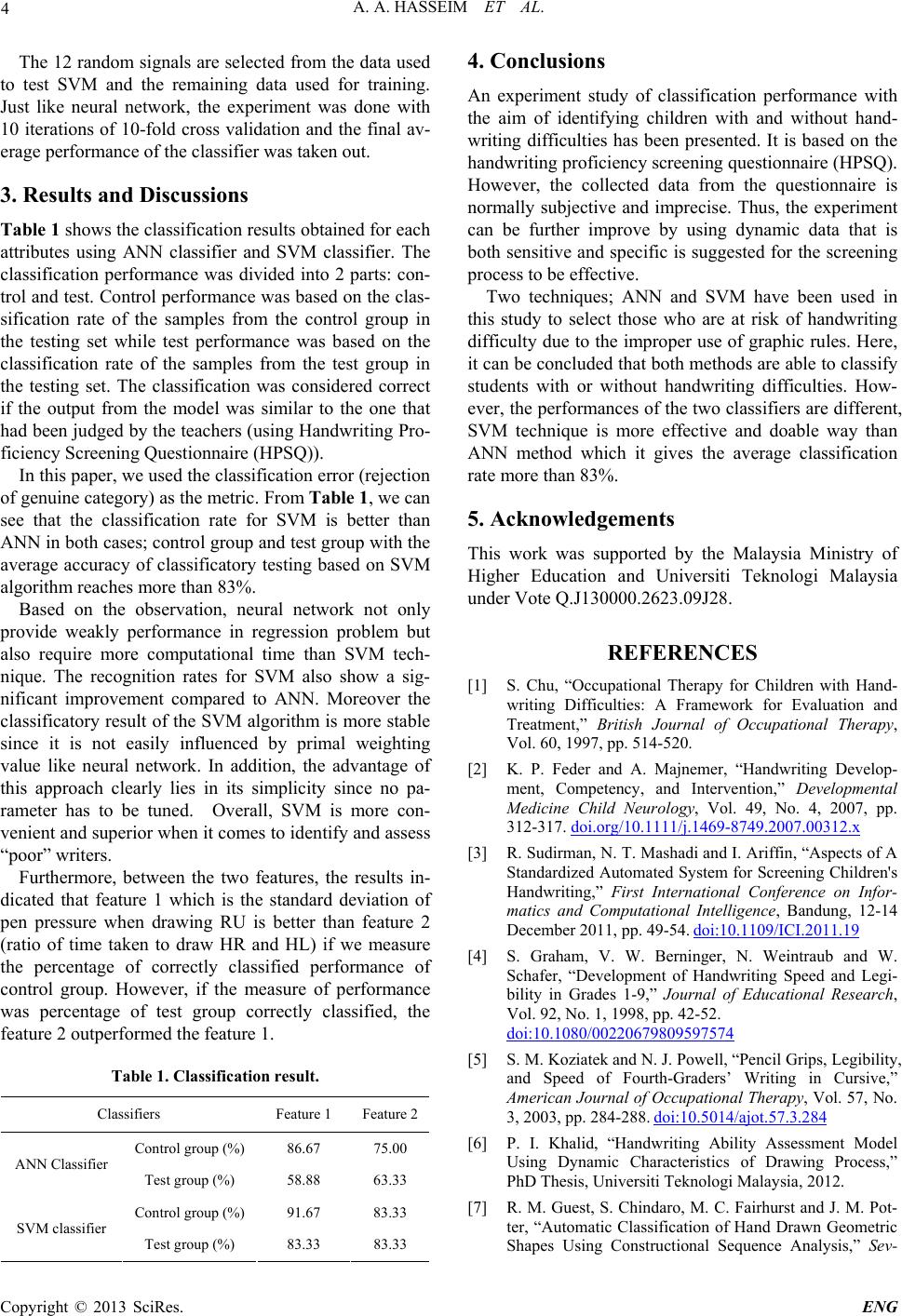
A. A. HASSEIM ET AL.
4
The 12 random signals are selected from the data used
to test SVM and the remaining data used for training.
Just like neural network, the experiment was done with
10 iterations of 10-fold cross validation and the final av-
erage performance of the classifier was taken out.
