 Journal of Transportation Technologies, 2012, 2, 285-296 http://dx.doi.org/10.4236/jtts.2012.24031 Published Online October 2012 (http://www.SciRP.org/journal/jtts) Temporal Stability and Transferability of Non-Motorized and Total Trip Generation Models Judith L. Mwakalonge1*, Juhann C. Waller2, Judy A. Perkins3 1Department of Civil and Mechanical Engineering and Technology, South Carolina State University, Orangeburg, USA 2Department of Civil and Environmental Engineering, North Carolina A & T State University, Greensboro, USA 3Department of Civil and Environmental Engineering, Prairie View A & M University, Prairie View, USA Email: *jmwakalo@scsu.edu, juhann.waller@covington-waller.com, juperkins@pvamu.edu Received June 23, 2012; revised July 21, 2012; accepted August 17, 2012 ABSTRACT Transportation systems provide a means for moving people and the goods from which they are spatially separated. Of the two means of surface transportation, the motorized mode is used extensively for utilitarian travel in developed countries. The increasing reliance on motorized travel has contributed to increased traffic congestion, air pollution, and greenhouse emissions. Non-motorized travel has recently received significant attention as a means to reduce congestion and environmental problems and improve human health. However, non-motorized modeling is generally underde- veloped. This study investigated some changes in non-motorized and total travel and the characteristics of the traveling public in 1990, 1995, 2001, and 2009 using a national travel survey. The study also investigated the temporal transfer- ability of linear-regression trip generation models for non-motorized and total travel under such changes. High-income households made fewer non-motorized trips in 1990 and 1995 compared to 2001 and 2009. Persons aged 50 and over showed an increased demand for non-motorized travel, whereas children aged 0 - 15 showed a decreasing preference for non-motorized travel over time. Regarding temporal stability, only the coefficient for single-adult households with no children was stable across all of the analysis years. For both non-motorized and total travel, most model parameter estimates were stable short term but not long term. In general, the total travel models transferred better than non-mo- torized models, both short term and long term. Despite not finding universal stability in model parameter estimates, the models were marginally able to replicate travel in 2009 relative to the locally estimated 2009 model. Keywords: Non-Motorized; Transferability; Temporal; Total Travel 1. Introduction Transportation systems provide a means for moving peo- ple and the goods from which they are spatially sepa- rated. There are two means of transportation: motorized and non-motorized. The motorized segment is mostly comprised of passenger and freight vehicles that are used extensively for utilitarian travel in developed countries. The non-motorized travel segment is mostly comprised of bicycling and walking, which are not typically used extensively as means for utilitarian travel in developed countries. In the US, the use of motorized vehicles as a means of transportation has been dominant for years and has been associated with the sprawling land use patterns in most US cities as well as the relatively low cost of operating motorized vehicles and nominal parking costs [1,2]. The increasing reliance on motorized means of transportation has contributed to increased traffic con- gestion, air pollution, and greenhouse emissions. The Urban Transportation Report Card [3] reports that trans- portation is responsible for 20 to 60 percent of the carbon emissions in major US cities. Additionally, the Urban Mobility Report [4] shows that the peak congested hours increased from 4.5 hours per day in 1982 to 7.1 hours per day in 2002. The travel time index, which is defined as the ratio of travel time in rush hour to the travel time during the free-flow period increased from 1.09 in 1982 to 1.24 in 2007, and wasted fuel per peak traveler in- creased by 15 gallons during the same period [5]. Fur- thermore, the report indicates that in 2002, 58 percent of all major road systems were congested, compared to only 34 percent in 1982. The World Health Organization (WHO) at the European Region (1999) also reported that auto- generated pollution is responsible for more deaths than all traffic accidents. Consequently, more agencies are seeking or implementing strategies to reduce reliance on motorized travel. *Corresponding author. C opyright © 2012 SciRes. JTTs
