 Psychology 2013. Vol.4, No.3A, 363-373 Published Online March 2013 in SciRes (http://www.scirp.org/journal/psych) http://dx.doi.org/10.4236/psych.2013.43A053 Copyright © 2013 SciRes. 363 Re-Evaluation of Attractor Neural Network Model to Explain Double Dissociation in Semantic Memory Disorder* Shin-ichi Asakawa Center for Information Sciences, Tokyo Woman’s Christian University, Tokyo, Japan Email: asakawa@ieee.org Received December 20th, 2012; revised January 20th, 2013; accepted February 15th, 2013 Structure of semantic memory was investigated in the way of neural network simulations in detail. In the literature, it is well-known that brain damaged patients often showed category specific disorder in various cognitive neuropsychological tasks like picture naming, categorisation, identification tasks and so on. In order to describe semantic memory disorder of brain damaged patients, the attractor neural network model originally proposed Hinton and Shallice (1991) was employed and was tried to re-evaluate the model performance. Especially, in order to answer the question about organization of semantic memory, how our semantic memories are organized, computer simulations were conducted. After the model learned data set (Tyler, Moss, Durrant-Peatfield, & Levy, 2000), units in hidden and cleanup layers were removed and observed its performances. The results showed category specificity. This model could also explain the double dissociation phenomena. In spite of the simplicity of its architecture, the attractor neural network might be considered to mimic human behavior in the meaning of semantic memory organization and its disorder. Although this model could explain various phenomenon in cognitive neuropsychology, it might become obvious that this model had one limitation to explain human behavior. As far as investigation in this study, asymmetry in category specificity between animate and inanimate objects might not be ex- plained on this model without any additional assumptions. Therefore, further studies must be required to improve our understanding for semantic memory organisation. Keywords: Attractor Neural Network; Double Dissociation; Category Specificity; Semantic Memory; Brain Damage Introduction Cognitive neuropsychological evidence about semantic memory disorder have given deep impacts to studies of cogni- tive science and psychology. Among the cognitive neuropsy- chological data, disorder about distinction between animate and inanimate objects is suggestive in order to understand organiza- tion of our semantic memories. Because patients with semantic memory disorder often have tendency known as “double disso- ciation”. Some patients show deficits in identification, naming, and categorization tasks of animate objects, but their knowl- edge of inanimate objects (i.e. tools, outdoor objects, jewelries, body parts, and so on) remains intact (Caramazza & Shelton, 1998; De Renzi & Lucchelli, 1994; Hillis & Caramazza, 1991; Warrington & Shallice, 1984). On the other hand, there exits another kind of patients who are not able to identify, to name, and to categorize inanimate objects. However, their knowledge about animals remains intact (Hillis & Caramazza, 1991; War- rington & McCarthy, 1987). Although many studies controlled for confounding factors such as familiarity and frequency (Ca- ramazza & Shelton, 1998; De Renzi & Lucchelli, 1994), these factors failed to explain explain the double dissociation. In the literature, this double dissociation was first described by Niel- sen (1946) Capitani, Laiaconna, Mahon, and Caramazza (2003) reviewed evidences in category specific processing in the hu- man brain which has selective impairments in recognizing par- ticular types of objects. Based upon their clinical evidences, Warrington and her colleagues (Warrington, 1981; Warrington & McCarthy, 1983; Warrington & Shallice, 1984; Warrington & McCarthy, 1994) have tried to explain that the structure of semantic memory and its nature. Would these data suggest that different contents of semantic memory are localized in the brain (maybe the left lateral inferior gurus)? Might these data suggest that the information of these two categories are stored in dis- tributed manner in the brain? Or might these data emerge from the inter- and intra-correlations between objects? In this paper, it was intended to focus upon these questions. Neuroimaging Studies Neuroimaging studies revealed a similar double dissociation. In a review of functional neuroimaging studies in normal sub- jects, Martin and Chao (2001), Martin and Caramazza (2003) mentioned that animate objects had tendency to show peak activity in both the lateral portion of the fusiform gyrus in both hemispheres and the right superior temporal sulcus while in- animate objects had tendency to show peak activity in the me- dial portion of the fusiform gyrus, the left middle temporal gyrus, and the ventral premotor and parietal cortex in the left hemisphere. Similar conclusions have been made in other re- view papers (Josephs, 2001; Lewis, 2006; Thompson-Schill, 2003). These areas are possible candidates responsible to per- form semantic memory tasks. However, it is worth noticing that these findings might be inconsistent with cognitive neuropsy- chological findings (see the next section). *The author would like to thank Sachiyo Iwafune for her help.
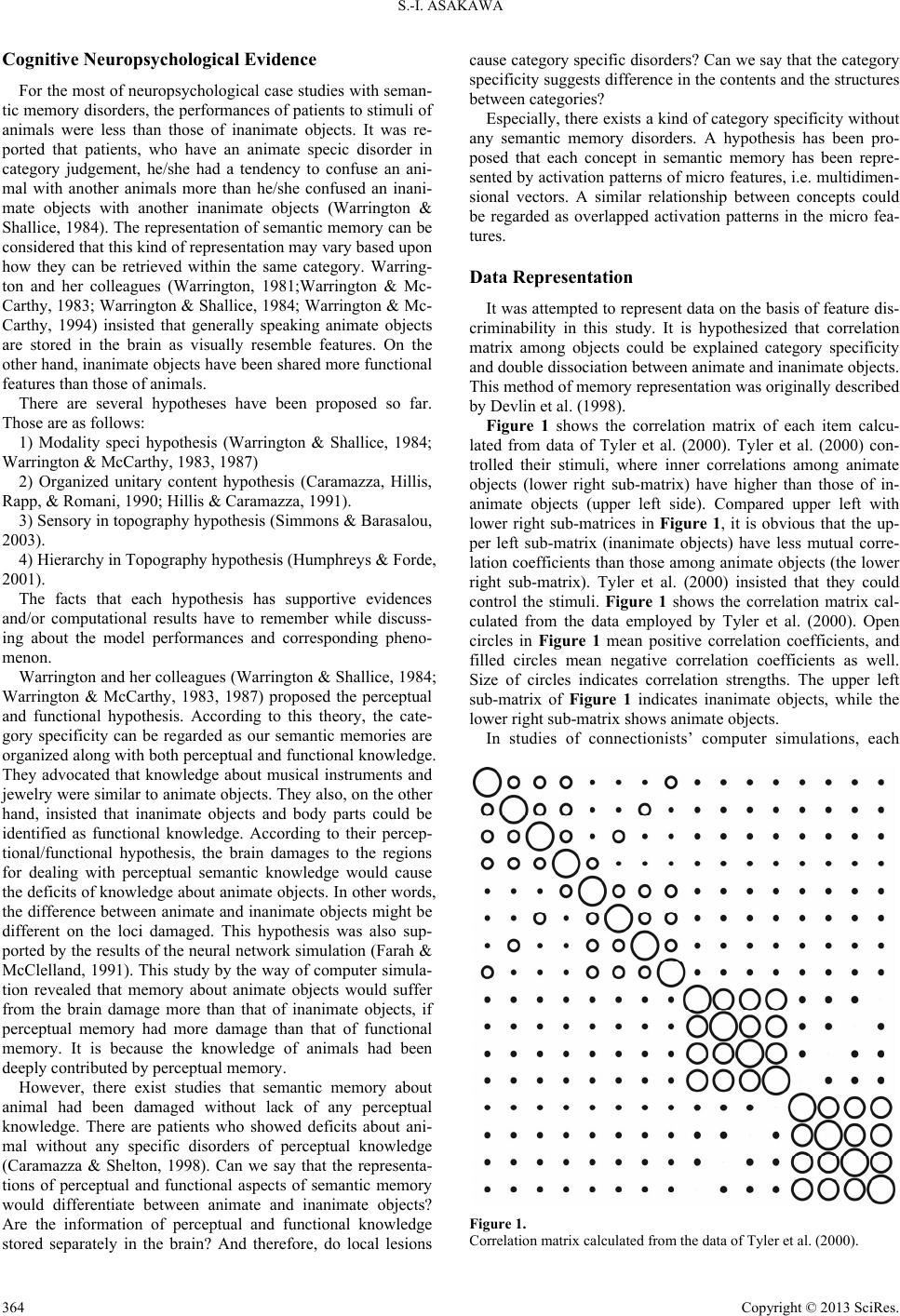 S.-I. ASAKAWA Cognitive Neuropsychologic al Evide nce For the most of neuropsychological case studies with seman- tic memory disorders, the performances of patients to stimuli of animals were less than those of inanimate objects. It was re- ported that patients, who have an animate specic disorder in category judgement, he/she had a tendency to confuse an ani- mal with another animals more than he/she confused an inani- mate objects with another inanimate objects (Warrington & Shallice, 1984). The representation of semantic memory can be considered that this kind of representation may vary based upon how they can be retrieved within the same category. Warring- ton and her colleagues (Warrington, 1981;Warrington & Mc- Carthy, 1983; Warrington & Shallice, 1984; Warrington & Mc- Carthy, 1994) insisted that generally speaking animate objects are stored in the brain as visually resemble features. On the other hand, inanimate objects have been shared more functional features than those of animals. There are several hypotheses have been proposed so far. Those are as follows: 1) Modality speci hypothesis (Warrington & Shallice, 1984; Warrington & McCarthy, 1983, 1987) 2) Organized unitary content hypothesis (Caramazza, Hillis, Rapp, & Romani, 1990; Hillis & Caramazza, 1991). 3) Sensory in topography hypothesis (Simmons & Barasalou, 2003). 