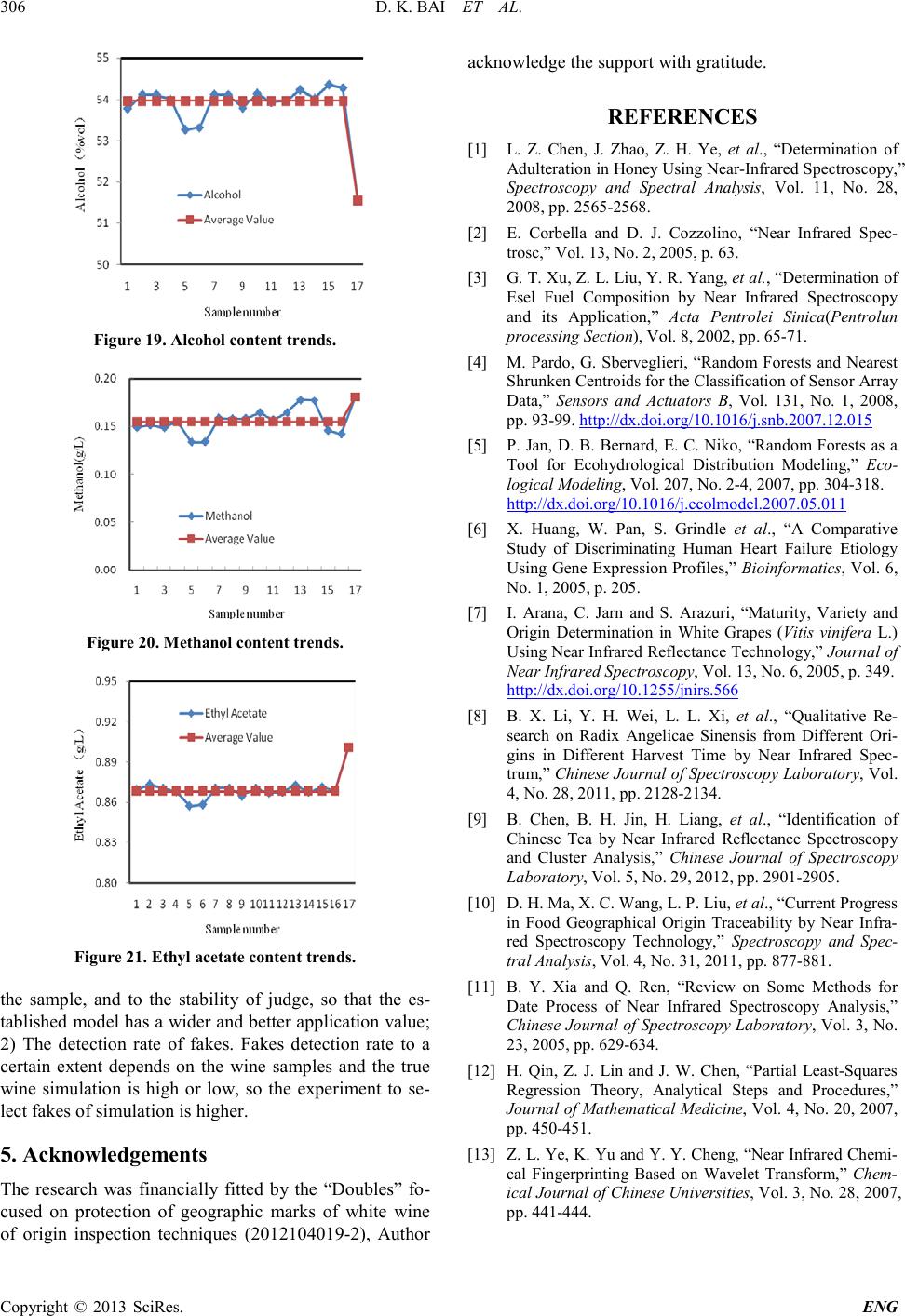
D. K. BAI ET AL.
Copyright © 2013 SciRes. ENG
Figure 19. Alcohol co ntent trends.
Figure 20. Me thanol c ontent tre nds.
