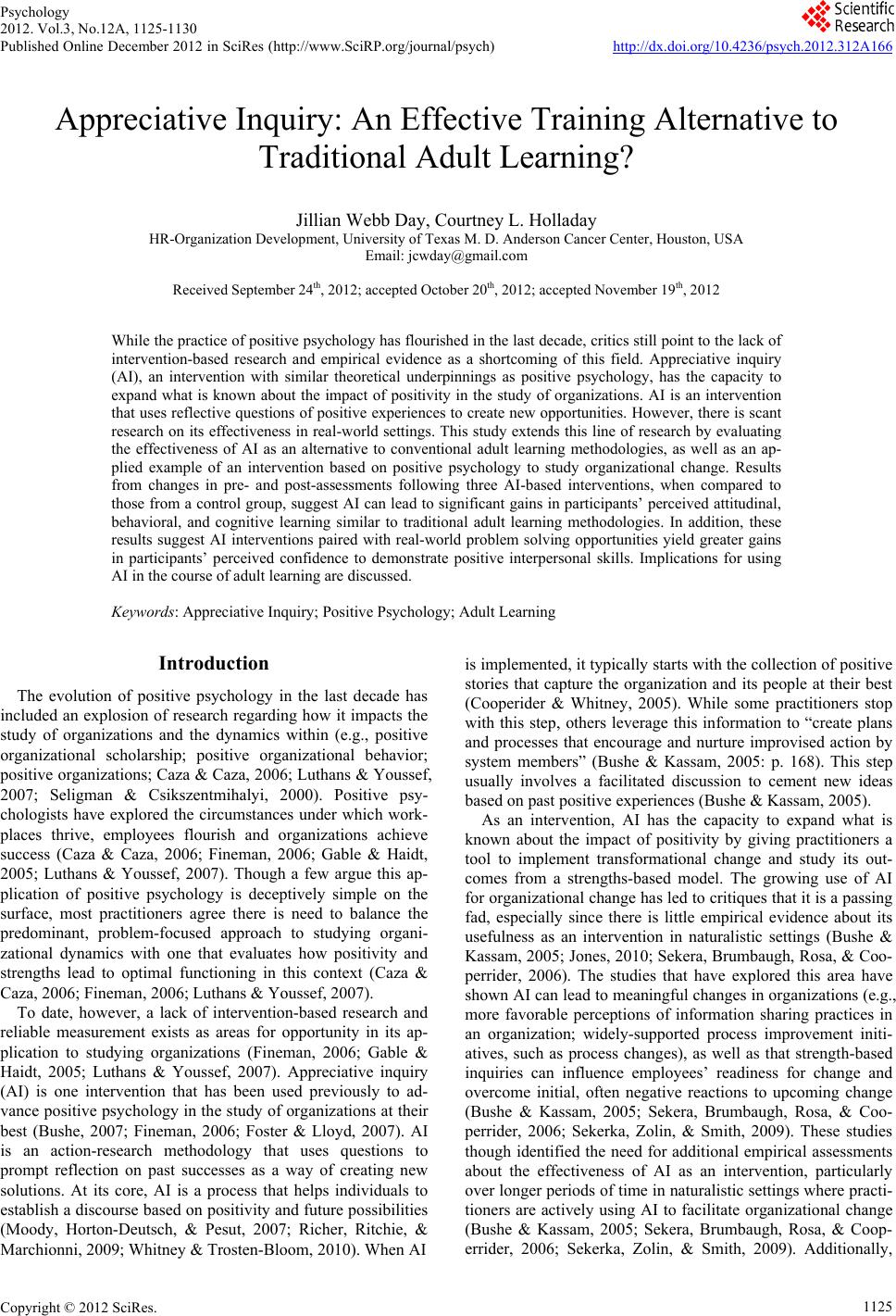 Psychology 2012. Vol.3, No.12A, 1125-1130 Published Online December 2012 in SciRes (http://www.SciRP.org/journal/psych) http://dx.doi.org/10.4236/psych.2012.312A166 Copyright © 2012 SciRes. 1125 Appreciative Inquiry: An Effective Training Alternative to Traditional Adult Learning? Jillian Webb Day, Courtney L. Holladay HR-Organization Development, University of Texas M. D. Anderson Cancer Center, Houston, USA Email: jcwday@gmail.com Received September 24th, 2012; accepted October 20th, 2012; accepted November 19th, 2012 While the practice of positive psychology has flourished in the last decade, critics still point to the lack of intervention-based research and empirical evidence as a shortcoming of this field. Appreciative inquiry (AI), an intervention with similar theoretical underpinnings as positive psychology, has the capacity to expand what is known about the impact of positivity in the study of organizations. AI is an intervention that uses reflective questions of positive experiences to create new opportunities. However, there is scant research on its effectiveness in real-world settings. This study extends this line of research by evaluating the effectiveness of AI as an alternative to conventional adult learning methodologies, as well as an ap- plied example of an intervention based on positive psychology to study organizational change. Results from changes in pre- and post-assessments following three AI-based interventions, when compared to those from a control group, suggest AI can lead to significant gains in participants’ perceived attitudinal, behavioral, and cognitive learning similar to traditional adult learning methodologies. In addition, these results suggest AI interventions paired with real-world problem solving opportunities yield greater gains in participants’ perceived confidence to demonstrate positive interpersonal skills. Implications for using AI in the course of adult learning are discussed. Keywords: Appreciative Inquiry; Positive Psychology; Adult Learning Introduction The evolution of positive psychology in the last decade has included an explosion of research regarding how it impacts the study of organizations and the dynamics within (e.g., positive organizational scholarship; positive organizational behavior; positive organizations; Caza & Caza, 2006; Luthans & Youssef, 2007; Seligman & Csikszentmihalyi, 2000). Positive psy- chologists have explored the circumstances under which work- places thrive, employees flourish and organizations achieve success (Caza & Caza, 2006; Fineman, 2006; Gable & Haidt, 2005; Luthans & Youssef, 2007). Though a few argue this ap- plication of positive psychology is deceptively simple on the surface, most practitioners agree there is need to balance the predominant, problem-focused approach to studying organi- zational dynamics with one that evaluates how positivity and strengths lead to optimal functioning in this context (Caza & Caza, 2006; Fineman, 2006; Luthans & Youssef, 2007). To date, however, a lack of intervention-based research and reliable measurement exists as areas for opportunity in its ap- plication to studying organizations (Fineman, 2006; Gable & Haidt, 2005; Luthans & Youssef, 2007). Appreciative inquiry (AI) is one intervention that has been used previously to ad- vance positive psychology in the study of organizations at their best (Bushe, 2007; Fineman, 2006; Foster & Lloyd, 2007). AI is an action-research methodology that uses questions to prompt reflection on past successes as a way of creating new solutions. At its core, AI is a process that helps individuals to establish a discourse based on positivity and future possibilities (Moody, Horton-Deutsch, & Pesut, 2007; Richer, Ritchie, & Marchionni, 2009; Whitney & Trosten-Bloom, 2010). When AI is implemented, it typically starts with the collection of positive stories that capture the organization and its people at their best (Cooperider & Whitney, 2005). While some practitioners stop with this step, others leverage this information to “create plans and processes that encourage and nurture improvised action by system members” (Bushe & Kassam, 2005: p. 168). This step usually involves a facilitated discussion to cement new ideas based on past positive experiences (Bushe & Kassam, 2005). As an intervention, AI has the capacity to expand what is known about the impact of positivity by giving practitioners a tool to implement transformational change and study its out- comes from a strengths-based model. The growing use of AI for organizational change