 Vol.3, No.1, 75-84 (2011) Natural Science http://dx.doi.org/10.4236/ns.2011.31011 Copyright © 2011 SciRes. OPEN ACCESS A numerical study of coupled maps representing energy exchange processes between two environmental interfaces regarded as biophysical complex systems Dragutin Mihailović1, Mirko Budinčević2, Darko Kapor3, Igor Balaž1, Dušanka Perišić2 1Faculty of Agriculture, University of Novi Sad, Novi Sad, Serbia; guto@polj.uns.ac.rs 2Department for Mathematics and Informatics, Faculty of Sciences, University of Novi Sad, Novi Sad, Serbia 3Department of Physics, Faculty of Sciences, University of Novi Sad, Novi Sad, Serbia Received 23 September 2010; revised 25 October 2010; accepted 29 October 2010. ABSTRACT The field of environmental sciences is abundant with various interfaces and is the right place for the application of new fundamental approaches leading towards a better understanding of en- vironmental phenomena. Following the defini- tion of environmental interface by Mihailovic and Balaž [1], such interface can be, for exam- ple, placed between: human or animal bodies and surrounding air, aquatic species and water and air around them, and natural or artificially built surfaces (vegetation, ice, snow, barren soil, water, urban communities) and the atmosphere, cells and surrounding environment, etc. Complex environmental interface systems are (i) open and hierarchically organised (ii) interactions between their constituent parts are nonlinear, and (iii) their interaction with the surrounding environ- ment is noisy. These systems are therefore very sensitive to initial conditions, deterministic ex- ternal perturbations and random fluctuations always present in nature. The study of noisy non-equilibrium processes is fundamental for modelling the dynamics of environmental inter- face regarded as biophysical complex system and for understanding the mechanisms of spa- tio-temporal pattern formation in contemporary environmental sciences. In this paper we will investigate an aspect of dynamics of energy flow based on the energy balance equation. The en- ergy exchange between interacting environmen- tal interfaces regarded as biophysical complex systems can be represented by coupled maps. Therefore, we will numerically investigate cou- pled maps representing that exchange. In ana- lysis of behaviour of these maps we applied Lyapunov exponent and cross sample entropy. Keywords: Environmental Interface; Nonlinearity; Chaos; Logistic Equation; Energy Balance Equation; Coupled Maps, Hierarchy, Biophysical Complex Systems 1. INTRODUCTION The field of environmental sciences is abundant with various interfaces and is the right place for the applica- tion of new fundamental approaches leading towards a better understanding of environmental phenomena. We defined the environmental interface as an interface be- tween two either abiotic or biotic environments which are in relative motion exchanging energy through bio- physical and chemical processes and fluctuating tempo- rally and spatially regardless of its space and time scale [1]. This definition broadly covers the unavoidable mul- tidisciplinary approach in environmental sciences and also includes the traditional approaches in sciences that deal with environmental space. The environmental in- terface as a complex system is a suitable area for the occurrence of irregularities in temporal variations of some physical, chemical or biological quantities de- scribing their interactions [2-4]. For example, such in- terface can be placed between: human or animal bodies and surrounding air, aquatic species and water and air around them, and natural or artificially built surfaces (vegetation, ice, snow, barren soil, water, urban commu- nities) and the atmosphere, cells and surrounding envi- ronment, etc. The environmental interface of different media was considered in different contexts [5-8]. Com- plex environmental interface systems are open and hier- archically organised and interactions between their parts
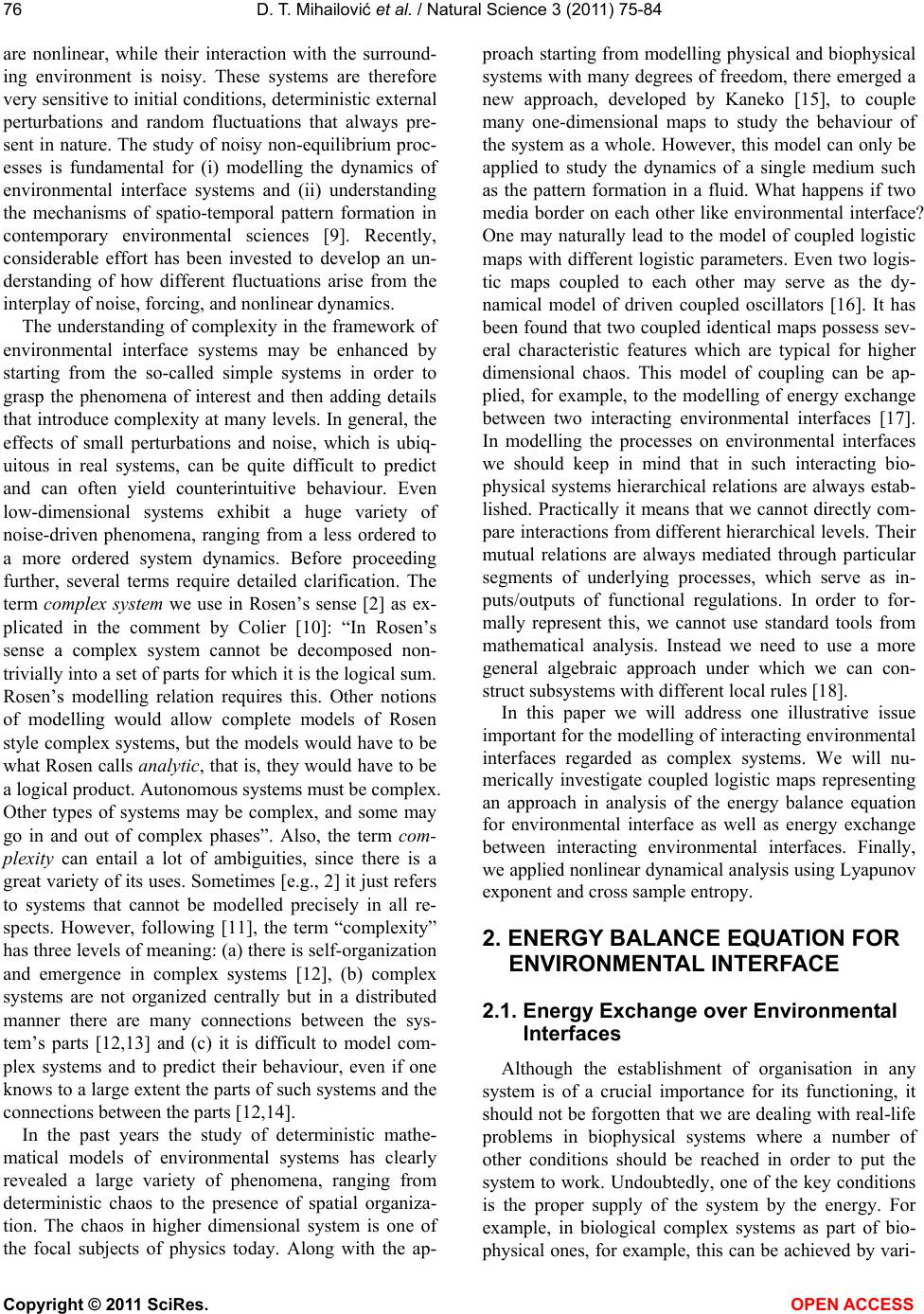 D. T. Mihailović et al. / Natural Science 3 (2011) 75-84 Copyright © 2011 SciRes. OPEN ACCESS 76 are nonlinear, while their interaction with the surround- ing environment is noisy. These systems are therefore very sensitive to initial conditions, deterministic external perturbations and random fluctuations that always pre- sent in nature. The study of noisy non-equilibrium proc- esses is fundamental for (i) modelling the dynamics of environmental interface systems and (ii) understanding the mechanisms of spatio-temporal pattern formation in contemporary environmental sciences [9]. Recently, considerable effort has been invested to develop an un- derstanding of how different fluctuations arise from the interplay of noise, forcing, and nonlinear dynamics. The understanding of complexity