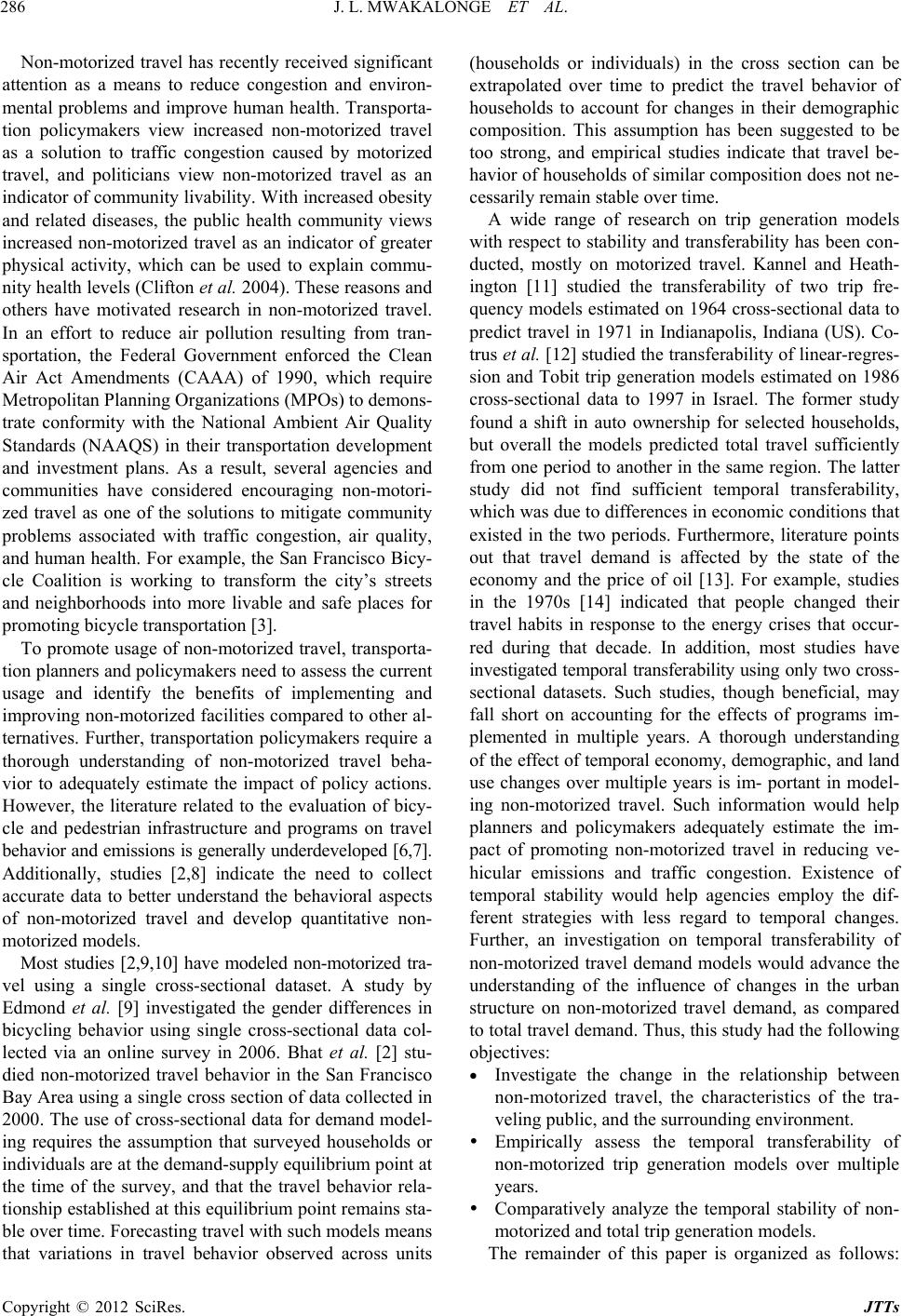 J. L. MWAKALONGE ET AL. 286 Non-motorized travel has recently received significant attention as a means to reduce congestion and environ- mental problems and improve human health. Transporta- tion policymakers view increased non-motorized travel as a solution to traffic congestion caused by motorized travel, and politicians view non-motorized travel as an indicator of community livability. With increased obesity and related diseases, the public health community views increased non-motorized travel as an indicator of greater physical activity, which can be used to explain commu- nity health levels (Clifton et al. 2004). These reasons and others have motivated research in non-motorized travel. In an effort to reduce air pollution resulting from tran- sportation, the Federal Government enforced the Clean Air Act Amendments (CAAA) of 1990, which require Metropolitan Planning Organizations (MPOs) to demons- trate conformity with the National Ambient Air Quality Standards (NAAQS) in their transportation development and investment plans. As a result, several agencies and communities have considered encouraging non-motori- zed travel as one of the solutions to mitigate community problems associated with traffic congestion, air quality, and human health. For example, the San Francisco Bicy- cle Coalition is working to transform the city’s streets and neighborhoods into more livable and safe places for promoting bicycle transportation [3]. To promote usage of non-motorized travel, transporta- tion planners and policymakers need to assess the current usage and identify the benefits of implementing and improving non-motorized facilities compared to other al- ternatives. Further, transportation policymakers require a thorough understanding of non-motorized travel beha- vior to adequately estimate the impact of policy actions. However, the literature related to the evaluation of bicy- cle and pedestrian infrastructure and programs on travel behavior and emissions is generally underdeveloped [6,7]. Additionally, studies [2,8] indicate the need to collect accurate data to better understand the behavioral aspects of non-motorized travel and develop quantitative non- motorized models. Most studies [2,9,10] have modeled non-motorized tra- vel using a single cross-sectional dataset. A study by Edmond et al. [9] investigated the gender differences in bicycling behavior using single cross-sectional data col- lected via an online survey in 2006. Bhat et al. [2] stu- died non-motorized travel behavior in the San Francisco Bay Area using a single cross section of data collected in 2000. The use of cross-sectional data for demand model- ing requires the assumption that surveyed households or individuals are at the demand-supply equilibrium point at the time of the survey, and that the travel behavior rela- tionship established at this equilibrium point remains sta- ble over time. Forecasting travel with such models means that variations in travel behavior observed across units (households or individuals) in the cross section can be extrapolated over time to predict the travel behavior of households to account for changes in their demographic composition. This assumption has been suggested to be too strong, and empirical studies indicate that travel be- havior of households of similar composition does not ne- cessarily remain stable over time. A wide range of research on trip generation models with respect to stability