has led to critiques that it is a passing fad, especially since there is little empirical evidence about its usefulness as an intervention in naturalistic settings (Bushe & Kassam, 2005; Jones, 2010; Sekera, Brumbaugh, Rosa, & Coo- perrider, 2006). The studies that have explored this area have shown AI can lead to meaningful changes in organizations (e.g., more favorable perceptions of information sharing practices in an organization; widely-supported process improvement initi- atives, such as process changes), as well as that strength-based inquiries can influence employees’ readiness for change and overcome initial, often negative reactions to upcoming change (Bushe & Kassam, 2005; Sekera, Brumbaugh, Rosa, & Coo- perrider, 2006; Sekerka, Zolin, & Smith, 2009). These studies though identified the need for additional empirical assessments about the effectiveness of AI as an intervention, particularly over longer periods of time in naturalistic settings where practi- tioners are actively using AI to facilitate organizational change (Bushe & Kassam, 2005; Sekera, Brumbaugh, Rosa, & Coop- errider, 2006; Sekerka, Zolin, & Smith, 2009). Additionally,
 J. W. DAY, C. L. HOLLADAY since AI is based on the idea that the method of inquiry shapes the information uncovered, there is a need to explore whether different modes of AI are more effective than others at facili- tating certain types of change (Sekera, Brumbaugh, Rosa, & Cooperrider, 2006; Sekerka, Zolin, & Smith, 2009). This study will expand on the research in this arena by em- pirically investigating the effectiveness of different modes of AI when compared to a traditional, problem-based approach to organizational change in a naturalistic setting. For our purposes, we define organizational change as it is depicted in Lewin’s (1951) force field analysis model. In the context of our study, this means changing employees’ perceptions of their soft skills from the current state to a more favorable state in which they are more confident in the relevant knowledge, skills and abili- ties. Specifically, this study seeks to provide preliminary evi- dence about the effectiveness of a strength-based intervention, namely AI, as a training intervention to enhance employees’ soft skills that contribute to the optimal functioning of organi- zations and workgroups. In doing so, this study seeks to address one of the criticisms regarding the use of positive psychology to study organizational change; namely, that there is little re- search on the efficacy of strength-based interventions in rela- tion to the predominant problem-based approach of investigat- ing organizations and their dynamics (Gable & Haidt, 2005; Luthans & Youssef, 2007). As previously mentioned, AI is an intervention frequently associated with and used in the name of positive psychology. This is primarily because both AI and positive psychology share some of the same philosophical underpinnings, namely focusing on strengths first, identifying the conditions under which people and organizations flourish, and constructing pos- sible realities with a focus on the positive elements that ener- gize (Bushe, 2007; Fineman, 2006; Foster & Lloyd, 2007). For example, asking questions to identify the conditions under which people have successfully performed in the past is one of the first steps taken when AI is used (Whitney & Trosten- Bloom, 2010). This step supports one of the earliest definitions of positive psychology by Seligman & Csikszentmihalyi (2000): that it is a “psychology of positive human functioning” (p. 13). The positive functioning in turn can lead to an exchange of meaningful dialogue to identify past successes that can help build a future in which people flourish (Moody, Horton- Deutsch, & Pesult, 2007; Whitney & Trosten-Bloom, 2010). In another instance within AI, it is the idea that positivity, spe- cifically positive inquiry, can lead to transformational change because the change in inquiry prompts new ways of looking at old problems and generates new possibilities for the future (Bushe, 2007, 2010; Whitney & Trosten-Bloom, 2010). While AI has been used in various organizational settings such as healthcare (Moody, Horton-Deutsch, & Pesut, 2007; Richer, Ritchie, & Marchionni, 2009), the few studies that evaluated its impact were limited by research design. (Bushe & Kassam, 2005; Jones, 2010; Sekera, Brumbaugh, Rosa, & Co- operrider, 2006). For example, the field studies by Sekura, Brumbaugh, Rosa and Cooperrider (2006) and Sekura and Zolin (2009) randomly assigned participants to study conditions and gathered