in the framework of environmental interface systems may be enhanced by starting from the so-called simple systems in order to grasp the phenomena of interest and then adding details that introduce complexity at many levels. In general, the effects of small perturbations and noise, which is ubiq- uitous in real systems, can be quite difficult to predict and can often yield counterintuitive behaviour. Even low-dimensional systems exhibit a huge variety of noise-driven phenomena, ranging from a less ordered to a more ordered system dynamics. Before proceeding further, several terms require detailed clarification. The term complex system we use in Rosen’s sense [2] as ex- plicated in the comment by Colier [10]: “In Rosen’s sense a complex system cannot be decomposed non- trivially into a set of parts for which it is the logical sum. Rosen’s modelling relation requires this. Other notions of modelling would allow complete models of Rosen style complex systems, but the models would have to be what Rosen calls analytic, that is, they would have to be a logical product. Autonomous systems must be complex. Other types of systems may be complex, and some may go in and out of complex phases”. Also, the term com- plexity can entail a lot of ambiguities, since there is a great variety of its uses. Sometimes [e.g., 2] it just refers to systems that cannot be modelled precisely in all re- spects. However, following [11], the term “complexity” has three levels of meaning: (a) there is self-organization and emergence in complex systems [12], (b) complex systems are not organized centrally but in a distributed manner there are many connections between the sys- tem’s parts [12,13] and (c) it is difficult to model com- plex systems and to predict their behaviour, even if one knows to a large extent the parts of such systems and the connections between the parts [12,14]. In the past years the study of deterministic mathe- matical models of environmental systems has clearly revealed a large variety of phenomena, ranging from deterministic chaos to the presence of spatial organiza- tion. The chaos in higher dimensional system is one of the focal subjects of physics today. Along with the ap- proach starting from modelling physical and biophysical systems with many degrees of freedom, there emerged a new approach, developed by Kaneko [15], to couple many one-dimensional maps to study the behaviour of the system as a whole. However, this model can only be applied to study the dynamics of a single medium such as the pattern formation in a fluid. What happens if two media border on each other like environmental interface? One may naturally lead to the model of coupled logistic maps with different logistic parameters. Even two logis- tic maps coupled to each other may serve as the dy- namical model of driven coupled oscillators [16]. It has been found that two coupled identical maps possess sev- eral characteristic features which are typical for higher dimensional chaos. This model of coupling can be ap- plied, for example, to the modelling of energy exchange between two interacting environmental interfaces [17]. In modelling the processes on environmental interfaces we should keep in mind that in such interacting bio- physical systems hierarchical relations are always estab- lished. Practically it means that we cannot directly com- pare interactions from different hierarchical levels. Their mutual relations are always mediated through particular segments of underlying processes, which serve as in- puts/outputs of functional regulations. In order to for- mally represent this, we cannot use standard tools from mathematical analysis. Instead we need to use a more general algebraic approach under which we can con- struct subsystems with different local rules [18]. In this paper we will address one illustrative issue important for the modelling of interacting environmental interfaces regarded as complex systems. We will nu- merically investigate coupled logistic maps representing an approach in analysis of the energy balance equation for environmental interface as well as energy exchange between interacting environmental interfaces. Finally, we applied nonlinear dynamical analysis using Lyapunov exponent and cross sample entropy. 2. ENERGY BALANCE EQUATION FOR ENVIRONMENTAL INTERFACE 2.1. Energy Exchange over Environmental Interfaces Although the establishment of organisation in any system is of a crucial importance for its functioning, it should not be forgotten that we are dealing with real-life problems in biophysical systems where a number of other conditions should be reached in order to put the system to work. Undoubtedly, one of the key conditions is the proper supply of the system by the energy. For example, in biological complex systems as part of bio- physical ones, for example, this can be achieved by vari-
