quarter. House prices are Table 6. Variance decomposition. Contributions from stock price shocks to lnGDP, lnCPI, lnHP, and RATE. Term lnGDP lnCPI lnHP RATE 1 0.000 0.000 0.000 0.078 2 2.651 0.056 1.074 1.364 3 6.491 0.656 2.590 4.694 4 9.676 2.193 3.742 8.673 5 11.564 4.451 4.327 12.358 12 12.314 11.454 4.678 22.449 24 12.438 11.436 4.738 23.961 positively affected by increases in stock prices, but this effect is not significant. As stock prices increase, the interest rate simultaneously increases, and this effect is significant from the third to the tenth quarter, becoming insignificant after the tenth quarter. This analysis in this paper assumed a traditional closed economic system. However, because Taiwan is a small open economy, the interaction between exchange rate and monetary policy might be significant, as previous studies suggest [19-21]. Exchange rates and international crude oil prices may be added as the foreign economic variables. Given that mainland China, the US and Taiwan are closely related in terms of economy, future studies may wish to include data on China and the US in the analysis. Additionally, this paper uses the weighted average lending rate as a proxy variable for monetary policy. However, the weighted average lending rate published by the Central Bank is not entirely subject to the control of monetary policy designed to regulate supply and demand in capital markets. Hence, future studies may wish to use monetary supply or non-borrowed money supply as a proxy vari- able for monetary policy. REFERENCES [1] H. C. Bjørnland and D. H. Jacobsen, “The Role of House Prices in the Monetary Policy Transmission Mechanism in Small Open Economies,” Journal of Financial Stability, Vol. 6, No. 4, 2010, pp. 218-229. http://dx.doi.org/10.1016/j.jfs.2010.02.001 [2] International Monetary Fund, “Lessons for Asset Price Fluctuations Form Monetary Policy,” World Economic Outlook, 2009, pp. 93-120. [3] R. Rigobon and B. Sack, “The Impact of Monetary Policy on Asset Prices,” Journal of Monetary Economics, Vol. 51, No. 8, 2004, pp. 1553-1575. http://dx.doi.org/10.1016/j.jmoneco.2004.02.004 [4] J. Zettelmeyer, “The Impact of Monetary Policy on the Exchange Rate: Evidence from Three Small Open Economies,” Journal of Monetary Economics, Vol. 51, No. 3, 2004, pp. 635-652. http://dx.doi.org/10.1016/j.jmoneco.2003.06.004 [5] B. S. Bernanke and K. N. Kuttner, “What Explains the Stock Market’s Reaction to Federal Reserve Policy?” The Journal of Finance, Vol. 60, No. 3, 2005, pp. 1221-1257. [6] K. E. Case, J. M. Quigley and R. J. Shiller, “Comparing Wealth Effects: The Stock Market Versus the Housing Market,” Advances in Macroeconomics, Vol. 5, No. 1, 2005, pp. 1-34. http://dx.doi.org/10.2202/1534-6013.1235 [7] B. S. Bernanke and A. S. Blinder, “The Federal Funds Rate and the Channels of Monetary Transmission,” Ameri- can Economic Review, Vol. 82, No. 4, 1992, pp. 901-921. [8] N. Girouard and S. Blöndal, “House Prices and Economic Activity,” OECD Economics Department Working Pa- pers, No. 279, 2001. Open Access AJIBM
