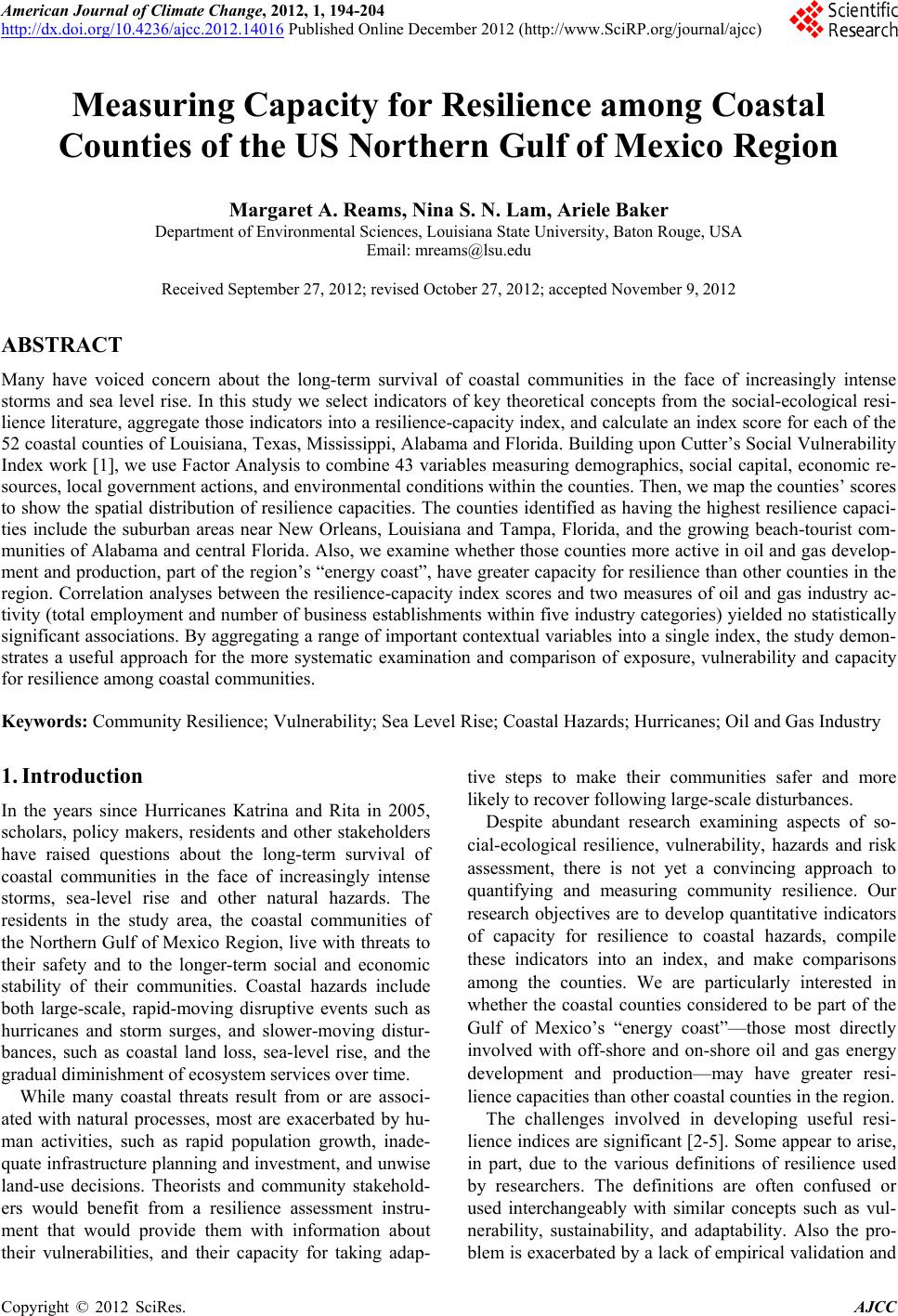 American Journal of Climate Change, 2012, 1, 194-204 http://dx.doi.org/10.4236/ajcc.2012.14016 Published Online December 2012 (http://www.SciRP.org/journal/ajcc) Measuring Capacity for Resilience among Coastal Counties of the US Northern Gulf of Mexico Region Margaret A. Reams, Nina S. N. Lam, Ariele Baker Department of Environmental Sciences, Louisiana State University, Baton Rouge, USA Email: mreams@lsu.edu Received September 27, 2012; revised October 27, 2012; accepted November 9, 2012 ABSTRACT Many have voiced concern about the long-term survival of coastal communities in the face of increasingly intense storms and sea level rise. In this study we select indicators of key theoretical concepts from the social-ecological resi- lience literature, aggregate those indicators into a resilience-capacity index, and calculate an index score for each of the 52 coastal counties of Louisiana, Texas, Mississippi, Alabama and Florida. Building upon Cutter’s Social Vulnerability Index work [1], we use Factor Analysis to combine 43 variables measuring demographics, social capital, economic re- sources, local government actions, and environmental conditions within the counties. Then, we map the counties’ scores to show the spatial distribution of resilience capacities. The counties identified as having the highest resilience capaci- ties include the suburban areas near New Orleans, Louisiana and Tampa, Florida, and the growing beach-tourist com- munities of Alabama and central Florida. Also, we examine whether those counties more active in oil and gas develop- ment and production, part of the region’s “energy coast”, have greater capacity for resilience than other counties in the region. Correlation analyses between the resilience-capacity index scores and two measures of oil and gas industry ac- tivity (total employment and number of business establishments within five industry categories) yielded no statistically significant associations. By aggregating a range of important contextual variables into a single index, the study demon- strates a useful approach for the more systematic examination and comparison of exposure, vulnerability and capacity for resilience among coastal communities. Keywords: Community Resilience; Vulnerability; Sea Level Rise; Coastal Hazards; Hurricanes; Oil and Gas Industry 1. Introduction In the years since Hurricanes Katrina and Rita in 2005, scholars, policy makers, residents and other stakeholders have raised questions about the long-term survival of coastal communities in the face of increasingly intense storms, sea-level rise and other natural hazards. The residents in the study area, the coastal communities of the Northern Gulf of Mexico Region, live with threats to their safety and to the longer-term social and economic stability of their communities. Coastal hazards include both large-scale, rapid-moving disruptive events such as hurricanes and storm surges, and slower-moving distur- bances, such as coastal land loss, sea-level rise, and the gradual diminishment of ecosystem services over time. While many coastal threats result from or are associ- ated with natural processes, most are exacerbated by hu- man activities, such as rapid population growth, inade- quate infrastructure planning and investment, and unwise land-use decisions. Theorists and community stakehold- ers would benefit from a resilience assessment instru- ment that would provide them with information about their vulnerabilities, and their capacity for taking adap- tive steps to make their communities safer and more likely to recover following large-scale disturbances. Despite abundant research examining aspects of so- cial-ecological resilience, vulnerability, hazards and risk assessment, there is not yet a convincing approach to quantifying and measuring community resilience. Our research objectives are to develop quantitative indicators of capacity for resilience to coastal hazards, compile these indicators into an index, and make comparisons among the counties. We are particularly interested in whether the coastal counties considered to be part of the Gulf of Mexico’s “energy coast”—those most directly involved with off-shore and on-shore oil and gas energy development and production—may have greater resi- lience capacities than other coastal counties in the region. The challenges involved in developing useful resi- lience indices are significant [2-5]. Some appear to arise, in part, due to the various definitions of resilience used by researchers. The definitions are often confused or used interchangeably with similar concepts such as vul- nerability, sustainability, and adaptability. Also the pro- blem is exacerbated by a lack of empirical validation and C opyright © 2012 SciRes. AJCC
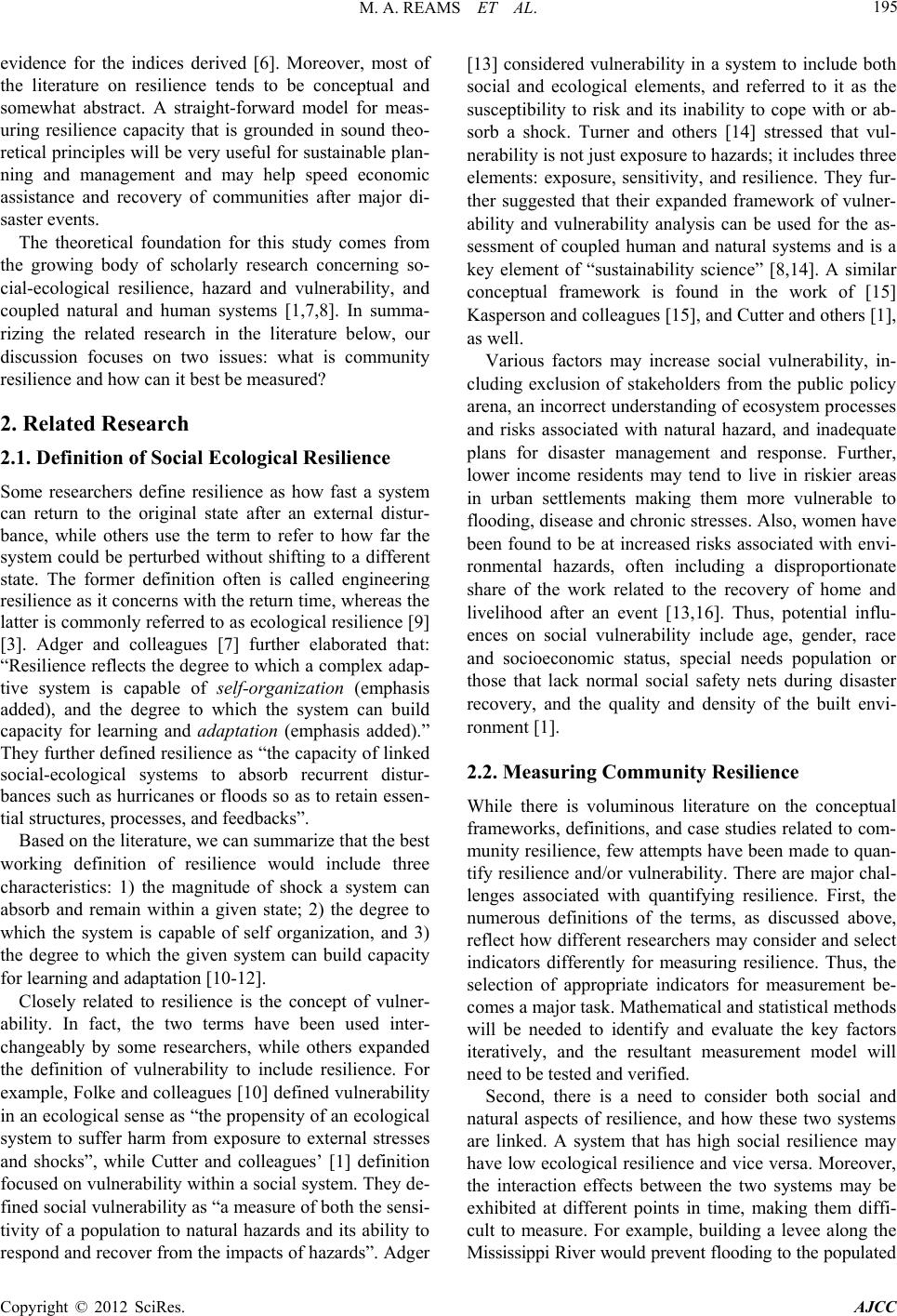 M. A. REAMS ET AL. 195 evidence for the indices derived [6]. Moreover, most of the literature on resilience tends to be conceptual and somewhat abstract. A straight-forward model for meas- uring resilience capacity that is grounded in sound theo- retical principles will be very useful for sustainable plan- ning and management and may help speed economic assistance and recovery of communities after major di- saster events. The theoretical foundation for this study comes from the growing body of scholarly research concerning so- cial-ecological resilience, hazard and vulnerability, and coupled natural and human systems [1,7,8]. In summa- rizing the related research in the literature below, our discussion focuses on two issues: what is community resilience and how can it best be measured? 