 Vol.3, No.8, 461-469 (2013) Open Journal of Preventiv e Me dic ine http://dx.doi.org/10.4236/ojpm.2013.38062 Healthcare intelligence risk detection systems* Reza Safdari, Jebraeil Farzi#, Marjan Ghazisaeidi, Mahboobeh Mirzaee, Azadeh Goodini Department of Health Information Management, School of Allied Medical Sciences, Tehran University of Medical Sciences, Tehran, Iran; #Corresponding author: j-farzi@razi.tums.ac.ir Received 21 August 2013; revised 28 September 2013; accepted 8 October 2013 Copyright © 2013 Reza Safdari et al. This is an open access article distributed under the Creative Commons Attribution License, which permits unrestricted use, distribution, and reproduction in any medium, provided the original work is properly cited. ABSTRACT Background: Today, in healthcare field that is changing rapidly, decision-makers encounter with ever-increasing inquiries on clinical and ad- ministrative information to realize customers’ le- gal and clinical requirements. Therefore, making decisions on healthcare has changed into a vital, complex and unstructured issue. The present paper mainly focuses on describing decision- making advantages, possible risk to improve ef- ficiency of decision-making on healthcare, and especially medical procedures. Methods: The present research is a review study, which has been carried out by searching through the au- thentic scientific sources, including Pubmed, Google scholar, Iranmedex, and other informa- tion sources. While defining care intelligence, here, we introduce Knowledge Discovery Data- base, the Clinical Support Systems, and Intelli- gence Risk Detection Model and provide the conceptual model. Other issues studied in this paper include the Risk Possibility Assessment T echnique, Risk Possibility Detection using know- ledge management techniques, and expert sys- tems. Result s & Conclusion: Modeling the Intelli- gence Support System is necessary for design- ing Real-Time Risk Detection Information Sys- tems in clinical measures. As taking medical procedures involves complex decision-makings and possibility of high risk, operational applica- tion of the techniques derived from knowledge and data mining models under study will play a crucial role in increasing possibility of success of the measure and pro moti ng safe ty of patients . Keywords: Risk Detect ion Sy stems; Decisi on Support; Healthcare Intelligence 1. INTRODUCTION An effective decision is vital in all healthcare activities. However, decision-making in this field is complex and unstructured; therefore, it is necessary for a decision maker to collect multi-spectral data and information to make an effective decision at the time he/she confronts with multiple options. Unstructured decision-making in complex and dynamic atmospheres is a challenging issue and a decision maker encounters with low-level informa- tion almost in every situation [1]. Needs for the pertinent knowledge, useful data and information are crucial and in fact, knowledge controlling values, tools acceptance, and knowledge management methods, technologies and tactics are essential to guarantee the efficiency and ade- quacy of decision-making process [2]. By recognizing this issue, Wickremesinghe and Schaffer [1] developed Intelligence Continuum. It is a regular method to enable application of principles and tools of KM, which is nec- essary for improving decision-making on healthcare, being confident of the favorability of the results obtained from the decision-making, and maximizing its advantage for patients. Therefore, this idea was developed in the present paper and specially focused on medical proce- dures; this is a field which is not only remarkable, but also requires several risks and vital decision-making processes and in fact it is a suitable atmosphere to de- scribe advantages of this method. This paper focuses on the way the Intelligence Risk Detection Model affects in real-time and appropriately on healthcare support systems. 2. METHODS The present research is a review study, which has been carried out by searching the Health, Intelligence Risk Detection and Systems keywords through the authentic scientific sources, including Pubmed (13 Item without 11 unrelated works), Google scholar (4 Item without 3 un- *Competing interests: The author(s) declare that they have no compet- ing interests. Authors’ contributions: RS, JF, MG, MM and AG contributed to conception and design, analysis and interpretation of data, and drafting and revising the manuscript. JF, MG, MM and AG acquisition of data. All authors read and approved the final manuscript. Copyright © 2013 SciRes. OPEN A CCESS