4) Hierarchy in Topography hypothesis (Humphreys & Forde, 2001). The facts that each hypothesis has supportive evidences and/or computational results have to remember while discuss- ing about the model performances and corresponding pheno- menon. Warrington and her colleagues (Warrington & Shallice, 1984; Warrington & McCarthy, 1983, 1987) proposed the perceptual and functional hypothesis. According to this theory, the cate- gory specificity can be regarded as our semantic memories are organized along with both perceptual and functional knowledge. They advocated that knowledge about musical instruments and jewelry were similar to animate objects. They also, on the other hand, insisted that inanimate objects and body parts could be identified as functional knowledge. According to their percep- tional/functional hypothesis, the brain damages to the regions for dealing with perceptual semantic knowledge would cause the deficits of knowledge about animate objects. In other words, the difference between animate and inanimate objects might be different on the loci damaged. This hypothesis was also sup- ported by the results of the neural network simulation (Farah & McClelland, 1991). This study by the way of computer simula- tion revealed that memory about animate objects would suffer from the brain damage more than that of inanimate objects, if perceptual memory had more damage than that of functional memory. It is because the knowledge of animals had been deeply contributed by perceptual memory. However, there exist studies that semantic memory about animal had been damaged without lack of any perceptual knowledge. There are patients who showed deficits about ani- mal without any specific disorders of perceptual knowledge (Caramazza & Shelton, 1998). Can we say that the representa- tions of perceptual and functional aspects of semantic memory would differentiate between animate and inanimate objects? Are the information of perceptual and functional knowledge stored separately in the brain? And therefore, do local lesions cause category specific disorders? Can we say that the category specificity suggests difference in the contents and the structures between categories? Especially, there exists a kind of category specificity without any semantic memory disorders. A hypothesis has been pro- posed that each concept in semantic memory has been repre- sented by activation patterns of micro features, i.e. multidimen- sional vectors. A similar relationship between concepts could be regarded as overlapped activation patterns in the micro fea- tures. Data Representation It was attempted to represent data on the basis of feature dis- criminability in this study. It is hypothesized that correlation matrix among objects could be explained category specificity and double dissociation between animate and inanimate objects. This method of memory representation was originally described by Devlin et al. (1998). Figure 1 shows the correlation matrix of each item calcu- lated from data of Tyler et al. (2000). Tyler et al. (2000) con- trolled their stimuli, where inner correlations among animate objects (lower right sub-matrix) have higher than those of in- animate objects (upper left side). Compared upper left with lower right sub-matrices in Figure 1, it is obvious that the up- per left sub-matrix (inanimate objects) have less mutual corre- lation coefficients than those among animate objects (the lower right sub-matrix). Tyler et al. (2000) insisted that they could control the stimuli. Figure 1 shows the correlation matrix cal- culated from the data employed by Tyler et al. (2000). Open circles in Figure 1 mean positive correlation coefficients, and filled circles mean negative correlation coefficients as well. Size of circles indicates correlation strengths. The upper left sub-matrix of Figure 1 indicates inanimate objects, while the lower right sub-matrix shows animate objects. In studies of connectionists’ computer simulations, each Figure 1. Correlation matrix calculated from the data of Tyler et al. (2000). Copyright © 2013 SciRes. 364
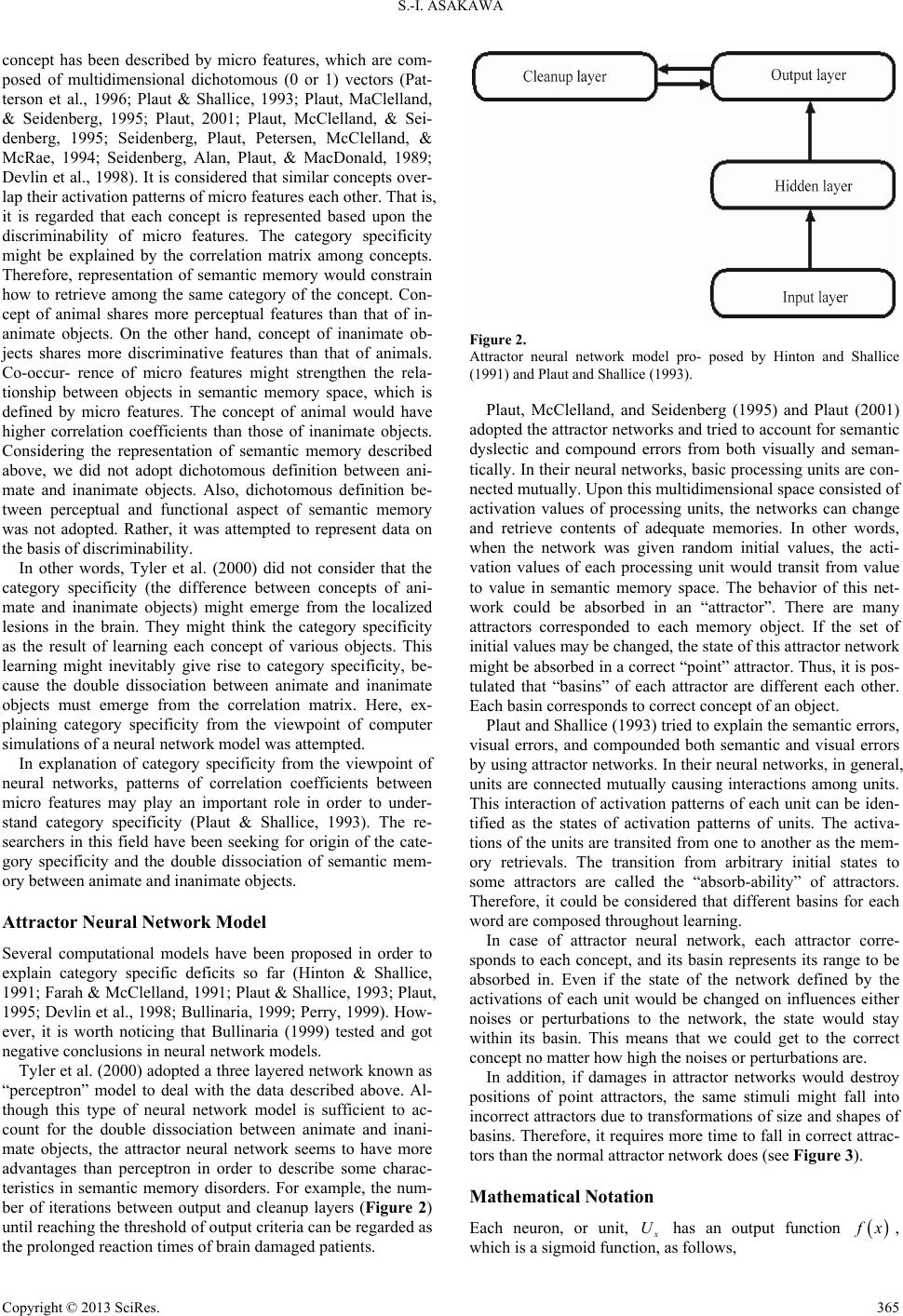 S.-I. ASAKAWA concept has been described by micro features, which are com- posed of multidimensional dichotomous (0 or 1) vectors (Pat- terson et al., 1996; Plaut & Shallice, 1993; Plaut, MaClelland, & Seidenberg, 1995; Plaut, 2001; Plaut, McClelland, & Sei- denberg, 1995; Seidenberg, Plaut, Petersen, McClelland, & McRae, 1994; Seidenberg, Alan, Plaut, & MacDonald, 1989; Devlin et al., 1998). It is considered that similar concepts over- lap their activation patterns of micro features each other. That is, it is regarded that each concept is represented based upon the discriminability of micro features. The category specificity might be explained by the correlation matrix among concepts. Therefore, representation of semantic memory would constrain how to retrieve among the same category of the concept. Con- cept of animal shares more perceptual features than that of in- animate objects. On the other hand, concept of inanimate ob- jects shares more discriminative features than that of animals. Co-occur- rence of micro features might strengthen the rela- tionship between objects in semantic memory space, which is defined by micro features. The concept of animal would have higher correlation coefficients than those of inanimate objects. Considering the representation of semantic memory described above, we did not adopt dichotomous definition between ani- mate and inanimate objects. Also, dichotomous definition be- tween perceptual and functional aspect of semantic memory was not adopted. Rather, it was attempted to represent data on the basis of discriminability. In other words, Tyler et al. (2000) did not consider that the category specificity (the difference between concepts of ani- mate and inanimate objects) might emerge from the localized lesions in the brain. They might think the category specificity as the result of learning each concept of various objects. This learning might inevitably give rise to category specificity, be- cause the double dissociation between animate and inanimate objects must emerge from the correlation matrix. Here, ex- plaining category specificity from the viewpoint of computer simulations of a neural network model was attempted. In explanation of category specificity from the viewpoint of neural networks, patterns of correlation coefficients between micro features may play an important role in order to under- stand category specificity (Plaut & Shallice, 1993). The re- searchers in this field have been seeking for origin of the cate- gory specificity and the double dissociation of semantic mem- ory between animate and inanimate objects. Attractor Neural Network Model Several computational models have been proposed in order to explain category specific deficits so far (Hinton & Shallice, 1991; Farah & McClelland, 1991; Plaut & Shallice, 1993; Plaut, 1995; Devlin et al., 1998; Bullinaria, 1999; Perry, 1999). How- ever, it is worth noticing that Bullinaria (1999) tested and got negative conclusions in neural network models. Tyler et al. (2000) adopted a three layered network known as “perceptron” model to deal with the data described above. Al- though this type of neural network model is sufficient to ac- count for the double dissociation between animate and inani- mate objects, the attractor neural network seems to have more advantages than perceptron in order to describe some charac- teristics in semantic memory disorders. For example, the num- ber of iterations between output and cleanup layers (Figure 2) until reaching the threshold of output criteria can be regarded as the prolonged reaction times of brain damaged patients. Figure 2. Attractor neural network model pro- posed by Hinton and Shallice (1991) and