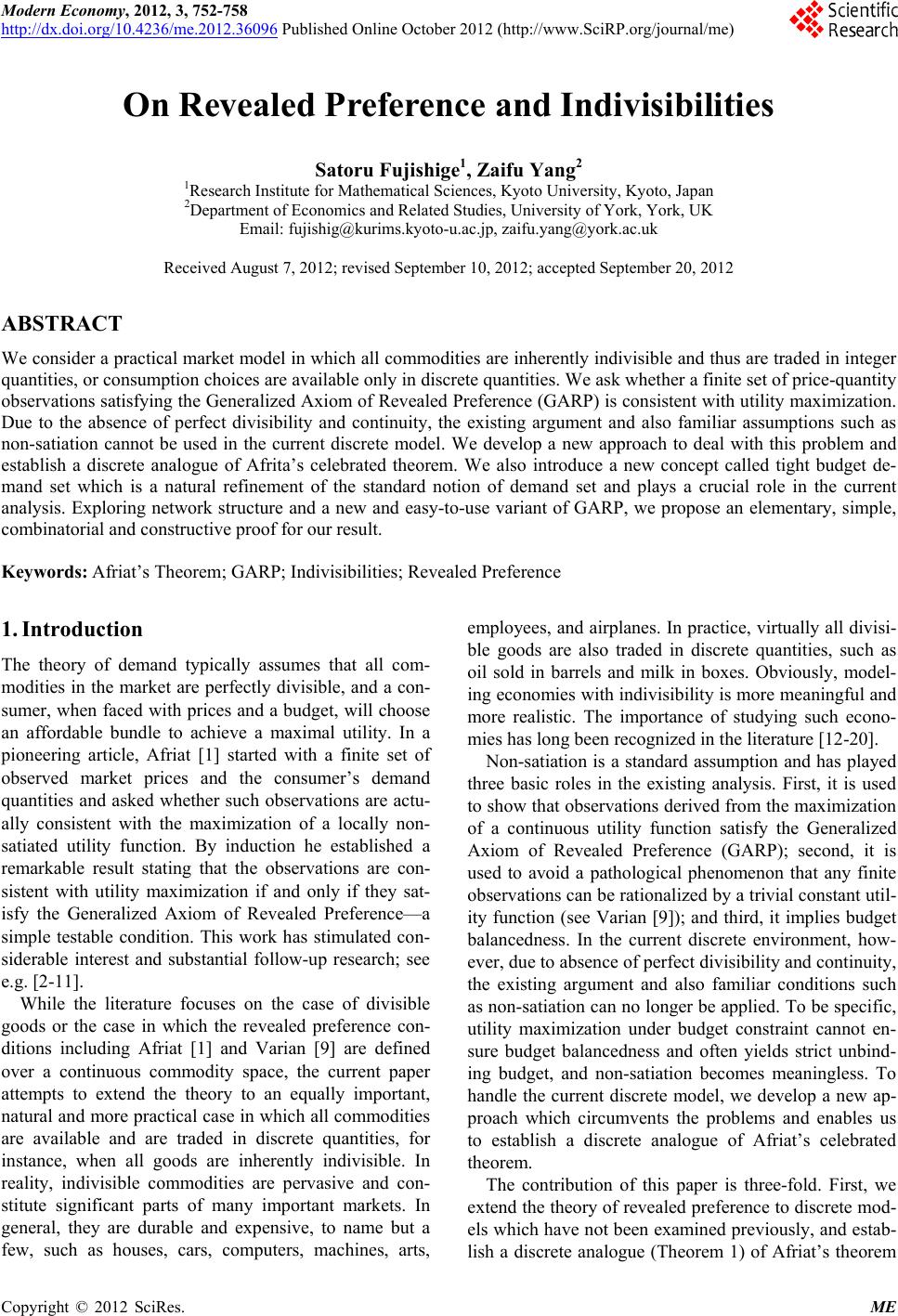 Modern Economy, 2012, 3, 752-758 http://dx.doi.org/10.4236/me.2012.36096 Published Online October 2012 (http://www.SciRP.org/journal/me) On Revealed Preference and Indivisibilities Satoru Fujishige1, Zaifu Yang2 1Research Institute for Mathematical Sciences, Kyoto University, Kyoto, Japan 2Department of Economics and Related Studies, University of York, York, UK Email: fujishig@kurims.kyoto-u.ac.jp, zaifu.yang@york.ac.uk Received August 7, 2012; revised September 10, 2012; accepted September 20, 2012 ABSTRACT We consider a practical market model in which all commodities are inh erently indivisible and thus are traded in integer quantities, or consumption choices are av ailab le only in discrete quantities. We ask whether a finite set of price-quantity observations satisfying th e Generalized Axiom of Revealed Preference (GARP) is consistent with utility maximization. Due to the absence of perfect divisibility and continuity, the existing argument and also familiar assumptions such as non-satiation cannot be used in the current discrete model. We develop a new approach to deal with this problem and establish a discrete analogue of Afrita’s celebrated theorem. We also introduce a new concept called tight budget de- mand set which is a natural refinement of the standard notion of demand set and plays a crucial role in the current analysis. Exploring network structure and a new and easy-to-use variant of GARP, we propose an elementary, simple, combinatorial and constru ctive proof for our result. Keywords: Afriat’s Theorem; GARP; Indivisibilities; Revealed Preference 1. Introduction The theory of demand typically assumes that all com- modities in the market are perfectly divisible, and a con- sumer, when faced with prices and a budget, will choose an affordable bundle to achieve a maximal utility. In a pioneering article, Afriat [1] started with a finite set of observed market prices and the consumer’s demand quantities and asked whether such observations are actu- ally consistent with the maximization of a locally non- satiated utility function. By induction he established a remarkable result stating that the observations are con- sistent with utility maximization if and only if they sat- isfy the Generalized Axiom of Revealed Preference—a simple testable condition. This work has stimulated con- siderable interest and substantial follow-up research; see e.g. [2-11]. While the literature focuses on the case of divisible goods or the case in which the revealed preference con- ditions including Afriat [1] and Varian [9] are defined over a continuous commodity space, the current paper attempts to extend the theory to an equally important, natural and more practical case in which all commodities are available and are traded in discrete quantities, for instance, when all goods are inherently indivisible. In reality, indivisible commodities are pervasive and con- stitute significant parts of many important markets. In general, they are durable and expensive, to name but a few, such as houses, cars, computers, machines, arts, employees, and airplanes. In practice, virtually all divisi- ble goods are also traded in discrete quantities, such as oil sold in barrels and milk in boxes. Obviously, model- ing economies with indivisibility is more meaningful and more realistic. The importance of studying such econo- mies has long been recognized in the literature [12-20]. Non-satiation is a standard assumption and has played three basic roles in the existing analysis. First, it is used to show that observations d erived from the maximization of a continuous utility function satisfy the Generalized Axiom of Revealed Preference (GARP); second, it is used to avoid a pathological phenomenon that any finite observations can b e rationalized by a trivial constan t util- ity function (see Varian [9]); and third, it implies budget balancedness. In the current discrete