 R. Safdari et al. / Open Journal of Preventive Medicine 3 (201 3) 461-469 462 related works), Iranmedex (3 Item), and other informa- tion sources. While defining care intelligence, here, we introduce Knowledge Discovery Database, the Clinical Support Systems, and Intelligence Risk Detection Model and provide the conceptual model. Other issues studied in this paper include the Risk Possibility Assessment Technique, Risk Possibility Detection using knowledge management techniques, and expert systems. 3. BACKGROUND AND RELATED WORKS Before the conceptualize the model we must explain the aspects of model and related works as HI, intelli- gence tools, risk assessments and applied systems of model. 3.1. HI The concept of intelligence is very extensive. As this concept entered the business fields, extensive concepts have formed in this concern, including competitive intel- ligence, care intelligence, business intelligence, and mar- keting intelligence. A major part of the sources related to intelligence is concentrated on industrial organizations and they have not taken into consideration sufficiently in the field of health; therefore, to have a better under- standing, we discuss the differences and similarities be- tween it and other fields [2,3] (Table 1). Therefore, healthcare intelligence helps administrative and clinical management to know the capabilities avail- able in health network. It facilitates administrative and clinical decision-making through combining all kinds of measurement principles on different kinds of internal and Table 1. Comparing characteristics of healthcare and other fields. Differences Similarities Management is concentrated in most fields; however, it has various clinical and administrative fields on health department. All departments require improvement in charge, quality and delay through integrated processes. Most departments have a certain group of customers with limited varieties of products. Healthcare requires multiplicity of actors with distinguished needs. Following the success of “Focus on Customer Method” in other fields, the focus in healthcare is mainly on patient; however, the variety of customers of other departments is also mentioned. Most industrial systems have principles of precise measurement. Feelings and choices of individuals are also taken into consideration in healthcare. Like other fields, healthcare takes advantage of system combination. external actors, which is caused by a wide variety of processes [1,3-5] (Figures 1 and 2). 3.2. Intelligence Tools The intelligence tools are very wide and varied. These tools, also called “computational intelligence”, include the following techniques: 1) Expert Systems: “Expert Systems” were formed since the mid-sixties and they have extensive applica- tions in different fields of business today. Expert Sys- tems are made of the following two main elements: a) Theoretical Understanding of Issue Collecting creative terms of problem solving are ob- tained from experience. Deduction ability is the strong point of an expert system and it is exactly the very factor, which make an expert system intelligent. Deduction in- cludes obtaining results of theorems. Therefore, in order to make a deduction, there should be a theorem and a result; the latter was achieved from that theorem [5]. 2) Artificial Neural Networks: Artificial Neural Net- works were introduced since 1940s by Mcculloch and Pitts. As these models were inspired by biology, artificial neurons in the neural networks receive sum of data from the rest of neurons or external stimuli. Here, artificial neurons’ action is similar to the one of brain neurons. Then, they transfer the modified data to the other neu- rons or external outputs; this process is similar to human brain function. Transfer of information from one neuron to another neuron is a method for activation and/or reac- tion to a specific neuronal response based on the data or stimuli it receives [4]. 3) Fuzzy Logic, Fuzzy Sets and Fuzzy Systems: Fuzzy Sets are defined based on a membership function, which is the image of a universal set in the range of [zero and one]. Each element has a grade of membership. A fuzzy set is made by generalization of classical sets the- ory. In classical sets theory the membership of elements in a set is assessed in binary terms. An element either belongs or does not belong to a set. By contrast, fuzzy set theory permits the gradual assessment of the membership of elements in a set [6]. 4) Rough Sets: This concept was introduced by Paw- lak in 1982. Rough sets theory is based on estimation of concepts (sets) using the available knowledge in an in- formation system. Its application on lost values is con- sidered as one of the strong points of this theory. Rough sets theory calculates two sets of upper and lower ap- proximations for processing incomplete data [5]. 5) Evolutionary Algorithms: Evolutionary algorithm is the algorithm, which is a subset of the evolutionary calculation and is placed in the subdivision of artificial intelligence. This algorithm uses different mechanisms, Copyright © 2013 SciRes. OPEN A CCESS