2. Related Research 2.1. Definition of Social Ecological Resilience Some researchers define resilience as how fast a system can return to the original state after an external distur- bance, while others use the term to refer to how far the system could be perturbed without shifting to a different state. The former definition often is called engineering resilience as it concerns with the return time, whereas the latter is commonly referred to as ecological resilience [9] [3]. Adger and colleagues [7] further elaborated that: “Resilience reflects the degree to which a complex adap- tive system is capable of self-organization (emphasis added), and the degree to which the system can build capacity for learning and adaptation (emphasis added).” They further defined resilience as “the capacity of linked social-ecological systems to absorb recurrent distur- bances such as hurricanes or floods so as to retain essen- tial structures, processes, and feedbacks”. Based on the literature, we can summarize that the best working definition of resilience would include three characteristics: 1) the magnitude of shock a system can absorb and remain within a given state; 2) the degree to which the system is capable of self organization, and 3) the degree to which the given system can build capacity for learning and adaptation [10-12]. Closely related to resilience is the concept of vulner- ability. In fact, the two terms have been used inter- changeably by some researchers, while others expanded the definition of vulnerability to include resilience. For example, Folke and colleagues [10] defined vulnerability in an ecological sense as “the propensity of an ecological system to suffer harm from exposure to external stresses and shocks”, while Cutter and colleagues’ [1] definition focused on vulnerability within a social system. They de- fined social vulnerability as “a measure of both the sensi- tivity of a population to natural hazards and its ability to respond and recover from the impacts of hazards”. Adger [13] considered vulnerability in a system to include both social and ecological elements, and referred to it as the susceptibility to risk and its inability to cope with or ab- sorb a shock. Turner and others [14] stressed that vul- nerability is not just exposure to hazards; it includes three elements: exposure, sensitivity, and resilience. They fur- ther suggested that their expanded framework of vulner- ability and vulnerability analysis can be used for the as- sessment of coupled human and natural systems and is a key element of “sustainability science” [8,14]. A similar conceptual framework is found in the work of [15] Kasperson and colleagues [15], and Cutter and others [1], as well. Various factors may increase social vulnerability, in- cluding exclusion of stakeholders from the public policy arena, an incorrect understanding of ecosystem processes and risks associated with natural hazard, and inadequate plans for disaster management and response. Further, lower income residents may tend to live in riskier areas in urban settlements making them more vulnerable to flooding, disease and chronic stresses. Also, women have been found to be at increased risks associated with envi- ronmental hazards, often including a disproportionate share of the work related to the recovery of home and livelihood after an event [13,16]. Thus, potential influ- ences on social vulnerability include age, gender, race and socioeconomic status, special needs population or those that lack normal social safety nets during disaster recovery, and the quality and density of the built envi- ronment [1]. 2.2. Measuring Community Resilience While there is voluminous literature on the conceptual frameworks, definitions, and case studies related to com- munity resilience, few attempts have been made to quan- tify resilience and/or vulnerability. There are major chal- lenges associated with quantifying resilience. First, the numerous definitions of the terms, as discussed above, reflect how different researchers may consider and select indicators differently for measuring resilience. Thus, the selection of appropriate indicators for measurement be- comes a major task. Mathematical and statistical methods will be needed to identify and evaluate the key factors iteratively, and the resultant measurement model will need to be tested and verified. Second, there is a need to consider both social and natural aspects of resilience, and how these two systems are linked. A system that has high social resilience may have low ecological resilience and vice versa. Moreover, the interaction effects between the two systems may be exhibited at different points in time, making them diffi- cult to measure. For example, building a levee along the Mississippi River would prevent flooding to the populated Copyright © 2012 SciRes. AJCC
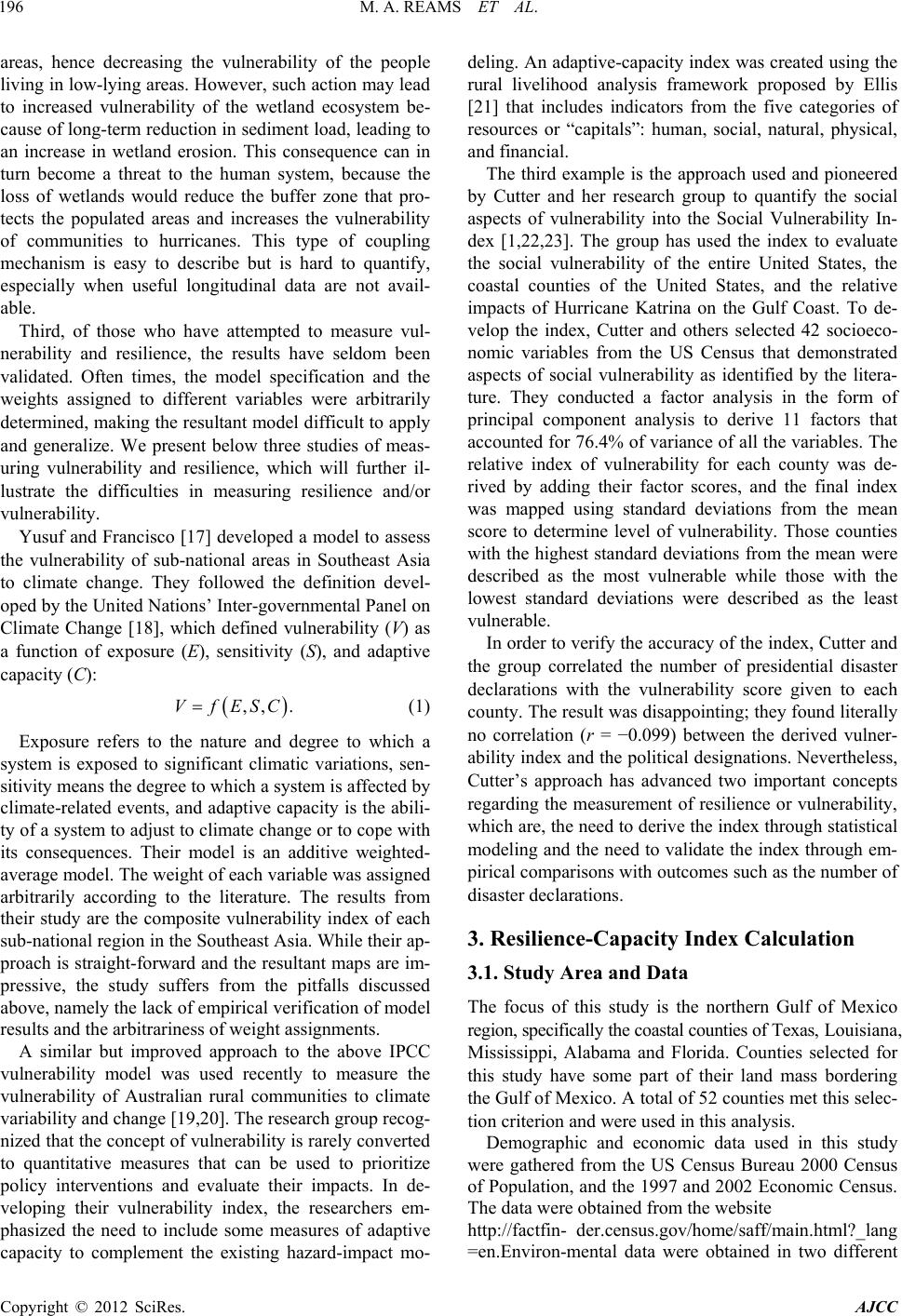 M. A. REAMS ET AL. 196 areas, hence decreasing the vulnerability of the people living in low-lying areas. However, such action may lead to increased vulnerability of the wetland ecosystem be- cause of long-term reduction in sediment load, leading to an increase in wetland erosion. This consequence can in turn become a threat to the human system, because the loss of wetlands would reduce the buffer zone that pro- tects the populated areas and increases the vulnerability of communities to hurricanes. This type of coupling mechanism is easy to describe but is hard to quantify, especially when useful longitudinal data are not avail- able. Third, of those who have attempted to measure vul- nerability and resilience, the results have seldom been validated. Often times, the model specification and the weights assigned to different variables were arbitrarily determined, making the resultant model difficult to apply and generalize. We present below three studies of meas- uring vulnerability and resilience, which will further il- lustrate the difficulties in measuring resilience and/or vulnerability. Yusuf and Francisco [17] developed a model to assess the vulnerability of sub-national areas in Southeast