 Journal of Behavioral and Brain Science, 2013, 3, 49-56 http://dx.doi.org/10.4236/jbbs.2013.31005 Published Online February 2013 (http://www.scirp.org/journal/jbbs) What Are the Chances? fMRI Correlates of Observing High and Low-Probability Actions Roger Newman-Norlund1, Kim Bruggink2, Raymond Cuijpers3, Harold Bekkering2 1Department of Exercise Science, University of South Carolina, Columbia, USA 2Donders Institute for Brain, Cognition and Behavior, Radboud University Nijmegen, Nijmegen, The Netherlands 3Industrial Engineering & Innovation Sciences, Human Technology Interaction Group, Eindhoven University of Technology, Eindhoven, The Netherlands Email: rnorlund@mailbox.sc.edu Received October 5, 2012; revised November 7, 2012; accepted November 15, 2012 ABSTRACT Cognitive scientists often use probabilistic equations to model human behavior in ambiguous situations. How, where, and even if such probabilities are represented in the human brain remains largely unknown. Here, we manipulated the probability of simple bottle-pouring action based on two considerations, the relative fullness of two glasses and the rela- tive distance between the two glasses and the bottle. Whole brain functional magnetic resonance imaging was used to measure brain activity while participants viewed probable and improbable pouring actions. Improbable actions elicited increased activity in the theory of mind (ToM) network, commonly found active when trying to grasp the intentions of others, whereas probable actions elicited increased activity in the human mirror neuron system (hMNS) and areas asso- ciated with mental imagery and memory. These data provide novel insight into the brain mechanisms humans use to distinguish between high and low-probability actions. Keywords: Probability; fMRI; Human Mirror Neuron System; Action Observation 1. Introduction The ability to make predictions about the future state of the world when placed in ambiguous situations is a fun- damental and very useful capacity present in humans. Athletes predict the actions of their opponents, mothers make predictions regarding their baby’s needs, and wri- ters predict the responses of their reviewers. Cognitive scientists have long relied on probabilistic models to ex- plain a wide variety of complex human behaviors in- cluding decision making, action planning, motor learning and behavior [1-5]. Probabilistic models are especially apt at making predictions in ambiguous situations like those mentioned above. In such models, events are typi- cally assigned a specific likelihood after taking into ac- count the current situation and prior experience accord- ing to a well known statistical approach known as em- pirical Bayes. While significant research has been con- ducted with regards to the neural correlates of probabili- ties as they relate to topics such as reinforcement learn- ing, risk taking behavior and reward [6-9], relatively lit- tle is known about the neural representation of action probabilities as they relate to object-directed actions en- countered in everyday situations. In the current experi- ment, we attempted to localize brain areas responsible for coding the probability of actions. Specifically, we con- ducted a functional magnetic resonance imaging (fMRI) experiment in which we could visualize brain activity during the observation of actions that were either likely or unlikely based on the relative distance between objects being combined or the context in which the combination of objects occurred. Based on previous research, we had strong reason to believe that human mirror neuron system (MNS) would be involved in the calculation of action probabilities. The MNS, a system comprised of the human inferior parietal and frontal lobes, is often cited as supporting various as- pects of action understanding. Activity within this system is modulated by the type of action sequences that are “likely” to follow a particular observed movement [10- 13]. For example, Fogassi and colleagues [14] found that activity recorded from primate MNs during the grasp of a peanut depends critically on whether the peanut was then eaten or placed in a cup. In humans, Iacoboni and col- leagues [15] showed that activity recorded from the human MNS during the observation of a grasping action also varies as a function of the subsequent to-be per- formed movements. This sensitivity to future actions, highly relevant to the discussion of action probabilities, has been referred to as “action forecasting”. Here, the idea is that MNs respond most strongly to actions which C opyright © 2013 SciRes. JBBS