 R. Safdari et al. / Open Journal of Preventive Medicine 3 (201 3) 461-469 Copyright © 2013 SciRes. 463 Figure 1. Processes of healthcare field [3,4]. OPEN A CCESS Figure 2. Framework of technology in healthcare intelligence. including production, leap, composition, and selection. Selected solutions for optimization problems play the role of components and, among these components and people, cost function decides which solutions are re- mained. These algorithms are frequently used in different parts of control systems, design, scheduling, optimization, data restoring, and management [5,7]. a) Combined Techniques: Most of the above tech- niques can be combined with respect to terms of use to achieve a new method; for instance, both fuzzy systems and neural networks can be used simultaneously in health business. b) Data Mining, Web Mining: “Data Mining” is a term, which is used to describe knowledge discovery from databases. Data Mining is a process that uses statistical, mathematical, artificial intelligence and machine learning techniques to extract and identify useful information and knowledge on large databases. According to the new definition, “It is a process to find mathematical patterns among large sets of data”. These patterns may include rules, dependencies, procedures or models of anticipation [7]. Web Mining can also be defined as exploration and analysis of the information of interest and useful infor- mation on a web and about web, which is usually defined by the web-based tools. The term “Web Mining” was first used by Etzioni (1996) [6]. 6) MAS: AOP is an approach in programming, the importance of which is ever increasing and some know it as a more developed form of the Object-Oriented Pro- gramming. The agent is a software entity, which is placed on a dynamic environment and is able to recog- nize characteristics and conditions of the environment and act autonomously (independently) to achieve a goal [5]. 3.3. Applied Systems of Model In the following section, general topics about key scopes of decision support systems, risk detection system, expert system and their importance for designing risk detection design is discussed to improve healthcare deci- sion-making process. 3.3.1. Expert Systems Expert systems (knowledge-based systems) are also
 R. Safdari et al. / Open Journal of Preventive Medicine 3 (201 3) 461-469 464 known as Rule-based Systems. Analyses and programs of these systems are based on If-Then. The algorithm de- signed for expert systems is programmable and/or is de- signed in the form of shells in which the rules and knowledge should be created and executed. Expert sys- tems protect knowledge, increase optimization, improve quality of services and products, do activities in danger- ous working environments, provide high reliability, dis- tribute knowledge through its rapid transfer, and reduce costs in an organization [5]. One of the knowledge-based systems is KDD which is defined as an important recognition process for authentic, new, and probably useful and understandable patterns in data [8,9]. One of the most common applications of KDD in the field of healthcare is IC. Intelligence Con- tinuum includes application of data extraction methods, BI and KM, so that offering favorable healthcare would be easier in IC model [1]. 3.3.2. DSS Decision Support Systems is a subdivision of Opera- tion Research, which were created due to the quantifica- tion techniques of complex issues of management. DSS includes OR application in supporting management deci- sion-making processes [5]. Although some studies have been carried out about DSS in the field of healthcare, DSS applications in clini- cal and diagnosis activities became 10 times bigger dur- ing recent decades [10]. Principally, the present research encompasses the clinical and medical aspects and fo- cuses on how to apply IT and improve decision-making efficiency for physicians. Specifically, the computer- based decision support systems merely focus on the software designed for this purpose to help clinical deci- sion-making, and features of patients are in accordance with computer knowledge basis to carry out patient’s specific assessment and/or recommendation to physi- cians [11]. In addition, computer registration of patient’s information, internet, common decision-making proc- esses, and new rules facilitate medical decision-making supporting systems [12]. Consequently, to conduct our present research, sources containing CDSS and MDSS are equally related and computer systems are used for supporting decision-making of healthcare specialists. Decision making about medical procedures for pa- tients is considered as a complex and multilateral meas- ure in most cases. Patients may have various symptoms; however, this measure is mainly operational. Here, com- plete