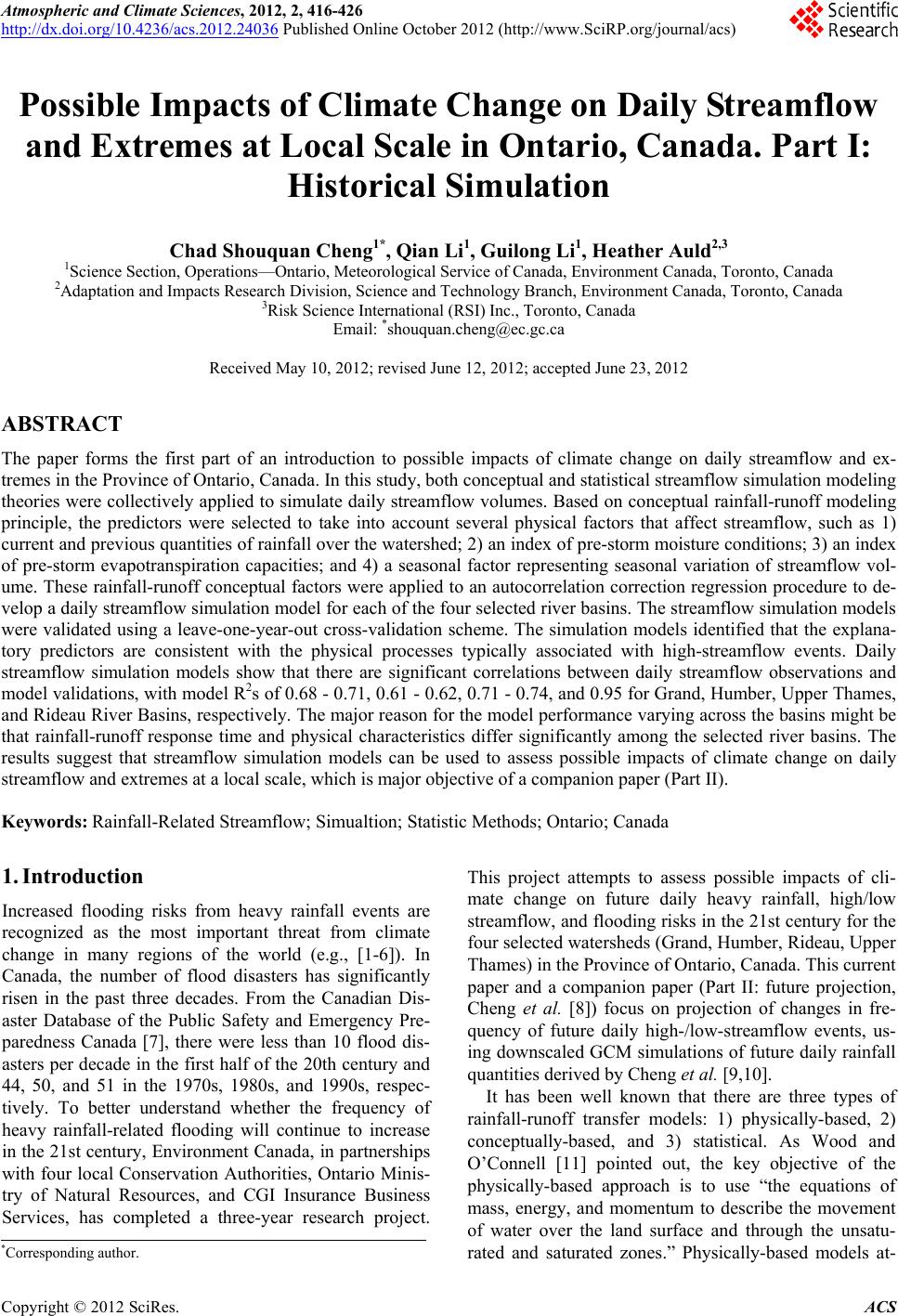 Atmospheric and Climate Sciences, 2012, 2, 416-426 http://dx.doi.org/10.4236/acs.2012.24036 Published Online October 2012 (http://www.SciRP.org/journal/acs) Possible Impacts of Climate Change on Daily Streamflow and Extremes at Local Scale in Ontario, Canada. Part I: Historical Simulation Chad Shouquan Cheng1*, Qian Li1, Guilong Li1, Heather Auld2,3 1Science Section, Operations—Ontario, Meteorological Service of Canada, Environment Canada, Toronto, Canada 2Adaptation and Impacts Research Division, Science and Technology Branch, Environment Canada, Toronto, Canada 3Risk Science International (RSI) Inc., Toronto, Canada Email: *shouquan.cheng@ec.gc.ca Received May 10, 2012; revised June 12, 2012; accepted June 23, 2012 ABSTRACT The paper forms the first part of an introduction to possible impacts of climate change on daily streamflow and ex- tremes in the Province of Ontario, Canada. In this study, both conceptual and statistical streamflow simulation modeling theories were collectively applied to simulate daily strea mflow volumes. Based on conceptual ra infall-runoff modeling principle, the predictors were selected to take into account several physical factors that affect streamflow, such as 1) current and prev ious quan tities of rainfall over the watershed; 2 ) an index o f pre-storm moisture con dition s; 3) an index of pre-storm evapotranspiration capacities; and 4) a seasonal factor representing seasonal variation of streamflow vol- ume. These rainfall-runoff conceptual factors were applied to an autocorrelation correction regression procedure to de- velop a daily streamflow simulation model for each of the four selected river basins. The streamflow simulation models were validated using a leave-one-year-out cross-validation scheme. The simulation models identified that the explana- tory predictors are consistent with the physical processes typically associated with high-streamflow events. Daily streamflow simulation models show that there are significant correlations between daily streamflow observations and model validations, with model R2s of 0.68 - 0.71, 0.61 - 0.62, 0.71 - 0.74, and 0.95 for Grand, Humber, Upper Thames, and Rideau River Basins, respectively. The major reason for the model performance varying across the basins might be that rainfall-runoff response time and physical characteristics differ significantly among the selected river basins. The results suggest that streamflow simulation models can be used to assess possible impacts of climate change on daily streamflow and extremes at a local scale, which is major objective of a companion paper (Part II). Keywords: Rainfall-Related Streamflow; Simualtion; Statistic Methods; Ontario; Canada 1. Introduction Increased flooding risks from heavy rainfall events are recognized as the most important threat from climate change in many regions of the world (e.g., [1-6]). In Canada, the number of flood disasters has significantly risen in the past three decades. From the Canadian Dis- aster Database of the Public Safety and Emergency Pre- paredness Canada [7], there were less than 10 flood dis- asters per decade in the first half of the 20th century and 44, 50, and 51 in the 1970s, 1980s, and 1990s, respec- tively. To better understand whether the frequency of heavy rainfall-related flooding will continue to increase in the 21st century, Environment Canada, in partnerships with four local Conservation Authorities, Ontario Minis- try of Natural Resources, and CGI Insurance Business Services, has completed a three-year research project. This project attempts to assess possible impacts of cli- mate change on future daily heavy rainfall, high/low streamflow, and flooding risks in the 21st century for the four selected watersheds (Grand, Humber, Rideau, Upper Thames) in the Province of Ontario, Canada. This current paper and a companion paper (Part II: future projection, Cheng et al. [8]) focus on projection of changes in fre- quency of future daily high-/low-streamflow events, us- ing downscaled GCM simulations of future daily rainfall quantities derived by Cheng et al. [9,10]. It has been well known that there are three types of rainfall-runoff transfer models: 1) physically-based, 2) conceptually-based, and 3) statistical. As Wood and O’Connell [11] pointed out, the key objective of the physically-based approach is to use “the equations of mass, energy, and momentum to describe the movement of water over the land surface and through the unsatu- rated and saturated zones.” Physically-based models at- *Corresponding a uthor. C opyright © 2012 SciRes. ACS