 R. NEWMAN-NORLUND ET AL. 50 are likely to be followed by other actions, i.e. actions that are the initial element of a likely-to-follow action se- quence. The idea that mirror neuron activity may reflect the probability of a given action is echoed by researchers working in the field of biological robotics. These re- searchers have gone so far as to suggest specific biological mechanisms whereby action probabilities could be corti- cally represented. Specifically, Metta and colleagues [16] have hypothesized that the probability of a given action A occurring, given the presence of a given object O, or P A|O, might be encoded by the response of canonical neu- rons located within core MNS areas. These neurons res- pond to the observation of objects that can be grasped, and it has been suggested that their activity reflects 1) the processing of the affordances of the object and 2) a “mental simulation” of actual object use and/or 3) sub- sequent actions that might possibly be executed [17]. In order to examine the anatomical basis of probability coding, we created a paradigm in which the probability of a simple pouring action (i.e. pouring a bottle of wine into one of two glasses) was dependent on two factors; the relative fullness of the two glasses which could po- tentially be poured into and the relative distance between the bottle and each of the two glasses. We arrived at these factors based on the computational model of Cuij- pers and colleagues [2] which predicts that 1) the com- bination of nearby objects is more likely than the combi- nation of distant objects, and that 2) logical combinations of objects (e.g. bolt + nut) are more probable than illo- gical combinations (e.g. bolt + screw). Accordingly, we hypothesized that the emptier a glass was, the more likely it would be for an actor to pour fluid into that glass. Similarly, we reasoned that the closer the bottle was to one of the glasses, the more likely it would be to pour into that glass. We expected the MNS to exhibit differen- tial response patterns based on the probability of pouring actions which we experimentally manipulated by varying these distance and fullness cues. Specifically, we pre- dicted the existence of sites within the MNS that would respond maximally to high-probability actions indepen- dent of whether the action’s probability was based dis- tance or fullness cues. 2. Methods 2.1. Participants Twenty-one right-handed subjects (5 males, 16 females) between the ages of 19 and 35 (mean ± SD age, 23.6 ± 4.1 years) participated in the experiment. All participants had normal or corrected-to-normal vision and were heal- thy adults (self-report). They gave written informed con- sent according to the institutional guidelines set forth by the local ethics committee (CMO region Arnhem-Nijme- gen, Netherlands) prior to the experiment. Subjects were compensated at the rate of 12.50 €/hr for their partici- pation. 2.2. Stimuli Stimuli for the scanning session consisted of photos pre- sented centrally on the screen on a plain black back- ground. Photos were made using a digital camera and resized to a 600 × 400 pixels image. Photos displayed a table with two glasses positioned on the left and right side of the table, a bottle and a person sitting behind the table (without showing the head). Photos were taken in such a way that the displayed person appeared to sit across the table facing the subject. Four types of stimuli can be distinguished. The first type of stimuli displayed the bottle on the table in between two wineglasses while the person is holding it with her/his right hand. Bottle po- sition varied from left to right in five positions (closest to left glass, left from the middle, middle, right from the middle, closest to right glass) and two different varieties of wine bottle were used in an attempt to maintain sub- jects’ attention. The second type of stimuli showed the person pouring from a bottle into either one of the glasses. Photos of glasses containing varying amounts of liquid (almost empty glasses, half full glasses and full glasses) were later overlayed on top of the glasses in the photos with the total setup. All 90 combinations were included (5 bottle positions × 2 possibilities for pouring × 3 fullness degrees for left glass × 3 fullness degrees for right glass = 90 possibilities). 