Figure 21. Ethyl acetate c onte nt tre nds.
the sample, and to the stability of judge, so that the es-
tablished mod el has a wider a nd better ap plication value ;
2) The detection rate of fakes. Fakes detection rate to a
certain extent depends on the wine samples and the true
wine simulation is high or low, so the experiment to se-
lect fakes of simul ation is higher .
5. Acknowledgements
The research was financially fitted by the “Doubles” fo-
cused on protection of geographic marks of white wine
of origin inspection techniques (2012104019-2), Author
acknowledge the suppo rt with gratitude .
REFERENCES
[1] L. Z. Chen, J. Zhao, Z. H. Ye, et al., “Determination of
Adulteration in Honey Using Near-Infrared Spectroscopy,”
Spectroscopy and Spectral Analysis, Vol. 11, No. 28,
2008, pp. 2565-2568.
[2] E. Corbella and D. J. Cozzo lino, “Near Infrared Spec-
trosc,” Vol. 13, No. 2, 2005, p. 63.
[3] G. T. Xu, Z. L. Liu, Y. R. Yang, et al., “Determination of
Esel Fuel Composition by Near Infrared Spectroscopy
and its Application,” Acta Pentrolei Sinica(Pentrolun
proce s s ing Se c t ion), Vol. 8, 2002, pp. 65-71.
[4] M. Pa rdo , G. Sberveglieri , “Random Forests and Nearest
Shrunken Centroids for the Classification of Sensor Array
Data,” Sensors and Actuators B, Vol. 131, No. 1, 2008,
pp. 93-99. http://dx.doi.org/10.1016/j.snb.2007.12.015
[5] P. Jan, D. B. Bernard, E. C. Niko, “Random Forests as a
Tool for Ecohydrological Distribution Modeling,” Eco-
logical Modeling, Vol. 207, No. 2-4, 2007, pp. 304-318.
http://dx.doi.org/10.1016/j.ecolmodel.2007.05.011
[6] X. Huang, W. Pan, S. Grindle et al., “A Comparative
Study of Discriminating Human Heart Failure Etiology
Using Gene Expression Profiles,” Bioinformatics, Vol. 6,
No. 1, 2005, p. 205.
[7] I. Arana, C. Jarn and S. Arazuri, “Maturity, Variety and
Origin Determination in White Grapes (Vitis vinifera L.)
Using Near I nfrared Refl ectance Technol ogy,” Journal of
Near Infr ar e d Spe c t r os c o py, Vol. 13, No. 6, 2005, p. 349.
http://dx.doi.org/10.1255/jnirs.566
[8] B. X. Li, Y. H. Wei, L. L. Xi, et al., “Qualitative Re-
search on Radix Angelicae Sinensis from Different Ori-
gins in Different Harvest Time by Near Infrared Spec-
trum,” Chinese Journal of Spectroscopy Laboratory, Vol.
4, No. 28, 2011, pp. 2128-2134.
[9] B. Chen, B. H. Jin, H. Li ang, et al., “Identification of
Chinese Tea by Near Infrared Reflectance Spectroscopy
and Cluster Analysis,” Chinese Journal of Spectroscopy
Laboratory, Vol. 5, No. 29, 2012, pp. 2901-2905.
[10] D. H. Ma, X. C. Wang , L. P. Liu, et al., “Current Progress
in Food Geographical Origin Traceability by Near Infra-
red Spectroscopy Technology,” Spectroscopy and Spec-
tral Analysis, Vol. 4, No. 31, 2011, pp. 877-881.
[11] B. Y. Xia and Q. Ren, “Review on Some Methods for
Date Process of Near Infrared Spectroscopy Analysis,”
Chinese Journal of Spectroscopy Laboratory, Vol. 3, No .
23, 2005, pp. 629-634.
[12] H. Qin, Z. J. Lin and J. W. Chen, “Partial Least-Squares
Regression Theory, Analytical Steps and Procedures,”
Journal of Mathematical Medicine, Vol. 4, No. 20, 2007,
pp. 450-451.
[13] Z. L. Ye, K. Yu and Y. Y. Cheng, “Near Infrared Chemi-
cal Fingerprinting Based on Wavelet Transform,” Chem-
ical Journal of Chinese Un iversi ties, Vol. 3, No. 28, 2007,
pp. 441-444.