anatomic restoration of an organ can be considered as an example. If it is decided to carry out restoration later, the risks and advantages of medical procedures in proportion to the possible risks of taking no measure should be weighed up. In addition, making a decision on an alternative treatment using drugs or taking a measure or a combination of both depend on several factors [1,2]. Decision-making process on medical procedure can be divided into 3 steps. In the first step or the step prior to taking measure, a physician acquires sufficient informa- tion about a patient and his/her medical conditions and decides whether such a measure is the best option as far as his profession is concerned or not. When the decision is made and prior to taking a measure, the patient should decide on the acceptance and rejection of the physician’s decision with respect to the anticipated results. When the patients and physicians are in accord, in step two, the decision-makings on the unique situations are considered during a measure. Finally, in the step after medical measure or step 3, decision are mainly made at two lev- els: the first level, the strategies for guaranteeing suc- cessful result for a patient during post-care period and step b, the lessons that should be applied for future cases. In order to overcome this complexity, we define two steps of decision-making within three different and key processes of decision-making on performing the clinical measures. The first type of decision-making is called medical decision-making, which is mainly related to physician. The second type of decision-making is called personal decision-making, which is mainly related to patients because some of the results of a medical proce- dure are related to the quality of life of patients in which the patient’s decision-making is vital. Although, DSS is usually discussed well in healthcare field, unfortunately, acceptance of these solutions is at a low level due to unwillingness of physicians to use computer systems for these purposes [13]. Special atten- tion should be paid to the guarantee of clinical applica- tion of the pertinent approach. Real-time risk detection system is probably useful and suitable and, in turn, its application and acceptance are reinforced. 3.3.3. Intelligence Risk Detection Framework in Healthcare A physician’s performance is usually assessed indi- rectly, using care results in a hospital, relevant tools, and probability of risk. Although determination of risk prob- ability is important for assessing performance and com- paring results for people or organizations, today, the present methods pay attention to more extended field of care. This seems to be rather misleading and physician’s performance is assessed after a procedure and usually 30 days after the procedure. The weak results may be due to technical errors, nurses’ errors, drug’s errors and/or care and the supervision lower than the standard level [14]. With respect to the study of resources, it can be real- ized that observing these criteria using risk probability indicators has been under development since 1980 and several conditions and health plans have been applied according to the plans for selecting type of medical Copyright © 2013 SciRes. OPEN A CCESS
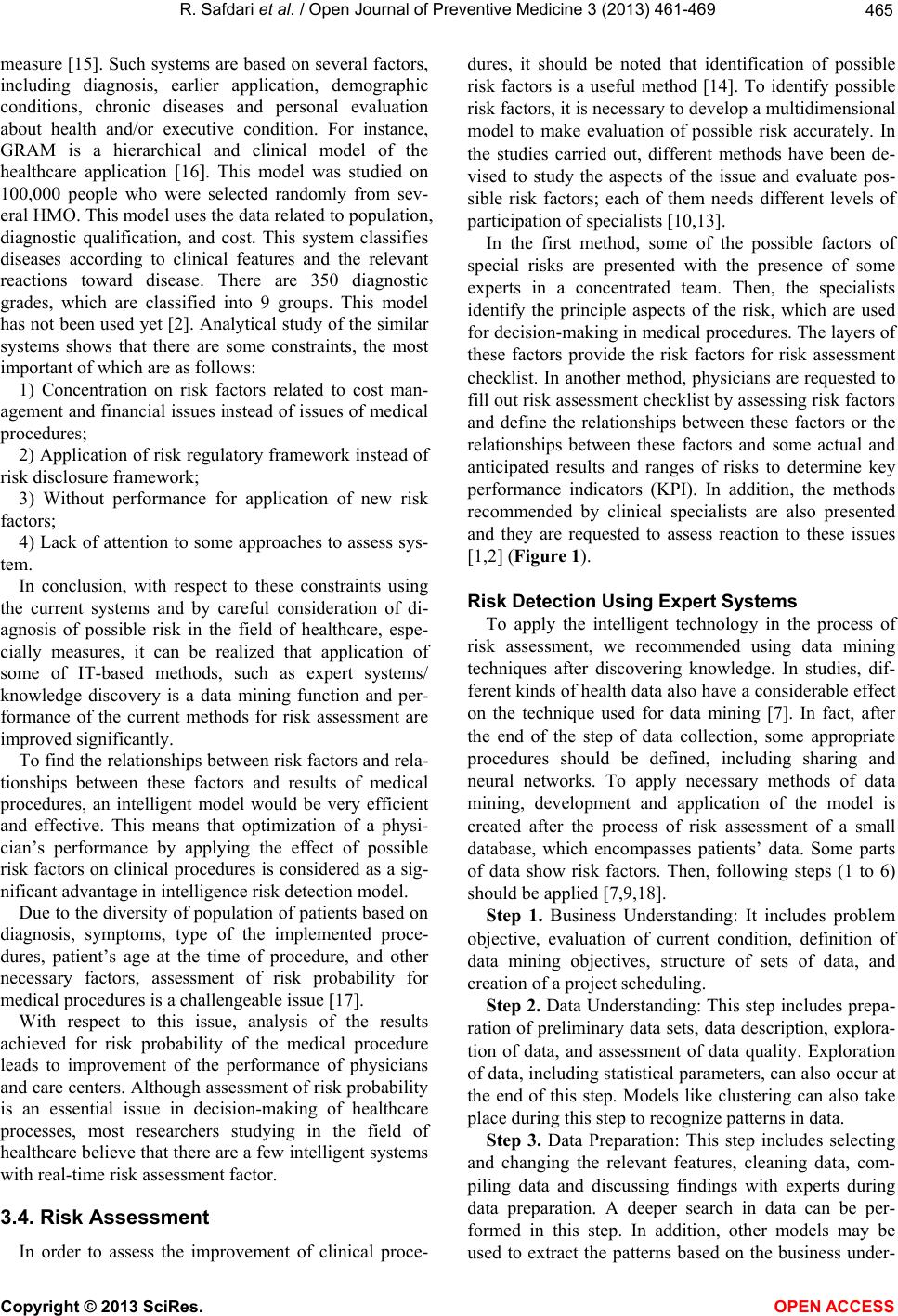 R. Safdari et al. / Open Journal of Preventive Medicine 3 (201 3) 461-469 465 measure [15]. Such systems are based on several factors, including diagnosis, earlier application, demographic conditions, chronic diseases and personal evaluation about health and/or executive condition. For instance, GRAM is a hierarchical and clinical model of the healthcare application [16]. This model was studied on 100,000 people who were selected randomly from sev- eral HMO. This model uses the data related to population, diagnostic qualification, and cost. This system classifies diseases according to clinical features and the relevant reactions toward disease. There are 350 diagnostic grades, which are classified into 9 groups. This model has not been used yet [2]. Analytical study of the similar systems shows that there are some constraints, the most important of which are as follows: 1) Concentration on risk factors related to cost man- agement and financial issues instead of issues of medical procedures; 2) Application of risk regulatory framework instead of risk disclosure framework; 3) Without performance for application of new risk factors; 4) Lack of attention to some approaches to assess sys- tem. In conclusion, with respect to these constraints using the current systems and by careful consideration of di- agnosis of possible risk in the field of healthcare, espe- cially measures, it can be realized that application of some of IT-based methods, such as expert systems/ knowledge discovery is a data mining function and per- formance of