environment, how- ever, due to absence of perfect divisibility and continu ity, the existing argument and also familiar conditions such as non-satiation can no longer be applied. To be specific, utility maximization under budget constraint cannot en- sure budget balancedness and often yields strict unbind- ing budget, and non-satiation becomes meaningless. To handle the current discrete model, we develop a new ap- proach which circumvents the problems and enables us to establish a discrete analogue of Afriat’s celebrated theorem. The contribution of this paper is three-fold. First, we extend the theory of revealed preference to discrete mod- els which have not been examined previously, and estab- lish a discrete analogue (Theorem 1) of Afriat’s theorem C opyright © 2012 SciRes. ME
 S. FUJISHIGE, Z. F. YANG 753 that any finite discrete price and quantity observations satisfy GARP if and only if there exists a discrete con- cave utility function that rationalizes the observations in the sense of tight budget utility maximization. Second, we offer a conceptual innovation of tight budget demand set which is a natural and meaningful refinement of the standard notion of demand set. The tight budget demand set is a family of bundles that are affordable, utility maximizers and have the least cost. This concept plays a crucial role in the current analysis and makes the non-satiation assumption obsolete (see Lemma 1). It can also easily avoid the well-known pathological phenome- non caused by the standard utility maximization that any finite number of observations can be rationalized by a trivial constant utility function. Third, we propose a new and easy-to-use variant (Definition 3) of GARP—a benchmark condition widely used in the revealed prefer- ence analysis due to Varian [9] as an alternative to Af- riat’s [1] Cyclical Consistency. Using network structure and the new variant of GARP, we present an elementary, simple, combinatorial and constructive proof for the re- sult. The basic idea of the necessity proof for our main Theorem 1 is similar to Teo and Vohra [8] and was also implicitly used in earlier literature. Here we make the argument very transparent and accessible without as- suming the reader’s familiarity with any fundamental result from graph theory, linear programming, or any other mathematical subject. Finally, it is worth pointing out that our method is not restricted to indivisible goods, and can be equally applied to divisible goods from which the long-standing non-satiation assumption can be drop- ped. 2. Main Results We begin by reviewing the purchase decision problem of a consumer. There are different types of commodities in the market. The consumer has a budget for con- sumption and a utility function . The fol- lowing notation is used throughout the paper. nb :n u n de- notes the nonnegative orthant of the n-dimensional Euclidean space . and stand for the set of all integral vectors in and , respectively. Sup- pose that is the vector of prevailing market prices, each component i indicating the price of com- modity . Then the consumer’s decision problem is to choose a bundle which gives him the highest utility and is also affordable to him. Such a bundle is called an optimal bundle. Alternatively, we can describe all his optimal bundles by using the demand set nn n p n x n n n p i , . n xbx un x ,argmax u Dpbuxp In the literature it is typically assumed that all com- modities are perfectly divisible and also the consumer’s utility function is locally non-satiated in the sense that for every , and in every neighborhood of , there is another bundle having a higher utility. Suppose that a market analyst wishes to examine the consumer’s demand behavior. It is natural to assume that the analyst does not know the consumer’s utility function and his budget flow but does know that the consumer does not change his preferences over a period of time. Suppose that e analyst has