information through one-on-one interviews. However, the practice of AI can involve individuals sharing their positive experiences in groups (Cooperrider & Whitney, 2005). Additionally, while these results suggest AI can posi- tively influence employees’ perceptions on a particular topic (i.e., readiness for change), they do not measure the changes in employees’ perceptions as a result of participating in an AI- based intervention. Indeed, the lack of intervention-based research is often a criticism of positive psychology in general. While AI could be a possible intervention to explore for this reason, the research on AI can be expanded to empirically evaluate its changes on employees’ perceptions when compared to the predominant, problem-based approaches to assess organizational problems within a naturalistic setting (Bushe & Kassam, 2005; Grant & Humphries, 2006; Jones, 2010; Sekera, Brumbaugh, Rosa, & Cooperrider, 2006). Problem-based approaches typically focus first on identifying the deficit in the organization, then devising a solution that fixes the problem (Sekerka, Zolin, & Smith, 2009). While a number of studies have demonstrated that AI can lead to meaningful changes in organizations, there are oppor- tunities to build upon this research (Bushe & Kassam, 2005; Jones, 2010; Sekera, Brumbaugh, Rosa, & Cooperrider, 2006). Our study seeks to address this gap by empirically evaluating how effective AI is as a training intervention to enhance em- ployees’ soft skills that contribute to positive organizational behavior. For that reason, Hypothesis 1 postulates that parti- cipants’ perceived knowledge, skills and attitudes related to creating positive interpersonal dynamics will be significantly higher after participating in AI-based interventions. As previously mentioned, AI is often critiqued for the lack of empirical evidence about its effectiveness (Bushe & Kassam, 2005; Jones, 2010; Sekera, Brumbaugh, Rosa, & Cooperrider, 2006). Instead, most of the literature on AI has provided anec- dotal evidence about the power of this method, as opposed to empirically evaluating when it works best or moderators influ- encing the effect of its impact and sustainability over time (Bushe, 2011; Jones, 2010). Consequently, although AI is touted as an intervention that can yield results quickly and is straightforward to implement (Jones, 2010), the sentiment re- mains from some critics that AI is a passing management fad. A limited number of studies have empirically evaluated the impact of AI as a strength-based intervention that can yield observable positive changes in organizations. Summarizing the research to date was a meta-analysis that reviewed the con- ditions under which AI led to transformational outcomes (Bushe & Kassam, 2005). More recent research has taken a field approach to examine different inquiry strategies’ (i.e., strength- or problem-based) influence on employees’ readiness for change. The studies found the highest positive effect in engagement to a change process was reported among groups that participated in an AI intervention as compared to those who were engaged through a problem-based approach (Sekera, Brumbaugh, Rosa, & Cooperrider, 2006; Sekerka, Zolin, & Smith, 2009). This evidence suggests that strength-based ap- proaches to organizational change, such as AI, can have a posi- tive impact on those experiencing the change and their readi- ness to engage and support it. However, as previously men- tioned, there are opportunities to expand this line of research by evaluating how AI impacts organizational change over time through changes in employees’ perceptions on different matters, such as their abilities to demonstrate positive interpersonal skills. Another opportunity to expand this research is to com- pare how different strength-based approaches, such as the variations in the delivery of AI, are more or less effective. In- deed, these are future areas of research identified as needing exploration (Sekera, Brumbaugh, Rosa, & Cooperrider, 2006; Copyright © 2012 SciRes. 1126