Asia to climate change. They followed the definition devel- oped by the United Nations’ Inter-governmental Panel on Climate Change [18], which defined vulnerability (V) as a function of exposure (E), sensitivity (S), and adaptive capacity (C): ,, .VfESC (1) Exposure refers to the nature and degree to which a system is exposed to significant climatic variations, sen- sitivity means the degree to which a system is affected by climate-related events, and adaptive capacity is the abili- ty of a system to adjust to climate change or to cope with its consequences. Their model is an additive weighted- average model. The weight of each variable was assigned arbitrarily according to the literature. The results from their study are the composite vulnerability index of each sub-national region in the Southeast Asia. While their ap- proach is straight-forward and the resultant maps are im- pressive, the study suffers from the pitfalls discussed above, namely the lack of empirical verification of model results and the arbitrariness of weight assignments. A similar but improved approach to the above IPCC vulnerability model was used recently to measure the vulnerability of Australian rural communities to climate variability and change [19,20]. The research group recog- nized that the concept of vulnerability is rarely converted to quantitative measures that can be used to prioritize policy interventions and evaluate their impacts. In de- veloping their vulnerability index, the researchers em- phasized the need to include some measures of adaptive capacity to complement the existing hazard-impact mo- deling. An adaptive-capacity index was created using the rural livelihood analysis framework proposed by Ellis [21] that includes indicators from the five categories of resources or “capitals”: human, social, natural, physical, and financial. The third example is the approach used and pioneered by Cutter and her research group to quantify the social aspects of vulnerability into the Social Vulnerability In- dex [1,22,23]. The group has used the index to evaluate the social vulnerability of the entire United States, the coastal counties of the United States, and the relative impacts of Hurricane Katrina on the Gulf Coast. To de- velop the index, Cutter and others selected 42 socioeco- nomic variables from the US Census that demonstrated aspects of social vulnerability as identified by the litera- ture. They conducted a factor analysis in the form of principal component analysis to derive 11 factors that accounted for 76.4% of variance of all the variables. The relative index of vulnerability for each county was de- rived by adding their factor scores, and the final index was mapped using standard deviations from the mean score to determine level of vulnerability. Those counties with the highest standard deviations from the mean were described as the most vulnerable while those with the lowest standard deviations were described as the least vulnerable. In order to verify the accuracy of the index, Cutter and the group correlated the number of presidential disaster declarations with the vulnerability score given to each county. The result was disappointing; they found literally no correlation (r = −0.099) between the derived vulner- ability index and the political designations. Nevertheless, Cutter’s approach has advanced two important concepts regarding the measurement of resilience or vulnerability, which are, the need to derive the index through statistical modeling and the need to validate the index through em- pirical comparisons with outcomes such as the number of disaster declarations. 3. Resilience-Capacity Index Calculation 3.1. Study Area and Data The focus of this study is the northern Gulf of Mexico region, specifically the coastal counties of Texas, Louisiana, Mississippi, Alabama and Florida. Counties selected for this study have some part of their land mass bordering the Gulf of Mexico. A total of 52 counties met this selec- tion criterion and were used in this analysis. Demographic and economic data used in this study were gathered from the US Census Bureau 2000 Census of Population, and the 1997 and 2002 Economic Census. The data were obtained from the website http://factfin- der.census.gov/home/saff/main.html?_lang =en.Environ-mental data were obtained in two different Copyright © 2012 SciRes. AJCC
 M. A. REAMS ET AL. 197 ways that will be discussed in more detail in the next section. Toxic Release Inventory (TRI) data from 2000 were obtained from the US Environmental Protection Agency from the website: http://iaspub.epa.gov/triexplor- er/tri_release.facility. Digital elevation measures were available through the USGS seamless map server at the website http://seamless.usgs.gov. 3.2. The Factor Analysis Method As introduced above, the social aspects of vulnerability were first quantified by Cutter and colleagues [1]. They developed the Social Vulnerability Index by selecting 42 socioeconomic indicators of social vulnerability and con- ducting a factor analysis in the form of principal compo- nent analysis (PCA) to create an index of these variables to measure social vulnerability. There are a few ways in which Cutter’s method could be improved. First the factor analysis method might be changed. Instead of using a principal component analysis to create the factors a principal axis factoring method could be used. A principal component analysis seeks to explain all the common and unique variance of the vari- ables, while a principal axis factoring method seeks only to explain the common variances. Secondly, a principal component analysis is a variance-based approach while principal axis factoring is a correlation-focused approach. This means that in a principal axis factoring method every variable is included in the analysis, yet not every variable is deemed important. In other