now collected a finite observed data set th ,1,, ii px im 1,, ,m with respect to the consumer over the time i in p in x where is the price vector and i p b u is the consumer’s demand bundle under prices and (probably an unobservable) budget i (which may vary over the time). The fundamental question raised by Afriat [1] is whether these observa- tions are consistent with the consumer’s demand behav- ior under a locally non-satiated utility func tion in the sense that , ii ui Dpb1,, .m for all i To verify the consistency, several criteria have been proposed. Among them, the Strong Axiom of Revealed Preference (SARP) and the Generalized Axiom of Revealed Prefer- ence (GARP) are most well-known and widely used. A consumer’s choice behavior is said to satisfy the Strong Axiom of Revealed Preference (SARP) if, for every sequence of pa irs of price vector and demand bun- dle 112 2 ,,,,, , mm pxpxp x 1 satisfying jjj px px 1,jm for all 1. mmm px px we have SARP was proposed by Houthakker [21]. Samuelson [22] introduced a more restrictive axiom than SARP, now known as the Weak Axiom of Revealed Preference (WARP). We also say that the consumer’s behavior satisfies the Generalized Axiom of Revealed Preference (GARP) if, for every sequence of pairs of price vector and demand bundle 112 2 ,,,,, , mm pxpxp x 1 satisfying jjj px px 1,jm for all 1. mmm px px we have GARP is clearly more general than SARP and was introduced in Varian [9]. GARP is equi- valent to Afriat’s Cyclical Consistency. , ii px i M Given a finite observed data set , where 1,,, in Mmp in x is a price vector and , ii ui is the corresponding demand bundle, we say that a utility function u rationalizes the observed be- havior if the data can be generated as the outcome of the utility-maximization, i.e. Dpbi b i for some , ii px iM and for all . The data set is said to satisfy GARP if, for every its subset ,1,, jj ii px jt1 , jjj ii ii px px 1jt for all 1. ttt iii i px px implies Afriat [1] estab- lished a celebrated result stating that a finite observed Copyright © 2012 SciRes. ME
 S. FUJISHIGE, Z. F. YANG 754 data set , ii px iM n n :n p is consistent with the maximi- zation of a locally non-satiated utility function if and only if the observations satisfy GARP. To prove that the observations derived from utility maximization satisfy GARP, the standard approach is to make use of the non-satiation assumption on the utility function; see e.g. Diewert [6], Fostel, Scarf and Todd [7] and Varian [9]. As stated earlier, our purpose is to consider the envi- ronment where all commodities are inherently indivisible, such as houses and cars, or consumption choices are available only in discrete quantities. Needless to say, it is more realistic to assume that all goods are traded in inte- ger (or rational) quantities. Thus in the current situation, the consumer’s consumption set will be instead of , and his utility function will be u. To make the model even more practical, the price space is also assumed to be instead of . For instance, no unit of a price is less than a penny or cent. Under the current framework, non-satiation is meaningless. This implies that the existing approach of using non-satiation to show that the observations derived from utility maxi- mization satisfy GARP can no longer be applied. To deal with the current model, we first need to modify the stan- dard notion of the consumer’s demand set. Given and budget the demand set of the con- sumer is given by n b n n , , . n xbx , u Dpb ,argmax u Dpbuxp We refine the dema nd set as follows: ,,. u yDpb *,Dpb ,Umx m *,, uu Dpb xDpbpxpy That is, u contains those bundles which not only give the consumer the highest utility under his budget but also have the least cost. This tight budget be- havior can be easily justified if we consider the following utility function that is strictly increasing in for each given , where stands for money and for the bundle of indivisible goods. Any