the current methods for risk assessment are improved significantly. To find the relationships between risk factors and rela- tionships between these factors and results of medical procedures, an intelligent model would be very efficient and effective. This means that optimization of a physi- cian’s performance by applying the effect of possible risk factors on clinical procedures is considered as a sig- nificant advantage in intelligence risk detection model. Due to the diversity of population of patients based on diagnosis, symptoms, type of the implemented proce- dures, patient’s age at the time of procedure, and other necessary factors, assessment of risk probability for medical procedures is a challengeable issue [17]. With respect to this issue, analysis of the results achieved for risk probability of the medical procedure leads to improvement of the performance of physicians and care centers. Although assessment of risk probability is an essential issue in decision-making of healthcare processes, most researchers studying in the field of healthcare believe that there are a few intelligent systems with real-time risk assessment factor. 3.4. Risk Assessment In order to assess the improvement of clinical proce- dures, it should be noted that identification of possible risk factors is a useful method [14]. To identify possible risk factors, it is necessary to develop a multidimensional model to make evaluation of possible risk accurately. In the studies carried out, different methods have been de- vised to study the aspects of the issue and evaluate pos- sible risk factors; each of them needs different levels of participation of specialists [10,13]. In the first method, some of the possible factors of special risks are presented with the presence of some experts in a concentrated team. Then, the specialists identify the principle aspects of the risk, which are used for decision-making in medical procedures. The layers of these factors provide the risk factors for risk assessment checklist. In another method, physicians are requested to fill out risk assessment checklist by assessing risk factors and define the relationships between these factors or the relationships between these factors and some actual and anticipated results and ranges of risks to determine key performance indicators (KPI). In addition, the methods recommended by clinical specialists are also presented and they are requested to assess reaction to these issues [1,2] (Figure 1). Risk Detection Using Expert Systems To apply the intelligent technology in the process of risk assessment, we recommended using data mining techniques after discovering knowledge. In studies, dif- ferent kinds of health data also have a considerable effect on the technique used for data mining [7]. In fact, after the end of the step of data collection, some appropriate procedures should be defined, including sharing and neural networks. To apply necessary methods of data mining, development and application of the model is created after the process of risk assessment of a small database, which encompasses patients’ data. Some parts of data show risk factors. Then, following steps (1 to 6) should be applied [7,9,18]. Step 1. Business Understanding: It includes problem objective, evaluation of current condition, definition of data mining objectives, structure of sets of data, and creation of a project scheduling. Step 2. Data Understanding: This step includes prepa- ration of preliminary data sets, data description, explora- tion of data, and assessment of data quality. Exploration of data, including statistical parameters, can also occur at the end of this step. Models like clustering can also take place during this step to recognize patterns in data. Step 3. Data Preparation: This step includes selecting and changing the relevant features, cleaning data, com- piling data and discussing findings with experts during data preparation. A deeper search in data can be per- formed in this step. In addition, other models may be used to extract the patterns based on the business under- Copyright © 2013 SciRes. OPEN A CCESS
 R. Safdari et al. / Open Journal of Preventive Medicine 3 (201 3) 461-469 Copyright © 2013 SciRes. 466 antee continuous improvement of the anticipated capa- bilities [1,2,13]. standing. Step 4. Modeling: Web mining software tools like (Visualization) and (Clustering Analysis) are useful for preliminary analysis. Tools like recognizing public rules are able to extract preliminary correlation rules. When a more understanding of the data is achieved by recogniz- ing the pattern, which is obtained with respect to the outputs of the preliminary models, more specialized models can be used in terms of the type of data. As risk factors are effective in reducing people’s qual- ity of life, the importance of a correct design of deci- sion-making processes in medical procedure has become significantly important. The way to follow procedures always involves innovation in medical technologies; therefore, by indentifying these risk factors, healthcare axes can be classified into four key components [1,2,4, 13]: Step 5. Evaluation: The results obtained from the models, which were used at the earlier steps are evalu- ated at the early stages using the performance evaluation set and within the defined issue and the defined objec- tives. This will lead to identifying the following needs. 