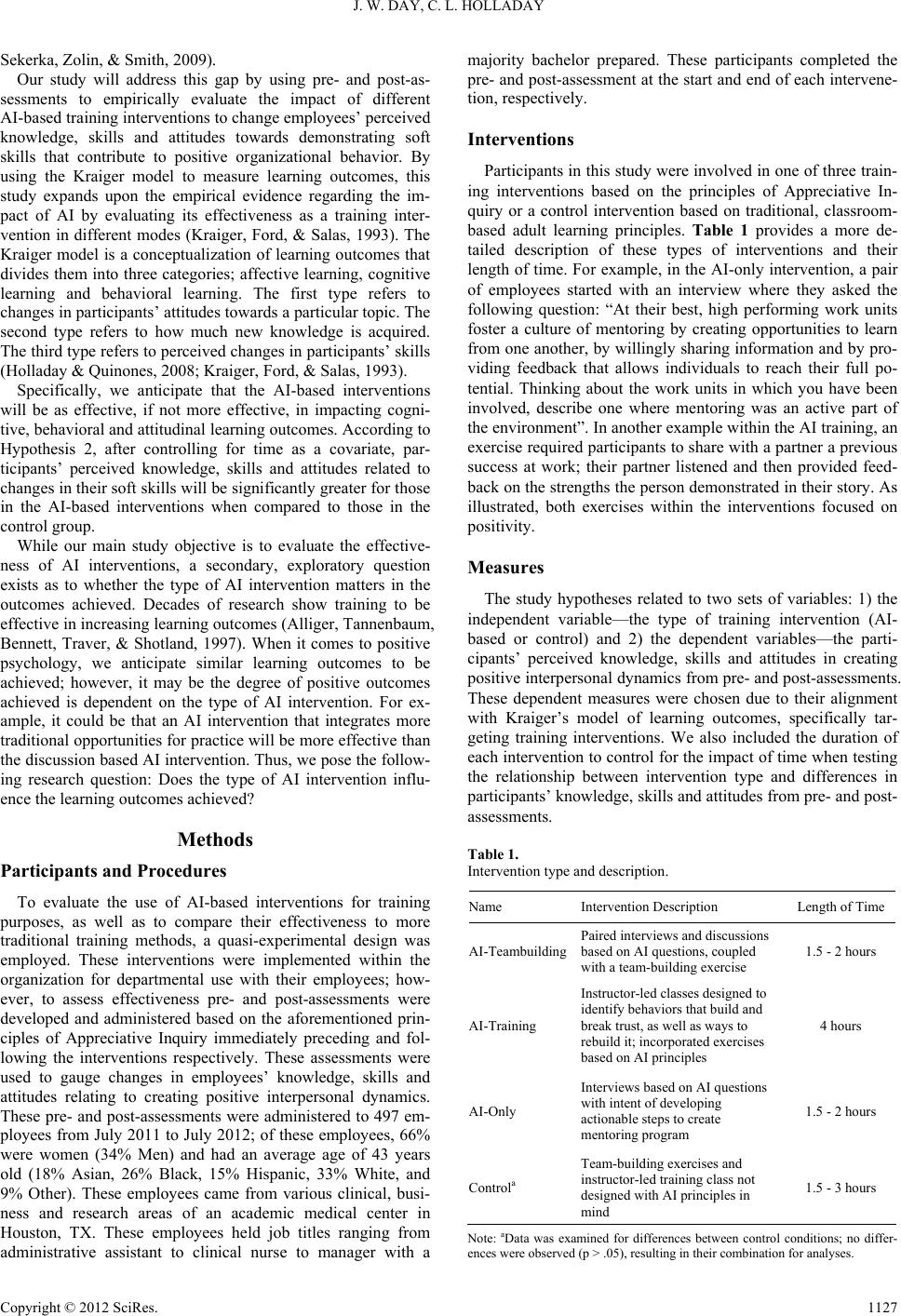 J. W. DAY, C. L. HOLLADAY Sekerka, Zolin, & Smith, 2009). Our study will address this gap by using pre- and post-as- sessments to empirically evaluate the impact of different AI-based training interventions to change employees’ perceived knowledge, skills and attitudes towards demonstrating soft skills that contribute to positive organizational behavior. By using the Kraiger model to measure learning outcomes, this study expands upon the empirical evidence regarding the im- pact of AI by evaluating its effectiveness as a training inter- vention in different modes (Kraiger, Ford, & Salas, 1993). The Kraiger model is a conceptualization of learning outcomes that divides them into three categories; affective learning, cognitive learning and behavioral learning. The first type refers to changes in participants’ attitudes towards a particular topic. The second type refers to how much new knowledge is acquired. The third type refers to perceived changes in participants’ skills (Holladay & Quinones, 2008; Kraiger, Ford, & Salas, 1993). Specifically, we anticipate that the AI-based interventions will be as effective, if not more effective, in impacting cogni- tive, behavioral and attitudinal learning outcomes. According to Hypothesis 2, after controlling for time as a covariate, par- ticipants’ perceived knowledge, skills and attitudes related to changes in their soft skills will be significantly greater for those in the AI-based interventions when compared to those in the control group. While our main study objective is to evaluate the effective- ness of AI interventions, a secondary, exploratory question exists as to whether the type of AI intervention matters in the outcomes achieved. Decades of research show training to be effective in increasing learning outcomes (Alliger, Tannenbaum, Bennett, Traver, & Shotland, 1997). When it comes to positive psychology, we anticipate similar learning outcomes to be achieved; however, it may be the degree of positive outcomes achieved is dependent on the type of AI intervention. For ex- ample, it could be that an AI intervention that integrates more traditional opportunities for practice will be more effective than the discussion based AI intervention. Thus, we pose the follow- ing research question: Does the type of AI intervention influ- ence the learning outcomes achieved? Methods Participants and Procedures To evaluate the use of AI-based interventions for training purposes, as well as to compare their effectiveness to more traditional training methods, a quasi-experimental design was employed. These interventions were implemented within the organization for departmental use with their employees; how- ever, to assess effectiveness pre- and post-assessments were developed and administered based on the aforementioned prin- ciples of Appreciative Inquiry immediately preceding and fol- lowing the interventions respectively. These assessments were used to gauge changes in employees’ knowledge, skills and attitudes relating to creating positive interpersonal dynamics. These pre- and post-assessments were administered to 497 em- ployees from July 2011 to July 2012; of these employees, 66% were women (34% Men) and had an average age of 43 years old (18% Asian, 26% Black, 15% Hispanic, 33% White, and 9% Other). These employees came from various clinical, busi- ness and research areas of an academic medical center in Houston, TX. These employees held job titles ranging from administrative assistant to clinical nurse to manager with a majority bachelor prepared. These participants completed the pre- and post-assessment at the start and end of each intervene- tion, respectively. Interventions Participants in this study were involved in one of three train- ing interventions based on the principles of Appreciative In- quiry or a control intervention based on traditional, classroom- based adult learning principles. Table 1 provides a more de- tailed description of these types of interventions and their length of time. For example, in the AI-only intervention, a pair of employees started with an interview where they asked the following question: “At their best, high performing work units foster a culture of mentoring by creating opportunities to learn from one another, by willingly sharing information and by pro- viding feedback that allows individuals to reach their full po- tential. Thinking about the work units in which you have been involved, describe one where mentoring was an active part of the environment”. In another example within the AI training, an exercise required participants to share with a partner a previous success at work; their partner listened and then provided feed- back on the strengths the person demonstrated in their story. As illustrated, both exercises within the interventions focused on positivity. Measures The study hypotheses related to two sets of variables: 1) the independent variable—the type of training intervention (AI- based or control) and 2) the dependent variables—the parti- cipants’ perceived knowledge, skills and attitudes in creating positive interpersonal