and transferability has been con- ducted, mostly on motorized travel. Kannel and Heath- ington [11] studied the transferability of two trip fre- quency models estimated on 1964 cross-sectional data to predict travel in 1971 in Indianapolis, Indiana (US). Co- trus et al. [12] studied the transferability of linear-regres- sion and Tobit trip generation models estimated on 1986 cross-sectional data to 1997 in Israel. The former study found a shift in auto ownership for selected households, but overall the models predicted total travel sufficiently from one period to another in the same region. The latter study did not find sufficient temporal transferability, which was due to differences in economic conditions that existed in the two periods. Furthermore, literature points out that travel demand is affected by the state of the economy and the price of oil [13]. For example, studies in the 1970s [14] indicated that people changed their travel habits in response to the energy crises that occur- red during that decade. In addition, most studies have investigated temporal transferability using only two cross- sectional datasets. Such studies, though beneficial, may fall short on accounting for the effects of programs im- plemented in multiple years. A thorough understanding of the effect of temporal economy, demographic, and land use changes over multiple years is im- portant in model- ing non-motorized travel. Such information would help planners and policymakers adequately estimate the im- pact of promoting non-motorized travel in reducing ve- hicular emissions and traffic congestion. Existence of temporal stability would help agencies employ the dif- ferent strategies with less regard to temporal changes. Further, an investigation on temporal transferability of non-motorized travel demand models would advance the understanding of the influence of changes in the urban structure on non-motorized travel demand, as compared to total travel demand. Thus, this study had the following objectives: Investigate the change in the relationship between non-motorized travel, the characteristics of the tra- veling public, and the surrounding environment. Empirically assess the temporal transferability of non-motorized trip generation models over multiple years. Comparatively analyze the temporal stability of non- motorized and total trip generation models. The remainder of this paper is organized as follows: Copyright © 2012 SciRes. JTTs
 J. L. MWAKALONGE ET AL. Copyright © 2012 SciRes. JTTs 287 First is a discussion of the data used in this study, fol- lowed by a descriptive analysis of selected variables as related to non-motorized travel. The model specification is then presented. Finally, the results are presented and discussed, followed by conclusions and recommenda- tions for future studies. 2. Methodology 2.1. Data The first set of data used consisted of the 1990 and 1995 National Person Travel Survey (NPTS), which was a one-day travel survey conducted by the Research Trian- gle Institute. The 1990 NPTS was conducted between March 1990 and March 1991 with 21,869 households and yielded a response rate of 84 percent. The 1995 NPTS was a telephone survey conducted between May 1995 and July 1996 that collected travel information from more than 42,000 households. The second set of data used included the National Household Travel Sur- vey (NHTS) from 2001 and 2009. The 2001 NHTS was a one-day travel survey that was collected via a telephone survey by Westat and Morpace from March 2001 to May 2002 and collected travel information from 69,817 households. The 2009 NHTS collected travel information from more than 150,000 households. Both the 2001 and 2009 NHTS included travel information from household members aged 0 - 4, which was not done in the previous surveys. For consistency throughout the analysis, only the travel information that was common from all data sets was used in this study. Further, the study used travel information from households residing within the metro- politan statistics areas (MSAs). The unit of analysis in this study was a household since it is believed that most decisions are made at a household level [15]. Therefore, the final sample sizes used in this study were 12,494, 29,585, 50,682, and 109,321 for 1990, 1995, 2001, and 2009, respectively. The details of each of these datasets are well documented on the NHTS website [16]. 