words, a principal- axis factoring method acts as a filter while a principal component analysis includes all the variables [24]. Secondly, factor scores are the sum of positive and negative values of variables around an axis for a case. They are in themselves an index of the relationship of indicators to each other. Therefore, to create an index of factor scores is to include all variables into the index, and create an index of an index. This can be impractical and hard to manage. As an alternative, could the factor ana- lysis provide a methodology to discern which variables aremost important to each dimension or factor instead of using factor scores? Thirdly, Cutter et al. [1] made no a priori assumptions about importance. They used an additive model that did not weight the variance explained by each factor. Each factor explains a percent of the variance (i.e. eigenvalue) within the data matrix and this varies based on the rela- tionship of the variables to each other within each factor. Therefore each factor should be weighted to its relative importance, and this is statistically determined when the factors are calculated. 3.3 A Modified Method to Create the Resilience Index The methods used by Cutter et al. [1] to create the Social Vulnerability Index were modified to create an index of community capacity for resilience. Socioeconomic vari- ables were obtained from the 2000 Census, 36 of these variables were taken from the research of Cutter and col- leagues and 7 new variables were added that measured additional aspects of vulnerability and resilience, includ- ing voting rates among residents, economic resources of local government, and environmental factors. All vari- ables used in this analysis are shown in Table 1. The variables taken from Cutter et al. [1] were se- lected because they measure generally accepted aspects of social vulnerability. These concepts include: limited access to economic resources and political power, fewer social networks, less structurally sound housing, and more physically limited individuals. Specific variables that identify these measures of vulnerability are age, gender, race and socioeconomic status. Other measures of the social capital of a community include housing type and abundance, rental properties and housing values. Measures of the economic conditions of the area include commercial development, manufacturing density, earn- ing density, and primary employments in an area. Sup- plemental Security Income (SSI) recipients were added as an additional measure of economic vulnerability. We added variables concerning voting rates among residents and local government spending, as well, be- cause these factors may affect a community’s ability to adapt and/or mitigate the damages associated with coa- stal disturbances. Additional variables that measure en- vironmental attributes and conditions were added be- cause they relate to the residents’ exposure to hazards. These variables included Toxic Release Inventory (TRI) values and the mean elevation of the county. The TRI reflects the estimated discharges of TRI-listed chemicals within each county and were included because they offer insight into local environmental conditions. Elevation was used because it indicates susceptibility to flooding, a relevant consideration in coastal, hurricane-prone areas. The Toxic Release Inventory (TRI) data was obtained from the EPA website using the TRI Explorer tool. Re- lease reports were selected for 2000. The data was se- lected by county, and total on site or offsite disposal or other releases with chemical name was used to obtain a measure of toxic pollution per county for all chemicals across all industries. These numbers were listed in of pounds. Data in the year 2000 ranged from: 0 releases of any chemical in Kleberg, TX, to 55,247,688 lbs in Es- cambia County, Florida. The median value of TRI re- lease in the Gulf of Mexico region in the year 2000 was 283,910 lbs of Toxic releases. The variable “mean elevation” was obtained through a multistep process. First, data was downloaded from the USGS Seamless Map Server Program in a Digital Eleva- tion Model format (DEM) for all the coastal counties Copyright © 2012 SciRes. AJCC
 M. A. REAMS ET AL. 198 Table 1. Variables used to construct index. Demographics PCTBLACK Percent African American PCTINDIAN Percent Indian PCTASIAN Percent Asian PCTKIDS Percent of population under 5years of age PCTOLD Percent of population over 65 PCTFEM Percent of population that is female PCTHISPANIC Percent Hispanic MEDAGE Median age AVGPERHH Average number of people per families BRATE Birth rate Social Capital PCTF_HH % female headed household CTRFRM % rural farm population PCTMOBL % housing units that are mobile homes PCTRENTER % housing units that are renter occupied PCTNOHS % over 25 with no high school diploma FEMLBR % civilian labor force that is female PCTVLUM % civilian labor force that is unemployed TOTCVLBF % of population participating in the labor force PCTPOV % of population below the poverty level HOSPCT03 Hospitals per capita, 2003 NRRESPC Number of nursing home residents per capita HOUDENUT Housing density per square mile Economics MVALOO Median value of owner occupied housing MEDINCOME Median income RPROPDEN Total value of farm products sold per sq. mile EARNDEN Earnings ($1000) of all establishments per sq. mile AGRIPC % employed in primary extractive industries TRANPC % employed in transportation, communications, public utilities SERVPC % employed in service occupations PCTHH75 % of households earning over $75,000 per year SSBENPC Per capita social security recipients MEDRENT Median rent MAESDEN Number manufacturing establishments per square mile PCTFARM % farm land as a percent of total land SSIREC % population receives supplemental security Insurance benefits COMDEVDN Number of commercial establishments per