dynamics from pre- and post-assessments. These dependent measures were chosen due to their alignment with Kraiger’s model of learning outcomes, specifically tar- geting training interventions. We also included the duration of each intervention to control for the impact of time when testing the relationship between intervention type and differences in participants’ knowledge, skills and attitudes from pre- and post- assessments. Table 1. Intervention type and description. Name Intervention Description Length of Time AI-Teambuilding Paired interviews and discussions based on AI questions, coupled with a team-building exercise 1.5 - 2 hours AI-Training Instructor-led classes designed to identify behaviors that build and break trust, as well as ways to rebuild it; incorporated exercises based on AI principles 4 hours AI-Only Interviews based on AI questions with intent of developing actionable steps to create mentoring program 1.5 - 2 hours Controla Team-building exercises and instructor-led training class not designed with AI principles in mind 1.5 - 3 hours Note: aData was examined for differences between control conditions; no differ- ences were observed (p > .05), resulting in their combination for analyses. Copyright © 2012 SciRes. 1127
 J. W. DAY, C. L. HOLLADAY Copyright © 2012 SciRes. 1128 Knowledge. This section of both the pre- and post-assess- ments was comprised of five true/false questions based on the principles of AI that were objectives in each AI-based interven- tion. The items were scored 0 (Incorrect) and 1 (Correct), and then summed to create the measure. A sample item from this section was “Questions are more powerful than answers”. Skills. In this part of the pre- and post-assessments, partici- pants were asked to rate their perceived level of confidence from 0% - 100% on ten questions that gauged their ability to demonstrate specific skills related to positive interpersonal dynamics. A sample question from this section was “How con- fident are you in your ability to use past successes to generate new, innovative solutions?” The ten-item scale for both the pre-assessment (α = .94) and the post-assessment (α = .96) had an adequate level of internal consistency. Attitudes. Participants were asked to rate their attitude re- garding the ability to create positive interpersonal dynamics as a result of the intervention in which they participated. Parti- cipants responded to the five items of this section on a 5-point scale ranging from 1 (strongly disagree) to 5 (strongly agree). A sample item from this section was “This session will help me to foster an environment of trust in my team.” Both the pre- assessment (α = .96) and post-assessment (α = .96) scales for this measure had an adequate level of consistency. Results To evaluate Hypothesis 1, paired t-tests were conducted be- tween participants’ pre and post assessments to evaluate sig- nificant changes in perceived knowledge, skills and attitudes after participating in one of the intervention types. In support of Hypothesis 1, there were significant gains in participants’ per- ceived knowledge, skills and attitudes from pre- and post- scores for each AI-intervention (see Table 2). For example, the AI-Only intervention showed that participants significantly increased their knowledge following the training (M = 2.73, SD = .68) as compared to before the training (M = 2.38, SD = .89; t = 3.97, p < .01). Partial support was found for Hypothesis 2. That is, in the control group, there was no significant difference in partici- pants’ pre- (M = 2.65, SD = 0.79) and post-knowledge scores (M = 2.78, SD = 0.84; t = 1.60, p > .05) of perceived know- ledge, though there was a significant difference for the AI inter- ventions. However, the AI interventions did not show signifi- cantly greater gains than the control intervention for skills and attitudes. To evaluate the research question, a MANCOVA was per- formed with the length of time for the three interventions as a covariate and changes in knowledge, skills and attitudes (mea- sured as difference scores from pre- and post-assessments) as the dependent variables. The research question then becomes whether the type of AI-based intervention differed in terms of effectiveness compared to each other after adjusting for time as a covariate. An overall effect was non-significant when exam- ining the effect of type of intervention (Wilks’ λ = .972, F (9, 1144.08) = .149, p >. 