bundle in will be called an optimal bundle with tight budget and the tight budget demand set. In this case, we say that the consumer is a tight budget utility maximizer. This refinement is very meaningful and natural, more importantly crucial to our analysis on the current discrete model. Of course, this concept can be applied to the continuous case as well from which the non-satiation assumption can be dropped. m b 12 ,minxx 2 12 ,xx n *, u Dpb x *, u Dp The next little example demonstrates that observations derived just from utility maximization without tight budget could violate GARP. Suppose that the consumer faces two indivisible goods and has the utility function of for every 12 uxx , and a budget of 32. The prevailing market prices are 110,11p and 211,10 ,p respectively. Then we have possible outcomes 11 1, 2,2,1,1, 2,1,1 u xDpb 221 2,1,, . uu Dpb Dpb Because and 121 10pxx 212 10pxx and , GARP is violated! However, using the tight budget demand set we have *1 *2 ,1,1 ,Dpb Dpb uu , so that out- comes should be 12 1,1xx . Because 1212120pxx pxx , GARP is satisfied! Let us make a comparison with the case of divisible goods. We have the same form of utility function min ,ux xx2 x 12 for every and the same budget of 32. The same market prices are 110,11p and 211,10p 2 , respectively. Note that because goods are perfectly divisible, the consumption space is the real space . In this case we have 1*12 *2 ,,, ,32 21,32 21 uuu u Dpb Dpb Dpb Dpb :. n u and GARP is trivially satisfied. Moreover the consumer achieves a higher utility of 32/21 than 1 in the case of indivisible good s. The following result shows a benefit of the introduc- tion of the tight budget demand set. Observe that we do not impose any condition on the consumer’s utility func- tion Th e proof is quite elementary but does make use of the definition of the tight budget demand set. Lemma 1. Ifa finite observed data set , iin n px iM for all , ii px iM with is derived from tight budget utility maximization, the data set must satisfy GARP. *, jj Proof. By assumption we know j Dpb 1, ,jm for all 1 . Suppose that if jjj px px 1, then could have been purchased at prices p 1. Since was not purchased at p, it cannot be strictly pre- so that ferred to 1. jj ux ux The entire se- quence of inequalities 1jj ux 1, ,1jmux , implies 1. m ux ux 1mmm px px Suppose to the contrary that . Then 1m ux ux 1mmm px px together with *, mm um would imply Dpb 1mmm px px , yield- ing a contradiction! So and GARP is satisfied. Q.E.D. It is also worth pointing out another advantage of tight budget utility maximization: it can avoid a well-known pathological phenomenon caused by the standard notion of utility maximization that any finite number of obser- vations can be rationalized by a trivial constant utility Copyright © 2012 SciRes. ME
 S. FUJISHIGE, Z. F. YANG 755 function; see Varian [9,10]. , the second from the fact that jj pxpx 0, j and A utility function is discrete concave if, for any with and any ra- tional numbers :n u 1tn , 0 t 12 ,,,xxtn x 12 0, 0, and the last equality from 1). 1 with 11 j j t and t n j j x 11 . tt , we have j jj jj ux ux We are now ready to present the major result of this paper. The result can be seen as a discrete analogue of the Afriat’s theorem and gives a simple testable neces- sary and sufficient condition that a finite observed data set must satisfy in order to be consistent with tight budg- et utility maximization. Theorem 1 The observations , jnn jM ,Bbm px for all satisfy GARP if and only if there exists a discrete concave and integer-valued utility function that rationalizes the observations in the sense of tight budget utility maximization. “If part” is proved in Lemma 1 above. The proof of `only if’ proceeds in several steps. First we construct the data matrix of order from the obser- vations ij , j px ij x x for all by defining jM ,.M bi ,bij s ,bij p i for all ij Observe that ,0i and all are integral, because