2.2. Descriptive Analysis Table 1 shows the changes in travel and selected socio- economic variables known to influence travel from 1990 to 2009. In all analysis years, the percentage of walk trips was higher than the percentage of bicycle trips. This may be attributed to the fact that everyone becomes a pede- strian at some point throughout the day, but the same cannot be said about becoming a cyclist. The higher walk trips may also be attributed to increased walks to gain transit access [9]. The percentage of motorized trips showed a marginal decrease, while non-motorized trips increased marginally from 1990 to 2009. The number of workers per household was lower in 2009, but the number of ve- hicles per worker was higher in this same year. This may be explained by the economic recession that was ongoing during data collection, which affected the labor market. The household size, average trip length, and average daily household trips decreased slightly from 2001 to 2009. 2.3. Distribution of Non-Motorized Trips by Age and Income Groups Non-motorized travel involves exertion of physical en- ergy; therefore, it is expected that the age of the indivi- dual is likely to influence his or her decision to use non- motorized modes. Likewise, income may indicate the ability of a family to own, operate, and maintain a vehi- cle. Therefore, non-motorized trips were cross-tabulated with age and income groups, and the frequencies of trips made by each age and income group are shown in Figure 1. In general, all else being equal, there was an opposite change in the non-motorized travel pattern for persons Table 1. Changes in trip and selected demographic characteristics. Percent of non-motorized trips Year Bike Walk Percent of motorized trips Household size Vehicles per driver Workers per household Vehicles per worker Average trip length (miles) Household trips per day 9.6 90.4 1990 7.46 92.54 2.63 1.11 1.17 1.48 9.47 6.91 12.8 87.2 1995 6.07 93.93 2.59 1.01 1.32 1.31 9.13 9.62 9.3 90.7 2001 8.87 91.13 2.60 1.06 1.37 1.36 10.04 9.56 8.6 91.4 2009 9.47 90.53 2.58 1.06 1.11 1.69 9.33 8.56
 J. L. MWAKALONGE ET AL. 288 (a) Tri p- r at e Di st r i but i on by Househol d Inc ome Category 0 0. 2 0. 4 0. 6 0. 8 1 1. 2 1. 4 1. 6 1. 8 2 1234567891011 1213 141516 17 income Cate gory trip rate 2009 2001 1995 1990 (b) Figure 1. Non-motorized household trip rate by age (a) and income (b). Note: 1 ≤ $5000, 2 = $5000 - $9999, 3 = $10,000 - $14,999, 4 = $15,000 - $19,999, 5 = $20,000 - $24,999, 6 = $25,000 - $29,999, 7 = $30,000 - $34,999, 8 = $35,000 - $39,999, 9 = $40,000 - $44,999, 10 = $45,000 - $49,999, 11 = $50,000 - $54,999, 12 = $55,000 - $59,999, 13 = $60,000 - $64,999, 14 = $65,000 - $69,999, 15 = $70,000 - $74,999, 16 = $75,000 - $79,999, 17 ≥ $80,000. aged 39 or less and persons aged 40 or more. Specifically, non-motorized trips made by the younger group gene- rally decreased over time, while non-motorized trips of the older group increased. This may be explained in part by the increased reliance on using private automobiles, rather than walking and bicycling, for making school trips, as reported in Ulfarsson and Shankar [17]. Persons aged 15 or less made more non-motorized trips than any other age group in 1990, 1995, and 2001, but this trend changed in 2009, when persons aged 50 to 59 outpaced the >15 group by more than 5 percent. Consistent with other studies, low-income households made more non- motorized trips compared to other income groups. How- ever, the high-income households showed a monotonic increase in making non-motorized trips over time. 2.4. Distribution of Non-Motorized Trips by Lifecycle Lifecycle defines the household