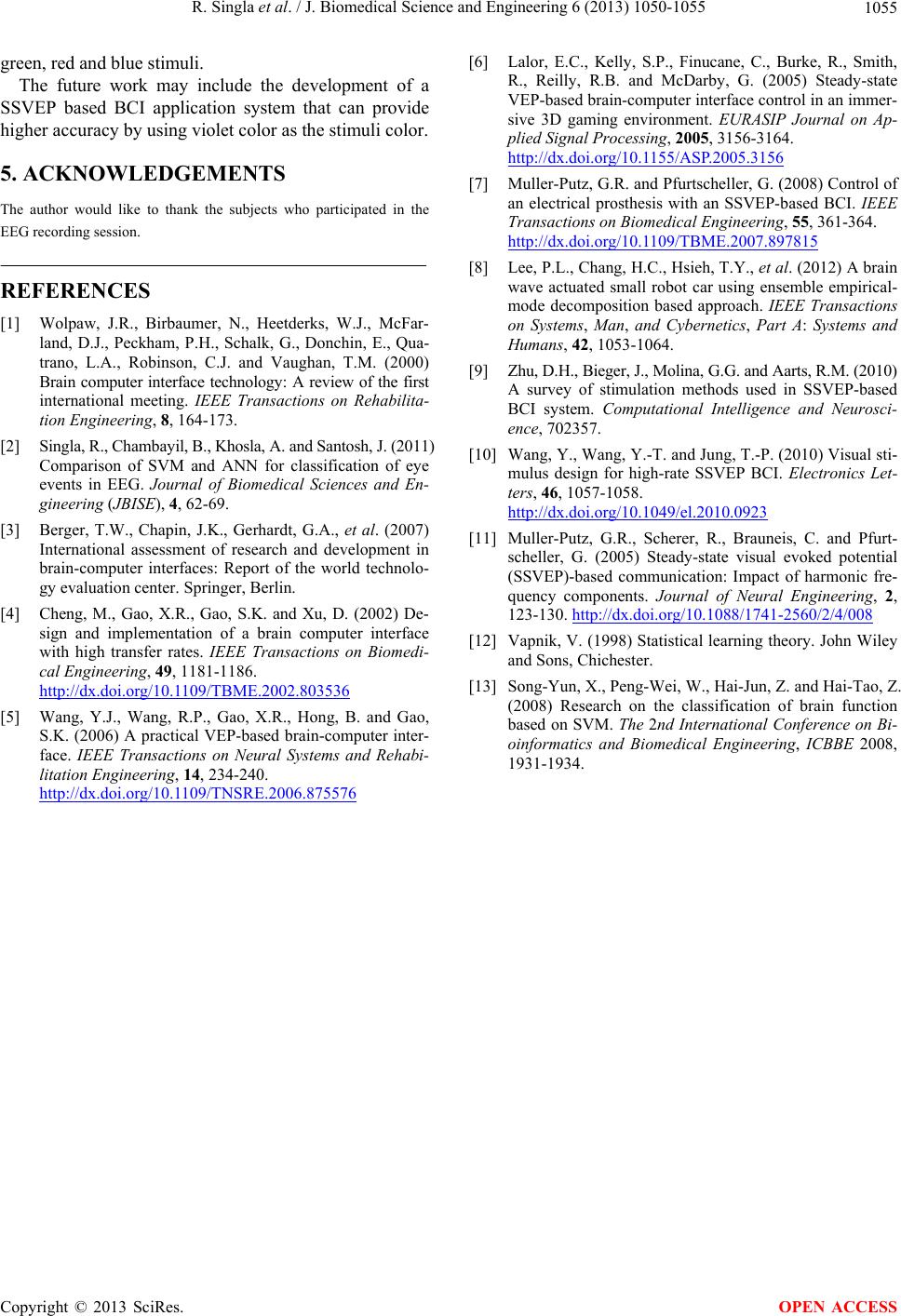
R. Singla et al. / J. Biomedical Science and Engineering 6 (2013) 1050-1055 1055
green, red and blue stimuli.