s and ps are integral. Following Afriat [1], let us first assume (in fact later we will show) that there exist integers 12 m ,,, 0, ,0 and 12 m 0, ,,,jij M to the following system of lin- ear inequalities—called Afriat inequalities jii bi (1) Now we define the utility function on n . mm p xx by 11 11 min,,mm x pxx Every term in this expression is linear and hence con- cave. Thus, , as their point-wise minimum, is also concave. Since all , , , p and are integral, is an integer value as long as is integral. Because is concave on , obviously its restriction on n n must be discrete concave and integer-valued. The next two steps show that rationalizes the observations. 1) j x for all By definition .jM , i j bij min jiM i x , where the mini- mum is taken from the Afriat inequalities. 2) jj pxpx implies x . Note that jj jjj pxx x , where the first inequality follows from the definition of We have shown that the Afriat inequalities imply the existence of a desirable utility function rationalizing the observations. We will soon prove that if the observa- tions ,, jj px jM** 1,m , satisfy GARP, the system (1) of Afriat inequalities must have integral solutions ** 0, ,0 ,Bbij and . 1m We use the data matrix to construct a ,,GMA n with directed graph , where 1, 2,, m 2,, m ,ijM is the set of vertices corresponding to the indices 1, of the observations, and for ,ijAij with the ordered pair is an arc with an integer length or weight i. Here i is the tail and j the head of the arc (i, j). Let ,bij 11,,1m be the m-vector of all 1’s. In the sequel, we first pay attention to the particular graph 1G . We need to borrow several basic definitions from graph theory. A path in a graph G is a sequence 1122231, ,, ,,, ,,,, kk k iiiiiiii i i, 1, ,jk where are vertices, and ,ii1,2,,1jk 1 i i i 1G B 1G 1jj, are arcs in the graph. In this case we also say that there is a path from vertex to vertex k i. 1 is called the starting vert ex an d k the terminal vertex of the path. A path is a shortest path from vertex i to vertex j in a graph if the sum of the lengths of all arcs on the path is smallest among all possible paths from i to j in the graph. A path with at least one arc is called a cycle if the starting vertex of the path coincides with its terminal vertex and the other vertices are distinct. A cycle is called a negative (zero, or positive) length cycle if the sum of the lengths of all arcs in the cycle is strictly less than zero (equal to zero, or strictly greater than zero). We may use C to de- note a cycle. For ease of notation, C means simply the collection of all arcs in the cycle C. A (sub)graph H is said to be strongly connected if for arbitrary two vertices u, v in the graph H there exists a path in H from u to v. A maximal strongly connected subgraph of a graph G is called a strongly connected component of the graph G. With respect to the graph , we can rephrase the Generalized Axiom of Revealed Preference (GARP) in three different ways. The first was used in Afriat [1] as Cyclical Consistency, the second was given in Teo and Vohra [8], and the third is new but similar to the second, and convenient to use in the following proof. Definition 1. The data matrix satisfies GARP if every cycle C in the graph with ,0bij for all arcs ,,ij C implies for all ,0bij ,ijC . Definition 2. The data matrix satisfies GARP if every negative length cycle in the graph contains at least one arc of positive length. B 1G The following definition differs from the second in Copyright © 2012 SciRes. ME