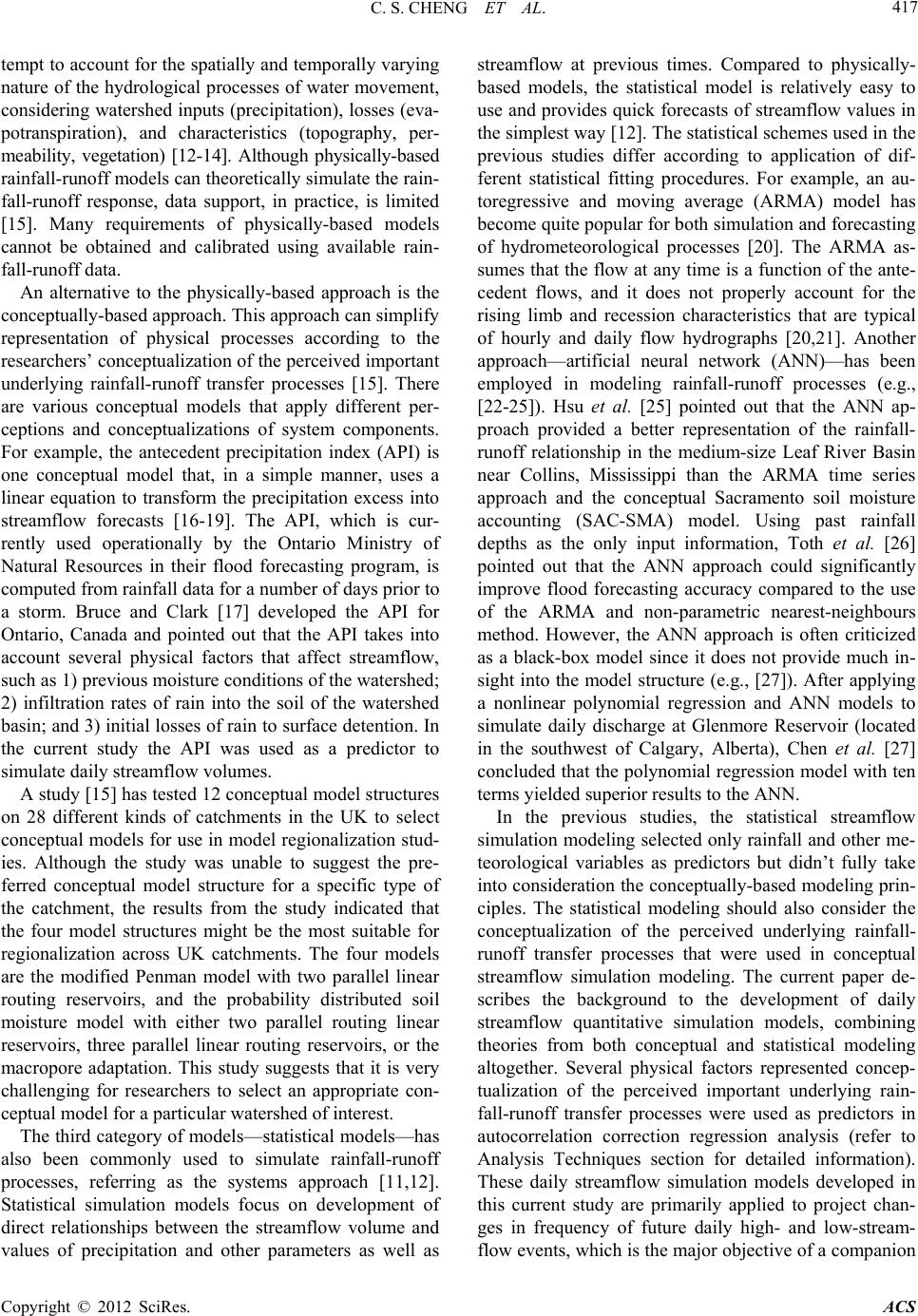 C. S. CHENG ET AL. Copyright © 2012 SciRes. ACS 417 tempt to account for the spatially an d temporally varying nature of the hydrological processes of water movement, considering watershed inpu ts (precipitation), losses (ev a- potranspiration), and characteristics (topography, per- meability, vegetation) [12-14]. Although physically-based rainfall-runoff models can theoretically si mulate the rain- fall-runoff response, data support, in practice, is limited [15]. Many requirements of physically-based models cannot be obtained and calibrated using available rain- fall-runoff data. An alternative to the physically-based approach is the conceptually-based approach. This approach can simplify representation of physical processes according to the researchers’ conceptualization of the perceived important underlying rainfall-runoff transfer processes [15]. There are various conceptual models that apply different per- ceptions and conceptualizations of system components. For example, the antecedent precipitation index (API) is one conceptual model that, in a simple manner, uses a linear equation to transform the precipitation excess into streamflow forecasts [16-19]. The API, which is cur- rently used operationally by the Ontario Ministry of Natural Resources in their flood forecasting program, is computed from rainfall data for a number of days prior to a storm. Bruce and Clark [17] developed the API for Ontario, Canada and pointed out that the API takes into account several physical factors that affect streamflow, such as 1) previous moisture cond itio ns of the watershe d; 2) infiltration rates of rain into the soil of the watershed basin; and 3) initial losses of rain to surface detention. In the current study the API was used as a predictor to simulate daily streamflow volumes. A study [15] has tested 12 conceptual model structures on 28 different kinds of catchments in the UK to select conceptual models for use in model regionalization stud- ies. Although the study was unable to suggest the pre- ferred conceptual model structure for a specific type of the catchment, the results from the study indicated that the four model structures might be the most suitable for regionalization across UK catchments. The four models are the modified Penman model with two parallel linear routing reservoirs, and the probability distributed soil moisture model with either two parallel routing linear reservoirs, three parallel linear routing reservoirs, or the macropore adaptation. This study suggests that it is very challenging for researchers to select an appropriate con- ceptual model for a particular watershed of interest. The third category of models—statistical models—has also been commonly used to simulate rainfall-runoff processes, referring as the systems approach [11,12]. Statistical simulation models focus on development of direct relationships between the streamflow volume and values of precipitation and other parameters as well as streamflow at previous times. Compared to physically- based models, the statistical model is relatively easy to use and provides quick forecasts of streamflow values in the simplest way [12]. The statistical schemes us ed in the previous studies differ according to application of dif- ferent statistical fitting procedures. For example, an au- toregressive and moving average (ARMA) model has become quite popular for both simulation and forecasting of hydrometeorological processes [20]. The ARMA as- sumes that the flow at any time is a function of the an te- cedent flows, and it does not properly account for the rising limb and recession characteristics that are typical of hourly and daily flow hydrographs [20,21]. Another approach—artificial neural network (ANN)—has been employed in modeling rainfall-runoff processes (e.g., [22-25]). Hsu et al. [25] pointed out that the ANN ap- proach provided a better representation of the rainfall- runoff relationship in the medium-size Leaf River Basin near Collins, Mississippi than the ARMA time series approach and the conceptual Sacramento soil moisture accounting (SAC-SMA) model. Using past rainfall depths as the only input information, Toth et al. [26] pointed out that the ANN approach