composition and the extent of responsibilities a given household encounters. In general, it is expected that households with children make more trips than their counterparts. Figure 2 shows the distribution of non-motorized trips for each lifecycle category, as defined in Table 2. Households with two or more adults and children aged 6 to 15 had the highest percentage of non-motorized trips in 1990 but showed a decreasing trend over time. This may be explained by the relative independence of children aged 6 to 15 and thus the likelihood of them bicycling or walking to school or to playgrounds and/or parks near their homes [2]. It is apparent from Figure 2 that single-parent households made relatively fewer non-motorized trips than dual- parent households. All else being equal, households with retired persons showed a monotonic increase in non- motorized travel. Considering the increase in the aging population, this may indicate the need for bicycling and walking facilities for communities with higher popula- tions of retired persons. 2.5. Model Specification Several variables contained within the four cross-sec- tional datasets are known to influence travel behavior. Thus, an analysis was performed to identify which vari- ables have a high influence on non-motorized and total travel, thus specifying a simple but robust model. After performing an analysis of variance and correlation ratio, Trip Dis t r ibut ion by Life cyc l e 0 5 10 15 20 25 30 35 12345678910 Life cycle cate go ry percent 2009 2001 1995 1990 (a) Trip - Rat e Di str ibution by Life cyc l e 0.0000 0.5000 1.0000 1.5000 2.0000 2.5000 12345678910 l i fecycl e trip-r at e 2009 2001 1995 1990 (b) Figure 2. Non-Motorized trip distribution by household life- cycle. Copyright © 2012 SciRes. JTTs
 J. L. MWAKALONGE ET AL. 289 Table 2. Description of lifecycle codes. Lifecycle Code Code Description 1 1 adult, no children 2 2 1 2 2 2 2 + adults, no children 3 adult, youngest child 0 - 5 4 + adults, youngest child 0 - 5 5 1 adult, youngest child 6 - 15 6 + adults, youngest child 6 - 15 7 1 adult, youngest child 16 - 21 8 + adults, youngest child 16 - 21 9 1 adult, retired, no children 10 + adults, retired, no children the lifecycle of the househd (lif_cyc), household size where xes household observations in period t, ehold i in pe- rio ol (hhsize), household income (income), number of workers (nworkers), number of licensed persons in a household (ndrivers), and number of vehicles (nvehicles) available for use by the household were specified in the final model. Though there were ten lifecycle categories, as previously defined in Table 2, only six categories were specified in the model since the other categories were statistically insignificant. Further analysis indicated that the model with log transformed household size (loghh- size) yielded a superior explanatory power than other specifications; thus, only the results for this specification are presented here. The formulated trip generation mod- els took the following form: K 1 it titk tkit k yαxβε i inde k indexes the household characteristics, it y is the number of trips made by hous d t, itk is the kth household characteristic of household i in constant term for period t, period t, t α is the tk is the kth coefficient of the kth explanatory variable in om term for household i in period t. pa- ra travel was identi- hown in Table 3, the increase in auto ownership im tal demand, the specified model yield- ed period t, and it ε is the rand The least squares estimator of the vector of model meters for each period/cross section t is given by –1 , years1,2,3,4. TT ttt tt βXXXY t 3. Results and Discussion 3.1. Model Estimation Output The best-fit model for non-motorized fied using the 1990 cross-sectional dataset as the base year. Similarly, for total travel, a less complex model with high explanatory power was estimated using the 1990 dataset. Thereafter, the same specification was es- timated using Stata 9.0 for each of the other three cross- sectional datasets. The model