The future work may include the development of a
SSVEP based BCI application system that can provide
higher accuracy by using violet color as the stimuli color.
5. ACKNOWLEDGEMENTS
The author would like to thank the subjects who participated in the
EEG recording session.
REFERENCES
[1] Wolpaw, J.R., Birbaumer, N., Heetderks, W.J., McFar-
land, D.J., Peckham, P.H., Schalk, G., Donchin, E., Qua-
trano, L.A., Robinson, C.J. and Vaughan, T.M. (2000)
Brain computer interface technology: A review of the first
international meeting. IEEE Transactions on Rehabilita-
tion Engineering, 8, 164-173.
[2] Singla, R., Chambayil, B., Khosla, A. and Santosh, J. (2011)
Comparison of SVM and ANN for classification of eye
events in EEG. Journal of Biomedical Sciences and En-
gineering (JBISE), 4, 62-69.
[3] Berger, T.W., Chapin, J.K., Gerhardt, G.A., et al. (2007)
International assessment of research and development in
brain-computer interfaces: Report of the world technolo-
gy evaluation center. Springer, Berlin.
[4] Cheng, M., Gao, X.R., Gao, S.K. and Xu, D. (2002) De-
sign and implementation of a brain computer interface
with high transfer rates. IEEE Transactions on Biomedi-
cal Engineering, 49, 1181-1186.
http://dx.doi.org/10.1109/TBME.2002.803536
[5] Wang, Y.J., Wang, R.P., Gao, X.R., Hong, B. and Gao,
S.K. (2006) A practical VEP-based brain-computer inter-
face. IEEE Transactions on Neural Systems and Rehabi-
litation Engineering, 14, 234-240.
http://dx.doi.org/10.1109/TNSRE.2006.875576
[6] Lalor, E.C., Kelly, S.P., Finucane, C., Burke, R., Smith,
R., Reilly, R.B. and McDarby, G. (2005) Steady-state
VEP-based brain-computer interface control in an immer-
sive 3D gaming environment. EURASIP Journal on Ap-
plied Signal Processing, 2005, 3156-3164.
http://dx.doi.org/10.1155/ASP.2005.3156
[7] Muller-Putz, G.R. and Pfurtscheller, G. (2008) Control of
an electrical prosthesis with an SSVEP-based BCI. IEEE
Transactions on Biomedical Engineering, 55, 361-364.
http://dx.doi.org/10.1109/TBME.2007.897815
[8] Lee, P.L., Chang, H.C., Hsieh, T.Y., et al. (2012) A brain
wave actuated small robot car using ensemble empirical-
mode decomposition based approach. IEEE Transactions
on Systems, Man, and Cybernetics, Part A: Systems and
Humans, 42, 1053-1064.
[9] Zhu, D.H., Bieger, J., Molina, G.G. and Aarts, R.M. (2010)
A survey of stimulation methods used in SSVEP-based
BCI system. Computational Intelligence and Neurosci-
ence, 702357.
[10] Wang, Y., Wang, Y.-T. and Jung, T.-P. (2010) Visual sti-
mulus design for high-rate SSVEP BCI. Electronics Let-
ters, 46, 1057-1058.
http://dx.doi.org/10.1049/el.2010.0923
[11] Muller-Putz, G.R., Scherer, R., Brauneis, C. and Pfurt-
scheller, G. (2005) Steady-state visual evoked potential
(SSVEP)-based communication: Impact of harmonic fre-
quency components. Journal of Neural Engineering, 2,
123-130. http://dx.doi.org/10.1088/1741-2560/2/4/008
[12] Vapnik, V. (1998) Statistical learning theory. John Wiley
and Sons, Chichester.
[13] Song-Yun, X., Peng-Wei, W., Hai-Jun, Z. and Hai-Tao, Z.
(2008) Research on the classification of brain function
based on SVM. The 2nd International Conference on Bi-
oinformatics and Biomedical Engineering, ICBBE 2008,
1931-1934.
Copyright © 2013 SciRes. OPEN ACCESS