could significantly improve flood forecasting accuracy compared to the use of the ARMA and non-parametric nearest-neighbours method. However, the ANN approach is often criticized as a black-box model since it does not provide much in- sight into the model structure (e.g., [27]). After applying a nonlinear polynomial regression and ANN models to simulate daily discharge at Glenmore Reservoir (located in the southwest of Calgary, Alberta), Chen et al. [27] concluded that the polynomial regression model with ten terms yielded superior results to the ANN. In the previous studies, the statistical streamflow simulation modeling selected only rainfall and other me- teorological variables as predictors but didn’t fully take into consideration the co nceptually-based modeling prin- ciples. The statistical modeling should also consider the conceptualization of the perceived underlying rainfall- runoff transfer processes that were used in conceptual streamflow simulation modeling. The current paper de- scribes the background to the development of daily streamflow quantitative simulation models, combining theories from both conceptual and statistical modeling altogether. Several physical factors represented concep- tualization of the perceived important underlying rain- fall-runoff transfer processes were used as predictors in autocorrelation correction regression analysis (refer to Analysis Techniques section for detailed information). These daily streamflow simulation models developed in this current study are primarily applied to project chan- ges in frequency of future daily high- and low-stream- flow events, which is the major objective of a companion
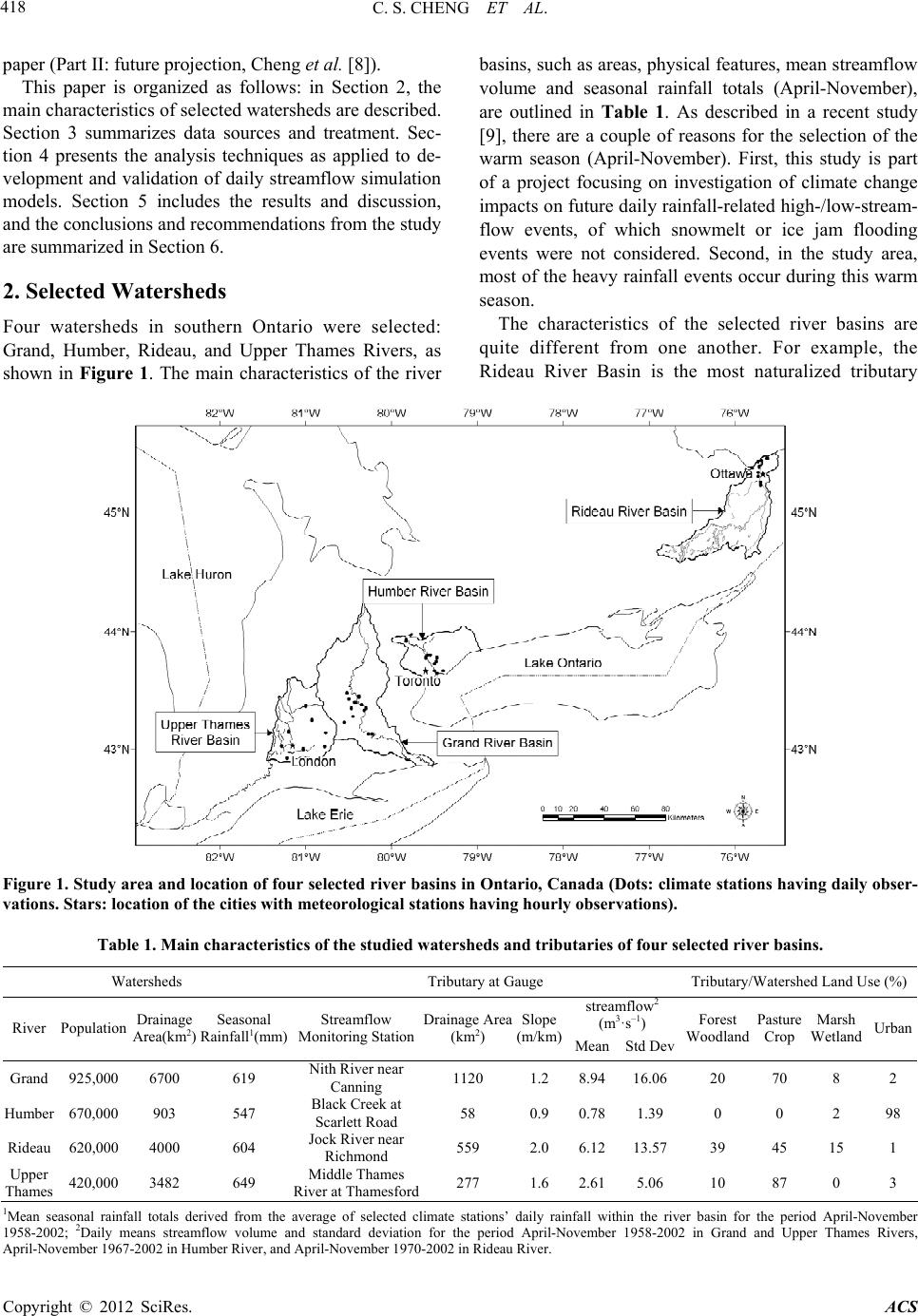 C. S. CHENG ET AL. Copyright © 2012 SciRes. ACS 418 paper (Part II: fut ure p ro jection , C heng et al. [8]). basins, such as areas, physical features, mean streamflow volume and seasonal rainfall totals (April-November), are outlined in Table 1. As described in a recent study [9], there are a couple of reasons for the selection of the warm season (April-November). First, this study is part of a project focusing on investigation of climate change impacts on future daily rainfall-related high-/low-stream- flow events, of which snowmelt or ice jam flooding events were not considered. Second, in the study area, most of the heavy rainfall events occur during this warm season. This paper is organized as follows: in Section 2, the main characteristics of selected watersheds are described. Section 3 summarizes data sources and treatment. Sec- tion 4 presents the analysis techniques as applied to de- velopment and validation of daily streamflow simulation models. Section 5 includes the results and discussion, and the conclusions and recommendations from the study are summarized in Section 6. 2. Selected Watersheds The characteristics of the selected river basins are quite different from one another. For example, the Rideau River Basin is the most naturalized tributary Four watersheds in southern Ontario were selected: Grand, Humber, Rideau, and Upper Thames Rivers, as shown in Figure 1. The main characteristics of the river Figure 1. Study area and location of four selected river basins in Ontario, Canada (Dots: climate stations having daily obser- vations. Stars: location of the cities with meteorological stations having hourly observations). Table 1. Main characteristics of the studied watersheds and tributaries of four selected river basins. Watersheds Tributary at Gauge Tributary/Watershed Land Use (%) streamflow2 (m3·s–1) River Population Drainage Area(km2) Seasonal Rainfall1(mm) Streamflow Monitoring StationDrainage Area (km2) Slope (m/km) MeanStd Dev Forest Woodland Pasture Crop Marsh Wetland Urban Grand 925,000 6700 619 Nith River near Canning 1120 1.2 8.9416.06 20 70 8 2 Humber 670,000 903 547 Black Creek at Scarlett Road 58 0.9 0.781.39 0 0 2 98 Rideau 620,000 4000 604 Jock River near Richmond 559 2.0 6.1213.5739 45 15 1 Upper Thames 420,000 3482 649 Middle Thames River at Thamesford277 1.6 2.615.06 10 87 0 3 1Mean seasonal rainfall totals derived from the average of selected climate stations’ daily rainfall within the river basin for the period April-November 1958-2002; 2Daily means streamflow volume and standard deviation for the period April-November 1958-2002 in Grand and Upper Thames Rivers, April-November 1967-2002 in Humber River, and April-November 1970-2002 in Rideau R i ver.