05). However, a significant main effect for type of intervention was observed for changes in participants’ skills from pre- to post-assessment, F (3, 173) = 3.287, p < .05. Significant pair- wise differences between the gains of participants’ skills were observed when compared by intervention type; namely, those who participated in the AI-Teambuilding and AI-Training in- terventions perceived significantly higher gains from pre- to post-assessment in their skills to build trust and collaboration when compared to those who participated in the AI-Only inter- vention. This finding suggests that participants perceived greater confidence to demonstrate positive interpersonal skills when the opportunity to practice was integrated into the inter- vention beyond the prescribed AI focused discussion. Discussion Proponents of positive psychology have advocated for its ex- ploration within organizations. One particular intervention, AI, relies on the tenants of positive psychology to help individuals within organizations achieve outcomes affecting the organi- Table 2. Descriptive statistics and paired t-test results. Pre-Test Post-Test Variable Intervention N Mean SD Mean SD t AI-Only 105 2.38 0.89 2.73 0.68 3.97* AI-Training 150 2.69 0.88 2.94 0.80 3.55* AI-Teambuilding 140 2.57 0.98 2.85 0.83 3.28* Knowledge Control 102 2.65 0.79 2.78 0.84 1.60 AI-Only 105 85.76 12.62 89.44 11.25 7.11* AI-Training 150 87.60 9.81 93.56 7.49 9.83* AI-Teambuilding 140 83.89 12.33 90.62 9.80 9.59* Skills Control 102 83.53 13.39 89.14 12.90 7.23* AI-Only 105 4.02 0.69 4.22 0.71 4.20* AI-Training 150 4.31 0.62 4.57 0.59 5.35* AI-Teambuilding 140 3.94 0.70 4.23 0.64 5.37* Attitudes Control 102 4.14 0.70 4.40 0.82 4.48* Note: *p < .01.
 J. W. DAY, C. L. HOLLADAY zation’s success. Such an intervention moves beyond traditional organizational interventions that focus on finding problems in processes and pointing to performance issues by instead recog- nizing positive performance and accomplishments. This redi- rected focus assumes that subsequent learning outcomes can be similar if not greater when considering successes. The present study demonstrated that AI-based interventions can in fact achieve attitudinal, behavioral, and cognitive learn- ing similar to traditional trainings. Further, the present study showed that AI interventions integrating a skills based applica- tion achieved greater learning than an AI discussion based in- tervention. This may have resulted from the possibilities par- ticipants were encouraged to explore as a way of practicing the skills taught during these interventions. In considering these possibilities, both hypothetical and actual in nature, participants were empowered to practice these skills before leaving the classroom. This likely increased participants’ perceptions that these skills were mastered. Similar findings from post-medical education suggest experiential learning enhances the skill de- velopment of participants over lecture-based learning because they can practice and refine them in a safe setting (Shreeve, 2008; Smits, de Buisonjé, Verbeek, van Dijk Metz, & ten Cate, 2003). Another focus of our study was to compare differences in learning outcomes between the AI-interventions and the control group. Our results only showed significant differences in the knowledge gained by those who participated in the AI-inter- ventions. In other words, participants learned more about AI because they participated in the AI-based interventions. These results are to be expected since the AI-interventions were de- signed based upon the principles of AI while the control group’s intervention was not. Participants in this study were not randomly assigned to the various interventions. However, previous studies exploring the effectiveness of AI indicated a need to empirically evaluate it under different circumstances, such as while being practiced over longer periods of time in controlled naturalistic settings (Bushe & Kassam, 2005; Sekera, Brumbaugh, Rosa, & Coo- perrider, 2006). While we cannot conclusively demonstrate the effectiveness of AI under the study’s current conditions, our findings suggest through our quasi-experimental design that AI-based interventions result in learning outcomes comparable to more conventional, problem-based training interventions, even when controlling for time. These results can serve as a springboard for future research to evaluate the effectiveness of AI under other study parameters. Limitations The present study did face unequal sample sizes across con- ditions that in turn may impact these results. Because the prob- ability of a Type 1 error increases with this imbalance, rela- tionships may have been falsely detected (Zimmerman, 