 S. FUJISHIGE, Z. F. YANG 756 that it does not need to use the sum of the lengths of all arcs in each cycle but instead it requires that if any cycle contains an arc of negative weight, it should also contain an arc of positive weight. Definition 3. The data matrix satisfies GARP if in the graph G every cycle that contains an arc of negative length must also contain an arc of positive length. B 1 We will now introduce a constructive and combinato- rial method which gives explicitly integral solutions ** 1,, m * 1,, m and 1m to the system (1) of Afriat inequalities. The method is based on an algorithm which uses the data matrix as input and yields integral solutions ** 0, ,0 B * ,0bij and 1m as output. The algorithm goes as follows. Observe that if for all then for every ** 0, ,0 iM,,ij M let- ting and for any given integer im- mediately gives a solution to the system (1) of Afriat inequalities. So in the sequel we will assume *1 i * i cc ,0bij for some . ,ijM 3. The Algorithm Initialization. Use the data matrix to construct the graph B 1,.GM ,1A Step 1. Remove all arcs ,ij ,0bij with positive weight from the graph 1G 1G 1G ,, , resulting in a directed graph . Step 2. Decompose the graph into strongly connected components 12 , HH where i s are indexed in such a way that if there exists a path from i to with i, then . If some component i jij contains an arc of negative length, then the observed data is not consistent with GARP, and the algorithm termi- nates. Step 3. Choose a sufficiently large integer e.g. 0,L take 1max,,Lmbij bij 0,,.ij M 1, 2,,d * For every , let the multiplier i of every ver- tex in the subgraph id be equal to *1 , d iL .L, G the th power of 1d Step 4. Use the integers ito construct the graph *,iM * . Take . For any i with , let * 1 0M 1i* i be equal to the length of a shortest path from vertex to vertex in the graph 1i * G . The numbering of the strongly connected components 12 ,,, HH is called a topological ordering, and each i is an equivalence class with respect to the binary relation induced by reachability by paths. Let us illustrate the working of the algorithm by an example. Suppose that the data set is given by ,10,1,1,2, 1,10 ,2,1,1 ii px i M where 1, 2,3,4.M 0, 1,9, 1 11,0,10,0 9, 1,0, 1 10,0, 11,0 B Then its corresponding data ma- trix is 1G It is easy to check that the graph consists of 3 strongly connected components 1 containing vertex 1, 10,11,1,1, 1,10,1,1, 2 vertex 3, and 3 vertices 2 and 4. We have *1, 3, 3,L 1 and *3, ** 9. iM 324 Computing shortest paths from vertex 1 to in the graph * G yields 13 and 24 ** 0, 9, **1. 1G We could also have another topological ordering due to the fact that in the graph , vertices 1 and 3 are not connected. So the graph 1G also consists of 3 strongly connected components 1 con- taining vertex 3, 2 vertex 1, and 3 vertices 2 and 4. We have 3, 3,L 3 1 *1, *3, and ** 24 9. Computing shortest paths in the graph * G*0, yields 1 and *27, **3. *0 *, 324 We are now ready to establish the following general result. Lemma 2. Under GARP, the integers i and i,iM generated by the algorithm, are the solution to the system (1) of Afriat inequalities. Proof. It is easy to see that the graph 1G gener- ated in Step 1 of the algorithm contains no negative length cycle because of GARP, but may contain zero length cycle with all arcs of zero length. Each zero length cycle with all arcs of zero length must be in one of strongly connected components i s . Notice that due to the decomposition into strongly connected components of 1G there exists no path from to i with . ji * See e.g. Fujishige [23] on the decomposition of more general graphs. Next we show that the graph G contains no negative length cycle. Set max,,,,0Kbij ijMbij * G 1.Lm KC Let be any cycle in . and If all the vertices of cycle belong to the vertex set of a single strongly connected component, the length of is nonnegative. Hence we assume that contains vertices of at least two strongly connected components. Let be the maximum index such that i C C C * i i con- tains a vertex of cycle . Then there exists an arc C ** ,yzC* in such that belongs to * i and to * * z ** .ji with Now suppose that the arcs in C of negative length are given by 11ll ,,,,. zyz 1, , Note that for each l , vertex y belongs to with *.ji Hence, the length of Copyright © 2012 SciRes. ME