estimation results are pre- sented in Table 3. Table 3 clearly shows that the de- mand for non-motorized and total travel increased with the increase in household size and the number of workers in a household, as indicated by the positive sign of their coefficients. This finding is consistent in all analyzed years. Similar findings have been reported in other stu- dies [1,2]. With respect to household lifecycle, single- adult households with either children aged 6 - 15 or no children made more non-motorized trips than their two- adult household counterparts. In fact, single-person house- holds made more non-motorized trips than all other house- hold categories. Households with younger children aged 0 - 5 relied on other modes of transportation than bicy- cling and walking, as indicated by the negative sign of their coefficient. In 1990 and 1995, demand for non- motorized travel declined with the increase in household income, as shown previously in Figure 1, and this is re- flected by the negative sign of the income coefficient shown in Table 3. As shown in Figure 1, in recent years, demand for non-motorized travel has gotten higher for less affluent and affluent households but lower for me- dium-income households. This is further reflected by the change in sign and increase in magnitude for the income coefficient. For all analysis years, with the exception of 2009, total travel demand increased with the increase in household income, and this trend increased over time, as reflected by the increase in the magnitude of its coeffi- cient. As s pacted the demand for non-motorized travel negatively. The associated negative sign of the coefficient suggests that households with a higher number of vehicles have a lower propensity to take walking or bicycling trips. The increase in the number of vehicles per driver, as shown in Table 1, reduced the demand for non-motorized travel, which is further indicated by the change in sign of the coefficient for the number of licensed persons in a household. In contrast, the number of drivers affected the total travel demand positively, but the magnitude of its effect diminished over time, as reflected by a decrease in the magnitude of the coefficient for the number of drivers. The exogenous variables specified in the non-motorized demand model yielded more explanatory power in 1990 and less in 2001. However, for to more explanatory power in 2001 and less in 1995. It is worth noting that the low explanatory power exhibited by the non-motorized model is consistent with what was encountered in the literature, indicating that the variables Copyright © 2012 SciRes. JTTs
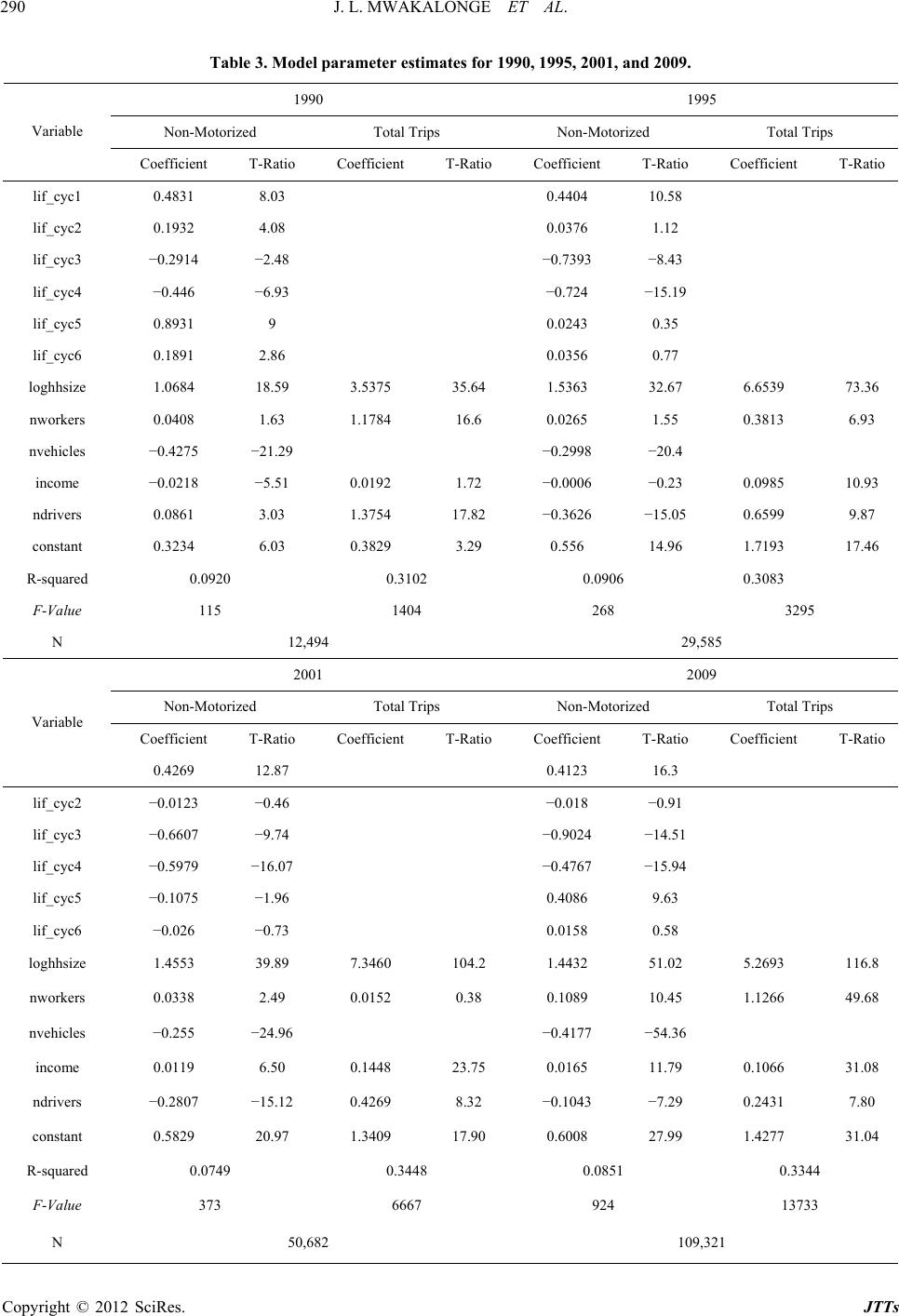 J. L. MWAKALONGE ET AL. Copyright © 2012 SciRes. JTTs 290 tesor 1990, 1995, 2001, and 2009. 1990 1995 Table 3. Model parameter estima f Non-Motorized Total Trips Non-Motorized Total Trips Variable CoefCoeffiT-Ratio Coeffficient T-Ratio cient ficient T-Ratio Coeficient T-Ratio lif_cyc1 0.4831 8.03 0.4404 10.58 lif_cyc2 0.1932 4.08 0.0376 1.12 lif_cyc3 −0.2914 −2.48 −0.7393 −8.43 lif_cyc4 −0.446 −6.93 −0.724 −15.19 lif_cyc5 0.8931 9 0.0243 0.35 lif_cyc6 0.1891 2 loghhsize 3.5375 35.6539 73. − −−− 0192 1.0985 10. 17. − 0920 3102 0906 295 12,494 29,585 .86 0.0356 0.77 1.0684 18.59 64 1.5363 32.67 6.36 nworkers 0.0408 1.63 1.1784 16.6 0.0265 1.55 0.3813 6.93 nvehicles 0.4275 21.29 0.2998 20.4 income −0.0218 −5.51 0.72 −0.0006 −0.23 0.93 ndrivers 0.0861 3.03 1.3754 82 −0.3626 15.05 0.6599 9.87 constant 0.3234 6.03 0.3829 3.29 0.556 14.96 1.7193 17.46 R-squared 0.0.0.0.3083 F-Value 115 1404 268 3 N 2001 2009 Non-Motorized Total Trips Non-Motorized Total Trips Coatio CoeffCoRatio Coeff-Ratioefficient T-Ricient T-Ratioefficient T-icient T Variable lif_cyc2 − − 0.4269 12.87 0.4123 16.3 0.0123 −0.46 −0.018 0.91 lif_cyc3 −0.6607 −9.74 −− loghhsize 7.3460 104.2 1.2693 116.8 −−− 0.1448 23.1066 31. − −− 17. R-0749 0.3448 0851 0.3344 N 50,682 109,321 0.9024 14.51 lif_cyc4 −0.5979 −16.07 −0.4767 −15.94 lif_cyc5 −0.1075 −1.96 0.4086 9.63 lif_cyc6 −0.026 −0.73 0.0158 0.58 1.4553 39.89 4432 51.02 5. nworkers 0.0338 2.49 0.0152 0.38 0.1089 10.45 1.1266 49.68 nvehicles −0.255 24.96 0.4177 54.36 income 0.0119 6.50 75 0.0165 11.79 0.08 ndrivers 0.2807 15.12 0.4269 8.32 0.1043 −7.29 0.2431 7.80 constant 0.5829 20.97 1.3409 90 0.6008 27.99 1.4277 31.04 squared 0.0. F-Value 373 6667 924 13733