2.3. Testing Procedure Functional magnetic resonance imaging was performed while participants watched short, two-frame action se- quences in which an actor gripped a wine bottle and poured it into one of two wine glasses. A single functio- nal run consisted of 99 trials, nine of which were a re- petition of the preceding trial. The other 90 trials were all different. The two different bottles were randomly dis- tributed over the 90 possibilities mentioned above, and these combinations were presented in random order. Each trial consisted of a sequence of two photos interleaved with a fixation cross after every second photo. The first photo portrayed the initial set-up of the bottle and glasses and was presented for 2000 ms. This was followed by a 1000 ms presentation of a photo showing the actor pour- ing wine into one of the glasses. Then a jitter stimulus containing a black screen with a white fixation cross in the middle was presented for 4000 - 8000 ms. Figure 1 illustrates the temporal progression of the task. Stimuli were presented using a projector with a resolution of 1280 × 1024 pixels, and viewed by participants lying in the fMRI scanner through a custombuilt mirror. All sti- Copyright © 2013 SciRes. JBBS
 R. NEWMAN-NORLUND ET AL. 51 (a) (b) Figure 1. Examples of experimental stimuli. Plates showing example stimuli used in the experiment. (a) From left to right, sequence of pictures in which the probability of the action is equal based on the distance between the bottle and glasses. Based on the relative fullness of the glasses, it is more likely that the actor will pour into the right glass. (b) In this series of photos, it is more likely that the actor will pour into the right glass based on the distance between the bottle and glasses. Based on the relative fullness of the glasses, it is more probable that the actor will pour in the left glass. muli were delivered using Presentation software version 9.90 (Neurobehavioral Systems, Davis, CA) run on a Dell Workstation (Austin, TX, USA). Subjects were in- structed to concentrate on the photos while in the scanner and to respond with a button press when they observed the same trial two times in a row. This was done to en- sure that participants were paying attention to the stimuli. 2.4. fMRI Data Acquisition All magnetic resonance imaging was conducted at the F.C. Donders Centre for Cognitive Neuroimaging (Nij- megen, The Netherlands). Functional images were ac- quired on a Trio 3T whole-body MR scanner (Siemens) using an ascending slice acquisition sequence and a bird- cage head coil (TR = 2.50 s, TE = 35 ms, 90˚ flip-angle, 34 axial slices, slice-matrix size = 64 × 64, slice thick- ness = 3 mm, slice gap = 0.5 mm, FOV = 22.4 cm, voxel size = 3.5 × 3.5 × 3.5 mm). Head movement was re- stricted using foam cushions. A single scanning block lasted approximately 17 minutes. Following acquisition of echo-coplanar images (EPIs), a T1-weighted 3D MP- RAGE sequence (volume TR = 1960 ms, TE = 4.43 ms, 8˚ flip-angle, 176 coronal slices, slice-matrix size = 256 × 208, slice thickness = 1.0 mm, voxel size = 1 × 1 × 1 mm) was acquired. 2.5. fMRI Data Analysis Functional data were preprocessed and analyzed using SPM2 (http://www.fil.ion.ucllac.uk/spm). All functional data were first corrected for motion artifacts using the bilinear interpolation method and coregistered with the high resolution T2-weighted anatomical image. Images were then normalized to the Montreal Neurological In- stitute (MNI) template with a resolution of 2 × 2 × 2 mm, and smoothed in three dimensions using a 6 × 6 × 6 mm Gaussian kernel. BOLD signal recorded during the ob- servation of the short action sequences was modeled as the primary epoch of interest. Null events in which the fixation cross remained on the screen (as opposed to the appearance of an action sequence) were modeled as the REST condition along with the first and last fifty seconds of the functional run during which time the fixation cross was also on the screen. 