square mile, Government EXPED Local expenditures for education PERVOTE % of population that voted in the 1992 presidential election LGFREVPERCAP Local government finance, revenue per capita PROPTACPC Property tax, per capita GENEXPPC Direct general expenditures per capita, Environmental MELE County mean elevation above sea level TRI lbs of toxic release per county bordering the Gulf of Mexico, and the entire state of Louisiana in a NED 1 arc second data format.This data was added to a GIS using ARCMap 9.2 as a layer file and then exported into a raster file. Once in a raster file format this data was able to be combined into one seam- less digital elevation data set. This procedure was fol- lowed for each coastal state. Once the DEMs were seam- lessly processed they were added as a layer file to a GIS. Over this layer a coastal county shape file was overlayed. Coastal county data was obtained from the Census Bu- reau: Counties 2000 shapefile option for all coastal sta- tes. Then using the Spatial Analyst Tool, digital elevation for each county was calculated. Mean elevation for each county was selected. Mean elevation data ranged from 0 feet above sea level in Orleans Parish the lowest county in the Gulf of Mexico region to 34.3 feet above sea level in Mobile County, Alabama. All variables were norma- lized by conversion into densities per square mile, per capita, or percents. Table 1 summarizes the variables used to construct the resilience-capacity scores for each county. The purpose of the analysis is to aggregate key vari- ables to create a descriptive measure of the relative ca- pacity for resilience among the counties. The 43 vari- ables were placed in a Principal Factor Analysis using the Varimax rotation option and six factors explaining 69% of the variance were derived. From each of these 6 factors the variable that had the highest loading was se- lected. The rotated factor matrix is shown in Table 2. Rotation Method: Principal Axis Factoring Varimax with Kaiser; rotation converged in 34 iterations The first 6 factors identified by the Factor Analysis were retained for further analysis because together these factors account for almost 70% of the variance in the data set. The highest loading variable on each of the first 6 factors was selected to be included in the calculation of the resilience capacity index. For example, the variable EXPED (Expenditures for Education) is the highest load- ing variable on the first factor. The weight given to the variable EXPED in the aggregation of the resilience- capacity index will be the eigenvalue (rescaled) of the first factor. The eigenvalue conveys the percent of vari- ance in the data set that is explained or accounted for by the variables that load onto that factor. To calculate the weights to be assigned to each of these 6 variables, we rescaled the 69% total variance accounted for by the six factors to equal 100% of the total explained by these variables. Table 3 contains a list of these 6 variables, the original eigenvalue of each factor, and the rescaled variance which serves as the weights for the aggregation of the 6 variables. Each of the six variables and their rescaled variances Copyright © 2012 SciRes. AJCC
 M. A. REAMS ET AL. 199 Table 2. Rotated factor matrix results. Rotation method: principal axis factoring varimax with kaiser; rotation con- verged in 34 iterations. 1 2 3 4 5 6 PCTBLA 0.095 ‒0.188 0.86 0.053 ‒0.005 0.036 PCTKIDS 0.045 ‒0.005 ‒0.132 0.033 0.896 ‒0.064 PCTOLD ‒0.074 ‒0.474 0.116 ‒0.6 ‒0.275 0.313 PCTHISP 0.37 0.285 ‒0.253 0.063 0.12 ‒0.333 MEDAGE ‒0.171 ‒0.133 ‒0.16 ‒0.153 ‒0.588 0.336 AVGPER ‒0.25 0.218 0.096 0.782 0.2240.147 FARM ‒0.628 ‒0.327 ‒0.092 ‒0.304 ‒0.387 0.084 PCTMOB ‒0.71 ‒0.145 ‒0.288 ‒0.053 ‒0.177 0.188 PCTREN 0.691 ‒0.052 0.346 ‒0.174 0.262 ‒0.449 PCTNOH 0.706 0.344 0.118 ‒0.156 ‒0.035 0.023 FEMLBR 0.303 0.021 0.793 ‒0.011 ‒0.0280.02 TOTCVL 0.497 0.569 ‒0.379 ‒0.03 0.231 0.198 PCTPOV ‒0.09 ‒0.699 0.603 ‒0.213 0.2180.106 MVALO 0.363 0.639 ‒0.185 0.489 ‒0.045 0.031 MEDREN 0.599 0.54 ‒0.364 0.262 0.04 ‒0.096 BLDPER 0.775 0.308 ‒0.053 0.166 0.0560.058 BRATE 0.112 0.015 0.137 0.04 0.8560.013 RPROPD ‒0.196 ‒0.147 0.362 ‒0.006 0.1580.304 AG ‒0.206 ‒0.753 ‒0.084 0.145 ‒0.052 0.093 TRAN 0.337 ‒0.076 ‒0.292 0.467 ‒0.075 0.086 VOTE92 ‒0.066 0.045 0.067 0.186 ‒0.074 0.853 GENEXP 0.072 0.053 0.091 0.477 ‒0.123 0.006 HOUDEN 0.83 0.031 0.088 0.093 ‒0.058 ‒0.064 MEDINC 0.198 0.745 ‒0.37 0.461 ‒0.059 0.024 EXPED 0.902 0.246 ‒0.02 0.045 0.033‒0.048 MELE ‒0.177 0.002 0.118 ‒0.675 ‒0.226 ‒0.13 TRI 0.294 0.415 0.009 0.208 0.0930.11 were then placed in a weighted-average model to derive the resilience capacity score for each county. The formula used was: minmax min 6 1 , I i ii i VXX XX V (2) The normalized raw data of the variable X was scaled from 0 to 1. This was renamed V, where V = normalized variable. V was then multiplied by the rescaled variance Table 3. Variables and eigenvalues used to construct weighted community resilience-capacity index. Variable Name Relation to Resilience Capacity % Original Variance Explained by Factor Rescaled Variance Expenditures for educationpositive 20.13 29.18 Median income of the parishpositive 13.53 19.61 Percent of the workforce that is female positive 10.4 15.08 Mean elevation of the parish positive 10.2 14.79 Percent of the population below 5 years old positive 9.1 13.1 Percent of the population that voted in the last presidential election positive 5.7 8.26 to create a weighted value for that variable per county. These new, weighted values were then summed to give an index value that ranged from 0 - 1. Thus, the resi- lience index had a possible range of 0 to 1, where 0 was the lowest resilience capacity, while 1 was the highest. The resilience-capacity index values for all counties are shown below in Table 4. The weighted index values for the Gulf of Mexico re- gion had a low value of 0.35 in Willacy County, TX, and seven counties had the highest possible value of 1. These counties were: Jefferson Parish, LA, Kenedy County, TX, Okaloosa County, FL, Hernando County, FL, Sarasota County, FL, Pinellas County, FL, and Hillsboro County, FL. The results of the index were mapped using a natural breaks method to visually demonstrate patterns of resi- lience capacity across the Gulf of Mexico region. Figure 1 depicts the results of the analysis for Texas and Louisiana while Figure 2 shows results for Mississippi, Alabama, and Florida. 