1) Physiological issues with respect to the importance of quality of life; 2) Technological issues based on new technologies and their performance; Step 6. Deployment: Deployment includes arranging a discussion with experts about data extraction to study the accuracy of the discussed theorems and to discover the possible or knowledge patterns from the results of data extraction. Therefore, if new risk factors or patterns are discovered, they will discuss the findings with deci- sion-makers to determine appropriate procedures. 3) Biomechanical issues with respect to health condi- tion of patients to perform remedial action; 4) Financial issues on costs of these technologies with quality and type of remedial action; Consequently, we discuss IRD Model to support deci- sion-making for a better treatment for this population of patients during medical procedures and after it. This can provide better results for the patients and their families. Of course, a different attitude was adopted toward the models proposed in literatures [1,2,13] in terms of gen- erality of issue and scope of discussion, which needs a detailed account [4]. 4. IRD PROPOSED MODEL Figure 3 shows the steps of risk assessment. The out- put of risk assessment process helps to determine the important risk factors of healthcare and to anticipated results based on the relevant factors. The anticipated re- sults enable physicians to decide on the care/treatment/ medical plan. To make the final decision, measures are taken to provide patients with necessary information on the care plan. Any conflict in making decision on proce- dure and patients indicates a high level of risk and is considered as a negative consequence for the procedure. Such a conflict is used to evaluate possible risk in the future for the same patient or the other patients in the system. After medical procedures, the actual results are also compared with the anticipated results. This com- parison is an evaluation approach for the model to guar- To develop such a model in terms of its theatrical and operational aspects, it is necessary to combine three key scopes of expert system, risk detection system and deci- sion support system (Figure 4). According to the decision-making process framework, two types of decision-makings (personal decision-mak- ing and decision-making based on medical procedures) are defined. This model (Figure 3) leads to detecting real-time possible risk factors and anticipation of results of medical procedures. The results in personal and medical decision-makings are of paramount importance [19]. Figure 3. Conceptual model for risk assessment [2]. OPEN A CCESS
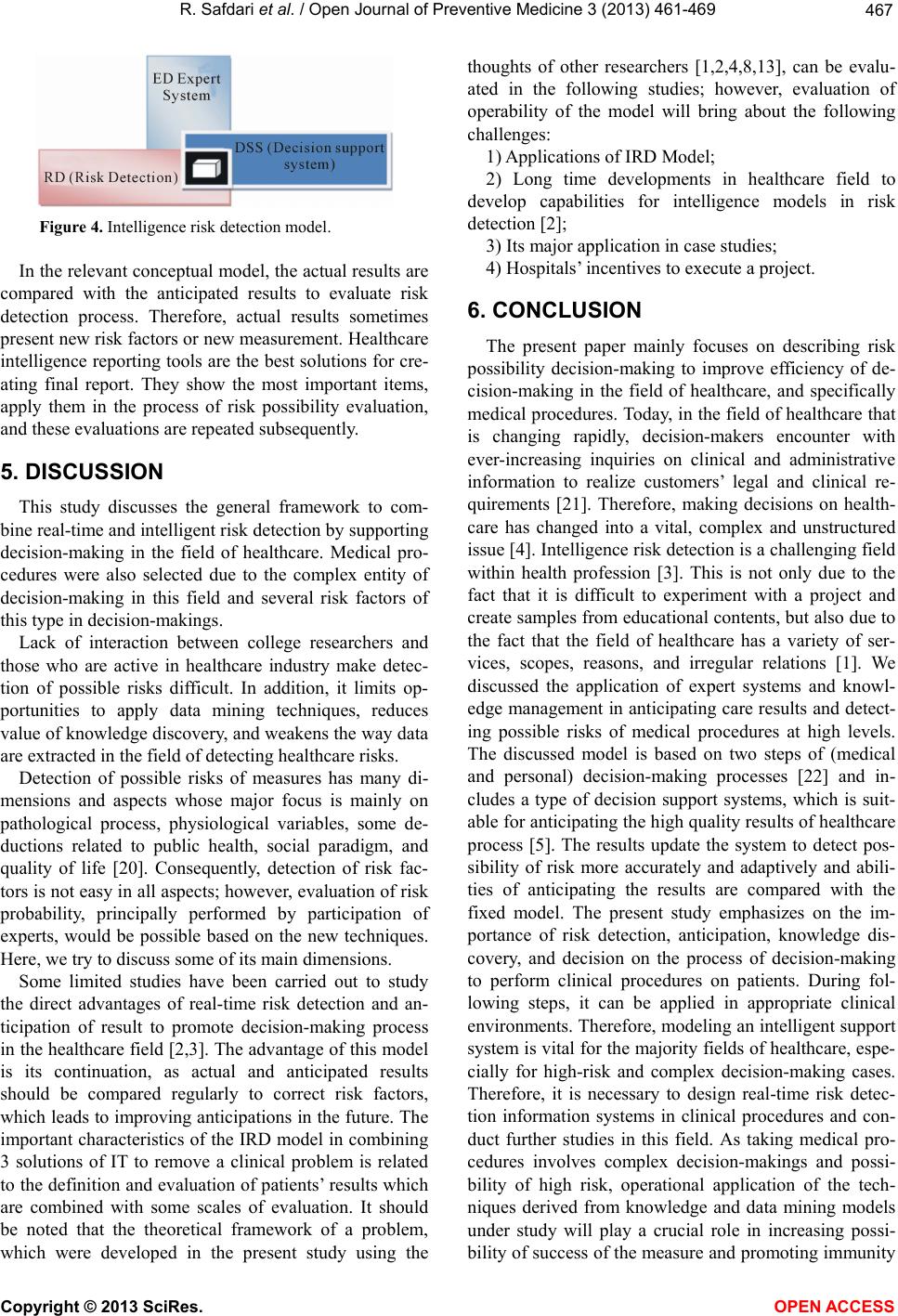 R. Safdari et al. / Open Journal of Preventive Medicine 3 (201 3) 461-469 467 Figure 4. Intelligence risk detection model. In the relevant conceptual model, the actual results are compared with the anticipated results to evaluate risk detection process. Therefore, actual results sometimes present new risk factors or new measurement. Healthcare intelligence reporting tools are the best solutions for cre- ating final report. They show the most important items, apply them in the process of risk possibility evaluation, and these evaluations are repeated subsequently. 5. DISCUSSION This study discusses the general framework to com- bine real-time and intelligent risk detection by supporting decision-making in the field of healthcare. Medical pro- cedures were also selected due to the complex entity of decision-making in this field and several risk factors of this type in decision-makings. Lack of interaction between college researchers and those who are active in healthcare industry make detec- tion of possible risks difficult. In addition, it limits op- portunities to apply data mining techniques, reduces value of knowledge discovery, and weakens the way data are extracted in the field of detecting healthcare risks. Detection of possible risks of measures has many di- mensions and aspects whose major focus is mainly on pathological process, physiological variables, some de- ductions related to public health, social paradigm, and quality of life [20]. Consequently, detection of risk fac- tors is not easy in all aspects; however, evaluation of risk probability, principally performed by participation of