 J. L. MWAKALONGE ET AL. 291 specifn the model provided littleation of the variation in non-motorized household trips. This reflects bility of Model Coefficients e ied i explan the difficulty in explaining non-motorized travel with con- ventional variables. 3.2. Temporal Sta As noted in the introduction, the collection and use of cross-sectional models relies on the assumption that th population is at an equilibrium point with respect to travel behavior and that the estimated model parameters can be applied to a future context. This assumption im- plies that the estimated model parameters are temporally invariant. To test temporal stability of estimated model parameters for both non-motorized and total travel, the 1990 cross-sectional model was tested against the more recent datasets; the same was done with the 1995 and 2001 models. Testing was done using the student t-test defined below: 1 1 var var T+ T T+ T ββ t β where 1T+ and T gn y ly, are the coefficients for a given variable e desiear model (T+1) and base year model (T from th ), respective and 1 var T+ β and var T are their respective variances. The resulting t-stati hown 4. In the 1990 model, the only co stics are sin Table efficient that was statistically st ation and application periods is Table 4. T-s From 1990 1995 2001 able at a 5 percent significance level was a dummy variable for single-person household with no children; this coefficient was invariant across all analysis years. In the 1995 model, the coefficients for lifecycles 1 through 3 and 6, logarithm of household size, and the constant term were temporally stable, whereas the other variables were either stable in 2001 or 2009 or not stable in 2001 and 2009. In the short-term temporal stability check from 2001 to 2009, not all of the model parameters were stable. Table 4 shows that six of the 2001 model parameters were stable at a 5 percent significance level. For total travel demand, only the constant term showed stability from 2001 to 2009. The difference in the vector of model parameter esti- mates for the estim tatistics. To 1995 2009 2001 2009 2001 2009 For Non-Morips torized T lif_cyc1 −0.58 −0.82 −0.25 −0.58 −0.35 −1.08 lif_cyc2 −2.68 −3.78 −4.12 −1.16 −1.43 −0.17 lif_cyc3 −3.06 −2.72 −4.60 0.71 −1.52 −2.63 lif_cyc4 −3.47 −2.04 −0.43 2.09 4.39 2.54 lif_cyc5 −7.15 −8.82 −4.49 −1.48 4.69 7.44 lif_cyc6 −1.90 −2.86 −2.42 −1.05 −0.37 0.93 loghhsize − − − For Ts 6.30 5.68 5.85 −1.36 −1.70 −0.26 nworkers −0.47 −0.25 2.52 0.33 4.13 4.40 nvehicles 5.13 7.66 0.46 2.50 −7.12 12.72 income 4.51 7.74 9.13 4.00 5.88 1.95 ndrivers 12.05 10.81 −5.99 2.69 9.22 7.52 constant 3.56 4.30 4.80 0.58 1.04 0.51 otal Trip loghhsize 23.18 31.28 6.02 −13.67 −24.81 15.88 nworkers −27.81 −32.93 −23.68 − α = 0.05 Critical value is ± 1.96 67.01−59.12 24.24 income −34.51 −34.12 −34.55 −71.60 −72.13 −5.45 ndrivers −24.04 −27.84 −31.67 −59.75 −66.84 −3.06 constant −13.00 −17.67 −19.29 −45.16 −51.39 0.99 Copyright © 2012 SciRes. JTTs
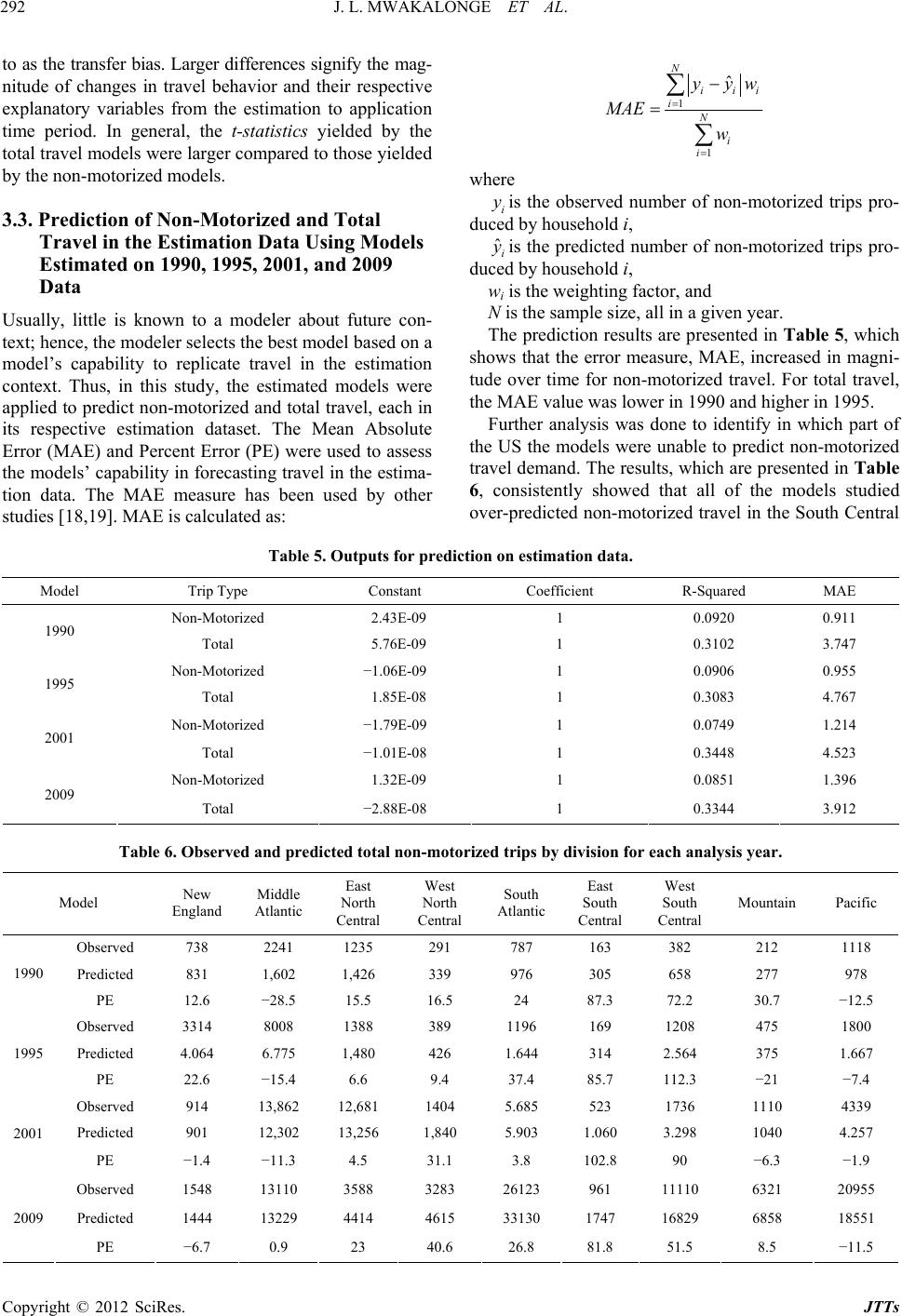 J. L. MWAKALONGE ET AL. 292 to as the transfer bias. Lfferences mag- nitudeges in travel behavior and their respective n Data Using Models Usua text; a modeability to replicate travel in the estimation arger disignify the of chan explanatory variables from the estimation to application time period. In general, the t-statistics yielded by the total travel models were larger compared to those yielded by the non-motorized models. 