Plaut and Shallice (1993). Plaut, McClelland, and Seidenberg (1995) and Plaut (2001) adopted the attractor networks and tried to account for semantic dyslectic and compound errors from both visually and seman- tically. In their neural networks, basic processing units are con- nected mutually. Upon this multidimensional space consisted of activation values of processing units, the networks can change and retrieve contents of adequate memories. In other words, when the network was given random initial values, the acti- vation values of each processing unit would transit from value to value in semantic memory space. The behavior of this net- work could be absorbed in an “attractor”. There are many attractors corresponded to each memory object. If the set of initial values may be changed, the state of this attractor network might be absorbed in a correct “point” attractor. Thus, it is pos- tulated that “basins” of each attractor are different each other. Each basin corresponds to correct concept of an object. Plaut and Shallice (1993) tried to explain the semantic errors, visual errors, and compounded both semantic and visual errors by using attractor networks. In their neural networks, in general, units are connected mutually causing interactions among units. This interaction of activation patterns of each unit can be iden- tified as the states of activation patterns of units. The activa- tions of the units are transited from one to another as the mem- ory retrievals. The transition from arbitrary initial states to some attractors are called the “absorb-ability” of attractors. Therefore, it could be considered that different basins for each word are composed throughout learning. In case of attractor neural network, each attractor corre- sponds to each concept, and its basin represents its range to be absorbed in. Even if the state of the network defined by the activations of each unit would be changed on influences either noises or perturbations to the network, the state would stay within its basin. This means that we could get to the correct concept no matter how high the noises or perturbations are. In addition, if damages in attractor networks would destroy positions of point attractors, the same stimuli might fall into incorrect attractors due to transformations of size and shapes of basins. Therefore, it requires more time to fall in correct attrac- tors than the normal attractor network does (see Figure 3). Mathematical Notation Each neuron, or unit, x U has an output function x, which is a sigmoid function, as follows, Copyright © 2013 SciRes. 365
 S.-I. ASAKAWA Figure 3. Schematic description of basins of attractor neural network model and its modification by damages against the model. 1 == 1e xax Ufx . (1) Throughout the numerical experiments in this study, it was fixed a constant . The units in the hidden layer = 4.0a h U can be expressed as follows: = hii iI UfwU , h (2) where, i means -th connection weight, i U means an output value of the -th input unit, and means a threshold value in the unit , the subscription means the output values in the units of input layer. wi i hθh I A unit in the output layer o U and a unit in the cleanup layer c U are denoted as (3) and (4); =θ oiiii iH iC U fwUwU o c (3) =θ c iO Uf (4) where, and in the equations denote threshold values in the output and the cleanup layers respectively. The states in units both the output and the cleanup layers were updated re- peatedly until the convergence criterion had been reached or until the maximum numbers of iterations (). θoθc τ50 In the learning phase, the mean square error can be defined as follow: 2 1 = 2ii Eut . (5) where, i indicated an i-th teacher signal. Actual learning of connection weights of each unit can be obtained by partial dif- ferential as follows: t = E ww , (6) where, indicates a learning rate fixed as throug- out this study. ηη= 0.01 The initial values of and θ were assigned in accordance with an uniform random value generator . w 0.1 ,θ0.1w Abilities of Attractor Neural Network Attractor networks show rather higher performances than the perceptrons. In general, it is said that three layered perceptron can be regarded as the function approximator in arbitrary preci- sion, when attractor neural network model has plenty of units in the hidden layer. Attractor neural network model, however, show good per- formances even with the limitation of units in hidden and cleanup layers. A good example is the exclusive OR problem. In the natural extension of an exclusive OR problem, there is a parity bit problem. This problem is more difficult than exclu- sive OR problem. And this problem is more general than exclu- sive OR problem. The attractor neural network model can solve 4 bits parity problem. The number of units in input layer is 4. The number of learning patterns to be learnt is 16. The 8 bits parity problem where the number of units in the input layer is 8, and the total number to be learned is 256 with the minimum hidden layer, 1 unit. Figure 4 shows a solution, which can solve 8 bits parity problem with 1 hidden unit and 1 cleanup unit. Furthermore, the attractor network with only one hidden layer unit and only one cleanup layer unit could solve the cate- gory condition in the data of Tyler et al. (2000). The architec- ture of the network was exactly the same as the Figure 4. Application of Attract or Neural Netw orks Hinton and Shallice (1991) and Plaut and Shallice (1993) showed that their attractor network could reproduce symptoms of a kind of dyslexia. According to their simulations, by means of the operation of semantic memory structure, they succeeded to account for the double dissociation between concrete and ab- stract words (Plaut, McClelland, & Seidenberg, 1995; Plaut, 2001). They constructed the semantic memory that the repre- sentations of concrete words have more micro features than those of abstract words. They postulated when the degree of the brain damages would be moderate, concrete words would show lighter deficits than abstract words. Further, if the degree of the brain damage would be severe, the concrete words would have more severe deficits than the abstract words. In this study, the dichotomous taxonomy, such as animate/ inanimate objects classification, was not adopted. Rather, the data on the basis of the discriminability and correlation was employed. Numerical Experiments Computer simulations were conducted under the three condi- tions described below. After learning completed, the effect of Figure 4. A set of connection weights which could solve a 8 bit parity problem. Copyright © 2013 SciRes. 366