4. Discussion The counties with the lowest resilience levels according to the weighted resilience index derived through the Factor Analysis (FA) were: Willacy County, TX, Cam- eron, TX, Kleberg, TX, Calhoun, TX, and Dixie, FL. With the exception of Calhoun, TX all these counties had median incomes below $30,000 per year. They also had relatively low voter turnout in the 1992 presidential elec- tion that ranged from 18% in Cameron, TX to 33% in Dixie, FL. Typically they had a higher percentage of the population under the age of 5 years. The percentage of Copyright © 2012 SciRes. AJCC
 M. A. REAMS ET AL. 200 Table 4. Resilience-capacity index scores for Gulf of Mexico coastal counties. County Index Score County Index Score Hillsboro, FL 1.00 Cameron, LA 0.75 Pinellas, FL 1.00 Chambers, TX 0.75 Sarasota, FL 1.00 Jefferson, FL 0.75 Hernando, FL 1.00 Jackson, MS 0.75 Okaloosa, FL 1.00 Lafourche, LA 0.74 Kenedy, TX 1.00 Nueces, TX 0.74 Jefferson, LA 1.00 Vermilion, LA 0.74 Santa Rosa, FL 0.95 Wakulla, FL 0.73 Manatee, FL 0.95 St. Mary, LA 0.73 Citrus, FL 0.95 Jefferson, TX 0.72 Charlotte, FL 0.94 Franklin, FL 0.72 Lee, FL 0.93 Hancock, MS 0.69 Walton, FL 0.92 Levy, FL 0.66 Pasco, FL 0.91 Terrebonne, LA 0.65 Escambia, FL 0.90 Harrison, MS 0.65 Baldwin, AL 0.90 Orange, TX 0.64 Mobile, AL 0.87 Taylor, FL 0.62 Bay, FL 0.85 San Patricio, TX 0.58 Gulf, FL 0.84 Aransas, TX 0.58 Galveston, TX 0.84 Matagorda, TX 0.57 Orleans, LA 0.84 Dixie, FL 0.55 St. Bernard, LA 0.82 Calhoun, FL 0.55 Monroe, FL 0.82 Kleberg, TX 0.52 Collier, FL 0.80 Cameron, TX 0.40 Iberia, LA 0.79 Willacy, TX 0.35 Plaquemines, LA 0.77 Brazoria, TX 0.77 children in the population of these counties ranged from 8.2% in Willacy, TX to 5.9% in Dixie, FL. Those counties with the highest resilience capacity scores include the suburban areas of New Orleans, and Tampa, and the growing beach communities in Alabama and central Florida. Surprisingly, Kenedy, TX also is calculated to be among the counties with high capacities for resilience. These counties all had a high percentage of women in the workforce (above 47%) and high voter turnout. Kenedy, TX had the highest voter turnout in the gulf region with 55%, and other counties that exhibited high resilience had above 40% voter turnout. In our analysis expenditures for education were wei- ghted at 29%. Areas with high expenditures for education were estimated to have greater capacity for resilience. These areas included the urban communities of Orleans, LA, Hillsborough, FL and Pinellas, FL. Hillsborough and Pinellas both received a score on the resilience capacity index of 1 or highest resilience capacity, while Orleans received a score of 0.84, placing it in the mid range of resilience capacity. The next important variable was median income. This was given a weight of 19.6%, while percent of the labor force that was female and mean elevation of the county were both weighted at 15%. The final two variables were percent of the population under 5 years old which was weighted at 13% and percent of the population that voted in the last presidential election was weighted at 8.2%. Affluence and education account for roughly 50% of resilience capacity. However, a combination of the other factors can place a county in the higher resilience capacity category. For example, Kenedy, TX, has the lowest expenditures for education in the region, a very low median income, a middle elevation, and the highest value of voter partici- pation. Given the weighting method a high level of voter participation is enough to push a county into a higher level of estimated resilience capacity, despite the pre- sence of other factors that would suggest socioeconomic vulnerability. 5. Resilience Capacity, Oil and Gas Activity Oil and gas activity can affect social-ecological resilience in a number of ways. For example, the oil and gas Indus- try provided more than $800 million in revenue for Lou- isiana in 2000 [25]. The economic impacts of oil and gas industry activities can lead to a higher occurrence of af- fluence, a variable that theoretically strengthens social resilience and recovery from major disturbances [26]. On the other hand, oil and gas exploration and production is suspected to be associated with wetland loss via erosion and subsidence as well as other forms of environmental degradation, problems that theoretically weaken ecolo- gical resilience [27]. Are social-ecological resilience and oil and gas acti- vity associated in any way? Specifically, do higher levels of employment within the oil and gas industry help make a community more resilient to large hurricanes and sea level rise? To address these questions, it is useful to consider pat- Copyright © 2012 SciRes. AJCC
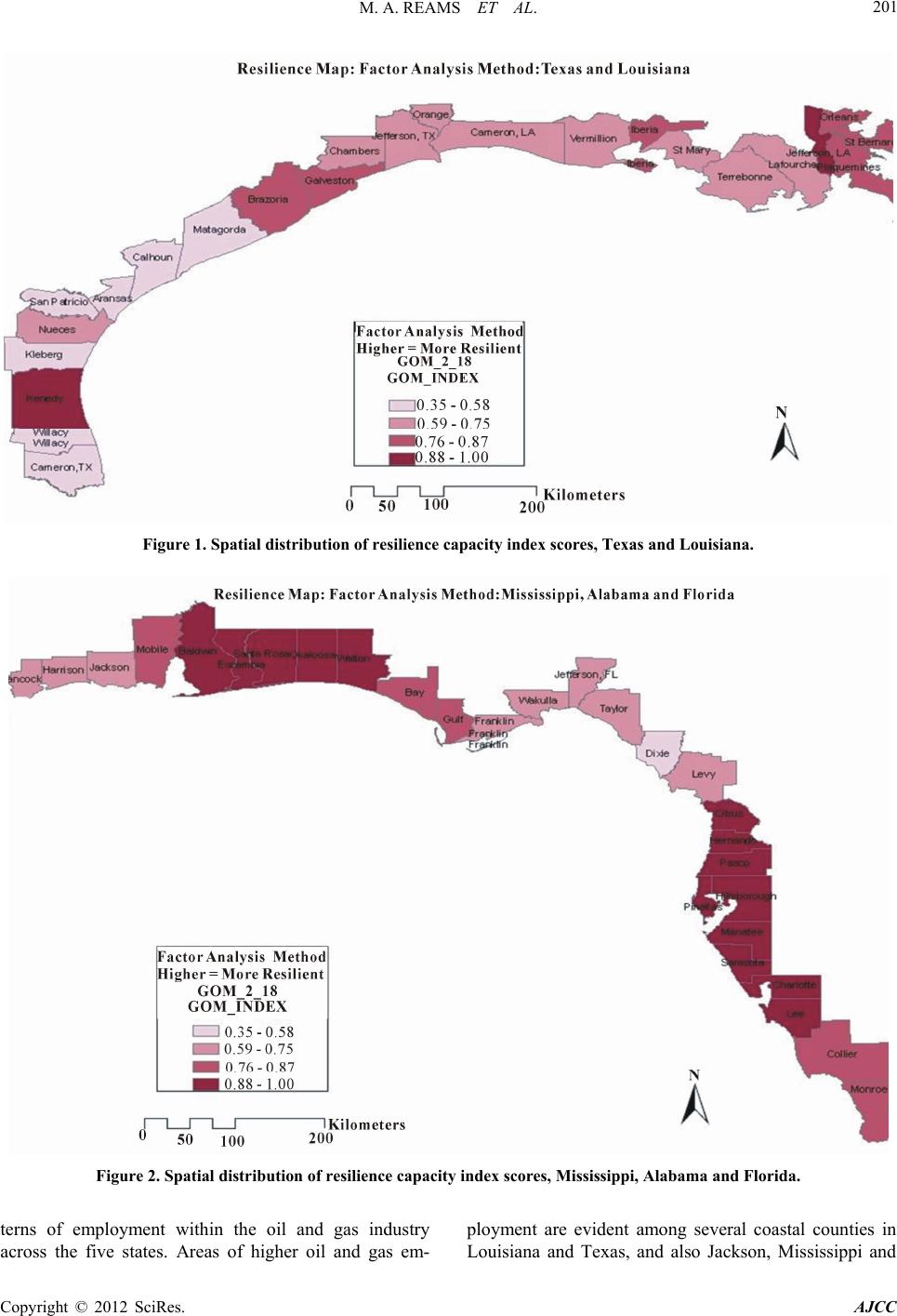 M. A. REAMS ET AL. Copyright © 2012 SciRes. AJCC 201 Figure 1. Spatial distribution of resilience capacity index scores, Texas and Louisiana. Figure 2. Spatial distribution of resilience capacity index scores, Mississippi, Alabama and Florida. terns of employment within the oil and gas industry across the five states. Areas of higher oil and gas em- ployment are evident among several coastal counties in Louisiana and Texas, and also Jackson, Mississippi and