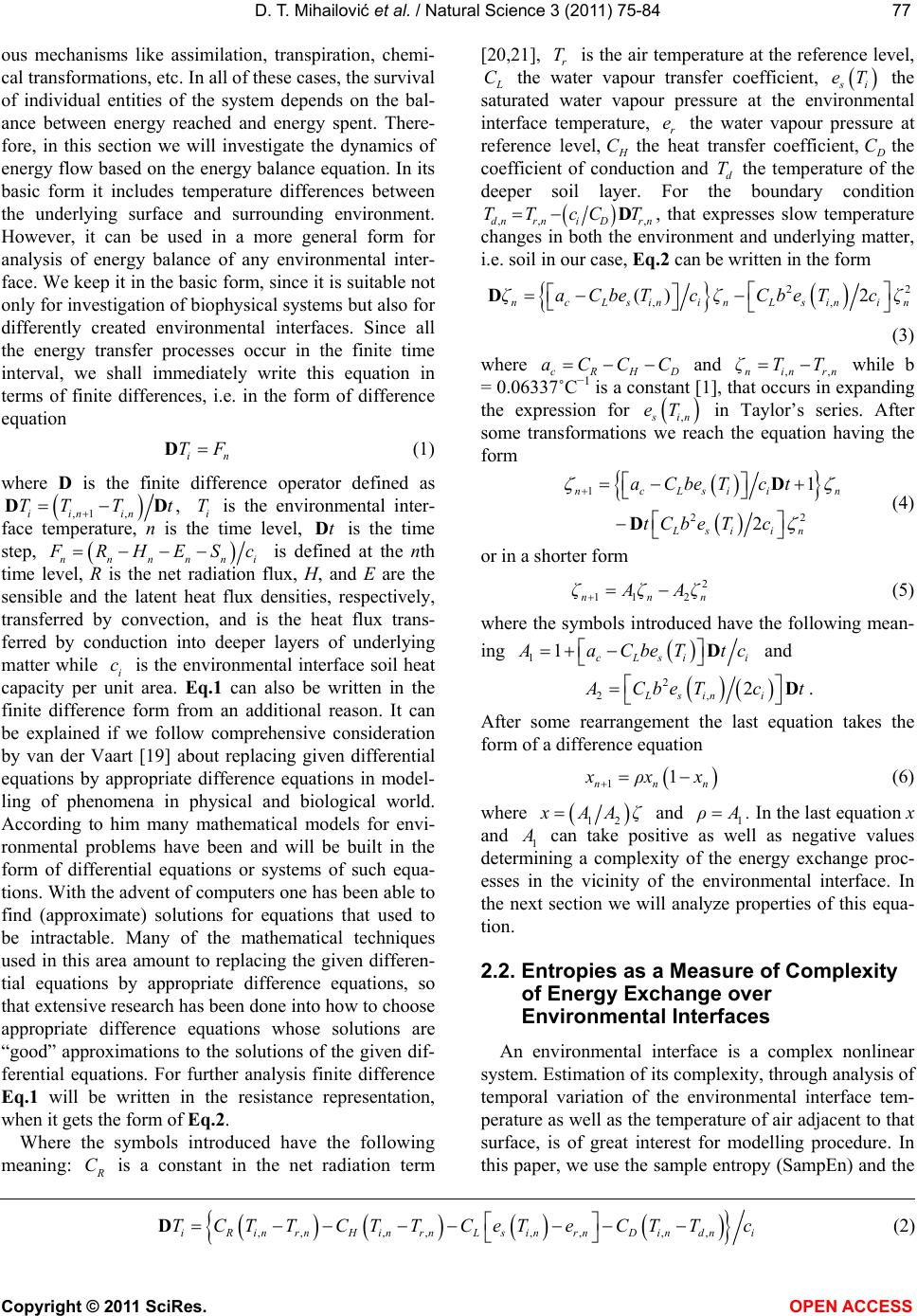 D. T. Mihailović et al. / Natural Science 3 (2011) 75-84 Copyright © 2011 SciRes. OPEN ACCESS 7777 ous mechanisms like assimilation, transpiration, chemi- cal transformations, etc. In all of these cases, the survival of individual entities of the system depends on the bal- ance between energy reached and energy spent. There- fore, in this section we will investigate the dynamics of energy flow based on the energy balance equation. In its basic form it includes temperature differences between the underlying surface and surrounding environment. However, it can be used in a more general form for analysis of energy balance of any environmental inter- face. We keep it in the basic form, since it is suitable not only for investigation of biophysical systems but also for differently created environmental interfaces. Since all the energy transfer processes occur in the finite time interval, we shall immediately write this equation in terms of finite differences, i.e. in the form of difference equation in TFD (1) where D is the finite difference operator defined as ,1 ,, iin in TT Tt DD i T is the environmental inter- face temperature, n is the time level, tD is the time step, nnnnni RHES c is defined at the nth time level, R is the net radiation flux, H, and E are the sensible and the latent heat flux densities, respectively, transferred by convection, and is the heat flux trans- ferred by conduction into deeper layers of underlying matter while i c is the environmental interface soil heat capacity per unit area. Eq.1 can also be written in the finite difference form from an additional reason. It can be explained if we follow comprehensive consideration by van der Vaart [19] about replacing given differential equations by appropriate difference equations in model- ling of phenomena in physical and biological world. According to him many mathematical models for envi- ronmental problems have been and will be built in the form of differential equations or systems of such equa- tions. With the advent of computers one has been able to find (approximate) solutions for equations that used to be intractable. Many of the mathematical techniques used in this area amount to replacing the given differen- tial equations by appropriate difference equations, so that extensive research has been done into how to choose appropriate difference equations whose solutions are “good” approximations to the solutions of the given dif- ferential equations. For further analysis finite difference Eq.1 will be written in the resistance representation, when it gets the form of Eq.2. Where the symbols introduced have the following meaning: C is a constant in the net radiation term [20,21], r T is the air temperature at the reference level, C the water vapour transfer coefficient, i eT the saturated water vapour pressure at the environmental interface temperature, r e the water vapour pressure at reference level, C the heat transfer coefficient, C the coefficient of conduction and d T the temperature of the deeper soil layer. For the boundary condition ,, ,dnrni Drn TT cCT D, that expresses slow temperature changes in both the environment and underlying matter, i.e. soil in our case, Eq.2 can be written in the form 22 ,, () 2 ncLs ininLsinin ζaCbeTcζCbeTcζ D (3) where cRHD aCC C and ,,ninrn ζTT while b = 0.06337˚C–1 is a constant [1], that occurs in expanding the expression for , in eT in Taylor’s series. After some transformations we reach the equation having the form 1 22 1 2 ncLsii n Lsiin aCbeT ct tCbe Tc D D (4) or in a shorter form 2 11 2nnn ζAζAζ (5) where the symbols introduced have the following mean- ing 11cLsi i aCbeT tc D and 2 2, 2 Lsin i CbeTct D. After some rearrangement the last equation takes the form of a difference equation 11 nnn xρ x (6) where 12 xAAζ and 1 ρ . In the last equation x and 1 can take positive as well as negative values determining a complexity of the energy exchange proc- esses in the vicinity of the environmental interface. In the next section we will analyze properties of this equa- tion. 2.2. Entropies as a Measure of Complexity of Energy Exchange over Environmental Interfaces An environmental interface is a complex nonlinear system. Estimation of its complexity, through analysis of temporal variation of the environmental interface tem- perature as well as the temperature of air adjacent to that surface, is of great interest for modelling procedure. In this paper, we use the sample entropy (SampEn) and the ,,,,,,, ,iRin rnHin rnLsinrnDin dni TCTTCTTCeT eCTTc D (2)