3. Results 3.1. One-Back Task In order to ensure that participants were paying attention to the stimuli, a small percentage of the trials (nine trials per functional fun) were replications of the immediately preceding trial. Participants pressed a response button as soon as they perceived one of these repeated trials. On average participants made 8.95 responses (SD = 1.31, Min = 5, Max = 12, Mode = 9) during the experimental run thus confirming that they were paying attention to the stimuli. 3.2. Brain Areas Responding to Low Probability Actions In order to isolate core brain areas responding more strongly to improbable as compared to probable actions we computed the intersection analysis (LPd − HPd) ∩ (LPf − HPf) (see Methods). This analysis revealed that BOLD signal was significantly greater during the obser- vation of low as compared to high-probability actions at sites in the left medial frontal cortex (MFC, BA 32) and the right middle superior temporal sulcus (mSTS, BA 48), (Table 1, Figure 2). 3.3. Brain Areas Responding to High Probability Actions We conducted a separate intersection analysis to deter- mine brain areas responding preferentially to the obser- vation of high-probability actions based on either dis- tance or fullness cues. Here, we calculated the conjunc- tion (HPd − LPd) ∩ (HPf − LPf). This analysis revealed that BOLD signal was significantly greater during the observation of high as compared to low-probability ac- tions at sites in the left SMG (BA 41 and 48), precuneus (BA 7), left superior frontal sulcus (BA 48) and bilateral isual/occipital cortex (BA 17,18,19) (Table 1, Figure 3). v Copyright © 2013 SciRes. JBBS
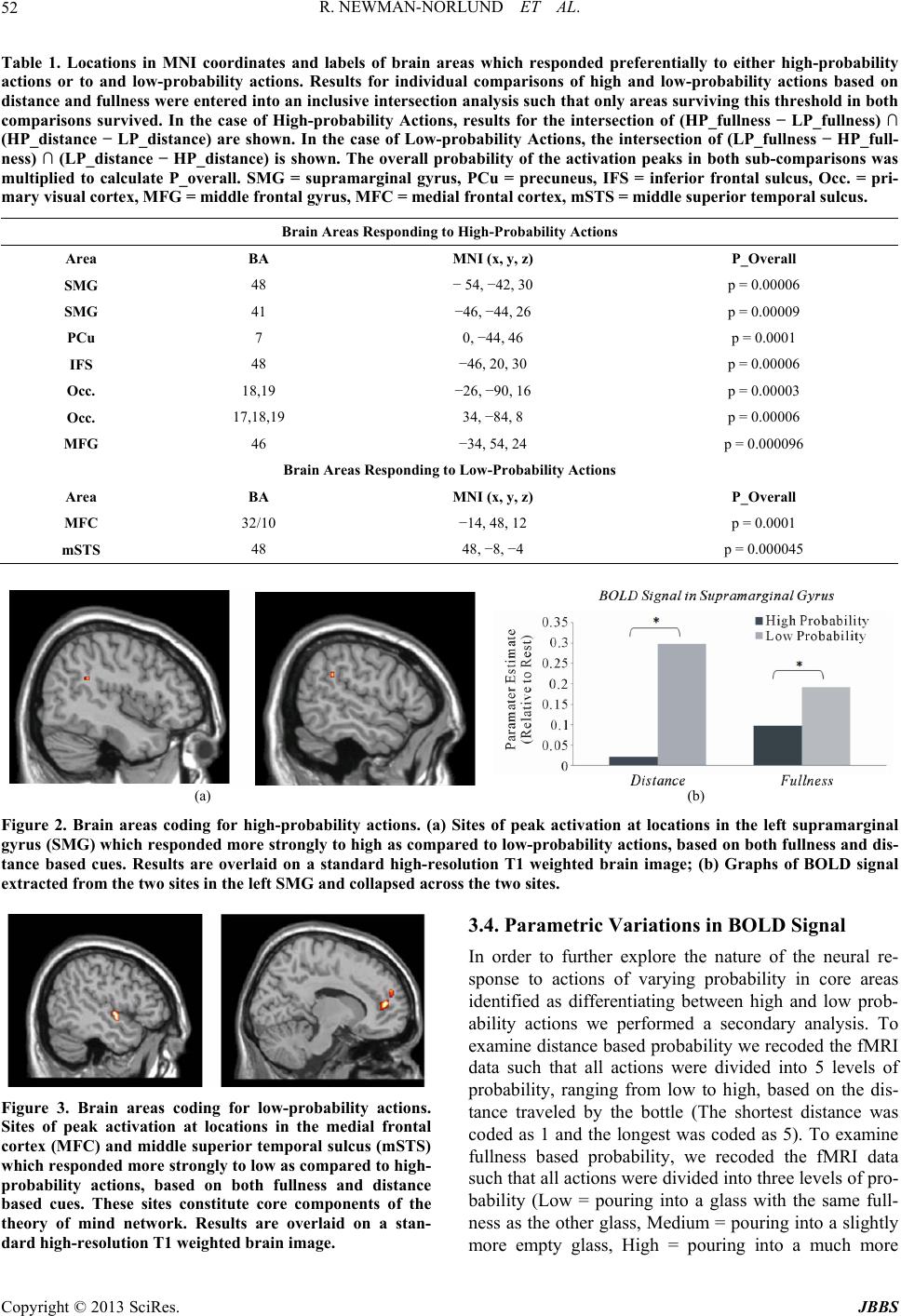 R. NEWMAN-NORLUND ET AL. Copyright © 2013 SciRes. JBBS 52 Table 1. Locations in MNI coordinates and labels of brain areas which responded preferentially to either high-probability actions or to and low-probability actions. Results for individual comparisons of high and low-probability actions based on distance and fullness were entered into an inclusive intersection analysis such that only areas surviving this threshold in both comparisons survived. In the case of High-probability Actions, results for the intersection of (HP_fullness − LP_fullness) ∩ (HP_distance − LP_distance) are shown. In the case of Low-probability Actions, the intersection of (LP_fullness − HP_full- ness) ∩ (LP_distance − HP_distance) is shown. The overall probability of the activation peaks in both sub-comparisons was multiplied to calculate P_overall. SMG = supramarginal gyrus, PCu = precuneus, IFS = inferior frontal sulcus, Occ. = pri- mary visual cortex, MFG = middle frontal gyrus, MFC = medial frontal cortex, mSTS = middle superior temporal sulcus. Brain Areas Responding to High-Probability Actions Area BA MNI (x, y, z) P_Overall SMG 48 − 54, −42, 30 p = 0.00006 SMG 41 −46, −44, 26 p = 0.00009 PCu 7 0, −44, 46 p = 0.0001 IFS 48 −46, 20, 30 p = 0.00006 Occ. 18,19 −26, −90, 16 p = 0.00003 Occ. 17,18,19 34, −84, 8 p = 0.00006 MFG 46 −34, 54, 24 p = 0.000096 Brain Areas Responding to Low-Probability Actions Area BA MNI (x, y, z) P_Overall MFC 32/10 −14, 48, 12 p = 0.0001 mSTS 48 48, −8, −4 p = 0.000045 (a) (b) Figure 2. Brain areas coding for high-probability actions. (a) Sites of peak activation at locations in the left supramarginal gyrus (SMG) which responded more strongly to high as compared to low-probability actions, based on both fullness and dis- tance based cues. Results are overlaid on a standard high-resolution T1 weighted brain image; (b) Graphs of BOLD signal extracted from the two sites in the left SMG and collapsed across the two sites. 3.4. Parametric Variations in BOLD Signal In order to further explore the nature of the neural re- sponse to actions of varying probability in core areas identified as differentiating between high and low prob- ability actions we performed a secondary analysis. To examine distance based probability we recoded the fMRI data such that all actions were divided into 5 levels of probability, ranging from low to high, based on the dis- tance traveled by the bottle (The shortest distance was coded as 1 and the longest was coded as 5). To examine fullness based probability, we recoded the fMRI data such that all actions were divided into three levels of pro- bability (Low = pouring into a glass with the same full- ness as the other glass, Medium = pouring into a slightly more empty glass, High = pouring into a much more Figure 3. Brain areas coding for low-probability actions. Sites of peak activation at locations in the medial frontal cortex (MFC) and middle superior temporal sulcus (mSTS) which responded more strongly to low as compared to high- probability actions, based on both fullness and distance based cues. These sites constitute core components of the theory of mind network. Results are overlaid on a stan- dard high-resolution T1 weighted brain image.