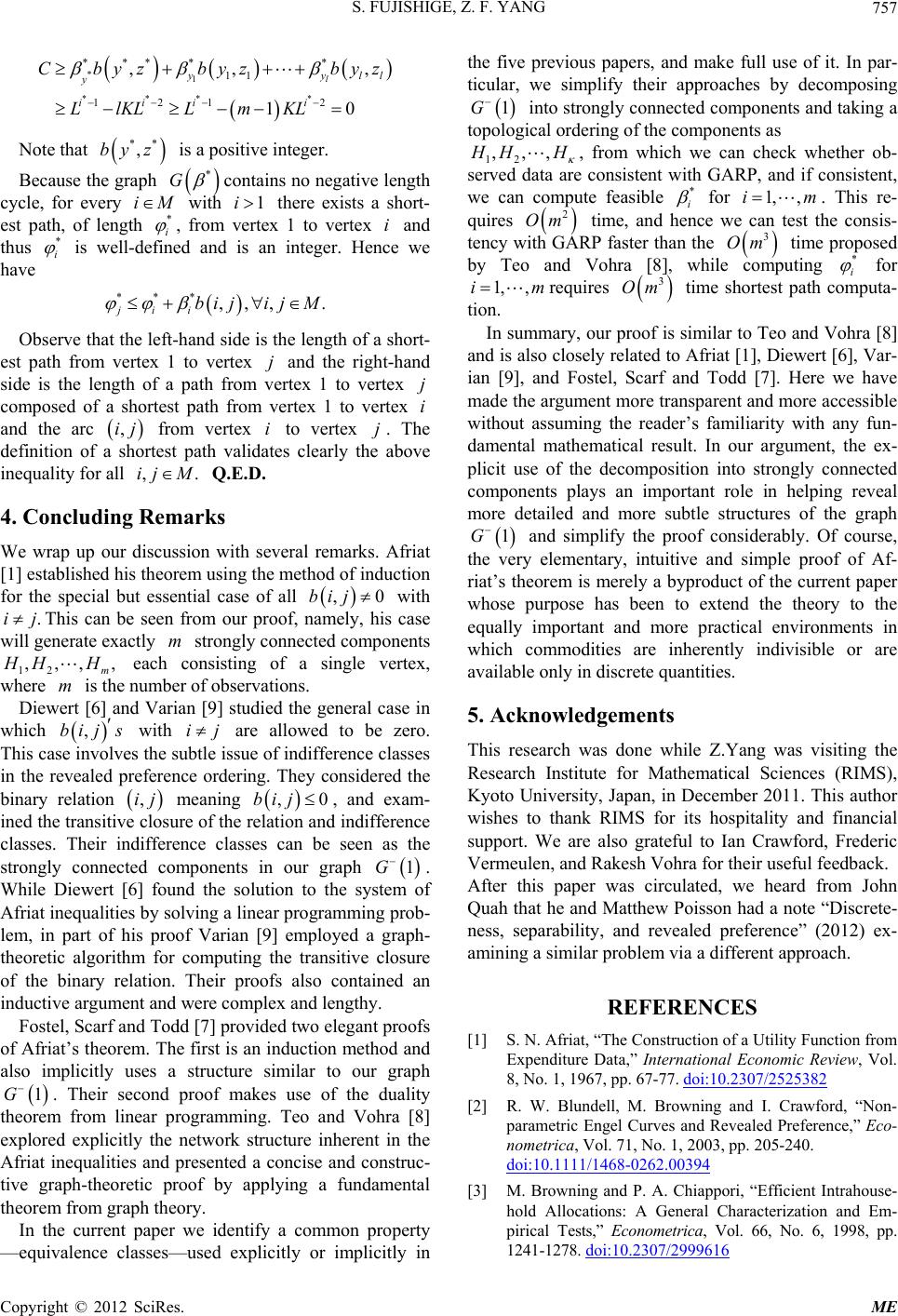 S. FUJISHIGE, Z. F. YANG 757 *1 *** **** 11 121 ,, * * 2 , 10 l yll i byz ** ,by z * G y ii i Cbyz byz LlKLLm KL Note that is a positive integer. Because the graph contains no negative length cycle, for every with there exists a short- est path, of length i* i M1i , from vertex 1 to vertex and thus i * i is well-defined and is an integer. Hence we have ,,, .jij M jj i ,ijj . M ,0bij *** jii bi Observe that the left-hand side is the length of a short- est path from vertex 1 to vertex and the right-hand side is the length of a path from vertex 1 to vertex composed of a shortest path from vertex 1 to vertex and the arc from vertex to vertex . The definition of a shortest path validates clearly the above inequality for all Q.E.D. ,ij i 4. Concluding Remarks We wrap up our discussion with several remarks. Afriat [1] established his theorem using the method of induction for the special but essential case of all with This can be seen from our proof, namely, his case will generate exactly strongly connected components 12 m .ijm ,,,, H mH bij s ij ,0bij each consisting of a single vertex, where is the number of observations. Diewert [6] and Varian [9] studied the general case in which with are allowed to be zero. This case involves the subtle issue of indifference classes in the revealed preference ordering. They considered the binary relation meaning , and exam- ined the transitive closure of the relation and indifference classes. Their indifference classes can be seen as the strongly connected components in our graph , ,ij 1G 1G . While Diewert [6] found the solution to the system of Afriat inequalities by solving a linear programming prob- lem, in part of his proof Varian [9] employed a graph- theoretic algorithm for computing the transitive closure of the binary relation. Their proofs also contained an inductive argument and were complex and lengthy. Fostel, Scarf and Todd [7] provided two elegant proofs of Afriat’s theorem. The first is an induction method and also implicitly uses a structure similar to our graph . Their second proof makes use of the duality theorem from linear programming. Teo and Vohra [8] explored explicitly the network structure inherent in the Afriat inequalities and presented a concise and construc- tive graph-theoretic proof by applying a fundamental theorem from graph theory. In the current paper we identify a common property —equivalence classes—used explicitly or implicitly in the five previous papers, and make full use of it. In par- ticular, we simplify their approaches by decomposing 1G ,,, into strongly connected components and taking a topological ordering of the components as 12 HH * , from which we can check whether ob- served data are consistent with GARP, and if consistent, we can compute feasible i for . This re- quires 1, ,im 2 Om 3 Om * time, and hence we can test the consis- tency with GARP faster than the time proposed by Teo and Vohra [8], while computing i for 1, ,imrequires 3 Om time shortest path computa- tion. In summary, our proof is similar to Teo and Vohra [8] and is also closely related to Afriat [1], Diewert [6], Var- ian [9], and Fostel, Scarf and Todd [7]. Here we have made the argument more transparent and more accessible without assuming the reader’s familiarity with any fun- damental mathematical result. In our argument, the ex- plicit use of the decomposition into strongly connected components plays an important role in helping reveal more detailed and more subtle structures of the graph 1G and simplify the proof considerably. Of course, the very elementary, intuitive and simple proof of Af- riat’s theorem is merely a byproduct of the current paper whose purpose has been to extend the theory to the equally important and more practical environments in which commodities are inherently indivisible or are available only in discrete quantities. 