 C. S. CHENG ET AL. Copyright © 2012 SciRes. ACS 419 among the selected river basins. Specifically, it has the greatest percentage coverage of forest (39%) and wet- land (15%). Conversely, the Humber River (the Black Creek tributary) flows through a developed, urban area; of all the selected watersheds, it has the greatest per- centage of urban coverage (98%). This urban watershed was selected, because of its canalized waterway with the faster rainfall-streamflow response timing compared to natural waterways in rural watersheds, to witness there is any difference in development of daily streamflow simulation models between urban and rural watersheds. Other two watersheds, the Upper Thames and Grand, are predominantly covered by agricultural fields with 87% and 70% coverage, respectively. The major soil types found in each of the river basins also differ: the Rideau is composed of clay and limestone, the Grand is governed by till soils (in the northern half) and sandy moraine soils (in the southern half), the Upper Thames is made up of loam and silt/clay loams, and the Humber is primarily concrete surfaces. 3. Data Sources and Treatment Historical observations on daily rainfall and daily mean temperature for the period April-November 1958-2002 were also used in this study. Daily rainfall data were extracted from Environment Canada’s National Climate Data and Information Archive. As shown in Figure 1, a number of climate stations within each of the river basins were selected for the analysis: 13, 12, 13, and 9 for Grand, Humber, Rideau, and Upper Thames Rivers, re- spectively, based on the length of the available data re- cord (e.g., above 25 years). Daily rainfall data observed at the selected climate stations in each river basin were used to calculate mean daily rainfall quantities, repre- senting average rainfall conditions for the catchments. Within-river-basin average daily rainfall amounts were used to develop daily streamflow simulation models in the current study. In addition, daily mean temperature observed at the meteorological station located in the in- ternational airport within each of the watersheds was used in daily streamflow simulation modeling. Daily streamflow data were retrieved from Environ- ment Canada’s HYDAT CD-ROM that provides access to the National Water Data Archive. The archive con- tains daily and monthly data for streamflow, water level, and sediment data for over 2500 active and 5500 discon- tinued hydrometric monitoring stations across Canada [28]. In each of the selected river basins, there are a number of streamflow monitoring stations available. To more effectively develop a rainfall-runoff transfer model, non-regulated streamflow monitoring stations should be used. One non-regulated streamflow monitoring station in each river basin was recommended and selected by scientists from the local Conservation Authorities, as shown in Table 1. Another consideration for selection of streamflow stations is the length of the data record. For both Grand and Upper Thames rivers, the data record of the period April-November 1958-2002 observed at the selected streamflow monitoring stations was used in the study, April-November 1967-2002 for Humber River, and April- November 1970-2002 for Rideau River. Of the selected streamflow monitoring stations, about 0.05% of the total days in the stud y period possess missing data for the Nith River near Canning in the Grand River Basin; there is no missing data for other selected streamflow monitoring stations in the remaining three watersheds. 4. Analysis Techniques 4.1. Development of Daily Streamflow Simulation Models Streamflow simulation models developed in this study are comprised of a two aspects: 1) selection of a regr- ession method and 2) selection of predictors. When time- series data, like daily streamflow volumes, are used in developing a regression-based prediction model, the se- rial correlation in time-series data should be taken into account. Without considering serial correlation, the or- dinary regression residuals, assumed to be independent of one other, are usually correlated over time due to autocorrelation [ 29]. Consequently, the regression analy- sis excluding autocorrelation is not suitable when using time-series data because the statistical assumptions on which the linear regression model is based are usually violated. On the other hand, the autoregressive model is not appropriate either as it does not account for the rising limb and recession characteristics of the daily flow hy- drographs [20,21]. To overcome these problems, an al- ternative technique that caters for both the serial correla- tion and rising limb/ recession characteristics should be employed to simulate daily streamflow volumes. Auto- regressive error correction regression is able to take into consideration both persistence and deterministic terms in daily streamflow simulation modeling [29]. The Statisti- cal Analysis Software (SAS) AUTOREG procedure [29] was used in the study since the procedure can simulta- neously estimate the regression coefficients by fitting an ordinary least squares model and the autoregressive error model parameters by fitting a generalized least squares model to correct the regression estimates for autocorrela- tion. Predictors used in the development of the daily stre- amflow simulation model are listed in Table 2. These predictors were selected based on a nalyses of the rela- tionships between streamflow and predictors as well as
 C. S. CHENG ET AL. Copyright © 2012 SciRes. ACS 420 Table 2. Predictors used in the development of daily stre- amflow simulation models. Predictor Description V01 Antecedent precipitation index (API) V02 API2 V03 Antecedent tem perature index (ATI) V04 Previous-days’ rainfall amount and current-day rainfall amount V05 Polynomial function of Julian day the results derived from the previous studies (e.g., [17,19]). The antecedent precipitation index (API) takes into account several physical factors that affect stream- flow, as Bruce and Clark [17] pointed out, such as 1) pre-storm moisture conditions in the watershed, 2) infil- tration rates of rain into the soil of the river basin, and 3) initial losses of rain to surface detention. The API was calculated following Kohler and Linsley’s equation [16], using river-basin-averaged daily rainfall data: 1 Int t t Pk AP (1) where Pt is precipitation during da y t, n is the number of antecedent days, and k is a decay constant. The Ontario Ministry of Natural Resources (MNR) has used the val- ues n = 24 and k = 0.84 in API calculations for Ontario operational flood forecasting program. Before applying these parameters to the current study, the relationship between the API and decay constants and the number of antecedent days needs to be evaluated, using river-basin- averaged rainfall data, to ascertain whether the parame- ter’s values are representative in the study area. As an example shown in Figure 2 for Upper Thames River, the API curved lines can be divided into two distinctive groups by the line with 0.84 of the decay constant (simi- lar results were discovered for other rivers as well). With a larger decay constant (>0.84), it takes very long time (more than one or two months) for the API to approach a stable value. On the other hand, when using a smaller decay constant (<0.84), the API approaches a stable value in a very short time period (only a few days). When k = 0.84 is used, it takes about 24 days for the API to reach a stable value. To calculate the API for April, the rainfall data observed in March were used in the analysis. In addition to the API, the API2 was also used in the study since there is a quadratic relationship be- tween the API and daily streamflow volumes in the se- lected river basins based on analysis. The antecedent temperature index (ATI) was com- puted from daily mean temperature data for the seven days prior to the present rainfall being recorded. Daily mean temperature was used to compute the ATI because of its stronger relationship with station elevations and latitudes than eith er maximum or minimum temperatures [30]. The ATI was first introduced by Hopkins and Hackett in 1961 as a factor in the prediction of runoff from storm rainfall in New England and New York, the United States. The ATI takes into account previous evapotranspiration capacities that affect streamflow , esp e- cially during drought summertime. The ATI was calcu- lated by the following algorithm modified from Hopkins and Hackett’s equation, using daily mean temperature observed at a meteorological station within each river basin: 7 11 1 ATI0.9ATI0.1 7 ii t t T (2) where subscript i represents today, and the second term of the equation’s right side represents mean temperature (T) for the past seven days. For the early April, the ATI was calculated using the temperature data observed in the late March. Another important predictor for development of streamflow simulation models is rainfall information. The current-day, previous-day, and day-before-yesterday rainfall quantities were considered as predictors when developing the daily streamflow simulation models. Which of those to be selected as predictors depend on the rainfall-streamflow response time of the river basin. These predictors were tested to develop daily streamflow simulation models based upon their relationship with streamflow for each of the river basins. The test results indicated that the current-day rainfall significantly con- tributes to the streamflow for all selected river basins. The previous-day rainfall is also included in streamflow simulation modeling, which was selected by autoregres- sive error correction regression for the Humber and Up- per Thames Rivers. The day-before-yest erday’s rainfall is selected to develop daily streamflow simulation mod- 0 5 10 15 20 25 30 147 10131619222528 API 0.92 0.88 0.84 0.80 0.76 0.72 0.68 0.64 0.60 K—decay constant Number of Antecedent Days Figure 2. Relationships between the antecedent precipita- tion index (API) and decay constants/the number of ante- cedent days using Upper Thames River Basin’s daily aver- age rainfall (1961-2002).