 S.-I. ASAKAWA brain damages were intended to mimic by removal of units in hidden and cleanup layers. In each brain damaged simulation, numbers of iteration were postulated to identify prolonged re- action times of patients with semantic memory disorders. Then, the effect of relearning was investigated. Method Conditions Tyler et al. (2000) adopted the isomorphic mappings in order to train their networks. In other words, their networks had to learn the output pattern identical to the input patterns. In this condition, the network must acquire the reproduction of the input pattern. However, it is possible to consider two more conditions (teacher signals in this case). One is that the target matrix (teacher signals) being the identity matrix, having 16 rows and 16 columns, all the diagonal elements being 1 and all the non-diagonal elements being 0. Another is that the matrix having 16 rows times 2 columns, where the elements of this matrix consisting (1, 0) when the item is an animate object, and (0, 1) when the item is an inanimate object. To summarize these three conditions; Category condition: the target matrix is a 16 rows × 2 col- umns matrix, where the targets to be learned are animate ob- jects, the output vectors are (1, 0). Otherwise (inanimate objects) the output vectors are (0, 1). Diag condition: the target matrix is an unitary matrix of 16 rows × 16 columns, where diagonal elements are 1 and other elements in this matrix are 0. Same condition: the target matrix is a 16 rows × 24 columns matrix. This target matrix is the same as the matrix of the input signals. This condition is the one which Tyler et al. (2000) adopted. The category condition can be regarded as the category judgement task in neuropsychological test. Under this condition, the neural network model must learn and discriminate both animate and inanimate concepts. This means that the network is required to learn higher concepts than each item to be learned as Tyler et al. (2000) suggested. In the diag condition, the net- work must learn precise knowledge of each member in the in- put patterns. The unitary matrix in this condition means that each item can play a roll to form the identical matrix. In the same condition, the network is required to learn the precise knowledge of each member in the input patterns. Network Architecture The number of units in the hidden layer was set to be 10, and the number of units in the cleanup layer to be 1. The reason for determining the number of units in the cleanup layer to be 1 is based on the preliminary experiment. Procedure The maximum iteration numbers between the output and the cleanup layers was set to be 10 for each item. If the error of this attractor network did not reach the convergence criteria, de- fined by the sum of squared errors being less than 0.05 for each item. Within the maximum number of iterations between the output and the cleanup layer, the program gave up to let the networks learn this item, and was given the next item to be learned. The order of the items to be learned was randomized within each epoch. This procedure was repeated until the net- work learned all the items. The initial values of the connections are decided by using a random number generator whose range were from −0.15 to +0.15 in accordance with uniform random numbers. The convergence criteria were set that all the sum of squared errors are below 0.05 throughout in this study. The network was given the input signals and teacher signals at a time to learn the output patterns. At first, the output values were calculated from the input patterns to the units in the output layer. Then iterations between the output and the cleanup layers started until the output values have reached the criteria, or the iteration numbers have been exceeded 50 times. Mean Conver g e n ce and Indi v i du a l C onvergence Computer simulations of neural networks, in general, have been considered that the convergence criteria have often been set as the mean square errors (MSE, hereafter) computed from the data set of the whole stimulus. When the MSE of the system outputs would reach the point blow the criteria, it is considered that the system (or the neural network model) could learn the given task. However, in case of both the data set of Tyler et al. (2000) adopted and the three conditions described above, it might be something strange when the mean convergence crite- ria was employed. For example, when we on the supposition that the MSE would be 0.06 when they know “lion”, and that the MSE would be 0.04 when they know “cheater”. In this case, the average MSE would be 0.05, and then the learning must be regarded to complete. However, it seems to be difficult to imag- ine that a man would know lions uncertainly and he would know cheaters certainly simultaneously. Ordinary persons, in general, have knowledge about both lions and cheaters are predatory animals and live in Africa. Here, in view of this rea- son, we decided to adopt the convergence criteria as the indi- vidual convergence. It means that the MSE for each item to be learned must be reached blow the point (0.05 in this study). But the mean convergence criteria were adopted in the category condition. Because the correct output of the first item is (1, 0) and the correct output of the second item is also (1, 0). It cannot be distinguished between these two items. For the same reason, from the fist item to the 8-th item, the correct output patterns are all the same (1, 0), also from the 9-th to 16-th patterns the outputs are (0, 1) as well. Therefore, it would not be able to discriminate the outputs of the neural network systems con- structed for this study could be produced from which output pattern. In case of category judgement tasks for actual human subjects, when the subjects would be asked to answer whether animals or not, they would answer the same way like neural network systems would, whether the object is a lion or a cheater. In this reason, it is adequate that we employed the mean con- vergence criteria for the category condition. On the other hand, the diag and same conditions have different situations. The correct answer for the first item matches only the first output. Therefore, we adopted the individual convergence criteria for these two conditions as it seems to be a natural interpretation like human subjects do. Results Comparison among Conditions We investigated the mean iteration numbers between the output Copyright © 2013 SciRes. 367
 S.-I. ASAKAWA and the cleanup layers. These numbers indicate the times that the initial value is absorbed in an attractor when the initial value was located within a basin of an attractor (Figure 5). This figure shows the mean iteration numbers for each con- dition. The category condition was the least among three condi- tions. This might come from that the system was required to discriminate between only two options in the category condi- tion. There, in this condition, were eight objects of (1, 0) and other eight objects of (0, 1). Other two conditions require that 16 objects must discriminate into 16 options. This simplicity of the output manner in the category condition might cause a kind of easiness of learning. In other words, category judgement task might be easy because of the small number of options. Effect of Damage In order to investigate the effect of damages, we removed the units after the system completed to learn the data set. Removal of units in the hidden layer caused severe disorders. The system failed to answer all the trials in all the conditions. The system had to relearn in order to get the correct answer again. This symptom might resemble that patients often would show severe declines of performance just after brain damage. The result of relearning is shown in the Figure 6. The horizontal axis in Figure 6 is the number of units re- moved. So, this axis can be considered as the severity of dam- Figure 5. The mean iteration numbers