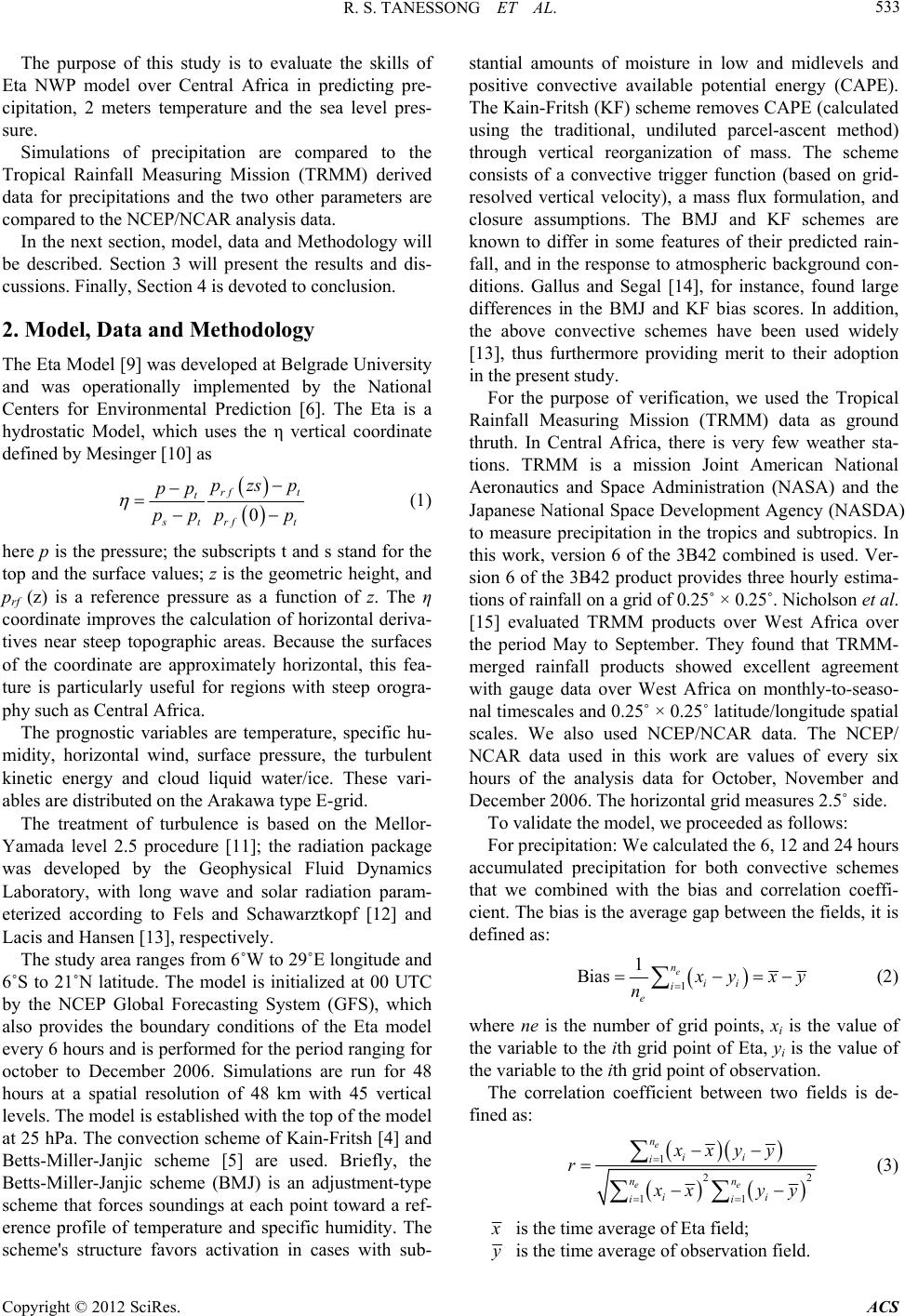
R. S. TANESSONG ET AL. 533
The purpose of this study is to evaluate the skills of
Eta NWP model over Central Africa in predicting pre-
cipitation, 2 meters temperature and the sea level pres-
sure.
Simulations of precipitation are compared to the
Tropical Rainfall Measuring Mission (TRMM) derived
data for precipitations and the two other parameters are
compared to the NCEP/NCAR analysis data.
In the next section, model, data and Methodology will
be described. Section 3 will present the results and dis-
cussions. Finally, Section 4 is devoted to conclusion.