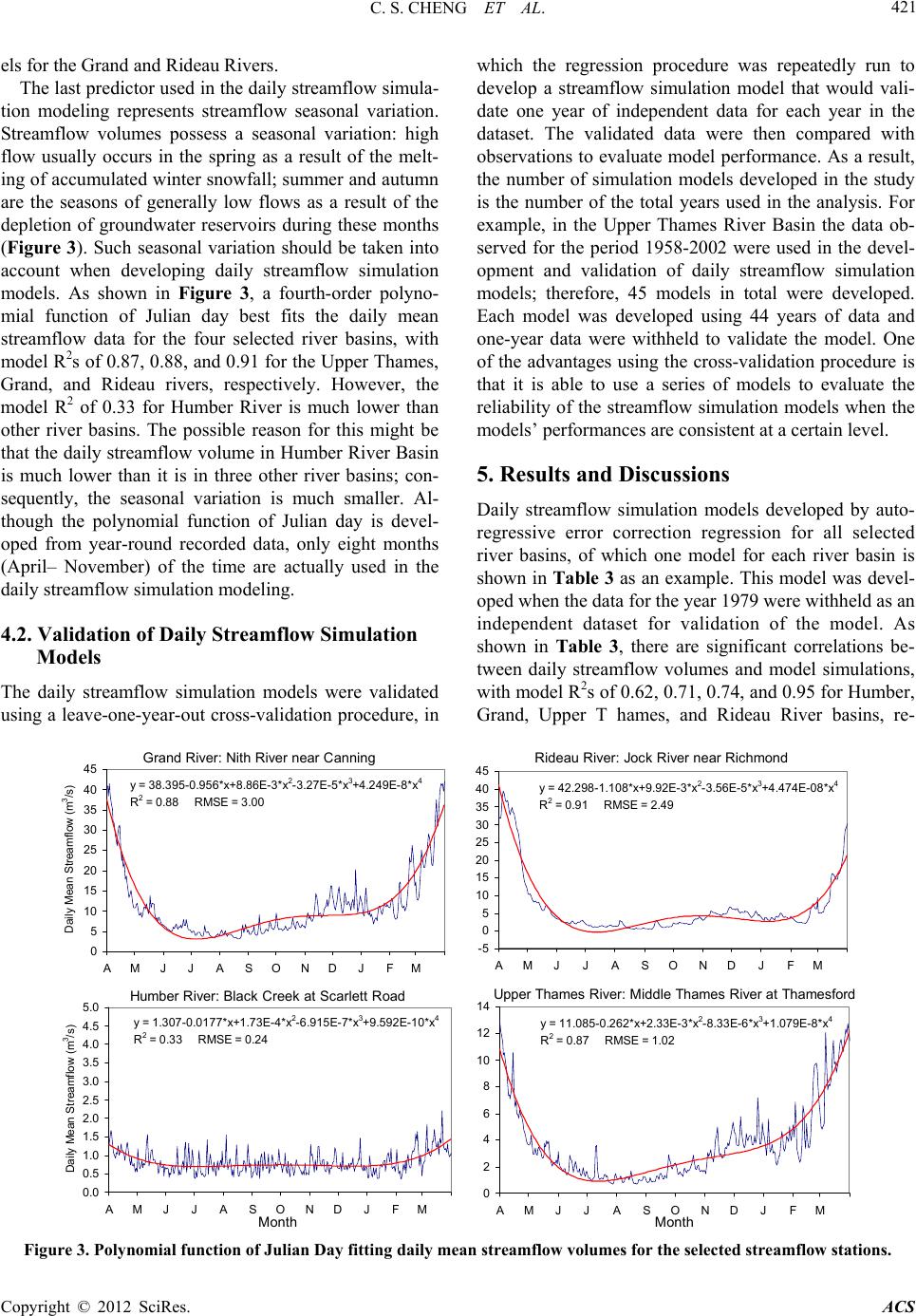 C. S. CHENG ET AL. Copyright © 2012 SciRes. ACS 421 els for the Grand and Rideau Rivers. The last predictor used in th e daily streamflow simula- tion modeling represents streamflow seasonal variation. Streamflow volumes possess a seasonal variation: high flow usually occurs in the spring as a result of the melt- ing of accumulated winter snowfall; summer and autumn are the seasons of generally low flows as a result of the depletion of groundwater reservoirs during these months (Figure 3). Such seasonal variation should be taken into account when developing daily streamflow simulation models. As shown in Figure 3, a fourth-order polyno- mial function of Julian day best fits the daily mean streamflow data for the four selected river basins, with model R2s of 0.87, 0.88, and 0.91 for the Upper Thames, Grand, and Rideau rivers, respectively. However, the model R2 of 0.33 for Humber River is much lower than other river basins. The possible reason for this might be that the daily streamflow volume in Humber River Basin is much lower than it is in three other river basins; con- sequently, the seasonal variation is much smaller. Al- though the polynomial function of Julian day is devel- oped from year-round recorded data, only eight months (April– November) of the time are actually used in the daily streamflow simulation modeling. 4.2. Validation of Daily Streamflow Simulation Models The daily streamflow simulation models were validated using a leave-one-year-out cross-validation procedure, in which the regression procedure was repeatedly run to develop a streamflow simulation model that would vali- date one year of independent data for each year in the dataset. The validated data were then compared with observations to evaluate model performance. As a result, the number of simulation models developed in the study is the number of the total years used in the analysis. For example, in the Upper Thames River Basin the data ob- served for the period 1958-2002 were used in the devel- opment and validation of daily streamflow simulation models; therefore, 45 models in total were developed. Each model was developed using 44 years of data and one-year data were withheld to validate the model. One of the advantages using the cross-validation procedure is that it is able to use a series of models to evaluate the reliability of the streamflow simulation models when the models’ performances are consistent at a certain level. 5. Results and Discussions Daily streamflow simulation models developed by auto- regressive error correction regression for all selected river basins, of which one model for each river basin is shown in Table 3 as an example. This model was devel- oped when the data for the year 1979 were withheld as an independent dataset for validation of the model. As shown in Table 3, there are significant correlations be- tween daily streamflow volumes and model simulations, with model R2s of 0.62, 0.71, 0.74, and 0.95 for Humber, Grand, Upper T hames, and Rideau River basins, re- Grand River: Nith River near Canning y = 38.395-0. 956* x+ 8. 8 6E -3*x2-3.27E-5*x3+4.249E-8*x4 R2 = 0.88 RMS E = 3. 00 0 5 10 15 20 25 30 35 40 45 AMJJ ASONDJ FM Daily Mean Streamflow (m 3 /s) Rideau River: Jock River near Richmond y = 42.29 8-1.108*x +9. 92E-3*x 2-3.56E-5*x3+4.474E-08*x4 R2 = 0. 91 RM S E = 2.49 -5 0 5 10 15 20 25 30 35 40 45 AMJJASONDJFM y = 11.08 5-0.262*x +2. 33E-3*x 2-8.33E-6*x3+1.079E-8*x4 R2 = 0. 87 RM S E = 1. 02 0 2 4 6 8 10 12 14 AM J JASOND JFM Month Upper Thames River: Middle Thames River at Thamesford Humber River: Black C reek at Sc arlet t R oad y = 1.30 7-0.0177*x +1. 73E-4*x 2-6.915E-7*x3+9.592E-10*x4 R2 = 0. 33 RM S E = 0. 24 0.0 0.5 1.0 1.5 2.0 2.5 3.0 3.5 4.0 4.5 5.0 AMJ J ASONDJ FM Month Daily Mean Streamflow (m 3 /s) Figure 3. Polynomial function of Julian Day fitting daily mean streamflow volumes for the selected streamflow stations.