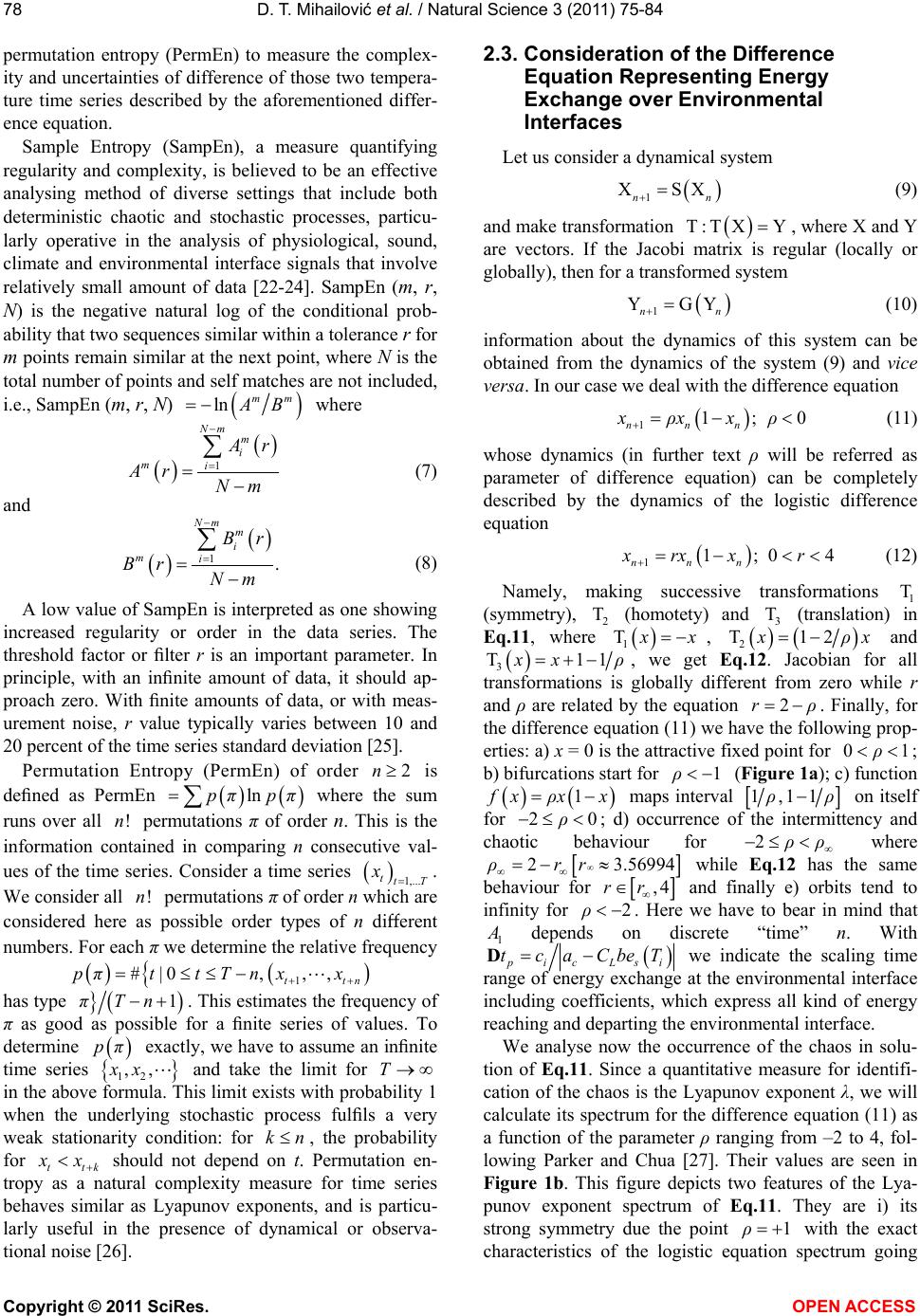 D. T. Mihailović et al. / Natural Science 3 (2011) 75-84 Copyright © 2011 SciRes. OPEN ACCESS 78 permutation entropy (PermEn) to measure the complex- ity and uncertainties of difference of those two tempera- ture time series described by the aforementioned differ- ence equation. Sample Entropy (SampEn), a measure quantifying regularity and complexity, is believed to be an effective analysing method of diverse settings that include both deterministic chaotic and stochastic processes, particu- larly operative in the analysis of physiological, sound, climate and environmental interface signals that involve relatively small amount of data [22-24]. SampEn (m, r, N) is the negative natural log of the conditional prob- ability that two sequences similar within a tolerance r for m points remain similar at the next point, where N is the total number of points and self matches are not included, i.e., SampEn (m, r, N) ln mm B where 1 Nm m i mi r Ar Nm (7) and 1. Nm m i mi Br Br Nm (8) A low value of SampEn is interpreted as one showing increased regularity or order in the data series. The threshold factor or filter r is an important parameter. In principle, with an infinite amount of data, it should ap- proach zero. With finite amounts of data, or with meas- urement noise, r value typically varies between 10 and 20 percent of the time series standard deviation [25]. Permutation Entropy (PermEn) of order 2n is defined as PermEn lnpπpπ where the sum runs over all !n permutations π of order n. This is the information contained in comparing n consecutive val- ues of the time series. Consider a time series 1,... ttT x. We consider all !n permutations π of order n which are considered here as possible order types of n different numbers. For each π we determine the relative frequency 1 #|0, ,, ttn pπttTnxx has type 1πTn . This estimates the frequency of π as good as possible for a finite series of values. To determine pπ exactly, we have to assume an infinite time series 12 ,,xx and take the limit for T in the above formula. This limit exists with probability 1 when the underlying stochastic process fulfils a very weak stationarity condition: for kn, the probability for ttk x should not depend on t. Permutation en- tropy as a natural complexity measure for time series behaves similar as Lyapunov exponents, and is particu- larly useful in the presence of dynamical or observa- tional noise [26]. 2.3. Consideration of the Difference Equation Representing Energy Exchange over Environmental Interfaces Let us consider a dynamical system 1 XSX nn (9) and make transformation T:T XY, where X and Y are vectors. If the Jacobi matrix is regular (locally or globally), then for a transformed system 1 YGY nn (10) information about the dynamics of this system can be obtained from the dynamics of the system (9) and vice versa. In our case we deal with the difference equation 11;0 nnn xρxxρ (11) whose dynamics (in further text ρ will be referred as parameter of difference equation) can be completely described by the dynamics of the logistic difference equation 11;0 4 nnn xrxx r (12) Namely, making successive transformations 1 T (symmetry), 2 T (homotety) and 3 T (translation) in Eq.11, where 1 T x , 2 T12xρ and 3 T11xx , we get Eq.12. Jacobian for all transformations is globally different from zero while r and ρ are related by the equation 2r . Finally, for the difference equation (11) we have the following prop- erties: a) x = 0 is the attractive fixed point for 01ρ ; b) bifurcations start for 1ρ (Figure 1a); c) function 1fx ρ x maps interval 1,11ρ on itself for 20ρ ; d) occurrence of the intermittency and chaotic behaviour for 2ρ where 2 3.56994ρrr while Eq.12 has the same behaviour for ,4rr and finally e) orbits tend to infinity for 2ρ . Here we have to bear in mind that 1 depends on discrete “time” n. With ic Lsi tcaCbeTD we indicate the scaling time range of energy exchange at the environmental interface including coefficients, which express all kind of energy reaching and departing the environmental interface. We analyse now the occurrence of the chaos in solu- tion of Eq.11. Since a quantitative measure for identifi- cation of the chaos is the Lyapunov exponent λ, we will calculate its spectrum for the difference equation (11) as a function of the parameter ρ ranging from –2 to 4, fol- lowing Parker and Chua [27]. Their values are seen in Figure 1b. This figure depicts two features of the Lya- punov exponent spectrum of Eq.11 . They are i) its strong symmetry due the point 1ρ with the exact characteristics of the logistic equation spectrum going