5. Acknowledgements This research was done while Z.Yang was visiting the Research Institute for Mathematical Sciences (RIMS), Kyoto University, Japan, in December 2011. This author wishes to thank RIMS for its hospitality and financial support. We are also grateful to Ian Crawford, Frederic Vermeulen, and Rakesh Vohra for their useful feedback. After this paper was circulated, we heard from John Quah that h e and Matthew Po isson had a note “Discrete - ness, separability, and revealed preference” (2012) ex- amining a similar problem via a different approach. REFERENCES [1] S. N. Afriat, “The Construction of a Utility Function from Expenditure Data,” International Economic Review, Vol. 8, No. 1, 1967, pp. 67-77. doi:10.2307/2525382 [2] R. W. Blundell, M. Browning and I. Crawford, “Non- parametric Engel Curves and Revealed Preference,” Eco- nometrica, Vol. 71, No. 1, 2003, pp. 205-240. doi:10.1111/1468-0262.00394 [3] M. Browning and P. A. Chiappori, “Efficient Intrahouse- hold Allocations: A General Characterization and Em- pirical Tests,” Econometrica, Vol. 66, No. 6, 1998, pp. 1241-1278. doi:10.2307/2999616 Copyright © 2012 SciRes. ME
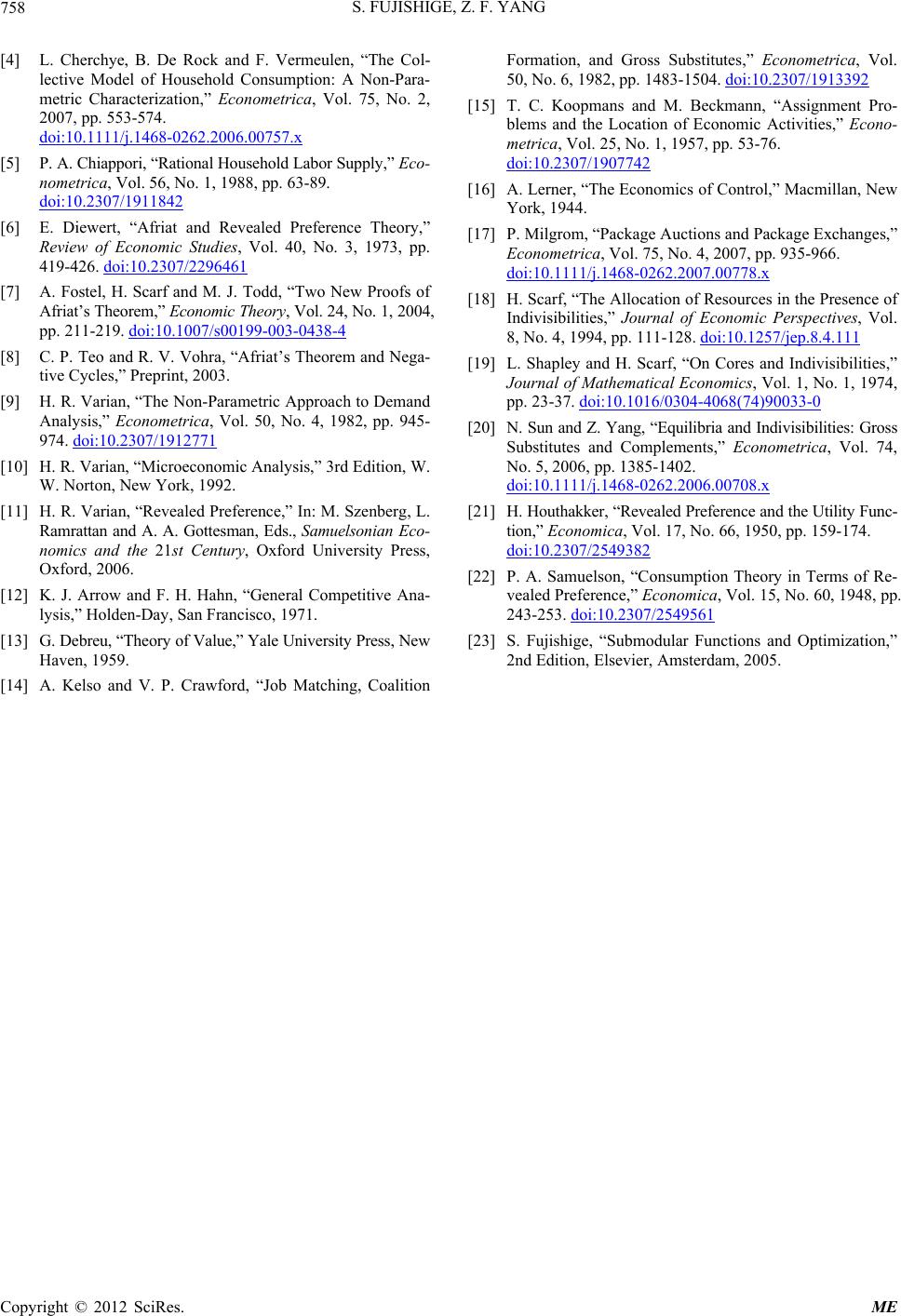 S. FUJISHIGE, Z. F. YANG Copyright © 2012 SciRes. ME 758 [4] L. Cherchye, B. De Rock and F. Vermeulen, “The Col- lective Model of Household Consumption: A Non-Para- metric Characterization,” Econometrica, Vol. 75, No. 2, 2007, pp. 553-574. doi:10.1111/j.1468-0262.2006.00757.x [5] P. A. Chiappori, “Rational Household Labor Sup ply ,” Eco- nometrica, Vol. 56, No. 1, 1988, pp. 63-89. doi:10.2307/1911842 [6] E. Diewert, “Afriat and Revealed Preference Theory,” Review of Economic Studies, Vol. 40, No. 3, 1973, pp. 419-426. doi:10.2307/2296461 [7] A. Fostel, H. Scarf and M. J. Todd, “Two New Proofs of Afriat’s Theorem,” Economic Theory, Vol. 24, No. 1, 20 04, pp. 211-219. doi:10.1007/s00199-003-0438-4 [8] C. P. Teo and R. V. Vohra, “Afriat’s Theorem and Nega- tive Cycles,” Preprint, 2003. [9] H. R. Varian, “The Non-Parametric Approach to Demand Analysis,” Econometrica, Vol. 50, No. 4, 1982, pp. 945- 974. doi:10.2307/1912771 [10] H. R. Varian, “Microeconomic Analysis,” 3rd Edition, W. W. Norton, New York, 1992. [11] H. R. Varian, “Revealed Preference,” In: M. Szenberg, L. Ramrattan and A. A. Gottesman, Eds., Samuelsonian Eco- nomics and the 21st Century, Oxford University Press, Oxford, 2006. [12] K. J. Arrow and F. H. Hahn, “General Competitive Ana- lysis,” Holden-Day, San Francisco, 1971. [13] G. Debreu, “The ory of Value,” Y ale Unive rsity Pr ess, Ne w Haven, 1959. [14] A. Kelso and V. P. Crawford, “Job Matching, Coalition Formation, and Gross Substitutes,” Econometrica, Vol. 50, No. 6, 1982, pp. 1483-1504. doi:10.2307/1913392 [15] T. C. Koopmans and M. Beckmann, “Assignment Pro- blems and the Location of Economic Activities,” Econo- metrica, Vol. 25, No. 1, 1957, pp. 53-76. doi:10.2307/1907742 [16] A. Lerner, “The Economics of Control,” Macmillan, New York, 1944. [17] P. Milgrom, “Package Auctions and Package Exchanges,” Econometrica, Vol. 75, No. 4, 2007, pp. 935-966. doi:10.1111/j.1468-0262.2007.00778.x [18] H. Scarf, “The Allocation of Resources in the Presence of Indivisibilities,” Journal of Economic Perspectives, Vol. 8, No. 4, 1994, pp. 111-128. doi:10.1257/jep.8.4.111 [19] L. Shapley and H. Scarf, “On Cores and Indivisibilities,” Journal of Mathematical Economics, Vol. 1, No. 1, 1974, pp. 23-37. doi:10.1016/0304-4068(74)90033-0 [20] N. Sun and Z. Yang, “Equilibria and Indivisibilities: Gross Substitutes and Complements,” Econometrica, Vol. 74, No. 5, 2006, pp. 1385-1402. doi:10.1111/j.1468-0262.2006.00708.x [21] H. Houthakker, “Revealed Preference and the Utility Func- tion,” Economica, Vol. 17, No. 66, 1950, pp. 159-174. doi:10.2307/2549382 [22] P. A. Samuelson, “Consumption Theory in Terms of Re- vealed Preference,” Economica, Vol. 15, No. 60, 1948, pp. 243-253. doi:10.2307/2549561 [23] S. Fujishige, “Submodular Functions and Optimization,” 2nd Edition, Elsevier, Amsterdam, 2005.
|