 C. S. CHENG ET AL. Copyright © 2012 SciRes. ACS 422 Table 3. Summary of the streamflow simulation models when the data of the year 1979 withheld as an independent dataset for validation of the model in the four selected river basins. Variable EstimateStd Err t Value Approx Pr > |t| Grand River Basin (R2 = 0.71, RMSE = 7.50) Intercept –4.44270.9743 –4.56 <0.0001 API 0.5021 0.0454 11.07 <0.0001 API2 0.0002 0.0009 0.18 0.8578 ATI –0.24540.0471 –5.21 <0.0001 Julian Day 0.9752 0.0556 17.53 <0.0001 Current-Day Rainfall 0.0035 0.0169 0.21 0.8372 Day-before-Yesterday Rainfall 0.5275 0.0145 36.39 <0.0001 AR1 –0.75440.0054 –139.18 <0.0001 Humber River Basin (R2 = 0.62, RMSE = 0.75) Intercept –0.29990.0557 –5.38 <0.0001 API 0.0151 0.0028 5.43 <0.0001 API2 0.000020.0001 0.31 0.7576 ATI –0.02550.0012 –21.71 <0.0001 Julian Day 0.7610 0.0619 12.30 <0.0001 Current-Day Rainfall 0.1313 0.0018 73.66 <0.0001 Previous-Day Rainfal l 0.1573 0.0022 72.68 <0.0001 AR1 –0.11000.0089 –12.37 <0.0001 Rideau River Basin (R2 = 0.95, RMSE = 2.95 ) Intercept 4.3389 0.9030 4.81 <0.0001 API 0.1983 0.0165 12.02 <0.0001 API2 0.0015 0.0003 5.06 <0.0001 ATI –0.25230.0586 –4.31 <0.0001 Julian Day 0.1612 0.0258 6.24 <0.0001 Current-Day Rainfall 0.0226 0.0059 3.83 0.0001 Day-before-Yesterday Rainfall 0.0608 0.0046 13.13 <0.0001 AR1 –0.96260.0025 –375.75 <0.0001 Upper Thames River Basin (R2 = 0.74, RMSE = 2.40) Intercept –2.32470.3478 –6.68 <0.0001 API 0.2190 0.0160 13.66 <0.0001 API2 0.0040 0.0003 13.08 <0.0001 ATI –0.14520.0174 –8.36 <0.0001 Julian Day 0.9333 0.0593 15.74 <0.0001 Current-Day Rainfall 0.0406 0.0059 6.89 <0.0001 Previous-Day Rainfal l 0.0546 0.0070 7.76 <0.0001 AR1 –0.73120.0056 –130.55 <0.0001 Note: RMSE = root mean squared error; API = antecedent precipitation index; ATI = antecedent temperature index; AR1 = the order 1 or lag 1 autocorr elation paramet er; Estimate = the coeff icient of t he regressi on algo- rithm; and Std Err = standard error. spectively. The simulation models have identified that the predictors are consistent with the physical processes typically associated with high flow events. From the simulation models provided in Table 3 it is seen that the first-order autoregressive process (AR1), which repre- sents the serial correlation of streamflow itself and the explanatory predicto rs, sign ificantly contribute to stream- flow simulation. Since a negative AR1 is built in the autoregressive error correction modeling, there is a posi- tive relationship between the AR1 and daily streamflow volumes. In addition to the persistence of high-stream- flow, rainfall/moisture conditions (i.e., today/previous- day rainfall and API) and streamflow seasonal variation (i.e., polynomial function of Julian day) are positively associated with daily streamflow volumes. Furthermore, there is a significantly negative relationship between the ATI and daily streamflow volumes since the ATI repre- sents evapotranspiration within the river basins. The ro- bustness of the developed streamflow simulation models is examined considering not only the AR1 but also the higher order parameters, such as the second-order to fifth-order autoregressive processes. The test results showed that considering any of higher order parameters, the simulation models didn’t improve much on the model performance in terms of model R2 (with 0.00 - 0.02 in- crease) and root mean squared errors (with 0.0 - 0.2 m3·s–1 decrease). As a result, it is not necessary to con- sider any of order parameters higher than the first-order autoregressive process in the daily streamflow simulation modeling. In addition to sample models summarized in Table 3, all streamflow simulation models’ R2s and root mean squared errors (RMSEs) for the selected river basins are shown in Table 4. For reference, overall daily mean streamflow volume and standard deviation for the entire study period are also listed in Table 4. Furthermore, the comparison between streamflow observations and model validations is illustrated in Figure 4, of which the model R2s and RMSEs are very similar to the model simulations as shown in Table 4. To effectively measure difference between model simulations and validations, the differ- ence between the two data sample means and variances was analyzed, respectively using t- and F-tests; no sig- nificant (<0.05 level) difference between the daily streamflow simulations and validations was detected. It implies that the stream- flow simulation models devel- oped in the study are reliable with a great potential to be used to downscale future daily streamflow volumes at a local scale. From Table 4 and Figure 4, it can be seen that the streamflow simulation model performance for Upper Th- ames and Grand Rivers is very similar, with model R2s ranging from 0.68 to 0.74. The streamflow simulation
 C. S. CHENG ET AL. 423 models for Rideau River demonstrated the strongest models of the selected river basins with R2 of 0.95; for Humber River, the simulation models are the weakest with R2 ranging from 0.61 to 0.62. This implies that the methods used in the study are more suitable to develop simulation models of daily rainfall- related streamflow volumes for nature waterways in rural watersheds, such as Rideau, Grand, and Upper Thames Rivers. The meth- ods are limited for an urban river basin like the Black Creek tributary of the Humber River. The reasons for this might be that rainfall-streamflow hydrological response time and physical characteristics differ significantly be- tween urban and rural river basins as described as fol- lows: 1) The API is not a good indicator to simulate daily streamflow for an urban river basin like the Humber River. As discussed above, the API takes into account several physical factors that affect streamflow, including previous soil moisture conditions of the watershed. The API is suitable to characterize the soil moisture condi- tions for rural river basins (e.g., Grand, Rideau, and Up- per Thames) but not for urban river basins with a con- crete waterway (e.g., Humber River). 2) The serial correlation of daily streamflow volumes, presented by the AR1 in streamflow simulation models, varies among the river basins. From Table 3, the AR1s for Rideau and Humber Rivers are the largest (–0.96) and smallest (–0.11) of the selected river basins, respectively; the parameters for Grand and Upper Thames Rivers fall in the middle with the similar values (i.e. , –0.77 and –0.73). 