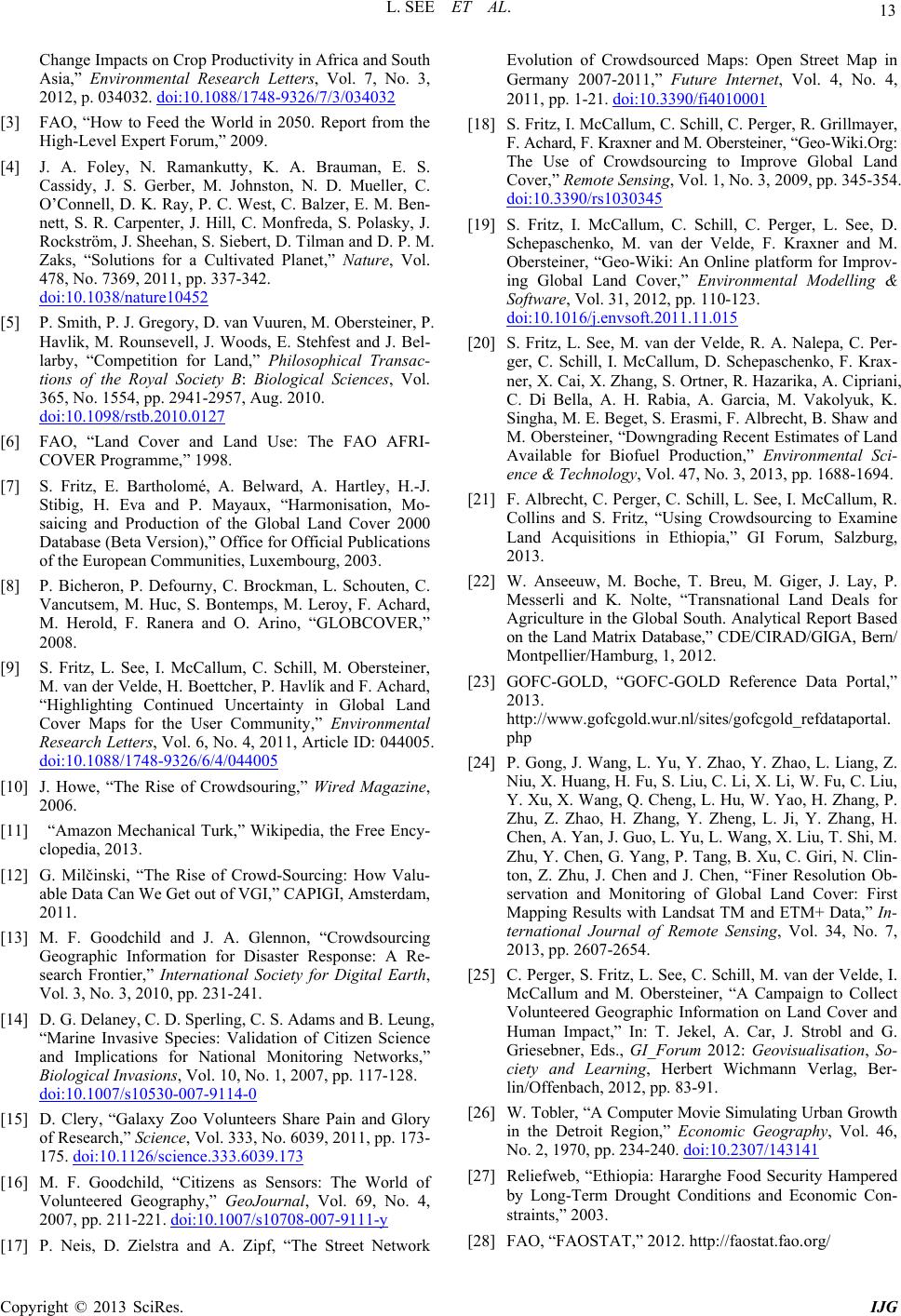
L. SEE ET AL.
Copyright © 2013 SciRes. IJG
13
Change Impacts on Crop Productivity in Africa and South
Asia,” Environmental Research Letters, Vol. 7, No. 3,
2012, p. 034032. doi:10.1088/1748-9326/7/3/034032
[3] FAO, “How to Feed the World in 2050. Report from the
High-Level Expert Forum,” 2009.