experts, would be possible based on the new techniques. Here, we try to discuss some of its main dimensions. Some limited studies have been carried out to study the direct advantages of real-time risk detection and an- ticipation of result to promote decision-making process in the healthcare field [2,3]. The advantage of this model is its continuation, as actual and anticipated results should be compared regularly to correct risk factors, which leads to improving anticipations in the future. The important characteristics of the IRD model in combining 3 solutions of IT to remove a clinical problem is related to the definition and evaluation of patients’ results which are combined with some scales of evaluation. It should be noted that the theoretical framework of a problem, which were developed in the present study using the thoughts of other researchers [1,2,4,8,13], can be evalu- ated in the following studies; however, evaluation of operability of the model will bring about the following challenges: 1) Applications of IRD Model; 2) Long time developments in healthcare field to develop capabilities for intelligence models in risk detection [2]; 3) Its major application in case studies; 4) Hospitals’ incentives to execute a project. 6. CONCLUSION The present paper mainly focuses on describing risk possibility decision-making to improve efficiency of de- cision-making in the field of healthcare, and specifically medical procedures. Today, in the field of healthcare that is changing rapidly, decision-makers encounter with ever-increasing inquiries on clinical and administrative information to realize customers’ legal and clinical re- quirements [21]. Therefore, making decisions on health- care has changed into a vital, complex and unstructured issue [4]. Intelligence risk detection is a challenging field within health profession [3]. This is not only due to the fact that it is difficult to experiment with a project and create samples from educational contents, but also due to the fact that the field of healthcare has a variety of ser- vices, scopes, reasons, and irregular relations [1]. We discussed the application of expert systems and knowl- edge management in anticipating care results and detect- ing possible risks of medical procedures at high levels. The discussed model is based on two steps of (medical and personal) decision-making processes [22] and in- cludes a type of decision support systems, which is suit- able for anticipating the high quality results of healthcare process [5]. The results update the system to detect pos- sibility of risk more accurately and adaptively and abili- ties of anticipating the results are compared with the fixed model. The present study emphasizes on the im- portance of risk detection, anticipation, knowledge dis- covery, and decision on the process of decision-making to perform clinical procedures on patients. During fol- lowing steps, it can be applied in appropriate clinical environments. Therefore, modeling an intelligent support system is vital for the majority fields of healthcare, espe- cially for high-risk and complex decision-making cases. Therefore, it is necessary to design real-time risk detec- tion information systems in clinical procedures and con- duct further studies in this field. As taking medical pro- cedures involves complex decision-makings and possi- bility of high risk, operational application of the tech- niques derived from knowledge and data mining models under study will play a crucial role in increasing possi- bility of success of the measure and promoting immunity Copyright © 2013 SciRes. OPEN A CCESS
 R. Safdari et al. / Open Journal of Preventive Medicine 3 (201 3) 461-469 468 of patients. 7. ACKNOWLEDGEMENTS We would like to thank Ahmad Soltani, expert of Health Information Management at research center for Health Information Management, for his contributions to the research presented here. We would also like to thank the reviewers for their insightful comments. REFERENCES [1] Wickramasinghe, N. and Schaffer, J. (2006) Creating knowledge-driven healthcare process with the intelligen- ce continuum. International Journal of Electronic Health- care, 2, 164-174. [2] Moghimi, H., Zadeh, H., Schaffer, J. and Wickrama- singhe, N. (2012) Incorporating intelligent risk detection to enable superior decision support: The example of or- thopaedic surgeries. Health and Technology, 2, 33-41. http://dx.doi.org/10.1007/s12553-011-0014-z [3] Mettler, T. and Vimarlund, V. (2008) Understanding busi- ness intelligence in the context of health care. Proceed- ings of the 13th International Symposium for Health In- formation Management Research (ISHIMR), Massey Uni- versity, 61-69. [4] Safdari, R., Torabi, M., Farzi, J., Mirzaee, M. and Goo- dini, A. (2012) Intelligence risk detection models: Tools to promote patients safety level. Proceedings of the 3th Symposium for E-hospital & Telemedicine, Tehran Uni- versity of Medical Sciences, Tehran, 56. [5] Mahler, E.M., Schmidt, R.M. and Kvitash, V.I. (1993) An artificial intelligence system to predict progression of immune dysfunction in healthy older patients. Journal of Medical Systems, 17, 173-181. http://dx.doi.org/10.1007/BF00996942 [6] Turban, E., Mclean, E. and Wetherbe. J. (2002) Informa- tion technology for management. 