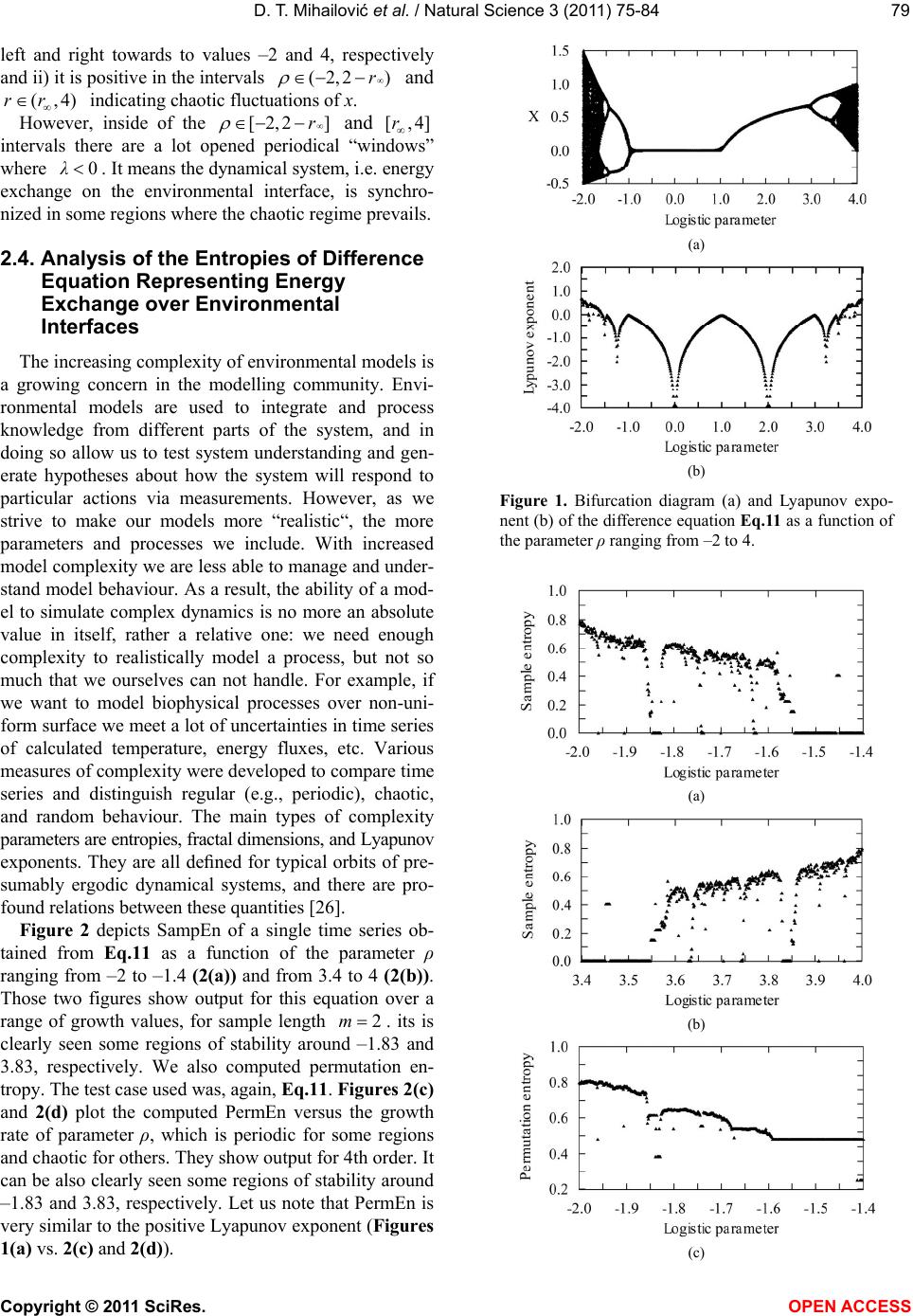 D. T. Mihailović et al. / Natural Science 3 (2011) 75-84 Copyright © 2011 SciRes. OPEN ACCESS 7979 left and right towards to values –2 and 4, respectively and ii) it is positive in the intervals (2,2)r and (,4)rr indicating chaotic fluctuations of x. However, inside of the [2,2 ]r and [,4]r intervals there are a lot opened periodical “windows” where 0λ. It means the dynamical system, i.e. energy exchange on the environmental interface, is synchro- nized in some regions where the chaotic regime prevails. 2.4. Analysis of the Entropies of Difference Equation Representing Energy Exchange over Environmental Interfaces The increasing complexity of environmental models is a growing concern in the modelling community. Envi- ronmental models are used to integrate and process knowledge from different parts of the system, and in doing so allow us to test system understanding and gen- erate hypotheses about how the system will respond to particular actions via measurements. However, as we strive to make our models more “realistic“, the more parameters and processes we include. With increased model complexity we are less able to manage and under- stand model behaviour. As a result, the ability of a mod- el to simulate complex dynamics is no more an absolute value in itself, rather a relative one: we need enough complexity to realistically model a process, but not so much that we ourselves can not handle. For example, if we want to model biophysical processes over non-uni- form surface we meet a lot of uncertainties in time series of calculated temperature, energy fluxes, etc. Various measures of complexity were developed to compare time series and distinguish regular (e.g., periodic), chaotic, and random behaviour. The main types of complexity parameters are entropies, fractal dimensions, and Lyapunov exponents. They are all defined for typical orbits of pre- sumably ergodic dynamical systems, and there are pro- found relations between these quantities [26]. Figure 2 depicts SampEn of a single time series ob- tained from Eq.11 as a function of the parameter ρ ranging from –2 to –1.4 (2(a )) and from 3.4 to 4 (2(b)). Those two figures show output for this equation over a range of growth values, for sample length 2m. its is clearly seen some regions of stability around –1.83 and 3.83, respectively. We also computed permutation en- tropy. The test case used was, again, Eq.11. Figures 2(c) and 2(d) plot the computed PermEn versus the growth rate of parameter ρ, which is periodic for some regions and chaotic for others. They show output for 4th order. It can be also clearly seen some regions of stability around –1.83 and 3.83, respectively. Let us note that PermEn is very similar to the positive Lyapunov exponent (Figures 1(a) vs. 2(c) and 2(d)). (a) (b) Figure 1. Bifurcation diagram (a) and Lyapunov expo- nent (b) of the difference equation Eq.11 as a function of the parameter ρ ranging from –2 to 4. (a) (b) (c)
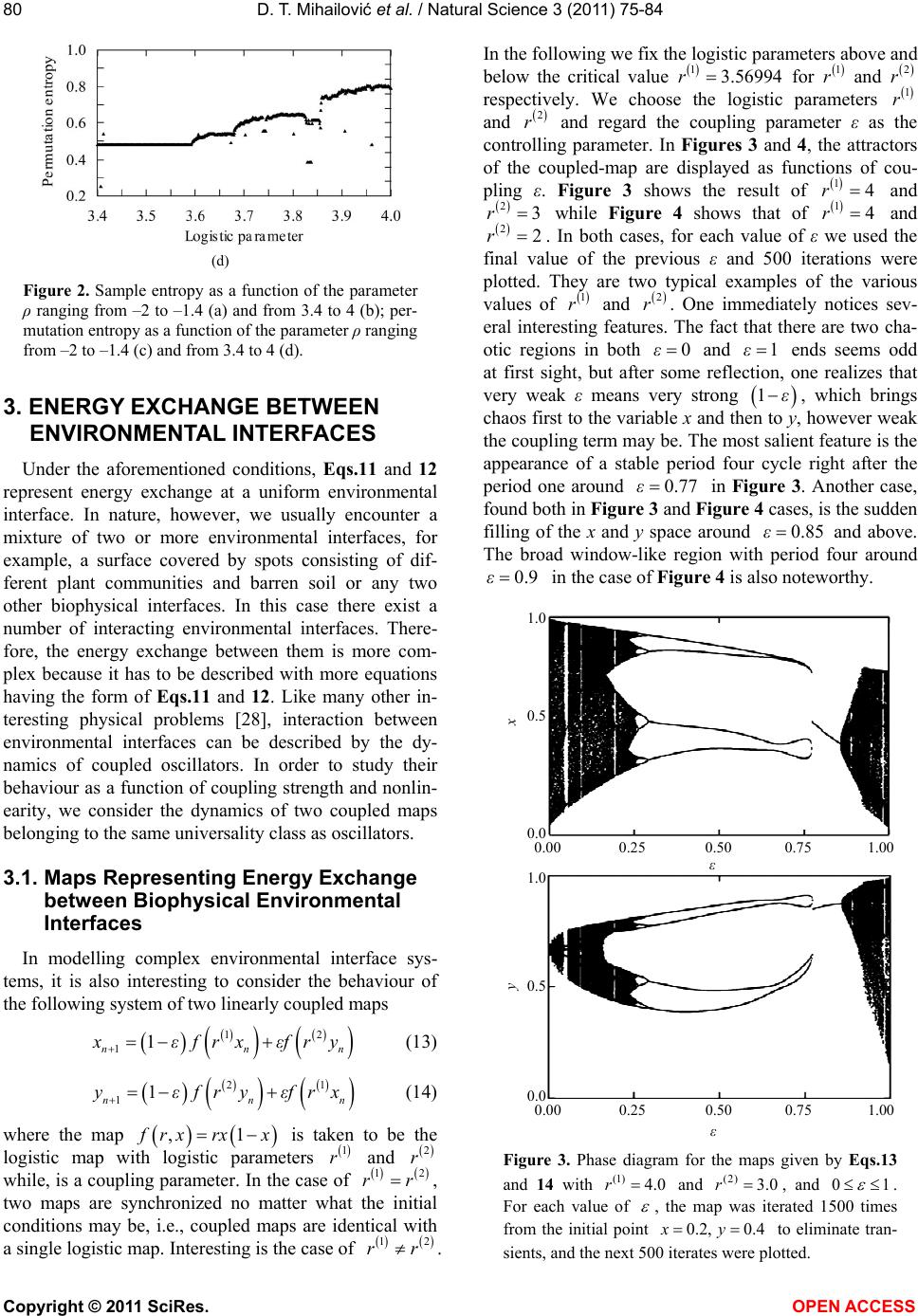 D. T. Mihailović et al. / Natural Science 3 (2011) 75-84 Copyright © 2011 SciRes. OPEN ACCESS 80 (d) Figure 2. Sample entropy as a function of the parameter ρ ranging from –2 to –1.4 (a) and from 3.4 to 4 (b); per- mutation entropy as a function of the parameter ρ ranging from –2 to –1.4 (c) and from 3.4 to 4 (d). 3. ENERGY EXCHANGE BETWEEN ENVIRONMENTAL INTERFACES Under the aforementioned conditions, Eqs.11 and 12 represent energy exchange at a uniform environmental interface. In nature, however, we usually encounter a mixture of two or more environmental interfaces, for example, a surface covered by spots consisting of dif- ferent plant communities and barren soil or any two other biophysical interfaces. In this case there exist a number of interacting environmental interfaces. There- fore, the energy exchange between them is more com- plex because it has to be described with more equations having the form of Eqs.11 and 12. Like many other in- teresting physical problems [28], interaction between environmental interfaces can be described by the dy- namics of coupled oscillators. In order to study their behaviour as a function of coupling strength and nonlin- earity, we consider the dynamics of two coupled maps belonging to the same universality class as oscillators. 3.1. Maps Representing Energy Exchange between Biophysical Environmental Interfaces In modelling complex environmental interface sys- tems, it is also interesting to consider the behaviour of the following system of two linearly coupled maps 12 11 nnn xεfrx ε ry (13) 21 11 nnn yεfryεfrx (14) where the map ,1 rx rxx is taken to be the logistic map with logistic parameters 1 r and 2 r while, is a coupling parameter. In the case of 12 rr , two maps are synchronized no matter what the initial conditions may be, i.e., coupled maps are identical with a single logistic map. Interesting is the case of 12 rr . In the following we fix the logistic parameters above and below the critical value 13.56994r for 1 r and 2 r respectively. We choose the logistic parameters 1 r and 2 r and regard the coupling parameter ε as the controlling parameter. In Figures 3 and 4, the attractors of the coupled-map are displayed as functions of cou- pling ε. Figure 3 shows the result of 14r and 