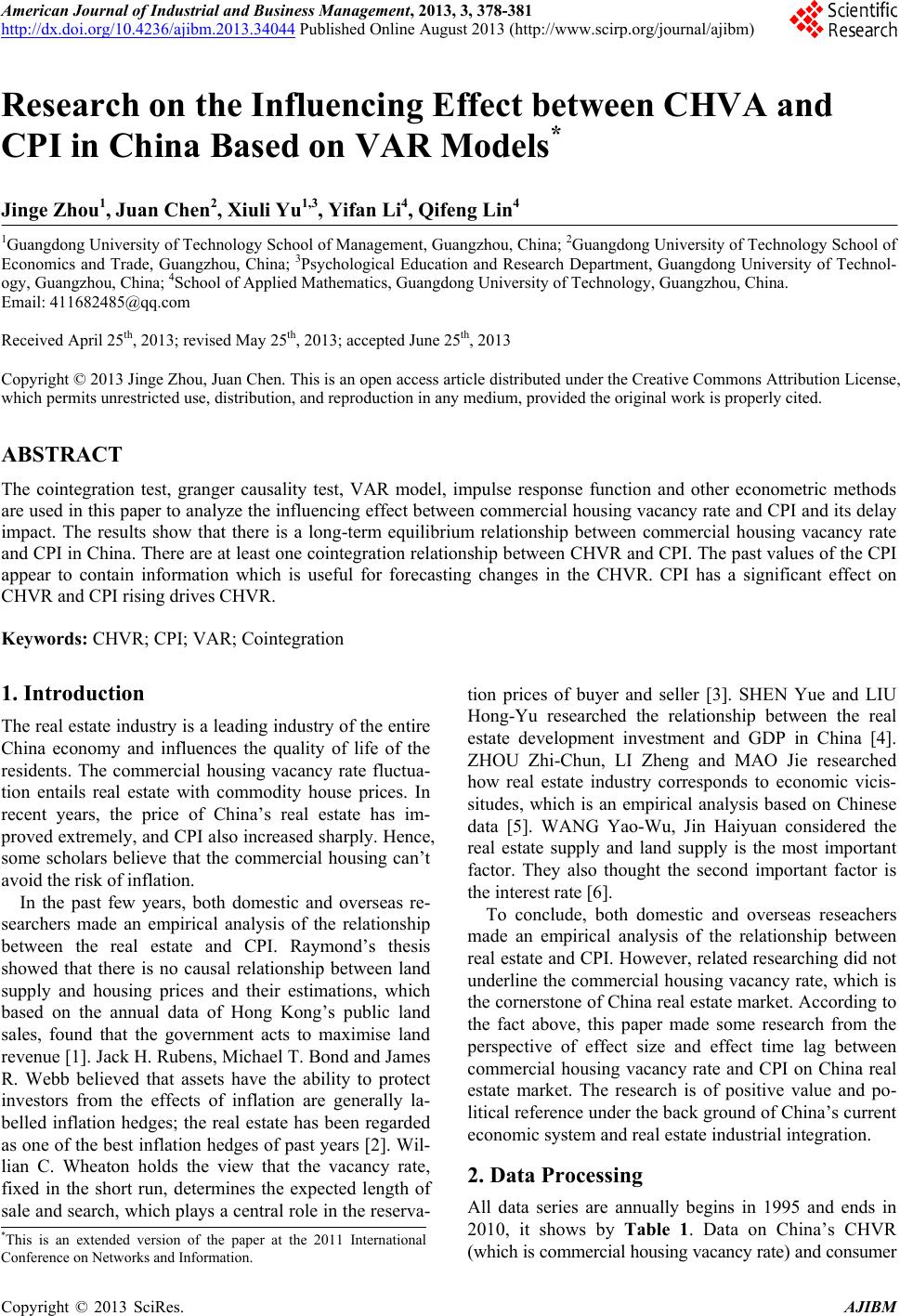
American Journal of Industrial and Business Management, 2013, 3, 378-381
http://dx.doi.org/10.4236/ajibm.2013.34044 Published Online August 2013 (http://www.scirp.org/journal/ajibm)
Research on the Influencing Effect between CHVA and
CPI in China Based on VAR Models*
Jinge Zhou1, Juan Chen2, Xiuli Yu1,3, Yifan Li4, Qifeng Lin4
1Guangdong University of Technology School of Management, Guangzhou, China; 2Guangdong University of Technology School of
Economics and Trade, Guangzhou, China; 3Psychological Education and Research Department, Guangdong University of Technol-
ogy, Guangzhou, China; 4School of Applied Mathematics, Guangdong University of Technology, Guangzhou, China.