 M. A. REAMS ET AL. 202 Mobile, Alabama. To determine whether there are statis- tically significant associations between the presence of the oil and gas industry and the county-level resilience scores, we conducted Pearson Correlation Analyses be- tween variables measuring industry activity and the re- silience capacity scores for each of the coastal counties in the study area. The Pearson r Correlation Coefficient ran- ges from 0 to 1, with values closer to 1 indicating a stronger association between two variables. The direc- tional sign of the Pearson r indicates whether the associa- tion is inverse or positive. Also, the p value determines whether an observed association is statistically signifi- cant, with a smaller value (less than 0.10) signaling a more significant association [28]. We used 2008 employment data from the WholeData information service, purchased and made available for this study by the LSU Center for Energy Studies. WholeData estimated employment figures from County Business Patterns (CBP) data, using iterative estimation techniques to fill in the gaps where data may have been missing in the original CBP data. The employment data reflects five oil and gas employment categories: NAICS codes 211 (Oil and Gas Extraction), 213111 (Drilling Oil and Gas Wells), 213112 (Support Activities for Oil and Gas), 333132 (Oil and Gas Field Machinery Manufac- turing), and 541360 (Geophysical Surveying). We used the sum of employment and establishments for these 5 codes for each county to create two measures of oil and gas industry presence. The two counties with the most oil and gas industry employees are Louisiana’s Terrebonne Parish with 6078 employees, and Nueces County in Texas, with 3420 workers. These counties are followed by several parishes (counties) in Louisiana: St. Mary Parish (2993), Jefferson Parish (2540), Orleans Parish (1866), Lafourche Parish (1491), Iberia Parish (1395), Plaquemine Parish (941); and two in Texas, Brazoria County (751) and Jefferson County (517). We ran bi-variate correlation analyses between the two measures of oil and gas industry activity (total employ- ment of the five NAICS categories and number of estab- lishments) and the resilience capacity scores for the coas- tal counties within the study area. The analysis examin- ing the resilience scores for the 52 counties and the oil and gas activity variables resulted in no statistically sig- nificant correlations between resilience capacity scores and industry activity. The resilience-capacity index scores and total oil and gas industry employment yielded a Pearson r coefficient of −0.59, p = 0.680. Similarly, the resilience-capacity index scores and the number of oil and gas business establishments within a county resulted in a Pearson r of −0.050 and a p value of 0.756. 6. Conclusions The study built upon methodology developed by Cutter and colleagues in their earlier construction of the Social Vulnerability Index. We included several new variables drawn from the social-ecological resilience literature that indicate the capacity for adaptability or resilience. These include measures of the financial resources and public investment patterns of local governments, land elevation, and citizen involvement in public affairs. By combining these indicators with measures of social vulnerability, our resilience-capacity index yields useful information about the relative vulnerabilities and adaptive resources of these communities. Counties with higher capacities for resilience include the suburban areas of New Orleans and Tampa, and the rapidly growing beach tourist communi- ties of Alabama and Florida. Counties that scored higher tended to invest more in education, have higher per capi- ta incomes, more women in the workforce, higher mean land elevation, more children and higher rates of voter participation. The Pearson Correlation Analyses yielded no evidence of statistically significant associations between the cal- culated resilience-capacity scores and measures of oil and gas industry activity within the coastal counties. The communities located in the area known as the “energy coast” of Louisiana and Texas, areas with higher em- ployment in the oil and gas industry, were not found to have greater capacity for resilience than other counties of the northern Gulf of Mexico. The calculation of the resilience-capacity index scores helps identify sources of community resilience to distur- bances such as hurricanes and sea level rise. As such, the analysis demonstrates a useful approach for the more systematic examination and comparison of factors related to social vulnerability and capacity for resilience among coastal communities. Insights into which communities may be more likely to recover following large-scale storms, floods and coastal land loss as a result of sea level rise is useful information for researchers, policy- makers and residents of coastal communities facing nu- merous, increasing climate-related hazards. 7. Acknowledgements This research was supported by a grant from the U.S. Bureau of Ocean Energy Management (BOEM), Coope- rative Agreement number M07AC12941 and in part by a joint grant from the National Science Foundation (NSF) and the US Department of Agriculture (USDA), Federal Award Number: USDA 2010-65401-21312. REFERENCES [1] S. L. Cutter, B. J. Boruff and W. L. Shirley, “Social Vul- nerability to Environmental Hazards,” Social Science Quarterly, Vol. 84, No. 2, 2003, pp. 242-261. Copyright © 2012 SciRes. AJCC