23r while Figure 4 shows that of 14r and 22r . In both cases, for each value of ε we used the final value of the previous ε and 500 iterations were plotted. They are two typical examples of the various values of 1 r and 2 r. One immediately notices sev- eral interesting features. The fact that there are two cha- otic regions in both 0ε and 1ε ends seems odd at first sight, but after some reflection, one realizes that very weak ε means very strong 1ε, which brings chaos first to the variable x and then to y, however weak the coupling term may be. The most salient feature is the appearance of a stable period four cycle right after the period one around 0.77ε in Figure 3. Another case, found both in Figure 3 and Figure 4 cases, is the sudden filling of the x and y space around 0.85ε and above. The broad window-like region with period four around 0.9ε in the case of Figure 4 is also noteworthy. 1.0 0.5 0.0 0.00 0.25 0.50 0.75 1.00 0.00 0.25 0.50 0.75 1.00 ε x y 1.0 0.5 0.0 Figure 3. Phase diagram for the maps given by Eqs.13 and 14 with (1) 4.0r and (2) 3.0r, and 01 . For each value of , the map was iterated 1500 times from the initial point 0.2, 0.4xy to eliminate tran- sients, and the next 500 iterates were plotted.
 D. T. Mihailović et al. / Natural Science 3 (2011) 75-84 Copyright © 2011 SciRes. OPEN ACCESS 8181 1.0 0.5 0.0 ε 0.00 0.25 0.50 0.75 1.00 ε x y 1.0 0.5 0.00.00 0.25 0.50 0.75 1.00 Figure 4. Phase diagram for the maps given by Eqs.13 and 14 with (1) 4.0r and (2) 2.0r, and 01 . For each value of , the map was iterated 1500 times from the initial point 0.2, 0.4xy to eliminate tran- sients, and the next 500 iterates were plotted. 3.2. Lyapunov Exponent and Cross Sample Entropy of Map Representing Energy Exchange between Biophysical Environmental Interfaces Nonlinear dynamical analysis is powerful approach in understanding biophysical complex systems. We will consider two parameters included in the archive of this analysis Lyapunov exponent and cross sample entropy (Cross-SampEn). Consider the general vector mapping 1F, 0,1, nn xxn (15) and its thN iterate 1 FFF NN x with 1 FF x . The asymptotic behaviour of a series of iterates of the map can be characterized by the largest Lyapunov exponent, which, for an initial point 0 is an attracting region, is defined to be () 0 ln D( ) lim N N x λN (16) where is the norm of the Jacobi matrix D and D for the mappings F and FN respectively. For mapping given by Eqs.13 and 14 12 12 112 12 D 121 12 εrxεry εrx εry (17) This exponent measures how rapidly two nearby or- bits in attracting region converge or diverge. It can be evaluated by noting that 1 000 DDFD NN xx ; so if 01 2 ,, ,xxx are successive iterates of the map, then 0110 DDDD. N N xxx (18) In practice, λ is computed by initially iterating the map many times to eliminate transient behaviour and then using a large number N of successive points to compute the derivative matrix as indicated in Eq.18. Finally, the quantity () 0 ln D() N N is used as an approximate value of the Lyapunov exponent for the attracting region [29]. This exponent provides a way to distinguish among periodic, quasiperiodic, and chaotic motion. Specifically, if 0 is part of a stable periodic orbit of length K, then the norm of the derivative matrix DK will be less than one for every x in the K cycle. Thus the exponent will be negative and will char- acterize the rate at which small perturbations from the fixed cycle decay. A zero value for the exponent indi- cates quasiperiodic behaviour in which nearby paths maintain their distance on average. Finally, when λ be- comes positive, nearby points in the attracting region diverge from each other giving chaotic motion. In gen- eral, the exponent will depend on the initial point used in the iteration because there may be several stable attrac- tors, each with a separate basin of attraction [28]. We calculated the Lyapunov exponent λ to see the behaviour of the coupled maps given by Eqs.13 and 14 depending on different values of coupling parameter ε. Figures 5(a) an d 5(b) show Lyapunov exponent for the coupled maps as a function of ε ranging from 0 to 1. Each point was obtained by iterating 1500 times from the initial condition to eliminate transient behaviour and then averaging over another 500 iterations starting from initial condition (1) 0.20r and (2) 0.25r with 500 ε values. This simple analysis, where we consider Lyapu- nov exponent, shows a very interesting features of two coupled logistic maps representing interaction of two environmental interfaces, regarded as biophysical com- plex systems, through exchange of energy between them. For, example it is seen from Figure 5(a) and 5(b) that the region with positive Lyapunov exponent, respect to ε, is more emphasised when the one of the logistic map has larger values of the logistic parameter.