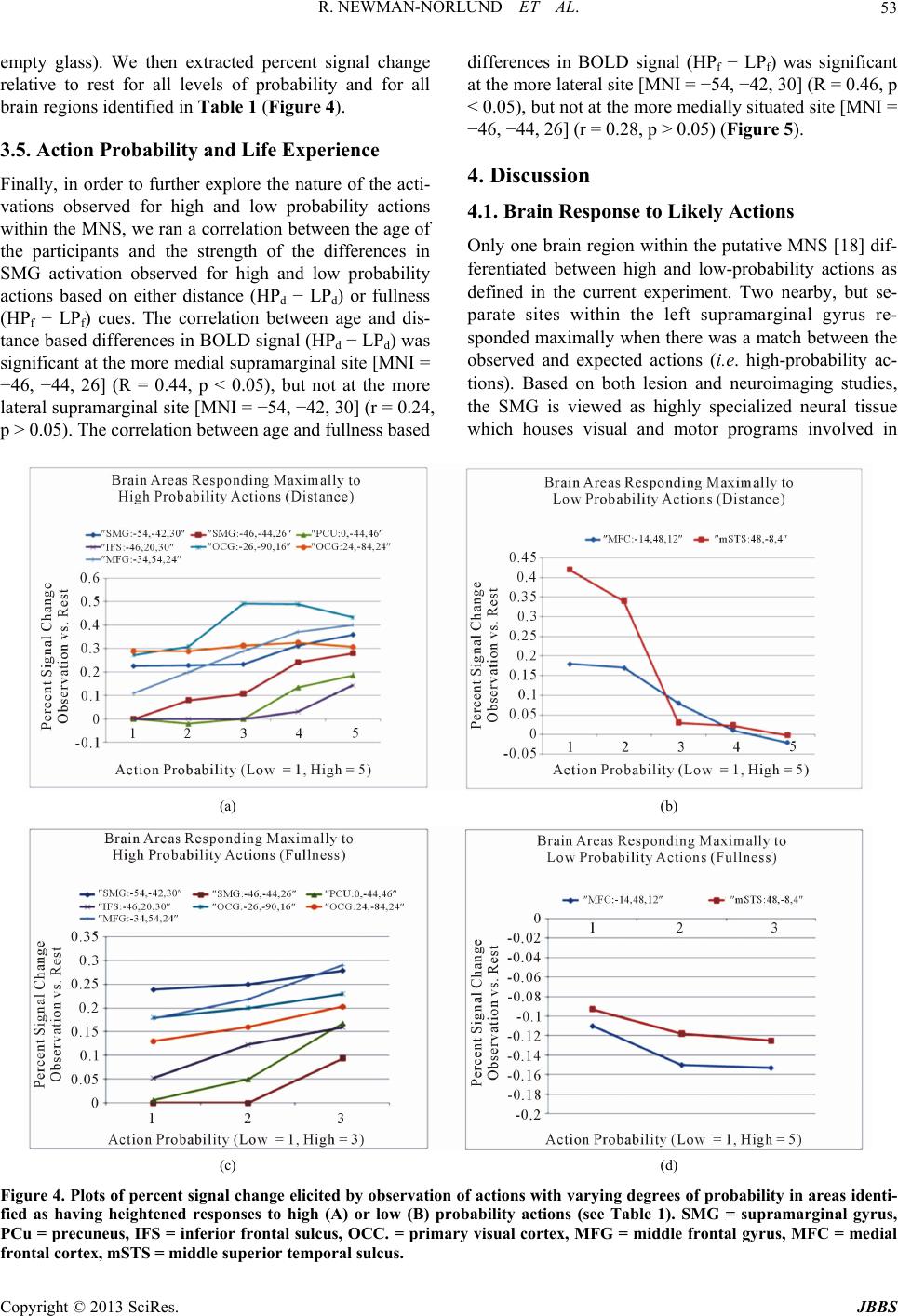 R. NEWMAN-NORLUND ET AL. 53 empty glass). We then extracted percent signal change relative to rest for all levels of probability and for all brain regions identified in Table 1 (Figure 4). 3.5. Action Probability and Life Experience Finally, in order to further explore the nature of the acti- vations observed for high and low probability actions within the MNS, we ran a correlation between the age of the participants and the strength of the differences in SMG activation observed for high and low probability actions based on either distance (HPd − LPd) or fullness (HPf − LPf) cues. The correlation between age and dis- tance based differences in BOLD signal (HPd − LPd) was significant at the more medial supramarginal site [MNI = −46, −44, 26] (R = 0.44, p < 0.05), but not at the more lateral supramarginal site [MNI = −54, −42, 30] (r = 0.24, p > 0.05). The correlation between age and fullness based differences in BOLD signal (HPf − LPf) was significant at the more lateral site [MNI = −54, −42, 30] (R = 0.46, p < 0.05), but not at the more medially situated site [MNI = −46, −44, 26] (r = 0.28, p > 0.05) (Figure 5). 4. Discussion 4.1. Brain Response to Likely Actions Only one brain region within the putative MNS [18] dif- ferentiated between high and low-probability actions as defined in the current experiment. Two nearby, but se- parate sites within the left supramarginal gyrus re- sponded maximally when there was a match between the observed and expected actions (i.e. high-probability ac- tions). Based on both lesion and neuroimaging studies, the SMG is viewed as highly specialized neural tissue which houses visual and motor programs involved in (a) (b) (c) (d) Figure 4. Plots of percent signal change elicited by observation of actions with varying degrees of probability in areas identi- fied as having heightened responses to high (A) or low (B) probability actions (see Table 1). SMG = supramarginal gyrus, PCu = precuneus, IFS = inferior frontal sulcus, OCC. = primary visual cortex, MFG = middle frontal gyrus, MFC = medial frontal cortex, mSTS = middle superior temporal sulcus. Copyright © 2013 SciRes. JBBS
 R. NEWMAN-NORLUND ET AL. 54 Figure 5. Correlation between age and the strength of the difference in BOLD signal elicited by high and low-probability ac- tions (HP-LP) at sites in the supramarginal gyrus. Actions could be categorized as high or low probability based on either 1) the relative Fullness of the glass being poured into or 2) the Distance between the bottle and poured-into glass. At one site in the supramarginal gyrus [MNI = −54, −42, 30], the correlation between Fullness based HP-LP was significant, (R = 0.46, p < 0.05), but when the probability was based on Distance, the correlation was not significant (R = 0.24, p > 0.05). At the other supramarginal site [MNI = −46, −44, 26], the correlation between Distance based HP-LP was significant (R = 0.44, p < 0.05), while the strength of this correlation was not significant when probability was based on Fullness. skilled tool use [19-23]. Interestingly, sites in the MNS were found to be co-activated with the SMG in this and other experiments, suggesting a close functional relation- ship with each other [24,25]. The current data suggest an important role for the left SMG in differentiating be- tween actions of low and high probability. Adopting a probabilistic perspective allows us to look at skilled tool use in a new way. Tool use is made possible by the abi- lity to infer future actions specific to that tool. Such in- ferences are especially important to tool/object use be- cause proper handling of these items is typically asso- ciated with complex sequences of actions which must be executed in the appropriate order. For example, a mallet implies a series of actions associated with pounding in or removing a nail. It may be interesting to consider how certain brain sites, such as the SMG, come