[4] J. A. Foley, N. Ramankutty, K. A. Brauman, E. S.
Cassidy, J. S. Gerber, M. Johnston, N. D. Mueller, C.
O’Connell, D. K. Ray, P. C. West, C. Balzer, E. M. Ben-
nett, S. R. Carpenter, J. Hill, C. Monfreda, S. Polasky, J.
Rockström, J. Sheehan, S. Siebert, D. Tilman and D. P. M.
Zaks, “Solutions for a Cultivated Planet,” Nature, Vol.
478, No. 7369, 2011, pp. 337-342.
doi:10.1038/nature10452
[5] P. Smith, P. J. Gregory, D. van Vuuren, M. Obersteiner, P.
Havlik, M. Rounsevell, J. Woods, E. Stehfest and J. Bel-
larby, “Competition for Land,” Philosophical Transac-
tions of the Royal Society B: Biological Sciences, Vol.
365, No. 1554, pp. 2941-2957, Aug. 2010.
doi:10.1098/rstb.2010.0127
[6] FAO, “Land Cover and Land Use: The FAO AFRI-
COVER Programme,” 1998.
[7] S. Fritz, E. Bartholomé, A. Belward, A. Hartley, H.-J.
Stibig, H. Eva and P. Mayaux, “Harmonisation, Mo-
saicing and Production of the Global Land Cover 2000
Database (Beta Version),” Office for Official Publications
of the European Communities, Luxembourg, 2003.
[8] P. Bicheron, P. Defourny, C. Brockman, L. Schouten, C.
Vancutsem, M. Huc, S. Bontemps, M. Leroy, F. Achard,
M. Herold, F. Ranera and O. Arino, “GLOBCOVER,”
2008.
[9] S. Fritz, L. See, I. McCallum, C. Schill, M. Obersteiner,
M. van der Velde, H. Boettcher, P. Havlík and F. Achard,
“Highlighting Continued Uncertainty in Global Land
Cover Maps for the User Community,” Environmental
Research Letters, Vol. 6, No. 4, 2011, Article ID: 044005.
doi:10.1088/1748-9326/6/4/044005
[10] J. Howe, “The Rise of Crowdsouring,” Wired Magazine,
2006.
[11] “Amazon Mechanical Turk,” Wikipedia, the Free Ency-
clopedia, 2013.
[12] G. Milčinski, “The Rise of Crowd-Sourcing: How Valu-
able Data Can We Get out of VGI,” CAPIGI, Amsterdam,
2011.
[13] M. F. Goodchild and J. A. Glennon, “Crowdsourcing
Geographic Information for Disaster Response: A Re-
search Frontier,” International Society for Digital Earth,
Vol. 3, No. 3, 2010, pp. 231-241.
[14] D. G. Delaney, C. D. Sperling, C. S. Adams and B. Leung,
“Marine Invasive Species: Validation of Citizen Science
and Implications for National Monitoring Networks,”
Biological Invasions, Vol. 10, No. 1, 2007, pp. 117-128.
doi:10.1007/s10530-007-9114-0
[15] D. Clery, “Galaxy Zoo Volunteers Share Pain and Glory
of Research,” Science, Vol. 333, No. 6039, 2011, pp. 173-
175. doi:10.1126/science.333.6039.173
[16] M. F. Goodchild, “Citizens as Sensors: The World of
Volunteered Geography,” GeoJournal, Vol. 69, No. 4,
2007, pp. 211-221. doi:10.1007/s10708-007-9111-y
[17] P. Neis, D. Zielstra and A. Zipf, “The Street Network
Evolution of Crowdsourced Maps: Open Street Map in
Germany 2007-2011,” Future Internet, Vol. 4, No. 4,
2011, pp. 1-21. doi:10.3390/fi4010001
[18] S. Fritz, I. McCallum, C. Schill, C. Perger, R. Grillmayer,
F. Achard, F. Kraxner and M. Obersteiner, “Geo-Wiki.Org:
The Use of Crowdsourcing to Improve Global Land
Cover,” Remote Sensing, Vol. 1, No. 3, 2009, pp. 345-354.
doi:10.3390/rs1030345
[19] S. Fritz, I. McCallum, C. Schill, C. Perger, L. See, D.