 D. T. Mihailović et al. / Natural Science 3 (2011) 75-84 Copyright © 2011 SciRes. OPEN ACCESS 82 (a) (b) (c) (d) Figure 5. Lyapunov exponent (a) and (b) and the Cross-Sem- ple Entropies (c) and (d) of the coupled maps as a function of coupling parameter ε ranging from 0 to 1 for parameter values with different the logistic parameters. The logistic equation with the logistic parameter r = 4.0 is coupled with the logistic equation having the following logistic parameters: (1) 2r and (2) 3r . Cross-SampEn measure of asynchrony is a recently introduced technique for comparing two different time series to assess their degree of asynchrony or dissimilar- ity [30,31]. Let 1, 2,uu uuN and 1, 2,vvvvN fix input parameters m and r. Vector sequences: ,1, 1xiui uiuim and ,1, 1yjvj vjvjm and N is the number of data points of time series, ,ij 1Nm . For each iNm set m i Brvu= (number of jNm such that , mm dxi yjr ) Nm, where j ranges from 1 to Nm. And then 1 Nm m i mi Brvu Brvu Nm (18) which is the average value of m i Bvu . Similarly we define m and m i as m i rvu = (number of jNm such that , mm dxi yjr ) Nm). 1 Nm m i mi rvu Arvu Nm (19)
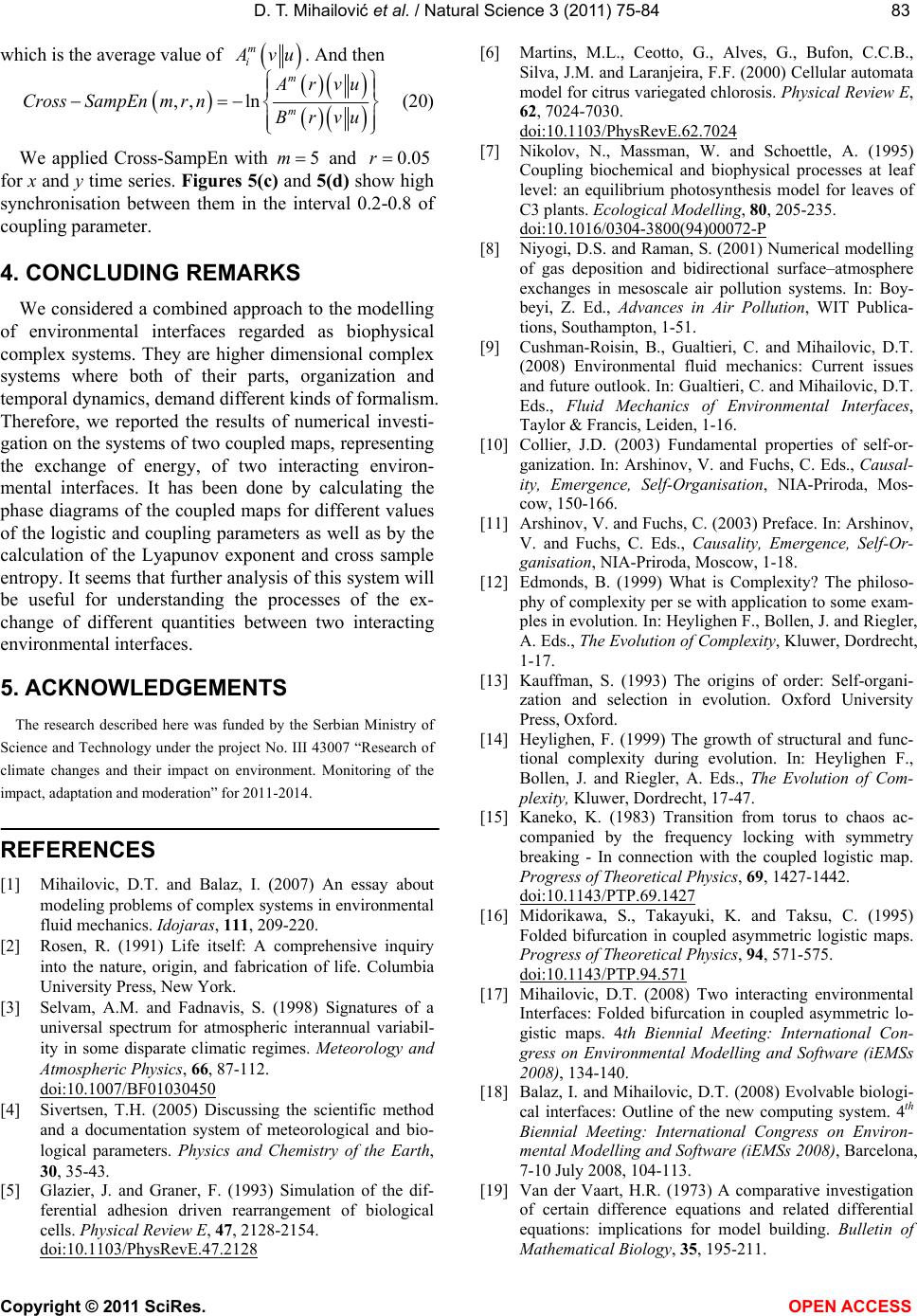 D. T. Mihailović et al. / Natural Science 3 (2011) 75-84 Copyright © 2011 SciRes. OPEN ACCESS 8383 which is the average value of m i vu . And then ,, ln m m rvu CrossSampEnmrnBrvu (20) We applied Cross-SampEn with 5m and 0.05r for x and y time series. Figures 5(c) and 5(d) show high synchronisation between them in the interval 0.2-0.8 of coupling parameter. 4. CONCLUDING REMARKS We considered a combined approach to the modelling of environmental interfaces regarded as biophysical complex systems. They are higher dimensional complex systems where both of their parts, organization and temporal dynamics, demand different kinds of formalism. Therefore, we reported the results of numerical investi- gation on the systems of two coupled maps, representing the exchange of energy, of two interacting environ- mental interfaces. It has been done by calculating the phase diagrams of the coupled maps for different values of the logistic and coupling parameters as well as by the calculation of the Lyapunov exponent and cross sample entropy. It seems that further analysis of this system will be useful for understanding the processes of the ex- change of different quantities between two interacting environmental interfaces. 5. ACKNOWLEDGEMENTS The research described here was funded by the Serbian Ministry of Science and Technology under the project No. III 43007 “Research of climate changes and their impact on environment. Monitoring of the impact, adaptation and moderation” for 2011-2014. REFERENCES [1] Mihailovic, D.T. and Balaz, I. (2007) An essay about modeling problems of complex systems in environmental fluid mechanics. Idojaras, 111, 209-220. [2] Rosen, R. (1991) Life itself: A comprehensive inquiry into the nature, origin, and fabrication of life. Columbia University Press, New York. [3] Selvam, A.M. and Fadnavis, S. (1998) Signatures of a universal spectrum for atmospheric interannual variabil- ity in some disparate climatic regimes. Meteorology and Atmospheric Physics, 66, 87-112. doi:10.1007/BF01030450 [4] Sivertsen, T.H. (2005) Discussing the scientific method and a documentation system of meteorological and bio- logical parameters. Physics and Chemistry of the Earth, 30, 35-43. [5] Glazier, J. and Graner, F. (1993) Simulation of the dif- ferential adhesion driven rearrangement of biological cells. Physical Review E, 47, 2128-2154. doi:10.1103/PhysRevE.47.2128 [6] Martins, M.L., Ceotto, G., Alves, G., Bufon, C.C.B., Silva, J.M. and Laranjeira, F.F. (2000) Cellular automata model for citrus variegated chlorosis. Physical Review E, 62, 7024-7030. doi:10.1103/PhysRevE.62.7024 [7] Nikolov, N., Massman, W. and Schoettle, A. (1995) Coupling biochemical and biophysical processes at leaf level: an equilibrium photosynthesis model for leaves of C3 plants. Ecological Modelling, 80, 205-235. doi:10.1016/0304-3800(94)00072-P [8] Niyogi, D.S. and Raman, S. (2001) Numerical modelling of gas deposition and bidirectional surface–atmosphere exchanges in mesoscale air pollution systems. In: Boy- beyi, Z. Ed., Advances in Air Pollution, WIT Publica- tions, Southampton, 1-51. [9] Cushman-Roisin, B., Gualtieri, C. and Mihailovic, D.T. (2008) Environmental fluid mechanics: Current issues and future outlook. In: Gualtieri, C. and Mihailovic, D.T. Eds., Fluid Mechanics of Environmental Interfaces, Taylor & Francis, Leiden, 