3) The seasonal variation of daily mean streamflow (the polynomial function of Ju l i a n day) , whi ch is another Table 4. Streamflow simulation model R2s and root mean squared errors (RMSE), using a leave-one-year-out cross- validation scheme, for the selected river basins (overall daily mean streamflow volume and standard deviation are listed for reference). River Basin Grand Humber Rideau Upper Thames Model R2 0.68 - 0.710.61 - 0.62 0.95 - 0.95 0.71 - 0.74 RMSE (m3·s–1)7.71 - 8.030.74 - 0.76 2.85 - 2.95 2.40 - 2.56 Daily mean (Std Dev) (m3·s–1)8.94 (16.06)0.78 (1.39) 6.12 (13.57) 2.61 (5.06) Note: Overall daily mean streamflow volume and standard deviation were calculated for the period April-November 1958-2002 in Grand and Upper Thames Rivers, April-November 1967-2002 in Humber River, and April- November 1970-2002 in Rideau Rive r. Gr and River Basin R 2 = 0.70 RMSE = 8.72 0 40 80 120 160 200 240 280 320 04080120 160200 240280 320 Verifica tion (m 3 s -1 ) Ri deau River Basin R 2 = 0.95 RMSE = 2.98 0 15 30 45 60 75 90 105 120 135 150 0153045607590105120 135150 Humber River Basin R 2 = 0.61 RMSE = 0.87 0 5 10 15 20 25 0510 1520 25 O bservation m 3 s -1 Upper Thames River Basi n R 2 = 0. 73 RMSE = 2.61 0 15 30 45 60 75 90 105 120 0 15304560759010512 Verification (m 3 s -1 ) Obser vati on (m 3 s -1 ) Figure 4. Relationships between streamflow observations and model validations using a leave-one-year-out cross-validation scheme in the selected river basins (solid line represents a regression line; dashed line is a perfect fit. Grand and Upper Thames Rivers: 1958-2002; Humber River: 1967-2002; Rideau River: 1970-2002). Copyright © 2012 SciRes. ACS
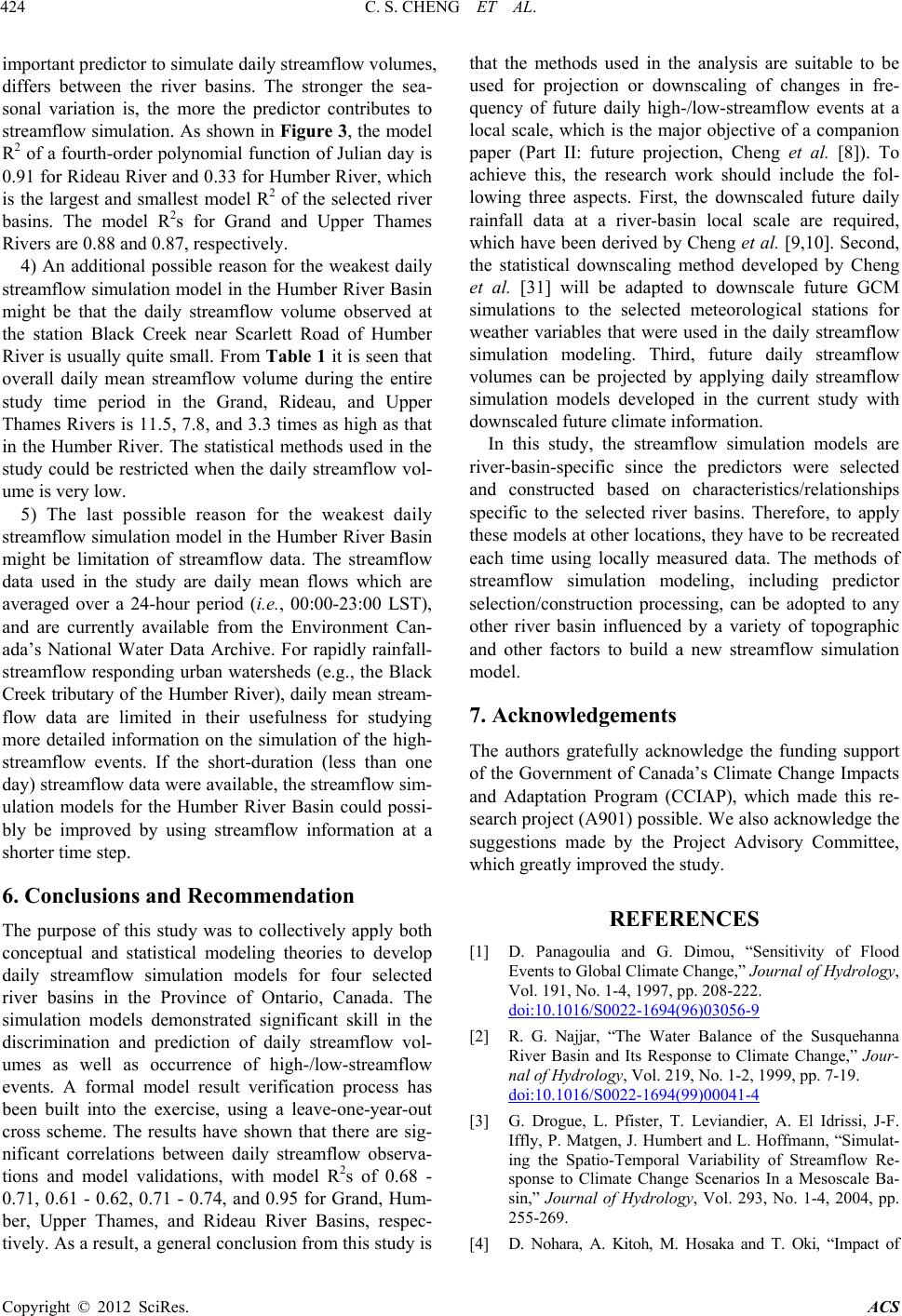 C. S. CHENG ET AL. 424 important predictor to simulate daily streamflow volumes, differs between the river basins. The stronger the sea- sonal variation is, the more the predictor contributes to streamflow simulation. As shown in Figure 3, the model R2 of a fourth-order polynomial function of Julian day is 0.91 for Rideau River and 0.33 for Humber River, which is the largest and smallest model R2 of the selected river basins. The model R2s for Grand and Upper Thames Rivers are 0.88 and 0.87 , resp ect i vely . 4) An additional possible reason for the weakest daily streamflow simulation model in the Humber River Basin might be that the daily streamflow volume observed at the station Black Creek near Scarlett Road of Humber River is usually quite small. From Table 1 it is seen that overall daily mean streamflow volume during the entire study time period in the Grand, Rideau, and Upper Thames Rivers is 11.5, 7.8, and 3.3 times as high as that in the Humber River. The statistical methods used in the study could be restricted when the daily streamflow vol- ume is very low. 5) The last possible reason for the weakest daily streamflow simulation model in the Humber River Basin might be limitation of streamflow data. The streamflow data used in the study are daily mean flows which are averaged over a 24-hour period (i.e., 00:00-23:00 LST), and are currently available from the Environment Can- ada’s National Water Data Archive. For rapidly rainfall- streamflow responding urban watersheds (e.g., the Black Creek tributary of the Humber River), daily mean stream- flow data are limited in their usefulness for studying more detailed information on the simulation of the high- streamflow events. If the short-duration (less than one day) streamflow data were available, the streamflow sim- ulation models for the Humber River Basin could possi- bly be improved by using streamflow information at a shorter time step. 6. Conclusions and Recommendation The purpose of this study was to collectively apply both conceptual and statistical modeling theories to develop daily streamflow simulation models for four selected river basins in the Province of Ontario, Canada. The simulation models demonstrated significant skill in the discrimination and prediction of daily streamflow vol- umes as well as occurrence of high-/low-streamflow events. A formal model result verification process has been built into the exercise, using a leave-one-year-out cross scheme. The results have shown that there are sig- nificant correlations between daily streamflow observa- tions and model validations, with model R2s of 0.68 - 0.71, 0.61 - 0.62, 0.71 - 0.74, and 0.95 for Grand, Hum- ber, Upper Thames, and Rideau River Basins, respec- tively. As a result, a general con clusion from this stud y is that the methods used in the analysis are suitable to be used for projection or downscaling of changes in fre- quency of future daily high-/low-streamflow events at a local scale, which is the major objective of a companion paper (Part II: future projection, Cheng et al. [8]). To achieve this, the research work should include the fol- lowing three aspects. First, the downscaled future daily rainfall data at a river-basin local scale are required, which have been derived by Cheng et al. [9,10]. Second, the statistical downscaling method developed by Cheng et al. [31] will be adapted to downscale future GCM simulations to the selected meteorological stations for weather variables that were used in the daily streamflow simulation modeling. Third, future daily streamflow volumes can be projected by applying daily streamflow simulation models developed in the current study with downscaled future climate information. In this study, the streamflow simulation models are river-basin-specific since the predictors were selected and constructed based on characteristics/relationships specific to the selected river basins. Therefore, to apply these models at other locations, they have to be recreated each time using locally measured data. The methods of streamflow simulation modeling, including predictor selection/construction processing, can be adopted to any other river basin influenced by a variety of topographic and other factors to build a new streamflow simulation model. 7. Acknowledgements The authors gratefully acknowledge the funding support of the Government of Canada’s Climate Change Impacts and Adaptation Program (CCIAP), which made this re- search project (A901) possible. We also acknowledge the suggestions made by the Project Advisory Committee, which greatly improved the study. REFERENCES [1] D. Panagoulia and G. Dimou, “Sensitivity of Flood Events to Global Climate Change,” Journal of Hydrology, Vol. 191, No. 1-4, 1997, pp. 208-222. doi:10.1016/S0022-1694(96)03056-9 [2] R. G. Najjar, “The Water Balance of the Susquehanna River Basin and Its Response to Climate Change,” Jour- nal of Hydrology, Vol. 219, No. 1-2, 1999, pp. 7-19. doi:10.1016/S0022-1694(99)00041-4 [3] G. Drogue, L. Pfister, T. Leviandier, A. El Idrissi, J-F. Iffly, P. Matgen, J. Humbert and L. Hoffmann, “Simulat- ing the Spatio-Temporal Variability of Streamflow Re- sponse to Climate Change Scenarios In a Mesoscale Ba- sin,” Journal of Hydrology, Vol. 293, No. 1-4, 2004, pp. 255-269. [4] D. Nohara, A. Kitoh, M. Hosaka and T. Oki, “Impact of Copyright © 2012 SciRes. ACS