to respond differently to actions of either high or low probabilities. The simplest and most parsimonious explanation is that probabilities are, as implied by Bayes’ Rule, based on prior experience or entrainment. In the case of the current experiment, participants’ personal experience in social drinking may have led to the formation of specific expectations regard- ing the relationship between the relative fullness of two glasses and subsequent pouring actions. The relationship between inter-object distance and probability, on the other hand, may be rooted in the more general experience of preferring the combination of proximate objects across a variety of contexts, something commonly referred to as the Gestalt principle of Proximity (e.g. we might eat the closest French fry off our plate first, the most distant last). The present research indicates a common neural substrate for evaluating action probabilities based on both types of cues (fullness and distance). An interesting finding in the current experiment is that we did observe a correlation between age, which is re- presentative of a participant’s general life experience, the strength of the probability coding at sites in the supra marginal gyrus. It seems that the two sites within the left supramarginal gyrus, while both responding more strongly to high-probability actions, were differentially sensitive whether or not the likelihood of an action was related to the proximity of objects being combined or the relative need to refill a certain glass. First, this finding makes it less likely that the supramarginal activations uncovered in the conjunction analysis are the result of insufficient thresholding (i.e. Type I errors). Second, this finding seems to support the idea that the supramarginal gyrus’s response to action probabilities is, at least in part, go- verned by prior experience (either amount or type). This finding also raises the interesting possibility that different sub-areas within the parietal lobe may code for proba- bility of actions based on different types of cues (e.g. fullness or distance based). Of course this claim is highly speculative and further experimentation is needed to con- firm this hypothesis. More sensitive questionnaires, per- haps dealing with specific types of motor experience (which would not rely on assuming on the link between age and specific types of experiences), could be used in future studies which examine patterns of neural activity elicited by high and low-probability actions. Finally, we would like to note that the precuneus and primary visual cortices showed the same pattern of res- ponses as the SMG during observation of probable and Copyright © 2013 SciRes. JBBS
 R. NEWMAN-NORLUND ET AL. 55 improbable actions, although these activity patterns were not correlated with participants’ age. The precuneus is thought to be involved in visuo-spatial imagery (as is pri- mary visual cortex) and episodic memory retrieval [26, 27]. The co-activation of these areas during the obser- vation of high-probability actions suggests a relationship between past experience, upon which action probabilities are probably built and mental imagery, which could be used to replay or simulate previously observed actions. The precise nature of the relationship between learning, mental imagery and probability coding requires further investigation. 4.2. Brain Response to Unlikely Actions During observation of low probability actions, sites com- monly associated with evaluating the intentions of others, including the medial frontal cortex and the superior tem- poral sulcus, were found to be active. The MFC is a core component of the theory of mind (ToM) network, a net- work found to be active when people evaluate about or consider the intentions of other people. Based on nu- merous studies reporting co-activation of STS and MFC [28-31], as well as the known anatomical connectivity between these two areas [32], co-activation in these two areas has been hypothesized to reflect core mentalizing processes [33]. The current data are consistent with this interpretation. As such, we take this activity in the MFC and mSTS to represent subjects’ attempt to understand the intentions behind the actor’s improbable movement choice. Also informative is our failure to find MNS in- volvement during the observation of low-probability ac- tions. Indeed, the exact role of the MNS in the processing of atypical, or odd actions, is currently under debate. Some evidence suggests that humans rely on their own motor systems to make sense of others’ actions, and to recognize their intentions [11,14,18,34], while other au- thors have failed to observe additional MNS activation during the observation of atypical actions [13]. 