2. Model, Data and Methodology
The Eta Model [9] was developed at Belgrade University
and was operationally implemented by the National
Centers for Environmental Prediction [6]. The Eta is a
hydrostatic Model, which uses the η vertical coordinate
defined by Mesinger [10] as
0
rf t
t
trf t
pzsp
pp
ppp p
(1)
here p is the pressure; the subscripts t and s stand for the
top and the surface values; z is the geometric height, and
prf (z) is a reference pressure as a function of z. The η
coordinate improves the calculation of horizontal deriva-
tives near steep topographic areas. Because the surfaces
of the coordinate are approximately horizontal, this fea-
ture is particularly useful for regions with steep orogra-
phy such as Central Africa.
The prognostic variables are temperature, specific hu-
midity, horizontal wind, surface pressure, the turbulent
kinetic energy and cloud liquid water/ice. These vari-
ables are distributed on the Arakawa type E-grid.
The treatment of turbulence is based on the Mellor-
Yamada level 2.5 procedure [11]; the radiation package
was developed by the Geophysical Fluid Dynamics
Laboratory, with long wave and solar radiation param-
eterized according to Fels and Schawarztkopf [12] and
Lacis and Hansen [13], respectively.
The study area ranges from 6˚W to 29˚E longitude and
6˚S to 21˚N latitude. The model is initialized at 00 UTC
by the NCEP Global Forecasting System (GFS), which
also provides the boundary conditions of the Eta model
every 6 hours and is performed for the period ranging for
october to December 2006. Simulations are run for 48
hours at a spatial resolution of 48 km with 45 vertical
levels. The model is established with the top of the model
at 25 hPa. The convection scheme of Kain-Fritsh [4] and
Betts-Miller-Janjic scheme [5] are used. Briefly, the
Betts-Miller-Janjic scheme (BMJ) is an adjustment-type
scheme that forces soundings at each point toward a ref-
erence profile of temperature and specific humidity. The
scheme's structure favors activation in cases with sub-
stantial amounts of moisture in low and midlevels and
positive convective available potential energy (CAPE).
The Kain-Fritsh (KF) scheme removes CAPE (calculated
using the traditional, undiluted parcel-ascent method)
through vertical reorganization of mass. The scheme
consists of a convective trigger function (based on grid-
resolved vertical velocity), a mass flux formulation, and
closure assumptions. The BMJ and KF schemes are
known to differ in some features of their predicted rain-
fall, and in the response to atmospheric background con-
ditions. Gallus and Segal [14], for instance, found large
differences in the BMJ and KF bias scores. In addition,
the above convective schemes have been used widely
[13], thus furthermore providing merit to their adoption
in the present study.
For the purpose of verification, we used the Tropical
Rainfall Measuring Mission (TRMM) data as ground
thruth. In Central Africa, there is very few weather sta-
tions. TRMM is a mission Joint American National
Aeronautics and Space Administration (NASA) and the
Japanese National Space Development Agency (NASDA)
to measure precipitation in the tropics and subtropics. In
this work, version 6 of the 3B42 combined is used. Ver-
sion 6 of the 3B42 product provides three hourly estima-
tions of rainfall on a grid of 0.25˚ × 0.25˚. Nicholson et al.
[15] evaluated TRMM products over West Africa over
the period May to September. They found that TRMM-
merged rainfall products showed excellent agreement
with gauge data over West Africa on monthly-to-seaso-
nal timescales and 0.25˚ × 0.25˚ latitude/longitude spatial
scales. We also used NCEP/NCAR data. The NCEP/
NCAR data used in this work are values of every six
hours of the analysis data for October, November and
December 2006. The horizontal grid measures 2.5˚ side.
To validate the model, we proceeded as follows:
For precipitation: We calculated the 6, 12 and 24 hours
accumulated precipitation for both convective schemes
that we combined with the bias and correlation coeffi-
cient. The bias is the average gap between the fields, it is
defined as:
1
1
Bias e
n
ii
i
e
yxy
n
(2)
where ne is the number of grid points, xi is the value of
the variable to the ith grid point of Eta, yi is the value of
the variable to the ith grid point of observation.
The correlation coefficient between two fields is de-
fined as:
1
2
11
e
ee
n
ii
i
nn
ii
ii
xxyy
r2
xyy
(3)
is the time average of Eta field;
is the time average of observation field.
Copyright © 2012 SciRes. ACS