Schepaschenko, M. van der Velde, F. Kraxner and M.
Obersteiner, “Geo-Wiki: An Online platform for Improv-
ing Global Land Cover,” Environmental Modelling &
Software, Vol. 31, 2012, pp. 110-123.
doi:10.1016/j.envsoft.2011.11.015
[20] S. Fritz, L. See, M. van der Velde, R. A. Nalepa, C. Per-
ger, C. Schill, I. McCallum, D. Schepaschenko, F. Krax-
ner, X. Cai, X. Zhang, S. Ortner, R. Hazarika, A. Cipriani,
C. Di Bella, A. H. Rabia, A. Garcia, M. Vakolyuk, K.
Singha, M. E. Beget, S. Erasmi, F. Albrecht, B. Shaw and
M. Obersteiner, “Downgrading Recent Estimates of Land
Available for Biofuel Production,” Environmental Sci-
ence & Technology, Vol. 47, No. 3, 2013, pp. 1688-1694.
[21] F. Albrecht, C. Perger, C. Schill, L. See, I. McCallum, R.
Collins and S. Fritz, “Using Crowdsourcing to Examine
Land Acquisitions in Ethiopia,” GI Forum, Salzburg,
2013.
[22] W. Anseeuw, M. Boche, T. Breu, M. Giger, J. Lay, P.
Messerli and K. Nolte, “Transnational Land Deals for
Agriculture in the Global South. Analytical Report Based
on the Land Matrix Database,” CDE/CIRAD/GIGA, Bern/
Montpellier/Hamburg, 1, 2012.
[23] GOFC-GOLD, “GOFC-GOLD Reference Data Portal,”
2013.
http://www.gofcgold.wur.nl/sites/gofcgold_refdataportal.
php
[24] P. Gong, J. Wang, L. Yu, Y. Zhao, Y. Zhao, L. Liang, Z.
Niu, X. Huang, H. Fu, S. Liu, C. Li, X. Li, W. Fu, C. Liu,
Y. Xu, X. Wang, Q. Cheng, L. Hu, W. Yao, H. Zhang, P.
Zhu, Z. Zhao, H. Zhang, Y. Zheng, L. Ji, Y. Zhang, H.
Chen, A. Yan, J. Guo, L. Yu, L. Wang, X. Liu, T. Shi, M.
Zhu, Y. Chen, G. Yang, P. Tang, B. Xu, C. Giri, N. Clin-
ton, Z. Zhu, J. Chen and J. Chen, “Finer Resolution Ob-
servation and Monitoring of Global Land Cover: First
Mapping Results with Landsat TM and ETM+ Data,” In-
ternational Journal of Remote Sensing, Vol. 34, No. 7,
2013, pp. 2607-2654.
[25] C. Perger, S. Fritz, L. See, C. Schill, M. van der Velde, I.
McCallum and M. Obersteiner, “A Campaign to Collect
Volunteered Geographic Information on Land Cover and
Human Impact,” In: T. Jekel, A. Car, J. Strobl and G.
Griesebner, Eds., GI_Forum 2012: Geovisualisation, So-
ciety and Learning, Herbert Wichmann Verlag, Ber-
lin/Offenbach, 2012, pp. 83-91.
[26] W. Tobler, “A Computer Movie Simulating Urban Growth
in the Detroit Region,” Economic Geography, Vol. 46,
No. 2, 1970, pp. 234-240. doi:10.2307/143141
[27] Reliefweb, “Ethiopia: Hararghe Food Security Hampered
by Long-Term Drought Conditions and Economic Con-
straints,” 2003.
[28] FAO, “FAOSTAT,” 2012. http://faostat.fao.org/