5. Summary and Conclusions Results from the present experiment are consistent with the idea that the human brain differentiates between ac- tions of differing probability insofar as the functional sig- natures of probable and improbable actions can be dif- ferentiated using modern neuroscience techniques. As such, these data take an important step towards validating mathematical models of psychological phenomenon which incorporate probabilistic equations. The current results also raise a number of interesting questions regarding the neural underpinnings of the highly sophisticated human apacity for estimating action likeli- hoods. For example, how exactly do specific brain areas become entrained to differentiate high and low probabil- ity actions? And does our ability to estimate an action’s likelihood emerge differently depending on the specific types of cues we use to arrive at our estimates? Perhaps most interestingly, what sorts of computational models of mirror neurons might account for the responses observed in euroimaging experiments involving observation of us- ual and unusual actions? Besides obvious implications for researchers studying action/intention recognition, so- cial-motor control and joint action, it is very likely that a better understanding of the neural mechanisms that sup- port the human capacity for action prediction could lead to radical improvements in the quality of human-human and human-computer interactions. 6. Acknowledgements The present research was supported by the EU-project Joint Action Science and Technology (JAST) (IST-FP6- 003747). REFERENCES [1] H. R. Heekeren, S. Marrett and L. G. Ungerleider, “The Neural Systems That Mediate Human Perceptual Deci- sion Making,” Nature Reviews Neuroscience, Vol. 9, No. 6, 2008, pp. 467-479. doi:10.1038/nrn2374 [2] R. H. Cuijpers, et al., “Goals and Means in Action Ob- servation: A Computational Approach,” Neural Networks, Vol. 19, No. 3, 2006, pp. 311-322. doi:10.1016/j.neunet.2006.02.004 [3] M. Haruno, D. M. Wolpert and M. Kawato, “MOSAIC Model for Sensorimotor Learning and Control,” Neural Computation, Vol. 13, No. 10, 2001, pp. 2201-2220. doi:10.1162/089976601750541778 [4] E. Oztop, D. Wolpert and M. Kawato, “Mental State In- ference Using Visual Control Parameters,” Cognitive Brain Research, Vol. 22, No. 2, 2005, pp. 129-151. doi:10.1016/j.cogbrainres.2004.08.004 [5] D. M. Wolpert, “Probabilistic Models in Human Senso- rimotor Control,” Human Movement Science, Vol. 26, No. 4, 2007, pp. 511-524. doi:10.1016/j.humov.2007.05.005 [6] J. I. Gold and M. N. Shadlen, “The Neural Basis of Deci- sion Making,” Annual Review of Neuroscience, Vol. 30, 2007, pp. 535-574. doi:10.1146/annurev.neuro.29.051605.113038 [7] T. Yang and M. N. Shadlen, “Probabilistic Reasoning by Neurons,” Nature, Vol. 447, No. 7148, 2007, pp. 1075- 1080. doi:10.1038/nature05852 [8] K. Preuschoff, S. R. Quartz and P. P. Bossaerts, “Human Insula Activation Reflects Risk Prediction Errors as Well as Risk,” Journal of Neuroscience, Vol. 28, No. 1, 2008, pp. 2745-2752. doi:10.1523/JNEUROSCI.4286-07.2008 [9] K. Samejima, et al., “Representation of Action-Specific Reward Values in the Striatum,” Science, Vol. 310, No. 5752, 2005, pp. 1337-1340. doi:10.1126/science.1115270 [10] B. Calvo-Merino, et al., “Seeing or Doing? Influence of Copyright © 2013 SciRes. JBBS