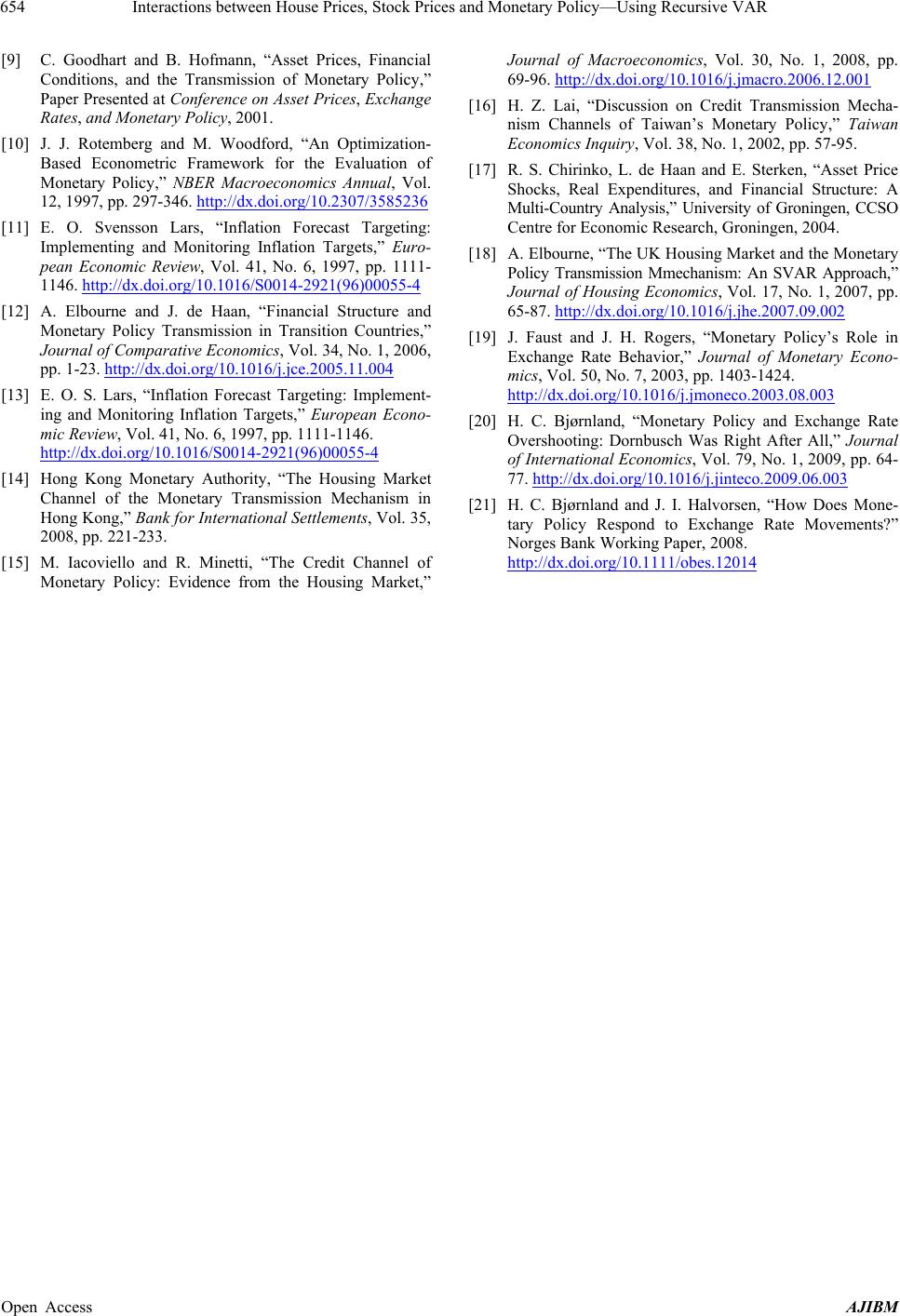 Interactions between House Prices, Stock Prices and Monetary Policy—Using Recursive VAR Open Access AJIBM 654 [9] C. Goodhart and B. Hofmann, “Asset Prices, Financial Conditions, and the Transmission of Monetary Policy,” Paper Presented at Conference on Asset Prices, Exchange Rates, and Monetary Policy, 2001. [10] J. J. Rotemberg and M. Woodford, “An Optimization- Based Econometric Framework for the Evaluation of Monetary Policy,” NBER Macroeconomics Annual, Vol. 12, 1997, pp. 297-346. http://dx.doi.org/10.2307/3585236 [11] E. O. Svensson Lars, “Inflation Forecast Targeting: Implementing and Monitoring Inflation Targets,” Euro- pean Economic Review, Vol. 41, No. 6, 1997, pp. 1111- 1146. http://dx.doi.org/10.1016/S0014-2921(96)00055-4 [12] A. Elbourne and J. de Haan, “Financial Structure and Monetary Policy Transmission in Transition Countries,” Journal of Comparative Economics, Vol. 34, No. 1, 2006, pp. 1-23. http://dx.doi.org/10.1016/j.jce.2005.11.004 [13] E. O. S. Lars, “Inflation Forecast Targeting: Implement- ing and Monitoring Inflation Targets,” European Econo- mic Review, Vol. 41, No. 6, 1997, pp. 1111-1146. http://dx.doi.org/10.1016/S0014-2921(96)00055-4 [14] Hong Kong Monetary Authority, “The Housing Market Channel of the Monetary Transmission Mechanism in Hong Kong,” Bank for International Settlements, Vol. 35, 2008, pp. 221-233. [15] M. Iacoviello and R. Minetti, “The Credit Channel of Monetary Policy: Evidence from the Housing Market,” Journal of Macroeconomics, Vol. 30, No. 1, 2008, pp. 69-96. http://dx.doi.org/10.1016/j.jmacro.2006.12.001 [16] H. Z. Lai, “Discussion on Credit Transmission Mecha- nism Channels of Taiwan’s Monetary Policy,” Taiwan Economics Inquiry, Vol. 38, No. 1, 2002, pp. 57-95. [17] R. S. Chirinko, L. de Haan and E. Sterken, “Asset Price Shocks, Real Expenditures, and Financial Structure: A Multi-Country Analysis,” University of Groningen, CCSO Centre for Economic Research, Groningen, 2004. [18] A. Elbourne, “The UK Housing Market and the Monetary Policy Transmission Mmechanism: An SVAR Approach,” Journal of Housing Economics, Vol. 17, No. 1, 2007, pp. 65-87. http://dx.doi.org/10.1016/j.jhe.2007.09.002 [19] J. Faust and J. H. Rogers, “Monetary Policy’s Role in Exchange Rate Behavior,” Journal of Monetary Econo- mics, Vol. 50, No. 7, 2003, pp. 1403-1424. http://dx.doi.org/10.1016/j.jmoneco.2003.08.003 [20] H. C. Bjørnland, “Monetary Policy and Exchange Rate Overshooting: Dornbusch Was Right After All,” Journal of International Economics, Vol. 79, No. 1, 2009, pp. 64- 77. http://dx.doi.org/10.1016/j.jinteco.2009.06.003 [21] H. C. Bjørnland and J. I. Halvorsen, “How Does Mone- tary Policy Respond to Exchange Rate Movements?” Norges Bank Working Paper, 2008. http://dx.doi.org/10.1111/obes.12014
|