3. Results and Discussions
Table 1 shows the classification results obtained for each
attributes using ANN classifier and SVM classifier. The
classification performance was divided into 2 parts: con-
trol and test. Control performance was based on the clas-
sification rate of the samples from the control group in
the testing set while test performance was based on the
classification rate of the samples from the test group in
the testing set. The classification was considered correct
if the output from the model was similar to the one that
had been judged by the teachers (using Handwriting Pro-
ficiency Screening Questionnaire (HPSQ)).
In this paper, we used the classification error (rejection
of genuine category) as the metric. From Table 1, we can
see that the classification rate for SVM is better than
ANN in both cases; control group and test group with the
average accuracy of classificatory testing based on SVM
algorithm reaches more than 83%.
Based on the observation, neural network not only
provide weakly performance in regression problem but
also require more computational time than SVM tech-
nique. The recognition rates for SVM also show a sig-
nificant improvement compared to ANN. Moreover the
classificatory result of the SVM algorithm is more stable
since it is not easily influenced by primal weighting
value like neural network. In addition, the advantage of
this approach clearly lies in its simplicity since no pa-
rameter has to be tuned. Overall, SVM is more con-
venient and superior when it comes to identify and assess
“poor” writers.
Furthermore, between the two features, the results in-
dicated that feature 1 which is the standard deviation of
pen pressure when drawing RU is better than feature 2
(ratio of time taken to draw HR and HL) if we measure
the percentage of correctly classified performance of
control group. However, if the measure of performance
was percentage of test group correctly classified, the
feature 2 outperformed the feature 1.
Table 1. Classification result.
Classifiers Feature 1 Feature 2
Control group (%) 86.67 75.00
ANN Classifier
Test group (%) 58.88 63.33
Control group (%) 91.67 83.33
SVM classifier
Test group (%) 83.33 83.33
4. Conclusions
An experiment study of classification performance with
the aim of identifying children with and without hand-
writing difficulties has been presented. It is based on the
handwriting proficiency screening questionnaire (HPSQ).
However, the collected data from the questionnaire is
normally subjective and imprecise. Thus, the experiment
can be further improve by using dynamic data that is
both sensitive and specific is suggested for the screening
process to be effective.
Two techniques; ANN and SVM have been used in
this study to select those who are at risk of handwriting
difficulty due to the improper use of graphic rules. Here,
it can be concluded that both methods are able to classify
students with or without handwriting difficulties. How-
ever, the performances of the two classifiers are different,
SVM technique is more effective and doable way than
ANN method which it gives the average classification
rate more than 83%.
5. Acknowledgements
This work was supported by the Malaysia Ministry of
Higher Education and Universiti Teknologi Malaysia
under Vote Q.J130000.2623.09J28.
REFERENCES
[1] S. Chu, “Occupational Therapy for Children with Hand-
writing Difficulties: A Framework for Evaluation and
Treatment,” British Journal of Occupational Therapy,
Vol. 60, 1997, pp. 514-520.
[2] K. P. Feder and A. Majnemer, “Handwriting Develop-
ment, Competency, and Intervention,” Developmental
Medicine Child Neurology, Vol. 49, No. 4, 2007, pp.
312-317. doi.org/10.1111/j.1469-8749.2007.00312.x
[3] R. Sudirman, N. T. Mashadi and I. Ariffin, “Aspects of A
Standardized Automated System for Screening Children's
Handwriting,” First International Conference on Infor-
matics and Computational Intelligence, Bandung, 12-14
December 2011, pp. 49-54. doi:10.1109/ICI.2011.19
[4] S. Graham, V. W. Berninger, N. Weintraub and W.
Schafer, “Development of Handwriting Speed and Legi-
bility in Grades 1-9,” Journal of Educational Research,
Vol. 92, No. 1, 1998, pp. 42-52.
doi:10.1080/00220679809597574
[5] S. M. Koziatek and N. J. Powell, “Pencil Grips, Legibility,
and Speed of Fourth-Graders’ Writing in Cursive,”
American Journal of Occupational Therapy, Vol. 57, No.
3, 2003, pp. 284-288. doi:10.5014/ajot.57.3.284
[6] P. I. Khalid, “Handwriting Ability Assessment Model
Using Dynamic Characteristics of Drawing Process,”
PhD Thesis, Universiti Teknologi Malaysia, 2012.
[7] R. M. Guest, S. Chindaro, M. C. Fairhurst and J. M. Pot-
ter, “Automatic Classification of Hand Drawn Geometric
Shapes Using Constructional Sequence Analysis,” Sev-
Copyright © 2013 SciRes. ENG