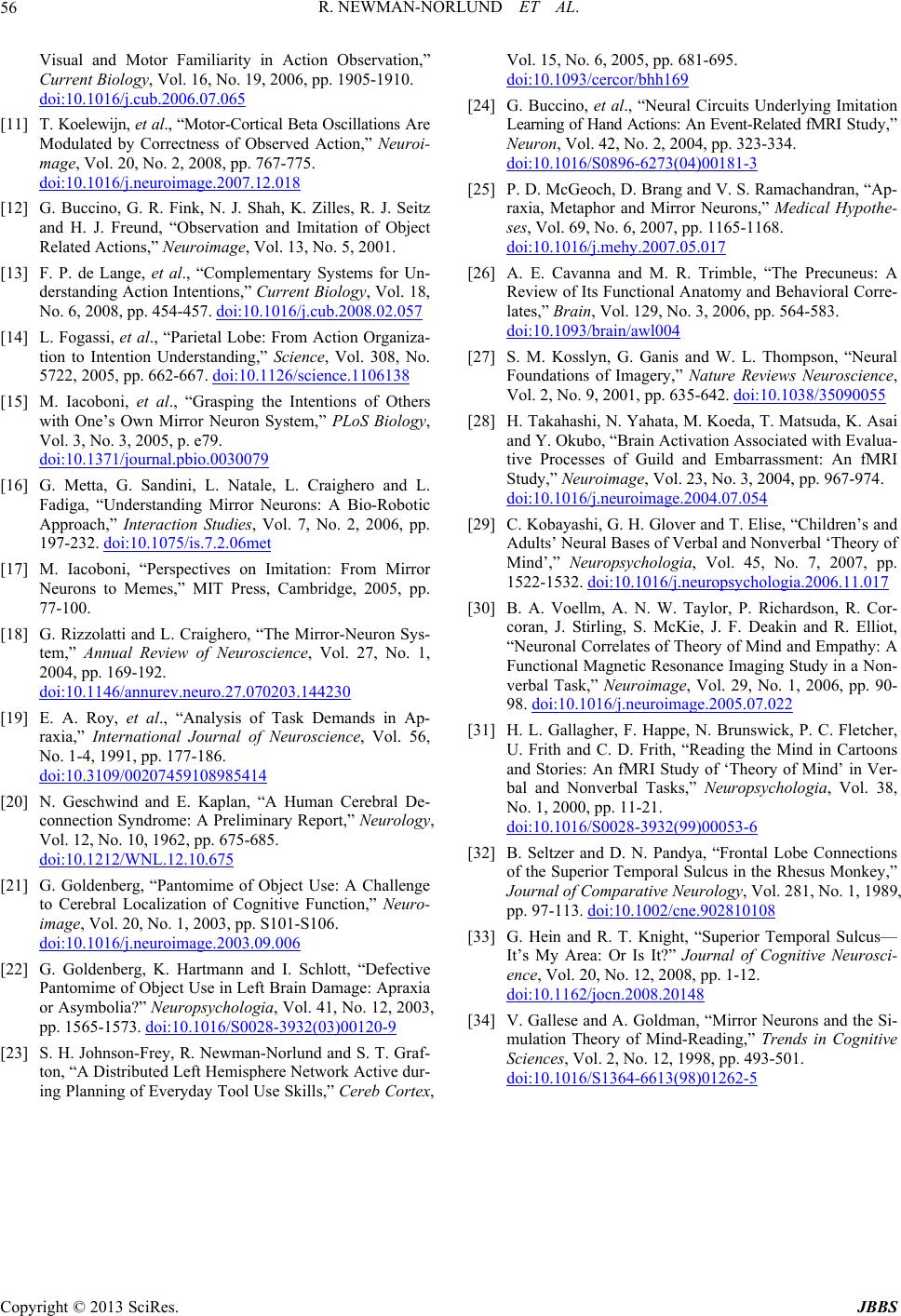 R. NEWMAN-NORLUND ET AL. Copyright © 2013 SciRes. JBBS 56 Visual and Motor Familiarity in Action Observation,” Current Biology, Vol. 16, No. 19, 2006, pp. 1905-1910. doi:10.1016/j.cub.2006.07.065 [11] T. Koelewijn, et al., “Motor-Cortical Beta Oscillations Are Modulated by Correctness of Observed Action,” Neuroi- mage, Vol. 20, No. 2, 2008, pp. 767-775. doi:10.1016/j.neuroimage.2007.12.018 [12] G. Buccino, G. R. Fink, N. J. Shah, K. Zilles, R. J. Seitz and H. J. Freund, “Observation and Imitation of Object Related Actions,” Neuroimage, Vol. 13, No. 5, 2001. [13] F. P. de Lange, et al., “Complementary Systems for Un- derstanding Action Intentions,” Current Biology, Vol. 18, No. 6, 2008, pp. 454-457. doi:10.1016/j.cub.2008.02.057 [14] L. Fogassi, et al., “Parietal Lobe: From Action Organiza- tion to Intention Understanding,” Science, Vol. 308, No. 5722, 2005, pp. 662-667. doi:10.1126/science.1106138 [15] M. Iacoboni, et al., “Grasping the Intentions of Others with One’s Own Mirror Neuron System,” PLoS Biology, Vol. 3, No. 3, 2005, p. e79. doi:10.1371/journal.pbio.0030079 [16] G. Metta, G. Sandini, L. Natale, L. Craighero and L. Fadiga, “Understanding Mirror Neurons: A Bio-Robotic Approach,” Interaction Studies, Vol. 7, No. 2, 2006, pp. 197-232. doi:10.1075/is.7.2.06met [17] M. Iacoboni, “Perspectives on Imitation: From Mirror Neurons to Memes,” MIT Press, Cambridge, 2005, pp. 77-100. [18] G. Rizzolatti and L. Craighero, “The Mirror-Neuron Sys- tem,” Annual Review of Neuroscience, Vol. 27, No. 1, 2004, pp. 169-192. doi:10.1146/annurev.neuro.27.070203.144230 [19] E. A. Roy, et al., “Analysis of Task Demands in Ap- raxia,” International Journal of Neuroscience, Vol. 56, No. 1-4, 1991, pp. 177-186. doi:10.3109/00207459108985414 [20] N. Geschwind and E. Kaplan, “A Human Cerebral De- connection Syndrome: A Preliminary Report,” Neurology, Vol. 12, No. 10, 1962, pp. 675-685. doi:10.1212/WNL.12.10.675 [21] G. Goldenberg, “Pantomime of Object Use: A Challenge to Cerebral Localization of Cognitive Function,” Neuro- image, Vol. 20, No. 1, 2003, pp. S101-S106. doi:10.1016/j.neuroimage.2003.09.006 [22] G. Goldenberg, K. Hartmann and I. Schlott, “Defective Pantomime of Object Use in Left Brain Damage: Apraxia or Asymbolia?” Neuropsychologia, Vol. 41, No. 12, 2003, pp. 1565-1573. doi:10.1016/S0028-3932(03)00120-9 [23] S. H. Johnson-Frey, R. Newman-Norlund and S. T. Graf- ton, “A Distributed Left Hemisphere Network Active dur- ing Planning of Everyday Tool Use Skills,” Cereb Cortex, Vol. 15, No. 6, 2005, pp. 681-695. doi:10.1093/cercor/bhh169 [24] G. Buccino, et al., “Neural Circuits Underlying Imitation Learning of Hand Actions: An Event-Related fMRI Study,” Neuron, Vol. 42, No. 2, 2004, pp. 323-334. doi:10.1016/S0896-6273(04)00181-3 [25] P. D. McGeoch, D. Brang and V. S. Ramachandran, “Ap- raxia, Metaphor and Mirror Neurons,” Medical Hypothe- ses, Vol. 69, No. 6, 2007, pp. 1165-1168. doi:10.1016/j.mehy.2007.05.017 [26] A. E. Cavanna and M. R. Trimble, “The Precuneus: A Review of Its Functional Anatomy and Behavioral Corre- lates,” Brain, Vol. 129, No. 3, 2006, pp. 564-583. doi:10.1093/brain/awl004 [27] S. M. Kosslyn, G. Ganis and W. L. Thompson, “Neural Foundations of Imagery,” Nature Reviews Neuroscience, Vol. 2, No. 9, 2001, pp. 635-642. doi:10.1038/35090055 [28] H. Takahashi, N. Yahata, M. Koeda, T. Matsuda, K. Asai and Y. Okubo, “Brain Activation Associated with Evalua- tive Processes of Guild and Embarrassment: An fMRI Study,” Neuroimage, Vol. 23, No. 3, 2004, pp. 967-974. doi:10.1016/j.neuroimage.2004.07.054 [29] C. Kobayashi, G. H. Glover and T. Elise, “Children’s and Adults’ Neural Bases of Verbal and Nonverbal ‘Theory of Mind’,” Neuropsychologia, Vol. 45, No. 7, 2007, pp. 1522-1532. doi:10.1016/j.neuropsychologia.2006.11.017 [30] B. A. Voellm, A. N. W. Taylor, P. Richardson, R. Cor- coran, J. Stirling, S. McKie, J. F. Deakin and R. Elliot, “Neuronal Correlates of Theory of Mind and Empathy: A Functional Magnetic Resonance Imaging Study in a Non- verbal Task,” Neuroimage, Vol. 29, No. 1, 2006, pp. 90- 98. doi:10.1016/j.neuroimage.2005.07.022 [31] H. L. Gallagher, F. Happe, N. Brunswick, P. C. Fletcher, U. Frith and C. D. Frith, “Reading the Mind in Cartoons and Stories: An fMRI Study of ‘Theory of Mind’ in Ver- bal and Nonverbal Tasks,” Neuropsychologia, Vol. 38, No. 1, 2000, pp. 11-21. doi:10.1016/S0028-3932(99)00053-6 [32] B. Seltzer and D. N. Pandya, “Frontal Lobe Connections of the Superior Temporal Sulcus in the Rhesus Monkey,” Journal of Comparative Neurology, Vol. 281, No. 1, 1989, pp. 97-113. doi:10.1002/cne.902810108 [33] G. Hein and R. T. Knight, “Superior Temporal Sulcus— It’s My Area: Or Is It?” Journal of Cognitive Neurosci- ence, Vol. 20, No. 12, 2008, pp. 1-12. doi:10.1162/jocn.2008.20148 [34] V. Gallese and A. Goldman, “Mirror Neurons and the Si- mulation Theory of Mind-Reading,” Trends in Cognitive Sciences, Vol. 2, No. 12, 1998, pp. 493-501. doi:10.1016/S1364-6613(98)01262-5
|