Email: 411682485@qq.com
Received April 25th, 2013; revised May 25th, 2013; accepted June 25th, 2013
Copyright © 2013 Jinge Zhou, Juan Chen. This is an open access article distributed under the Creative Commons Attribution License,
which permits unrestricted use, distribution, and reproduction in any medium, provided the original work is properly cited.
ABSTRACT
The cointegration test, granger causality test, VAR model, impulse response function and other econometric methods
are used in this paper to analyze the influencing effect between commercial housing vacancy rate and CPI and its delay
impact. The results show that there is a long-term equilibrium relationship between commercial housing vacancy rate
and CPI in China. There are at least one cointegration relationship between CHVR and CPI. The past values of the CPI
appear to contain information which is useful for forecasting changes in the CHVR. CPI has a significant effect on
CHVR and CPI rising drives CHVR.
Keywords: CHVR; CPI; VAR; Cointegration
1. Introduction
The real estate industry is a leading industry of the entire
China economy and influences the quality of life of the
residents. The commercial housing vacancy rate fluctua-
tion entails real estate with commodity house prices. In
recent years, the price of China’s real estate has im-
proved extremely, and CPI also increased sharply. Hence,
some scholars believe that the commercial housing can’t
avoid the risk of inflation.
In the past few years, both domestic and overseas re-
searchers made an empirical analysis of the relationship
between the real estate and CPI. Raymond’s thesis
showed that there is no causal relationship between land
supply and housing prices and their estimations, which
based on the annual data of Hong Kong’s public land
sales, found that the government acts to maximise land
revenue [1]. Jack H. Rubens, Michael T. Bond and James
R. Webb believed that assets have the ability to protect
investors from the effects of inflation are generally la-
belled inflation hedges; the real estate has been regarded
as one of the best inflation hedges of past years [2]. Wil-
lian C. Wheaton holds the view that the vacancy rate,
fixed in the short run, determines the expected length of
sale and search, which plays a central role in the reserva-
tion prices of buyer and seller [3]. SHEN Yue and LIU
Hong-Yu researched the relationship between the real
estate development investment and GDP in China [4].
ZHOU Zhi-Chun, LI Zheng and MAO Jie researched
how real estate industry corresponds to economic vicis-
situdes, which is an empirical analysis based on Chinese
data [5]. WANG Yao-Wu, Jin Haiyuan considered the
real estate supply and land supply is the most important
factor. They also thought the second important factor is
the interest rate [6].
To conclude, both domestic and overseas reseachers
made an empirical analysis of the relationship between
real estate and CPI. However, related researching did not
underline the commercial housing vacancy rate, which is
the cornerstone of China real estate market. According to
the fact above, this paper made some research from the
perspective of effect size and effect time lag between
commercial housing vacancy rate and CPI on China real
estate market. The research is of positive value and po-
litical reference under the back ground of China’s current
economic system and real estate industrial integration.
2. Data Processing
All data series are annually begins in 1995 and ends in
2010, it shows by Table 1. Data on China’s CHVR
(which is commercial housing vacancy rate) and consumer
*This is an extended version of the paper at the 2011 International
Conference on Networks and Information.
Copyright © 2013 SciRes. AJIBM