2004). However, the consistency of the results across multiple mea- sures, time and interventions points to the unlikelihood of the findings being strictly due to this error. Another potential limitation is the generalizability of the re- sults from this exploratory study to different industries. While other studies have been conducted in healthcare settings to evaluate the use of AI (Moody, Horton-Deutsch, & Pesut, 2007; Richer, Ritchie, & Marchionni, 2009), to the authors’ know- ledge, no assessment has been developed based on these princi- ples nor has one been tested to evaluate the effectiveness of AI-based interventions for training purposes (Bushe & Kassam, 2005; Jones, 2010). Since this exploratory study was conducted in a healthcare organization, these results may not be character- istic of the types of gains participants in other industries could achieve if AI-based training interventions were used. However, these results provide some of the first quantifiable evidence about the effectiveness of AI as a training intervention. To ex- tend the generalizability of these results, similar studies will need to be conducted in other industries and in organizations of different sizes. Implications Past research on the successes and gains from using this methodology are based on anecdotes and stories, including subjective opinions and perceptions (Bushe, 2011). The lack of quantifiable, measurable data to support the effectiveness of AI is one of the major criticisms associated with this methodology. Consequently, some researchers have compared AI to a “man- agement fad”—an idea which gains popularity quickly without hard evidence of its effectiveness (Jones, 2010). The present study provides some evidence to the idea that AI-based interventions are as effective as conventional adult training methodologies in developing interpersonal skills for the workplace, providing support for the plethora of anecdotes highlighting its effectiveness. The structure of learning in the three AI-based interventions was similar to problem-based learning, an approach to adult training, in that actual experi- ences formed the basis of learning in small, interactive groups lead by a facilitator (Gijbels, Dochy, Van den Bossche, & Segers, 2005; Smits, de Buisonjé, Verbeek, van Dijk Metz, & ten Cate, 2003). Research from post-medical education has shown problem-based learning can be as effective, if not more, in comparison to lecture-based learning at fostering long-term learning and changes to performance, such as interpersonal skills (e.g., Lin, Lu, Chung, & Yang, 2010; Schmidt, van der Molen, & te Winkel, 2009; Shreeve, 2008; Smits, de Buisonjé, Verbeek, van Dijk Metz, & ten Cate, 2003). Our study expands upon this area of research by providing empirical support for the use of AI as an alternative approach to problem-based learning, albeit with a different focus; namely, evaluating past successes as a source for new solutions instead of focusing exclusively on problems. Conclusion Appreciative Inquiry is an approach focusing on past succe- sses to influence future success with the underlying assumption that a positive approach can lead to positive outcomes. The present study observed that AI interventions can achieve learn- ing outcomes similar to traditional training methods, suggesting its effectiveness from an empirical perspective. The benefit to relying on positivity in the intervention is that employees are likely to leave it energized, which could have downstream effects on the climate of the organization, whereby employees could see the organization as one where possibilities are explored rather than one where problems are scrutinized. Such positivity ultimately benefits the organization in its retention of those employees and changes implemented by those employees. Copyright © 2012 SciRes. 1129
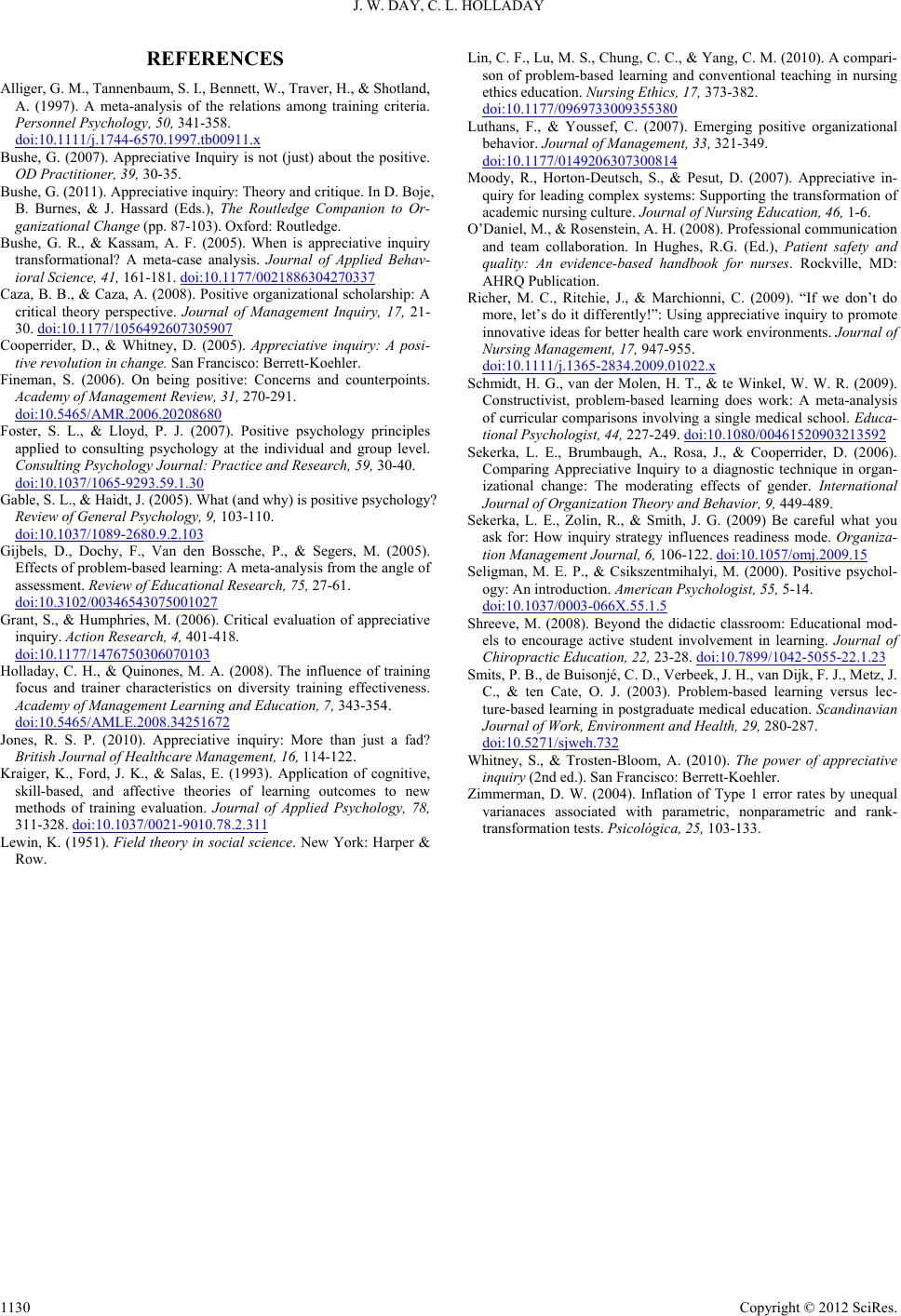 J. W. DAY, C. L. HOLLADAY REFERENCES Alliger, G. M., Tannenbaum, S. I., Bennett, W., Traver, H., & Shotland, A. (1997). A meta-analysis of the relations among training criteria. Personnel Psychology, 50, 341-358. doi:10.1111/j.1744-6570.1997.tb00911.x Bushe, G. (2007). Appreciative Inquiry is not (just) about the positive. OD Practitioner, 39, 30-35. Bushe, G. (2011). Appreciative inquiry: Theory and critique. In D. Boje, B. Burnes, & J. Hassard (Eds.), The Routledge Companion to Or- ganizational Change (pp. 87-103). Oxford: Routledge. Bushe, G. R., & Kassam, A. F. (2005). When is appreciative inquiry transformational? A meta-case analysis. Journal of Applied Behav- ioral Science, 41, 161-181. doi:10.1177/0021886304270337 