for learning completion. It shows the iteration numbers that each MSE reach- ed below 0.05. The whiskers indicate the standard deviations. Figure 6. A simulation of brain damages, the removal of the hidden units after the learning completed. The hori- zontal axis shows the number of units removed. The vertical axis indicates percent correct (n = 100). age. In this figure, the results of diag and same conditions are indicated. The system could easily recover from damages in category condition. Even if the rest of unit become 1, the sys- tem could recover 100% correct. So, we could not draw any curves in the figure. That is to say that the attractor neural net- work model have enough ability to solve this category judge- ment task. The figure also shows that the system was robust against damages in diag and same conditions. The system maintained rather good performance against damages. The performance declined suddenly when the number of units in the hidden layer were 2 or 3. In order confirm these findings above, we conducted another experiment with 5 units in the hidden layer and 2 units in the cleanup layer. The result shows in Figure 7. This figure reveals that the system showed relatively higher performance in cate- gory condition. The other two conditions, diag and same, were indicated that the performance of the system fell down sud- denly when damages became severe. It could be said that the system has an ability for relearning in category judgement task. On the other hand, object identifi- cation task (same condition) and naming task (diag condition) are difficult to recover when damages are severe. Iteration Number between Output and C l ea n u p Layers Iteration number between output and cleanup layers were in- vestigated. Attractor neural network model is a generalized model which includes three layered perceptron in the special case. If the organization of network is enough in order to solve given tasks, we could predict the iteration number between output and cleanup layers would be 0. Then, this iteration might apply to tasks which are required to use attractors. There were many cases of no iteration between output and cleanup layers in all conditions. After damages, the system needs to iterate in order to utilize attractors. Figure 8 shows one of the results. After learning completed, units in hidden layer were re- moved. The horizontal axis shows the number of units removed. Therefore, the number in the horizontal axis can be regarded as severity of brain damage. The vertical axis indicates iteration numbers between output and cleanup layers (n = 100). As it can be seen in the figure, the system had to use interaction between output and cleanup layers. This was the same in all the three conditions. If we could consider these iterations as delays of Figure 7. Simulation of brain damage, removal of units in hidden layer after completion of learning. The hori- zontal axis indicates the number of units removed. The vertical axis indicates percent correct (n = 100). Copyright © 2013 SciRes. 368
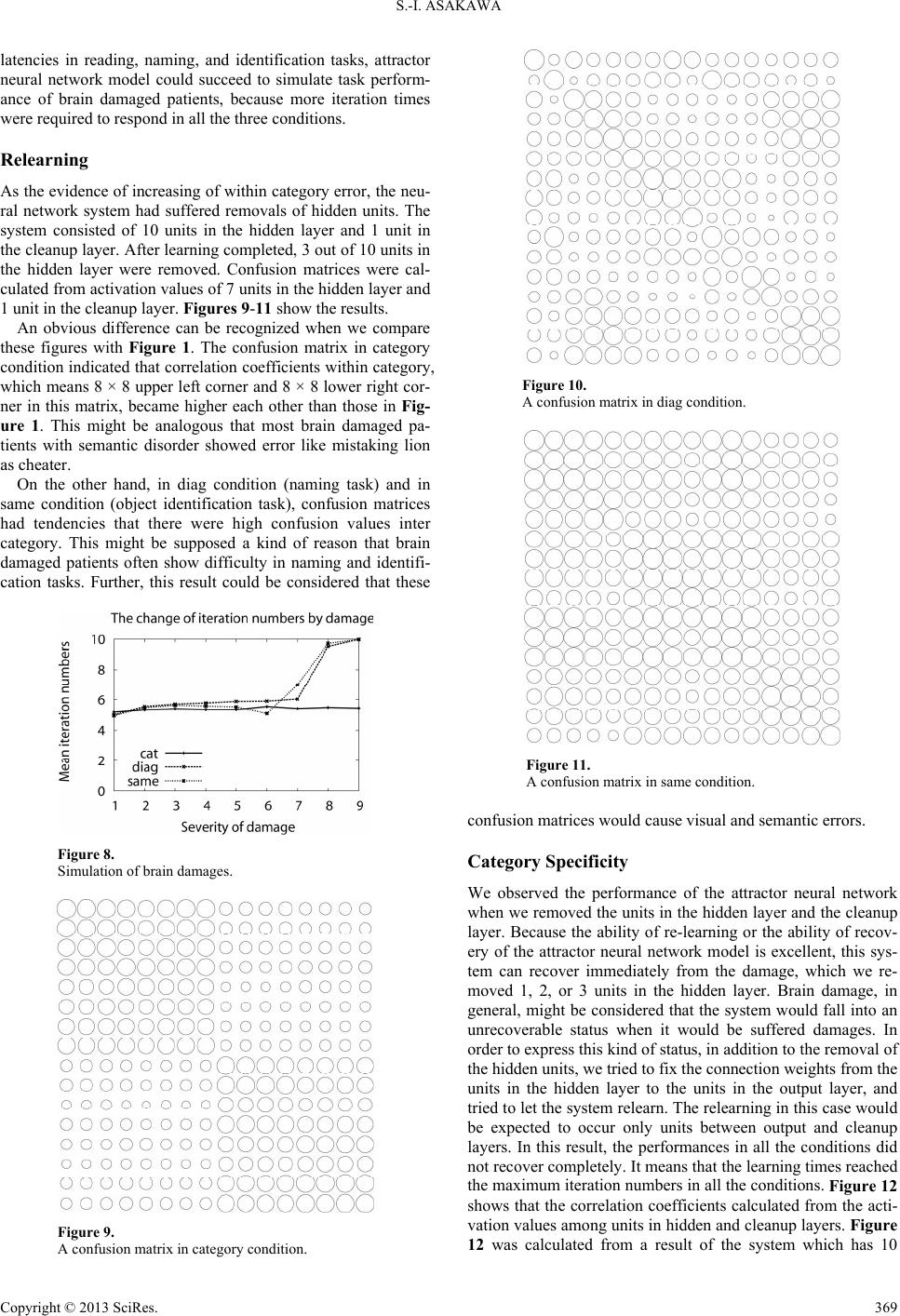 S.-I. ASAKAWA latencies in reading, naming, and identification tasks, attractor neural network model could succeed to simulate task perform- ance of brain damaged patients, because more iteration times were required to respond in all the three conditions. Relearning As the evidence of increasing of within category error, the neu- ral network system had suffered removals of hidden units. The system consisted of 10 units in the hidden layer and 1 unit in the cleanup layer. After learning completed, 3 out of 10 units in the hidden layer were removed. Confusion matrices were cal- culated from activation values of 7 units in the hidden layer and 1 unit in the cleanup layer. Figures 9-11 show the results. An obvious difference can be recognized when we compare these figures with Figure 1. The confusion matrix in category condition indicated that correlation coefficients within category, which means 8 × 8 upper left corner and 8 × 8 lower right cor- ner in this matrix, became higher each other than those in Fig- ure 1. This might be analogous that most brain damaged pa- tients with semantic disorder showed error like mistaking lion as cheater. On the other hand, in diag condition (naming task) and in same condition (object identification task), confusion matrices had tendencies that there were high confusion values inter category. This might be supposed a kind of reason that brain damaged patients often show difficulty in naming and identifi- cation tasks. Further, this result could be considered that these Figure 8. Simulation of brain damages. Figure 9. A confusion matrix in category condition. Figure 10. A confusion matrix in diag condition. Figure 11. A confusion matrix in same condition. confusion matrices would cause visual and semantic errors. Categor y Sp ecificity We observed the performance of the attractor neural network when we removed the units in the hidden layer and the cleanup layer. Because the ability of re-learning or the ability of recov- ery of the attractor neural network model is excellent, this sys- tem can recover immediately from the damage, which we re- moved 1, 2, or 3 units in the hidden layer. Brain damage, in general, might be considered that the system would fall into an unrecoverable status when it would be suffered damages. In order to express this kind of status, in addition to the removal of the hidden units, we tried to fix the connection weights from the units in the hidden layer to the units in the output layer, and tried to let the system relearn. The relearning in this case would be expected to occur only units between output and cleanup layers. In this result, the performances in all the conditions did not recover completely. It means that the learning times reached the maximum iteration numbers in all the conditions. Figure 12 shows that the correlation coefficients calculated from the acti- vation values among units in hidden and cleanup layers. Figure 12 was calculated from a result of the system which has 10 Copyright © 2013 SciRes. 369
 S.-I. ASAKAWA Figure 12. A visualization of a matrix of correlation coefficients among objects to be learned, calculated from the hidden and cleanup layers after relearning. units in hidden layer and 1 unit in cleanup layer. After learning completed, 3 units in the hidden layer were removed. Compare Figure 1 with Figure 12. Comparison between figures indi- cates that the correlation coefficients are relatively higher in Figure 12 than in Figure 1. It is possible to interpret that this result might cause confusions among objects. For example, brain damaged patients with animate specific disorder may confuse lion as cheater. The system may confuse objects in the data set as well. Removal of Units in Cleanup Layer We set the number of units in the cleanup layer as two and train the system, then we removed one of the units in the cleanup layer. We varied the initial values and performed simulations. The results are shown blow. Each line indicates each result. There are 16 items to be learned. The first 8 columns indicated by the digits from 0 to 7 mean inanimate objects, and the last 8 columns indicated by the digits from 8 to 15 means animate objects. Parentheses “()” indicate that the system failed to reach the correct answer within the limited iterations between output and cleanup layers. Each digit shows the number of items which the system produced (Table 1). This results might mean that brain damages would transform the basins. Therefore, it could be pointed out that a kind of confusion among other items occurred. Compared with animal objects, the system did not make any mistakes about inanimate objects. It is supposed that the correlation coefficients between inanimate objects were relatively smaller than those of animates. Table 2 shows the iteration numbers when the system suffered damage: removal of units. The iteration numbers between output and cleanup layers were increased in animate objects. If we could identify these iteration numbers as reaction times which brain damaged pa- tients show, the attractor neural network can be regarded as the model of semantic memory disorder to explain category speci- ficity. As an analysis of the types of error, objects are close each other in the data set of Tyler et al. (2000). So, if the system would suffer injuries or damages, it would give rise to mistakes the most likely objects. In fact, when we conducted a multidi- mensional scaling analysis to the data of Tyler2000, its result showed as Table 3. The coordinate values were calculated until Table 1. Example of the outputs when one of the units in the cleanup layer was removed. inanimate animate 0 1 2 3 4 5 6 7 (5) 9 (5) 11 (5) (5) (5) (5) 0 1 2 3 4 5 6 7 (7) (7) (7) (7) 12 (7) (7) 15 0 1 2 3 4 5 6 7 (6) (6) (6) (6) (6) (6) (6) (6) 0 1 2 3 4 5 6 7 (1) (1) (1) (1) (1) (1) 14 (1) 0 1 2 3 4 5 6 7 8 9 10 11 12 13 14 15 0 1 2 3 4 5 6 7 (1) (1) 10 (1) (1) 13 (1) (1) 0 1 2 3 4 5 6 7 8 (1) 10 11 (1) (1) (1) (1) 0 1 2 3 4 5 6 7 (4) (4) (4) 11 12 (4) 14 15 0 1 2 3 4 5 6 7 (7) 9 10 11 (7) (7) (7) (7) Table 2. Example of the iteration numbers (max = 20) when one of the units in the cleanup layer was removed. inanimate animate 0 0 0 0 0 0 0 0 2 2 2 2 1 2 2 2 2 0 0 0 0 0 0 0 (20) 2 (20) 2 (20) (20) (20) (20) 0 0 0 0 0 0 0 0 (20) (20) (20) (20) 2 (20) (20) 2 0 0 0 0 0 0 0 0 (20) (20) (20) (20) (20) (20) (20) (20) 0 0 0 0 0 0 0 0 (20) (20) (20) (20) (20) (20) 3 (20) 0 0 0 0 0 0 0 0 2 2 2 2 3 2 2 2 0 0 0 0 0 0 0 0 (20) (20) 2 (20) (20) 2 (20) (20) 0 0 0 0 0 0 0 0 2 (20) 2 3 (20) (20) (20) (20) 0 0 0 0 0 0 0 0 (20) (20) (20) 2 2 (20) 2 3 0 0 0 0 0 0 0 0 (20) 2 2 2 (20) (20) (20) (20) Table 3. Two dimensional values of the result of MDS for each object. objects Dimension 1 Dimension 2 1 −0.000000 −0.968246 2 −0.000000 −0.968246 3 −0.000000 −0.968246 4 −0.000000 −0.968246 5 −0.000000 −0.968246 6 −0.000000 −0.968246 7 −0.000000 −0.968246 8 −0.000000 −0.968246 9 −1.414214 0.968246 10 −1.414214 0.968246 11 −1.414214 0.968246 12 −1.414214 0.968246 13 1.414214 0.968246 14 1.414214 0.968246 15 1.414214 0.968246 16 1.414214 0.968246 Copyright © 2013 SciRes. 370
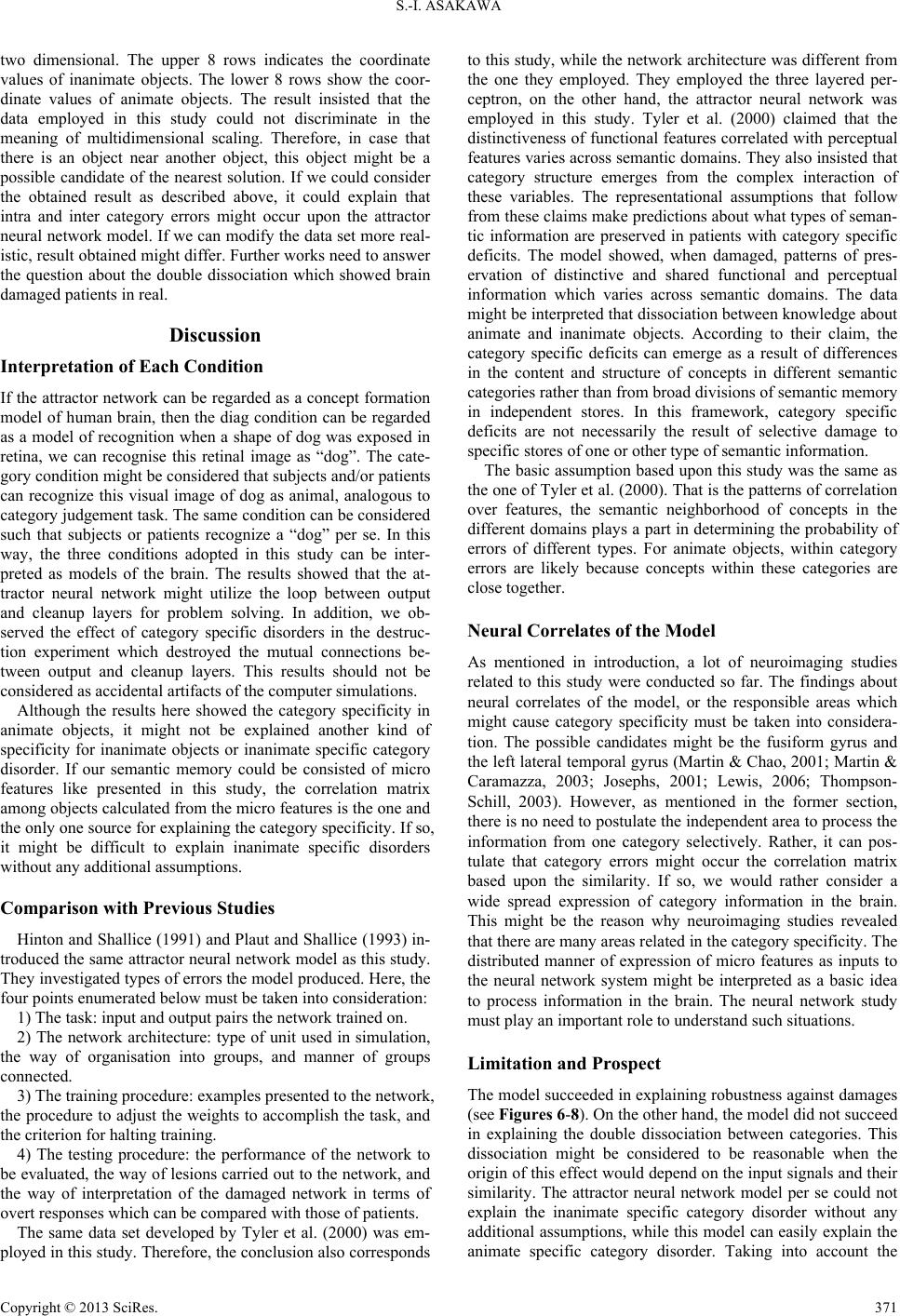 S.-I. ASAKAWA two dimensional. The upper 8 rows indicates the coordinate values of inanimate objects. The lower 8 rows show the coor- dinate values of animate objects. The result insisted that the data employed in this study could not discriminate in the meaning of multidimensional scaling. Therefore, in case that there is an object near another object, this object might be a possible candidate of the nearest solution. If we could consider the obtained result as described above, it could explain that intra and inter category errors might occur upon the attractor neural network model. If we can modify the data set more real- istic, result obtained might differ. Further works need to answer the question about the double dissociation which showed brain damaged patients in real. Discussion Interpretation of Each Condition If the attractor network can be regarded as a concept formation model of human brain, then the diag condition can be regarded as a model of recognition when a shape of dog was exposed in retina, we can recognise this retinal image as “dog”. The cate- gory condition might be considered that subjects and/or patients can recognize this visual image of dog as animal, analogous to category judgement task. The same condition can be considered such that subjects or patients recognize a “dog” per se. In this way, the three conditions adopted in this study can be inter- preted as models of the brain. The results showed that the at- tractor neural network might utilize the loop between output and cleanup layers for problem solving. In addition, we ob- served the effect of category specific disorders in the destruc- tion experiment which destroyed the mutual connections be- tween output and cleanup layers. This results should not be considered as accidental artifacts of the computer simulations. Although the results here showed the category specificity in animate objects, it might not be explained another kind of specificity for inanimate objects or inanimate specific category disorder. If our semantic memory could be consisted of micro features like presented in this study, the correlation matrix among objects calculated from the micro features is the one and the only one source for explaining the category specificity. If so, it might be difficult to explain inanimate specific disorders without any additional assumptions. Comparis o n with Previ ous Studies Hinton and Shallice (1991) and Plaut and Shallice (1993) in- troduced the same attractor neural network model as this study. They investigated types of errors the model produced. Here, the four points enumerated below must be taken into consideration: 1) The task: input and output pairs the network trained on. 2) The network architecture: type of unit used in simulation, the way of organisation into groups, and manner of groups connected. 3) The training procedure: examples presented to the network, the procedure to adjust the weights to accomplish the task, and the criterion for halting training. 4) The testing procedure: the performance of the network to be evaluated, the way of lesions carried out to the network, and the way of interpretation of the damaged network in terms of overt responses which can be compared with those of patients. The same data set developed by Tyler et al. (2000) was em- ployed in this study. Therefore, the conclusion also corresponds to this study, while the network architecture was different from the one they employed. They employed the three layered per- ceptron, on the other hand, the attractor neural network was employed in this study. Tyler et al. (2000) claimed that the distinctiveness of functional features correlated with perceptual features varies across semantic domains. They also insisted that category structure emerges from the complex interaction of these variables. The representational assumptions that follow from these claims make predictions about what types of seman- tic information are preserved in patients with category specific deficits. The model showed, when damaged, patterns of pres- ervation of distinctive and shared functional and perceptual information which varies across semantic domains. The data might be interpreted that dissociation between knowledge about animate and inanimate objects. According to their claim, the category specific deficits can