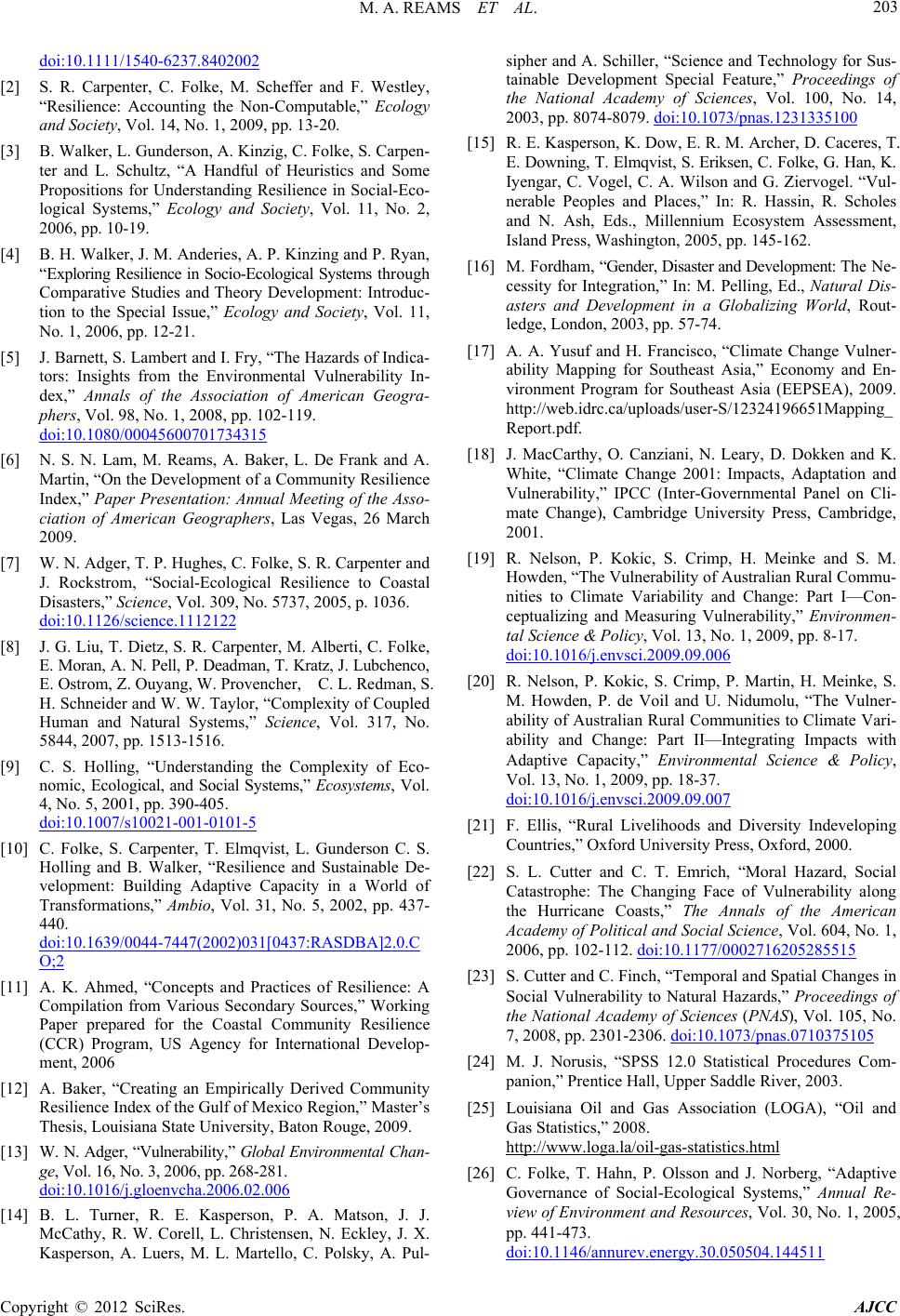 M. A. REAMS ET AL. 203 doi:10.1111/1540-6237.8402002 [2] S. R. Carpenter, C. Folke, M. Scheffer and F. Westley, “Resilience: Accounting the Non-Computable,” Ecology and Society, Vol. 14, No. 1, 2009, pp. 13-20. [3] B. Walker, L. Gunderson, A. Kinzig, C. Folke, S. Carpen- ter and L. Schultz, “A Handful of Heuristics and Some Propositions for Understanding Resilience in Social-Eco- logical Systems,” Ecology and Society, Vol. 11, No. 2, 2006, pp. 10-19. [4] B. H. Walker, J. M. Anderies, A. P. Kinzing and P. Ryan, “Exploring Resilience in Socio-Ecological Systems through Comparative Studies and Theory Development: Introduc- tion to the Special Issue,” Ecology and Society, Vol. 11, No. 1, 2006, pp. 12-21. [5] J. Barnett, S. Lambert and I. Fry, “The Hazards of Indica- tors: Insights from the Environmental Vulnerability In- dex,” Annals of the Association of American Geogra- phers, Vol. 98, No. 1, 2008, pp. 102-119. doi:10.1080/00045600701734315 [6] N. S. N. Lam, M. Reams, A. Baker, L. De Frank and A. Martin, “On the Development of a Community Resilience Index,” Paper Presentation: Annual Meeting of the Asso- ciation of American Geographers, Las Vegas, 26 March 2009. [7] W. N. Adger, T. P. Hughes, C. Folke, S. R. Carpenter and J. Rockstrom, “Social-Ecological Resilience to Coastal Disasters,” Science, Vol. 309, No. 5737, 2005, p. 1036. doi:10.1126/science.1112122 [8] J. G. Liu, T. Dietz, S. R. Carpenter, M. Alberti, C. Folke, E. Moran, A. N. Pell, P. Deadman, T. Kratz, J. Lubchenco, E. Ostrom, Z. Ouyang, W. Provencher, C. L. Redman, S. H. Schneider and W. W. Taylor, “Complexity of Coupled Human and Natural Systems,” Science, Vol. 317, No. 5844, 2007, pp. 1513-1516. [9] C. S. Holling, “Understanding the Complexity of Eco- nomic, Ecological, and Social Systems,” Ecosystems, Vol. 4, No. 5, 2001, pp. 390-405. doi:10.1007/s10021-001-0101-5 [10] C. Folke, S. Carpenter, T. Elmqvist, L. Gunderson C. S. Holling and B. Walker, “Resilience and Sustainable De- velopment: Building Adaptive Capacity in a World of Transformations,” Ambio, Vol. 31, No. 5, 2002, pp. 437- 440. doi:10.1639/0044-7447(2002)031[0437:RASDBA]2.0.C O;2 [11] A. K. Ahmed, “Concepts and Practices of Resilience: A Compilation from Various Secondary Sources,” Working Paper prepared for the Coastal Community Resilience (CCR) Program, US Agency for International Develop- ment, 2006 [12] A. Baker, “Creating an Empirically Derived Community Resilience Index of the Gulf of Mexico Region,” Master’s Thesis, Louisiana State University, Baton Rouge, 2009. [13] W. N. Adger, “Vulnerability,” Global Environmental Chan- ge, Vol. 16, No. 3, 2006, pp. 268-281. doi:10.1016/j.gloenvcha.2006.02.006 [14] B. L. Turner, R. E. Kasperson, P. A. Matson, J. J. McCathy, R. W. Corell, L. Christensen, N. Eckley, J. X. Kasperson, A. Luers, M. L. Martello, C. Polsky, A. Pul- sipher and A. Schiller, “Science and Technology for Sus- tainable Development Special Feature,” Proceedings of the National Academy of Sciences, Vol. 100, No. 14, 2003, pp. 8074-8079. doi:10.1073/pnas.1231335100 [15] R. E. Kasperson, K. Dow, E. R. M. Archer, D. Caceres, T. E. Downing, T. Elmqvist, S. Eriksen, C. Folke, G. Han, K. Iyengar, C. Vogel, C. A. Wilson and G. Ziervogel. “Vul- nerable Peoples and Places,” In: R. Hassin, R. Scholes and N. Ash, Eds., Millennium Ecosystem Assessment, Island Press, Washington, 2005, pp. 145-162. [16] M. Fordham, “Gender, Disaster and Development: The Ne- cessity for Integration,” In: M. Pelling, Ed., Natural Dis- asters and Development in a Globalizing World, Rout- ledge, London, 2003, pp. 57-74. [17] A. A. Yusuf and H. Francisco, “Climate Change Vulner- ability Mapping for Southeast Asia,” Economy and En- vironment Program for Southeast Asia (EEPSEA), 2009. http://web.idrc.ca/uploads/user-S/12324196651Mapping_ Report.pdf. [18] J. MacCarthy, O. Canziani, N. Leary, D. Dokken and K. White, “Climate Change 2001: Impacts, Adaptation and Vulnerability,” IPCC (Inter-Governmental Panel on Cli- mate Change), Cambridge University Press, Cambridge, 2001. [19] R. Nelson, P. Kokic, S. Crimp, H. Meinke and S. M. Howden, “The Vulnerability of Australian Rural Commu- nities to Climate Variability and Change: Part I—Con- ceptualizing and Measuring Vulnerability,” Environmen- tal Science & Policy, Vol. 13, No. 1, 2009, pp. 8-17. doi:10.1016/j.envsci.2009.09.006 [20] R. Nelson, P. Kokic, S. Crimp, P. Martin, H. Meinke, S. M. Howden, P. de Voil and U. Nidumolu, “The Vulner- ability of Australian Rural Communities to Climate Vari- ability and Change: Part II—Integrating Impacts with Adaptive Capacity,” Environmental Science & Policy, Vol. 13, No. 1, 2009, pp. 18-37. doi:10.1016/j.envsci.2009.09.007 [21] F. Ellis, “Rural Livelihoods and Diversity Indeveloping Countries,” Oxford University Press, Oxford, 2000. [22] S. L. Cutter and C. T. Emrich, “Moral Hazard, Social Catastrophe: The Changing Face of Vulnerability along the Hurricane Coasts,” The Annals of the American Academy of Political and Social Science, Vol. 604, No. 1, 2006, pp. 102-112. doi:10.1177/0002716205285515 [23] S. Cutter and C. Finch, “Temporal and Spatial Changes in Social Vulnerability to Natural Hazards,” Proceedings of the National Academy of Sciences (PNAS), Vol. 105, No. 7, 2008, pp. 2301-2306. doi:10.1073/pnas.0710375105 [24] M. J. Norusis, “SPSS 12.0 Statistical Procedures Com- panion,” Prentice Hall, Upper Saddle River, 2003. [25] Louisiana Oil and Gas Association (LOGA), “Oil and Gas Statistics,” 2008. http://www.loga.la/oil-gas-statistics.html [26] C. Folke, T. Hahn, P. Olsson and J. Norberg, “Adaptive Governance of Social-Ecological Systems,” Annual Re- view of Environment and Resources, Vol. 30, No. 1, 2005, pp. 441-473. doi:10.1146/annurev.energy.30.050504.144511 Copyright © 2012 SciRes. AJCC
 M. A. REAMS ET AL. Copyright © 2012 SciRes. AJCC 204 [27] D. Austin, B. Carriker, T. McGuire, J. Pratt, T. Priest and A. G. Pulsipher, “History of the Offshore Oil and Gas Industry in Southern Louisiana, Interim Report: Volume I,” Papers on the Evolving Offshore Industry, US De- partment of the Interior, Minerals Management Service, Gulf of Mexico OCS Region, New Orleans, 2004. [28] R. Antonius, “Interpreting Quantitative Data with SPSS,” Sage Publications, London, 2003.
|