1-16. [10] Collier, J.D. (2003) Fundamental properties of self-or- ganization. In: Arshinov, V. and Fuchs, C. Eds., Causal- ity, Emergence, Self-Organisation, NIA-Priroda, Mos- cow, 150-166. [11] Arshinov, V. and Fuchs, C. (2003) Preface. In: Arshinov, V. and Fuchs, C. Eds., Causality, Emergence, Self-Or- ganisation, NIA-Priroda, Moscow, 1-18. [12] Edmonds, B. (1999) What is Complexity? The philoso- phy of complexity per se with application to some exam- ples in evolution. In: Heylighen F., Bollen, J. and Riegler, A. Eds., The Evolution of Complexity, Kluwer, Dordrecht, 1-17. [13] Kauffman, S. (1993) The origins of order: Self-organi- zation and selection in evolution. Oxford University Press, Oxford. [14] Heylighen, F. (1999) The growth of structural and func- tional complexity during evolution. In: Heylighen F., Bollen, J. and Riegler, A. Eds., The Evolution of Com- plexity, Kluwer, Dordrecht, 17-47. [15] Kaneko, K. (1983) Transition from torus to chaos ac- companied by the frequency locking with symmetry breaking - In connection with the coupled logistic map. Progress of Theoretical Physics, 69, 1427-1442. doi:10.1143/PTP.69.1427 [16] Midorikawa, S., Takayuki, K. and Taksu, C. (1995) Folded bifurcation in coupled asymmetric logistic maps. Progress of Theoretical Physics, 94, 571-575. doi:10.1143/PTP.94.571 [17] Mihailovic, D.T. (2008) Two interacting environmental Interfaces: Folded bifurcation in coupled asymmetric lo- gistic maps. 4th Biennial Meeting: International Con- gress on Environmental Modelling and Software (iEMSs 2008), 134-140. [18] Balaz, I. and Mihailovic, D.T. (2008) Evolvable biologi- cal interfaces: Outline of the new computing system. 4th Biennial Meeting: International Congress on Environ- mental Modelling and Software (iEMSs 2008), Barcelona, 7-10 July 2008, 104-113. [19] Van der Vaart, H.R. (1973) A comparative investigation of certain difference equations and related differential equations: implications for model building. Bulletin of Mathematical Biology, 35, 195-211.
 D. T. Mihailović et al. / Natural Science 3 (2011) 75-84 Copyright © 2011 SciRes. OPEN ACCESS 84 [20] Bhumralkar, C.M. (1975) Numerical experiments on the computation of ground surface temperature in an atmos- pheric general circulation model. Journal of Applied Me- teorology, 14, 1246-1258. doi:10.1175/1520-0450(1975)014%3c1246:NEOTCO%3 e2.0.CO;2 [21] Holtslag A.A. and Van Ulden, A.P. (1983) A simple scheme for daytime estimates of the surface fluxes from routine weather data. Journal of Applied Meteorology, 22, 517-529. doi:10.1175/1520-0450(1983)022%3c0517:ASSFDE%3 e2.0.CO;2 [22] Kennel, M.B., Brown, R. and Abarbanel, H.D.I. (1992) Determining embedding dimension for phase-space re- construction using a geometrical construction. Physical Review A, 45, 3403-3411. doi:10.1103/PhysRevA.45.3403 [23] Richman, J.S. and Moorman, J.R. (2000) Physiological time-series analysis using approximate entropy and sam- ple entropy. American Journal of Physiology: Heart & Circulatory Physiology, 278, H2039-H2049. [24] Lake, D.E., Richman, J.S., Griffin, M.P. and Moorman, J.R. (2002) Sample entropy analysis of neonatal heart rate variability. American Journal of Physiology: Regu- latory Integrative and Comparative Physiology, 283, R789-R797. [25] Pincus, S.M. (1991) Approximate entropy as a measure of system complexity. Proceedings of the National Academy of Sciences of the United States, 88, 2297-2301. doi:10.1073/pnas.88.6.2297 [26] Bandt, C. and Pompe, B. (2002) Permutation entropy: A natural complexity measure for time series. Physical Re- view Letter, 88, 174102. doi:10.1103/PhysRevLett.88.174102 [27] Parker, T.S. and Chua, L.O. (1989) Practical Numerical Algorithms for Chaotic Systems. Springer-Verlag, New York. [28] Hogg, T. and Huberman, B.A. (1984) Generic behavior of coupled oscillators. Physical Review A, 29, 275-281. doi:10.1103/PhysRevA.29.275 [29] Feit, D. (1978) Characteristic exponents and strange attractors. Communications in Mathematical Physics, 61, 249. doi:10.1007/BF01940767 [30] Pincus, S. and Singer B.H. (1995) Randomness and de- grees of irregularity. Proceedings of the National Acad- emy of Sciences, 93, 2083-2088. doi:10.1073/pnas.93.5.2083 [31] Pincus, S.M., Mulligan, T., Iranmanesh, A., Gheorghiu, S., Godschalk, M. and Veldhuis J.D. (1996) Older males secrete luteinizing hormone and testosterone more ir- regularly, and jointly more asynchronously, than younger males. Proceedings of the National Academy of Sciences, 93, 14100-14105. doi:10.1073/pnas.93.24.14100
|