emerge as a result of differences in the content and structure of concepts in different semantic categories rather than from broad divisions of semantic memory in independent stores. In this framework, category specific deficits are not necessarily the result of selective damage to specific stores of one or other type of semantic information. The basic assumption based upon this study was the same as the one of Tyler et al. (2000). That is the patterns of correlation over features, the semantic neighborhood of concepts in the different domains plays a part in determining the probability of errors of different types. For animate objects, within category errors are likely because concepts within these categories are close together. Neural Correlates of the Model As mentioned in introduction, a lot of neuroimaging studies related to this study were conducted so far. The findings about neural correlates of the model, or the responsible areas which might cause category specificity must be taken into considera- tion. The possible candidates might be the fusiform gyrus and the left lateral temporal gyrus (Martin & Chao, 2001; Martin & Caramazza, 2003; Josephs, 2001; Lewis, 2006; Thompson- Schill, 2003). However, as mentioned in the former section, there is no need to postulate the independent area to process the information from one category selectively. Rather, it can pos- tulate that category errors might occur the correlation matrix based upon the similarity. If so, we would rather consider a wide spread expression of category information in the brain. This might be the reason why neuroimaging studies revealed that there are many areas related in the category specificity. The distributed manner of expression of micro features as inputs to the neural network system might be interpreted as a basic idea to process information in the brain. The neural network study must play an important role to understand such situations. Limitation and Prospect The model succeeded in explaining robustness against damages (see Figures 6-8). On the other hand, the model did not succeed in explaining the double dissociation between categories. This dissociation might be considered to be reasonable when the origin of this effect would depend on the input signals and their similarity. The attractor neural network model per se could not explain the inanimate specific category disorder without any additional assumptions, while this model can easily explain the animate specific category disorder. Taking into account the Copyright © 2013 SciRes. 371
 S.-I. ASAKAWA results obtained in neuroimaging studies and clinical neuro- psychology, the computational approach using neural network model must be worth considering. Hereafter, it is tried to de- scribe the relationship to the areas of cognitive neuropsychol- ogy and neural network. Contributio n to Cog ni tive Neuropsych ology The attractor neural network model employed in this study was originally developed with an intention to explain neuropsy- chological evidence (Hinton & Shallice, 1991; Plaut & Shallice, 1993). Therefore, the model can apply directly to the data in neuropsychology. The model could explain three different tasks: categorisation, naming, and identification tasks (see the condi- tions section in numerical experiments). This is one of the promising ways to bring our knowledge to further understand- ings. The more phenomenon which the model can explain, the better in the sense of parsimony. Contributio n t o Neural Netw ork The model employed in this study was one of applications of the generalised neural network model. The method of learning was also the general one known as the generalised delta rule (e.g. the back propagation method). The relation between the generalised model and its application to the particular area or evidence would make fruitful discussion to understand the concerning phenomenon. Bridge between Neuroimaging and Neuropsychological Studies Synthesis between neuroimaging and neuropsychological stud- ies must be required. While neuroimaging studies reveal that there are many related areas in the brain for category specificity, neuropsychological studies have tendency to emphasize the asymmetry or the double dissociation between animate and inanimate objects. Both findings must be explained simultane- ously based upon one integrated model. The value of the model employed in this study can exist in this point of view. This study was conducted to try to explain along with this point of view. Finally, what the author is thinking is enumerated as follow: 1) The disorder in semantic memory might reflect the struc- ture of the semantic memory. 2) This disorder might emerge neuropsychological level, which means that it occurs as the size of gyri and sulci. It is neither individual neuron nor whole system levels. 3) Attractor neural network can be considered as a model for semantic memory disorder. It might be a useful tool to investi- gate category specificity. 4) Synthesis between heterogeneous (category specific) and homogeneous (no neuroanatomical specialisation) point of view is possibly a promising way to describe phenomenon. Conclusion In spite of the simplicity, the attractor neural network could describe at least three cognitive neuropsychological tasks; categorisation, identification, and naming tasks. This is one of major advantages of this model. The model could succeed in predicting patients’ behaviour with animate specific memory disorder, however, the model could not explain inanimate spe- cific memory disorder without any additional assumptions. So, the possibility for this model to explain the double dissociation between animate and inanimate objects should be discussed further in separate papers. However, there still are possibilities for this model to account for the double dissociation between animate and inanimate objects. In this study, non-dichotomous memory representation like Figures 1 and 9 was adopted as the data set to be learned. The model’s behaviour depends on both its network architecture and its input data representation, which is defined by micro features. This micro feature constrains the model’s behaviour through the correlation matrix among ob- jects. The difference between intra- and inter-correlations shown in Figure 1 might cause the category specificity, because one category has higher inner-category correlations than that of the other category. The representations could be considered such that there needs no local representations to deal with both ani- mate and inanimate objects in our brains. On the contrary, category specificity might emerge necessarily and naturally as consequences of exposure of both categories. In addition to this consideration, these object representations adopted in this study might also produce category specific memory disorders when the system suffered damages. Therefore, the attractor neural network could be considered as the one of possible candidates to explain various cognitive neuropsychological phenomena. This model also provides useful suggestions about our semantic memory organisation. However, the model failed in explaining patients’ behaviour with inanimate specific memory disorder, while this model succeeded in explaining patients behaviour with animate