3rd Edition, John Wiely & Sons INS., Hoboken, 520-545. [7] Wu, J., Roy, J. and Stewart, W.F. (2010) Prediction mod- eling using EHR data: Challenges, strategies, and a com- parison of machine learning approaches. Medical Care, 48, S106-S113. http://dx.doi.org/10.1097/MLR.0b013e3181de9e17 [8] Wickramasinghe, N., Bali, R., Gibbons, C., Choi, C. and Schaffer, J. (2009) Optimization of health care operations with knowledge management. JHIMS, 3, 44-50. [9] Aral, K.D., Güvenir, H.A., Sabuncuoğlu, I. and Akar, A.R. (2012) A prescription fraud detection model. Computer Methods and Programs in Biomedicine, 106, 37-46. http://dx.doi.org/10.1016/j.cmpb.2011.09.003 [10] Miller, R.A. (1994) Medical diagnostic decision support systems—Past, present, and future: A threaded bibliogra- phy and brief commentary. Journal of the American Medical Informatics Association, 1, 8-27. http://dx.doi.org/10.1136/jamia.1994.95236141 [11] Hunt, D.L., Haynes, R.B., Hanna, S.E. and Smith, K. (1998) Effects of computer-based clinical decision sup- port outcomes: A systematic review systems on physician performance and patient. JAMA, 280, 1339-1346. http://dx.doi.org/10.1001/jama.280.15.1339 [12] Fieschi, M., Dufour, J.C., Staccini, P., Gouvernet, J. and Bouhaddou, O. (2003) Medical decision support systems: Old dilemmas and new paradigms? Tracks for successful integration and adoption. Methods of Information in Medicine, 42, 191-198. [13] Moghimi, F., Seif Zadeh, H., Cheung M. and Wickrama- singhe, N. (2011) An intelligent risk detection framework using business intelligence tools to improve decision effi- ciency in healthcare contexts. Proceedings of the 17th Americas Conference on Information Systems (AMCIS), United States, 4-7 August 2011, 1-8. http://aisel.aisnet.org/amcis2011_submissions/173/ [14] Larrazabal, L.A., Del Nido, P.J., Jenkins, K.J. and Gau- vreau, K. (2007) Measurement of technical performance in congenital heart surgery: A pilot study. The Annals of Thoracic Surgery, 83, 179-184. http://dx.doi.org/10.1016/j.athoracsur.2006.07.031 [15] Keenan, P., Buntin Beeuwkes, M., McGuire, T. and New- house, J.P. (2001) The prevalence of formal risk adjust- ment in health plan purchasing. Inquiry, 38, 245-259. http://dx.doi.org/10.5034/inquiryjrnl_38.3.245 [16] Hornbrook, M.C. and Goodman, M.J. (1996) Chronic disease, functional health status, and demographics: A multi-dimensional approach to risk adjustment. Health Services Research, 31, 283-307. [17] Kang, N., Cole, T., Tsang, V., Elliott, M. and de Leval, M. (2004) Risk stratification in paediatric open-heart surgery. European Journal Cardio-Thoracic Surgery, 26, 3-11. http://dx.doi.org/10.1016/j.ejcts.2004.03.038 [18] Kumar, A. and Gosain, A. (2009) Analysis of health care data using different data mining techniques. Interna- tional Conference on Intelligent Agent & Multi-Agent Systems (IAMA), Chennai. [19] Safdari, R., Farzi, J., Mirzaee, M. and Goodini, A. (2013) Intelligence Risk Detection systems for medical proce- dures. Proceedings of the 1st conference for Telemedicine, Amirkabir University, Tehran, 46. [20] Wilson, I.B. and Cleary, P.D. (1995) Linking clinical variables with health-related quality of life: A conceptual model of patient outcomes. JAMA, 273, 59-65. http://dx.doi.org/10.1001/jama.1995.03520250075037 [21] Farzi, J., Salem Safi, P., Zohoor, A.R. and Ebadi Fardazar, F. (2008) The study of National Diabetes Registry System: Model suggestion for Iran. Journal of Ardabil University of Medical Sciences & Health Services, 3, 288-293. [22] Garg, A.X., Adhikari, N.K., McDonald, H., Rosas-Are- llano, M.P., Devereaux, P.J., Beyene, J., Sam, J. and Hay- nes, R.B. (2005) Effects of computerized clinical decision support systems on practitioner performance and patient outcomes: A systematic review. JAMA, 293, 1223-1238. Copyright © 2013 SciRes. OPEN A CCESS
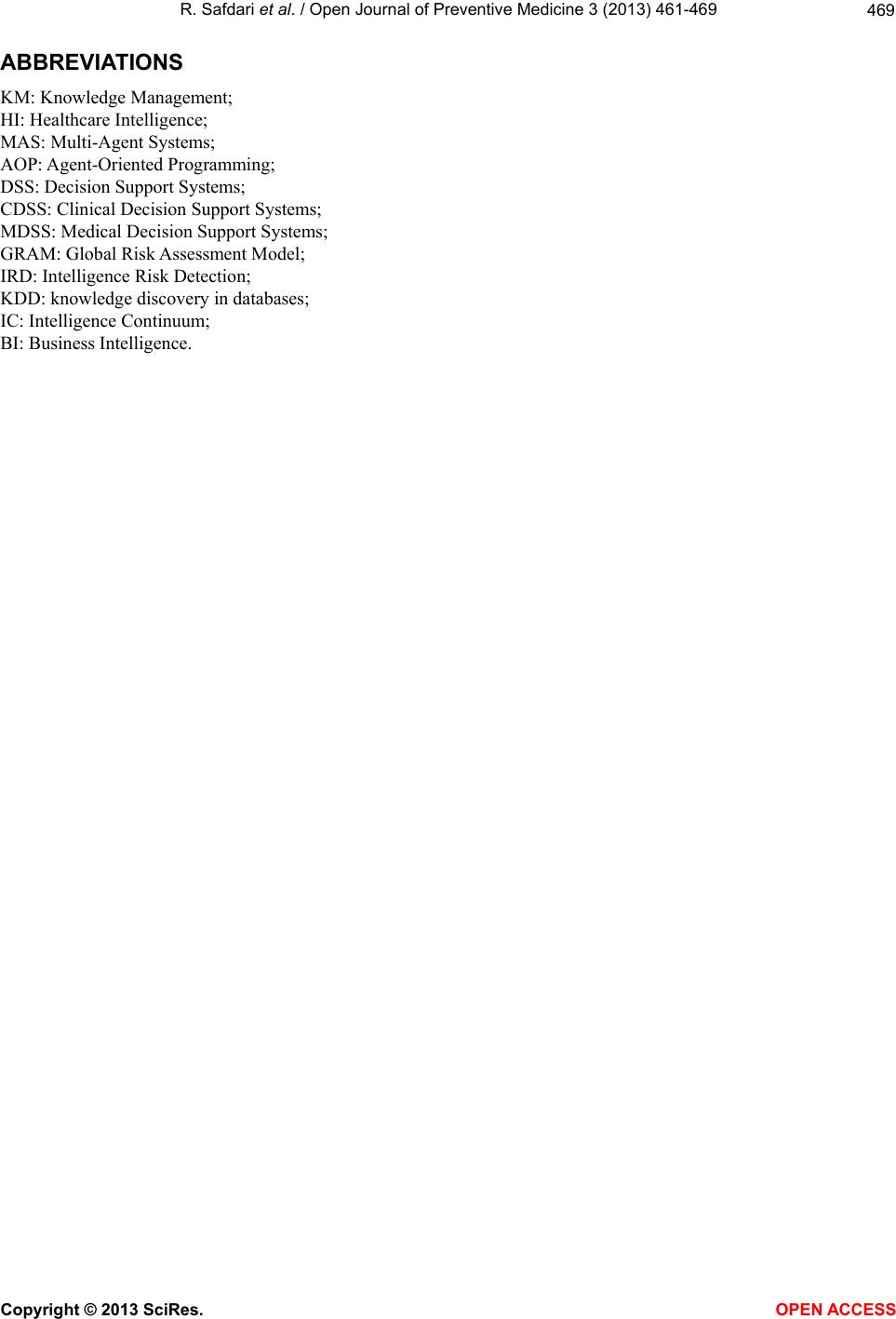 R. Safdari et al. / Open Journal of Preventive Medicine 3 (201 3) 461-469 469 ABBREVIATIONS KM: Knowledge Management; HI: Healthcare Intelligence; MAS: Multi-Agent Systems; AOP: Agent-Oriented Programming; DSS: Decision Support Systems; CDSS: Clinical Decision Support Systems; MDSS: Medical Decision Support Systems; GRAM: Global Risk Assessment Model; IRD: Intelligence Risk Detection; KDD: knowledge discovery in databases; IC: Intelligence Continuum; BI: Business Intelligence. Copyright © 2013 SciRes. OPEN A CCESS
|