 C. S. CHENG ET AL. 425 Climate Change on River Discharge Projected by Multi- model Ensemble,” Journal of Hydrometeorology, Vol. 7, No. 5, 2006, pp. 1076-1089. doi:10.1175/JHM531.1 [5] A. L. Kay, R. G. Jones and N. S. Reynard, “RCM Rain- fall for UK Flood Frequency Estimation. II: Climate Change Results,” Journal of Hydrology, Vol. 318, No. 1-4, 2006, pp. 163-172. doi:10.1016/j.jhydrol.2005.06.013 [6] W. S. Merritt, Y. Alisa, M. Barton, B. Taylor, S. Cohen and D. Neilsen, “Hydrologic Response to Scenarios of Climate Change in Sub-Watersheds of the Okanagan Ba- sin, British Columbia,” Journal of Hydrology, Vol. 326, No. 1-4, 2006, pp. 79-108. doi:10.1016/j.jhydrol.2005.10.025 [7] Public Safety and Emergency Preparedness, “Canadian Disaster Database Version 4.4,” Canada, 2006. [8] C. S. Cheng, Q. Li, G. Li and H. Auld, “Possible Impacts of Climate Change on Future Daily Streamflow and Ex- tremes at Local Scale in Ontario, Canada. Part II: Future projection,” Atmospheric and Climate Sciences, Vol. 2, No. 4, 2012, pp. 427-440. [9] C. S. Cheng, G. Li, Q. Li and H. Auld, “A Synoptic Wea- ther Typing Approach to Simulate Daily Rainfall and Ex- tremes in Ontario, Canada: Potential for Climate Change Projections,” Journal of Applied Meteorology and Cli- matology, Vol. 49, No. 5, 2010, pp. 845-866. doi:10.1175/2010JAMC2016.1 [10] C. S. Cheng, G. Li, Q. Li and H. Auld, “A Synoptic Weather-Typing Approach to Project Future Daily Rain- fall and Extremes at Local Scale in Ontario, Canada,” Journal of Climate, Vol. 24, No. 14, 2011, pp. 3667-3685. doi:10.1175/2011JCLI3764.1 [11] E. F. Wood and P. E. O’Connell, “Real-Time Forecast- ing,” In: M. G. Anderson and T. B. Burt, Eds., Hydrology Forecasting, John Wiley & Sons Ltd., New York, 1985, pp. 505-558. [12] H. Gupta, “Hydrological Modeling for Runoff Forecast- ing,” In: T. D. Potter and B. Colman, Eds., Handbook of Weather, Climate, and Water: Atmospheric Chemistry, Hydrology, and Societal Impacts, John Wiley & Sons, Inc., Hoboken, 2003, pp. 571-586. [13] K. Solander, L. Saito and F. Biondi, “Streamflow Simula- tion Using a Water-Balance Model with Annually-Re- solved Inputs,” Journal of Hydrology, Vol. 387, No. 1-2, 2010, pp. 46-53. doi:10.1016/j.jhydrol.2010.03.028 [14] C. Yang, Z. Lin, Z. Yu, Z. Hao and S. Liu, “Analysis and Simulation of Human Activity Impact on Streamflow in the Huaihe River Basin with a Large-Scale Hydrologic Model,” Journal of Hydrometeorology, Vol. 11, No. 3, 2010, pp. 810-821. doi:10.1175/2009JHM1145.1 [15] H. Lee, N. McIntyre, H. Wheater and A. Young, “Selec- tion of Conceptual Models for Regionalisation of the Rainfall-Runoff Relationship,” Journal of Hydrology, Vol. 312, No. 1-4, 2005, pp. 125-147. doi:10.1016/j.jhydrol.2005.02.016 [16] M. A. Kohler and R. K. Linsley, Jr., “Predicting Runoff from Storm Rainfall,” Research Paper 34, US Weather Bureau, Washington DC, 1951. [17] M. A. Bruce and R. H. Clark, “Introduction to Hydrome- teorology,” Pergamon Press, Oxford, 1966. [18] W. T. Sittner, C. E. Schauss and J. C. Monro, “Continu- ous Hydrograph Synthesis with an API-Type Hydrologic Model,” Water Resources Research, Vol. 5, No. 5, 1969, pp. 1007-1022. doi:10.1029/WR005i005p01007 [19] R. J. Heggen, “Normalized Antecedent Precipitation In- dex,” Journal of Hydrologic Engineering, Vol. 6, No. 5, 2001, pp. 377-381. doi:10.1061/(ASCE)1084-0699(2001)6:5(377) [20] J. D. Salas and R. A. Pielke, “Stochastic Characteristics and Modeling of Hydroclimatic Processes,” In: T. D. Pot- ter and B. Colman, Eds., Handbook of Weather, Climate, and Water: Atmospheric Chemistry, Hydrology, and So- cietal Impacts, John Wiley & Sons, Inc., Hoboken, 2003, pp. 587-605. [21] R. K. Linsley, “Rainfall-Runoff Models—An Overview,” In: V. P. Singh, Ed., Rainfall-runoff Relationship, Book- Crafters Inc., Kansas City, 1981, pp 3-22. [22] K. Hsu, H. V. Gupta and S. Sorooshian, “Artificial Neural Network Modeling of the Rainfall-Runoff Process,” Wa- ter Resources Research, Vol. 31, No. 10, 1995, pp. 2517- 2530. doi:10.1029/95WR01955 [23] J. M. Ehrman, K. Higuchi and T. A. Clair, “Backcasting to Test the Use of Neural Networks for Predicting Runoff in Ca nadia n Ri vers ,” Canadian Water Resources Journal, Vol. 25, No. 3, 2000, pp. 279-291. doi:10.4296/cwrj2503279 [24] A. Nazemi, H. N. Poorkhadem, R. Mohammad, T. Ak- barzadeh and S. M. Hosseini, “Evolutionary Neural Net- work Modeling for Describing Rainfall-Runoff Process,” Hydrology Days, 2003, pp. 224-235. [25] T. Asefa, “Ensemble Streamflow Forecast: A Glue-Based Neural Network Approach,” Journal of the American Water Resource Association, Vol. 45, No. 5, 2009, pp. 1155-1163. doi:10.1111/j.1752-1688.2009.00351.x [26] E. Toth, A. Brath and A. Montanari, “Comparison of Short-Term Rainfall Prediction Models for Real-Time Flood Forecasting,” Journal of Hydrology, Vol. 239, No. 1-4, 2000, pp. 132-147. doi:10.1016/S0022-1694(00)00344-9 [27] L. Chen, F. J. C. Bouchart and J. W. Davison, “Applica- tion of a New Polynomial Regression Method Based on Genetic Programming in Hydrologic Modeling,” The First Biennial Workshop of the Statistical Hydrology, Cana- dian Geophysical Union, Calgary, 18-21 May 2002, 6 pp. [28] Environment Canada, “HYDAT CD-ROM,” 2006. http://www.wsc.ec.gc.ca/products/hydat/main_e.cfm?cna me=hydat_e.cfm [29] SAS Institute Inc., “The AUTOREG Procedure: Regres- sion with Autocorrelation Errors,” 2006. http://support.sas.com/91doc/docMainpage.jsp [30] C. D. Hopkins Jr. and D. O. Hackett, “Average Antece- dent Temperatures As a Factor in Predicting Runoff from Storm Rainfall,” Journal of Geophysical Research, Vol. 66, No. 10, 1961, 3313-3318. doi:10.1029/JZ066i010p03313 [31] C. S. Cheng, G. Li, Q. Li and H. Auld, “Statist ical Down- Copyright © 2012 SciRes. ACS
 C. S. CHENG ET AL. Copyright © 2012 SciRes. ACS 426 scaling of Hourly and Daily Climate Scenarios for Vari- ous Meteorological Variables in South-Central Canada,” Theoretical and Applied Climatology, Vol. 91, No. 1-4, 2008, pp. 129-147. doi:10.1007/s00704-007-0302-8
|