specific disorder. It is obvious that the model has both advantage and shortcoming. The fact that three kinds of tasks could be explained by this model is clearly one of mani- fest advantages of this model. Further studies must be con- ducted to reveal the shortcoming. It is also obvious that the model might not be able to explain this shortcoming without any additional assumptions or modification of network archi- tecture. However, it can be considered that this study would be valuable because the model succeeded in showing clear insight about a direction of studies in the future. REFERENCES Bullinaria, J. A. (1999). Connectionisit dissociations, confounding fac- tors and modularity. Proceedings of the Fifth Neural Computation and Psychology Workshop, 52-63. Capitani, E., Laiaconna, M., Mahon, B., & Caramazza, A. (2003). What are the facts of semantic category-specific deficits? A critical review of the clinical evidence. Cognitive Neuropsychology , 20, 213-261. doi:10.1080/02643290244000266 Caramazza, A., Hillis, A., Rapp, B. C., & Romani, C. (1990). The mul- tiple semantics hypothesis: Multiple confusions? Cognitive Neuro- psycholgy, 7, 161-189. doi:10.1080/02643299008253441 Caramazza, A., & Shelton, J. (1998). Domain specific knowledge sys- tem in the brain: The animate-inanimate distinction. Journal of Cog- nitive Neuroscience, 10, 1-34. doi:10.1162/089892998563752 De Renzi, E., & Lucchelli, F. (1994). Are semantic systems separately represented in the brain? The case of living category impairment. Cortex, 30, 3-25. Devlin, J., Gonnerman, L., Andersen, E., & Seidenberg, M. (1998). Category specific semantic deficits in focal and widespred brain da- mage: A computational account. Journal of Cognitive Neuroscience, 10, 77-94. doi:10.1162/089892998563798 Farah, M. J., & McClelland, J. L. (1991). A computational model of se- mantic memory impairment: Modality specificity and emergent cate- gory specificity. Journal of Experimental Psychology: General, 120, 339-357. doi:10.1037/0096-3445.120.4.339 Copyright © 2013 SciRes. 372
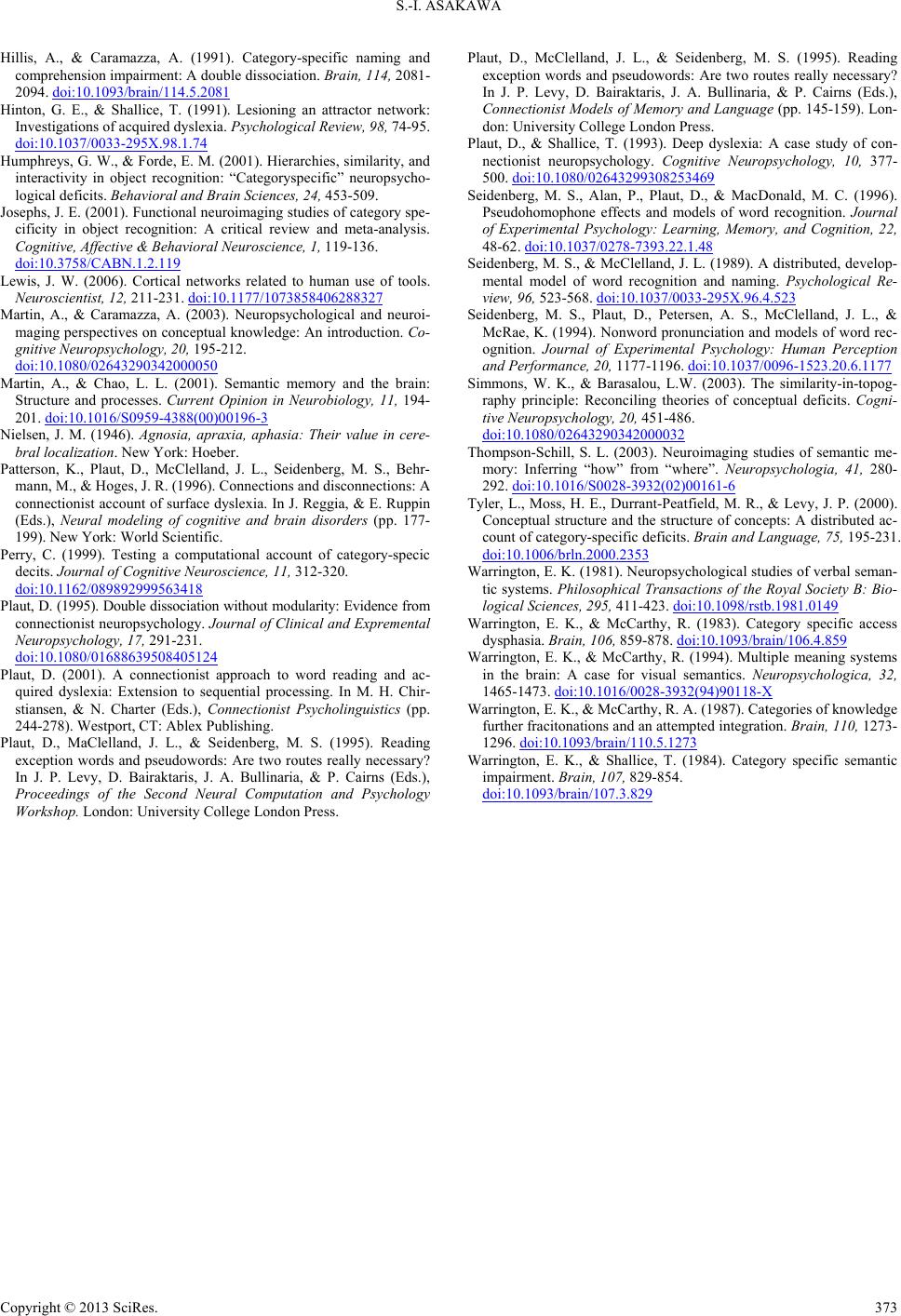 S.-I. ASAKAWA Copyright © 2013 SciRes. 373 Hillis, A., & Caramazza, A. (1991). Category-specific naming and comprehension impairment: A double dissociation. Brain, 114, 2081- 2094. doi:10.1093/brain/114.5.2081 Hinton, G. E., & Shallice, T. (1991). Lesioning an attractor network: Investigations of acquired dyslexia. Psychological Review, 98, 74-95. doi:10.1037/0033-295X.98.1.74 Humphreys, G. W., & Forde, E. M. (2001). Hierarchies, similarity, and interactivity in object recognition: “Categoryspecific” neuropsycho- logical deficits. Behavioral and Brain Sciences, 2 4, 453-509. Josephs, J. E. (2001). Functional neuroimaging studies of category spe- cificity in object recognition: A critical review and meta-analysis. Cognitive, Affective & Behavioral Neuroscience, 1, 119-136. doi:10.3758/CABN.1.2.119 Lewis, J. W. (2006). Cortical networks related to human use of tools. Neuroscientist, 12, 211-231. doi:10.1177/1073858406288327 Martin, A., & Caramazza, A. (2003). Neuropsychological and neuroi- maging perspectives on conceptual knowledge: An introduction. Co- gnitive Neuropsychology, 20 , 195-212. doi:10.1080/02643290342000050 Martin, A., & Chao, L. L. (2001). Semantic memory and the brain: Structure and processes. Current Opinion in Neurobiology, 11, 194- 201. doi:10.1016/S0959-4388(00)00196-3 Nielsen, J. M. (1946). Agnosia, apraxia, aphasia: Their value in cere- bral localization. New York: Hoeber. Patterson, K., Plaut, D., McClelland, J. L., Seidenberg, M. S., Behr- mann, M., & Hoges, J. R. (1996). Connections and disconnections: A connectionist account of surface dyslexia. In J. Reggia, & E. Ruppin (Eds.), Neural modeling of cognitive and brain disorders (pp. 177- 199). New York: World Scientific. Perry, C. (1999). Testing a computational account of category-specic decits. Journal of Cogn it i v e N e ur o science, 11, 312-320. doi:10.1162/089892999563418 Plaut, D. (1995). Double dissociation without modularity: Evidence from connectionist neuropsychology. Journal of Clinical and Expremental Neuropsychology, 17, 291-231. doi:10.1080/01688639508405124 Plaut, D. (2001). A connectionist approach to word reading and ac- quired dyslexia: Extension to sequential processing. In M. H. Chir- stiansen, & N. Charter (Eds.), Connectionist Psycholinguistics (pp. 244-278). Westport, CT: Ablex Publishing. Plaut, D., MaClelland, J. L., & Seidenberg, M. S. (1995). Reading exception words and pseudowords: Are two routes really necessary? In J. P. Levy, D. Bairaktaris, J. A. Bullinaria, & P. Cairns (Eds.), Proceedings of the Second Neural Computation and Psychology Workshop. London: University College London Press. Plaut, D., McClelland, J. L., & Seidenberg, M. S. (1995). Reading exception words and pseudowords: Are two routes really necessary? In J. P. Levy, D. Bairaktaris, J. A. Bullinaria, & P. Cairns (Eds.), Connectionist Models of Memory and Language (pp. 145-159). Lon- don: University College London Press. Plaut, D., & Shallice, T. (1993). Deep dyslexia: A case study of con- nectionist neuropsychology. Cognitive Neuropsychology, 10, 377- 500. doi:10.1080/02643299308253469 Seidenberg, M. S., Alan, P., Plaut, D., & MacDonald, M. C. (1996). Pseudohomophone effects and models of word recognition. Journal of Experimental Psychology: Learning, Memory, and Cognition, 22, 48-62. doi:10.1037/0278-7393.22.1.48 Seidenberg, M. S., & McClelland, J. L. (1989). A distributed, develop- mental model of word recognition and naming. Psychological Re- view, 96, 523-568. doi:10.1037/0033-295X.96.4.523 Seidenberg, M. S., Plaut, D., Petersen, A. S., McClelland, J. L., & McRae, K. (1994). Nonword pronunciation and models of word rec- ognition. Journal of Experimental Psychology: Human Perception and Performance, 20, 1177-1196. doi:10.1037/0096-1523.20.6.1177 Simmons, W. K., & Barasalou, L.W. (2003). The similarity-in-topog- raphy principle: Reconciling theories of conceptual deficits. Cogni- tive Neuropsychology, 20, 451-486. doi:10.1080/02643290342000032 Thompson-Schill, S. L. (2003). Neuroimaging studies of semantic me- mory: Inferring “how” from “where”. Neuropsychologia, 41, 280- 292. doi:10.1016/S0028-3932(02)00161-6 Tyler, L., Moss, H. E., Durrant-Peatfield, M. R., & Levy, J. P. (2000). Conceptual structure and the structure of concepts: A distributed ac- count of category-specific deficits. Brain and Language, 75, 195-231. doi:10.1006/brln.2000.2353 Warrington, E. K. (1981). Neuropsychological studies of verbal seman- tic systems. Philosophical Transactions of the Royal Society B: Bio- logical Sciences, 295, 411-423. doi:10.1098/rstb.1981.0149 Warrington, E. K., & McCarthy, R. (1983). Category specific access dysphasia. Brain, 106, 859-878. doi:10.1093/brain/106.4.859 Warrington, E. K., & McCarthy, R. (1994). Multiple meaning systems in the brain: A case for visual semantics. Neuropsychologica, 32, 1465-1473. doi:10.1016/0028-3932(94)90118-X Warrington, E. K., & McCarthy, R. A. (1987). Categories of knowledge further fracitonations and an attempted integration. Brain, 110, 1273- 1296. doi:10.1093/brain/110.5.1273 Warrington, E. K., & Shallice, T. (1984). Category specific semantic impairment. Brain, 107, 829-854. doi:10.1093/brain/107.3.829
|