Caza, B. B., & Caza, A. (2008). Positive organizational scholarship: A critical theory perspective. Journal of Management Inquiry, 17, 21- 30. doi:10.1177/1056492607305907 Cooperrider, D., & Whitney, D. (2005). Appreciative inquiry: A posi- tive revolution in change. San Francisco: Berrett-Koehler. Fineman, S. (2006). On being positive: Concerns and counterpoints. Academy of Management Review, 31, 270-291. doi:10.5465/AMR.2006.20208680 Foster, S. L., & Lloyd, P. J. (2007). Positive psychology principles applied to consulting psychology at the individual and group level. Consulting Psychology J ournal: Practice and Research, 59, 30-40. doi:10.1037/1065-9293.59.1.30 Gable, S. L., & Haidt, J. (2005). What (and why) is positive psychology? Review of General Psychology, 9, 103-110. doi:10.1037/1089-2680.9.2.103 Gijbels, D., Dochy, F., Van den Bossche, P., & Segers, M. (2005). Effects of problem-based learning: A meta-analysis from the angle of assessment. Review of Educational Research, 75, 27-61. doi:10.3102/00346543075001027 Grant, S., & Humphries, M. (2006). Critical evaluation of appreciative inquiry. Action Research, 4, 401-418. doi:10.1177/1476750306070103 Holladay, C. H., & Quinones, M. A. (2008). The influence of training focus and trainer characteristics on diversity training effectiveness. Academy of Management Le arn ing and Education, 7, 343-354. doi:10.5465/AMLE.2008.34251672 Jones, R. S. P. (2010). Appreciative inquiry: More than just a fad? British Journal of Healthcare Management, 16, 114-122. Kraiger, K., Ford, J. K., & Salas, E. (1993). Application of cognitive, skill-based, and affective theories of learning outcomes to new methods of training evaluation. Journal of Applied Psychology, 78, 311-328. doi:10.1037/0021-9010.78.2.311 Lewin, K. (1951). Field theory in social science. New York: Harper & Row. Lin, C. F., Lu, M. S., Chung, C. C., & Yang, C. M. (2010). A compari- son of problem-based learning and conventional teaching in nursing ethics education. Nursing Ethics, 17, 373-382. doi:10.1177/0969733009355380 Luthans, F., & Youssef, C. (2007). Emerging positive organizational behavior. Journal of Man age men t, 33, 321-349. doi:10.1177/0149206307300814 Moody, R., Horton-Deutsch, S., & Pesut, D. (2007). Appreciative in- quiry for leading complex systems: Supporting the transformation of academic nursing culture. Journal of Nursing Education, 46, 1-6. O’Daniel, M., & Rosenstein, A. H. (2008). Professional communication and team collaboration. In Hughes, R.G. (Ed.), Patient safety and quality: An evidence-based handbook for nurses. Rockville, MD: AHRQ Publication. Richer, M. C., Ritchie, J., & Marchionni, C. (2009). “If we don’t do more, let’s do it differently!”: Using appreciative inquiry to promote innovative ideas for better health care work environments. Journal of Nursing Management, 17, 947-955. doi:10.1111/j.1365-2834.2009.01022.x Schmidt, H. G., van der Molen, H. T., & te Winkel, W. W. R. (2009). Constructivist, problem-based learning does work: A meta-analysis of curricular comparisons involving a single medical school. Educa- tional Psychologist, 44, 227-249. doi:10.1080/00461520903213592 Sekerka, L. E., Brumbaugh, A., Rosa, J., & Cooperrider, D. (2006). Comparing Appreciative Inquiry to a diagnostic technique in organ- izational change: The moderating effects of gender. International Journal of Organization Theory and Behavior, 9, 449-489. Sekerka, L. E., Zolin, R., & Smith, J. G. (2009) Be careful what you ask for: How inquiry strategy influences readiness mode. Organiza- tion Management Journal, 6, 106-122. doi:10.1057/omj.2009.15 Seligman, M. E. P., & Csikszentmihalyi, M. (2000). Positive psychol- ogy: An introduction. American Psychologist, 55, 5-14. doi:10.1037/0003-066X.55.1.5 Shreeve, M. (2008). Beyond the didactic classroom: Educational mod- els to encourage active student involvement in learning. Journal of Chiropractic Education, 22, 23-28. doi:10.7899/1042-5055-22.1.23 Smits, P. B., de Buisonjé, C. D., Verbeek, J. H., van Dijk, F. J., Metz, J. C., & ten Cate, O. J. (2003). Problem-based learning versus lec- ture-based learning in postgraduate medical education. Scandinavian Journal of Work, Environment and Health, 29, 280-287. doi:10.5271/sjweh.732 Whitney, S., & Trosten-Bloom, A. (2010). The power of appreciative inquiry (2nd ed.). San Francisco: Berrett-Koehler. Zimmerman, D. W. (2004). Inflation of Type 1 error rates by unequal varianaces associated with parametric, nonparametric and rank- transformation tests. Psicológica, 25, 103-133. Copyright © 2012 SciRes. 1130
|