3.3. Prediction of Non-Motorized and Total Travel in the Estimatio Estimated on 1990, 1995, 2001, and 2009 Data lly, little is known to a modeler about future con- hence, the modeler selects the best model based on l’s cap context. Thus, in this study, the estimated models were applied to predict non-motorized and total travel, each in its respective estimation dataset. The Mean Absolute Error (MAE) and Percent Error (PE) were used to assess the models’ capability in forecasting travel in the estima- tion data. The MAE measure has been used by other studies [18,19]. MAE is calculated as: 1 1 ˆ N iii i N i i yyw MAE w where is the observed number of non-motorized trips pro- by household i, the predicted number of non-motorized trips pro- ctor, and N s are presented in Table 5, which sh increased in magni- tuor total travel, th le 6, ic Constant i y duced ˆi yis duced by household i, wi is the weighting fa is the sample size, all in a given year. The prediction result ows that the error measure, MAE, de over time for non-motorized travel. F e MAE value was lower in 1990 and higher in 1995. Further analysis was done to identify in which part of the US the models were unable to predict non-motorized travel demand. The results, which are presented in Tab consistently showed that all of the models studied over-predicted non-motorized travel in the South Central tion on estimation data. Coefficient R-Squared MAE Table 5. Outputs for pred Model Trip Type Non-Motorized0.0920 0.911 2.43E-09 1 1990 Total 5.76E-09 1 0.3102 3.747 Non-Motorized −1.06E-09 1 0.0906 0.955 1995 Total 1.85E-08 1 0.3083 4.767 Non-Motorized −1.79E-09 1 0.0749 1.214 2001 Total −1.01E-08 1 0.3448 4.523 Non-Motorized 1.32E-09 1 0.0851 1.396 2009 Total −2.88E-08 1 0.3344 3.912 Table 6d predicted otorized trips byivision for each a year. . Observed antotal non-m dnalysis Model New Middle East South East Wes Pacific Observed 212 1118 England Atlantic North Central North Central Atlantic South Central South Central Mountain West t 738 2241 1235 291 787 163 382 Predicted 1990 Prd 1. 1995 Pr1 1 2001 Pr 3 12009 PE −6.7 0.9 23 40.6 26.8 81.8 51.5 8.5 −11.5 831 1,602 1,426 339 976 305 658 277 978 PE 12.6 −28.5 15.5 16.5 24 87.3 72.2 30.7 −12.5 Observed 3314 8008 1388 389 1196 169 1208 475 1800 edicte4.064 6.775 1,480 426 644314 2.564 375 1.667 PE 22.6 −15.4 6.6 9.4 37.4 85.7 112.3 −21 −7.4 Observed 914 13,862 12,681 1404 5.685 523 1736 1110 4339 edicted 901 12,302 3,256,8405.903 1.060 3.298 1040 4.257 PE −1.4 −11.3 4.5 31.1 3.8 102.8 90 −6.3 −1.9 Observed 1548 13110 3588 3283 26123 961 11110 6321 20955 edicted 1444 13229 44144615 31301747 6829 6858 18551 Copyright © 2012 SciRes. JTTs
 J. L. MWAKALONGE ET AL. 293 Divisionsnclutes lxas se redicn-md traell fo the Pacific Division, which includes CaliThe ckluster prmance of all the presenteddels i data appl scribex (TI) was used to , which ide staike Teand Tennes- ee. Thmodels pted nootorizevel w fornia. r la erfo mon predicting travel in the East South Central Division may be attributed to low sample representation compared to other divisions. The differences in the models’ ability to predict travel in the different divisions implies differ- ences in travel behavior exhibited by households in the respective divisions. Divisions with lower PE values may have the advantage of using the national travel survey datasets for understanding non-motorized travel patterns and behavior in their regions and updating their travel demand models. This may be further investigated by formulating models for each division. 3.4. Prediction of Non-Motorized and Total Travel in 2009 Using Models Estimated on 1990, 1995, and 2001 Data The models estimated on the 1990, 1995, and 2001 were used to predict non-motorized and total travel in the ication contexts. Along with the MAE value de- ed previously, the Transfer Ind measure how well the estimation context model predicted the observed trips in the application context relative to the application context model. Mathematically, TI is ex- pressed as [20]: 2 transferred 2 local R TI R The results are presented in Table 7, which illustrates that the magnitude of the resulting error measure from the application of the 1990 model to predict travel de- mand in the three periodased wime. T0 model better explainedmotorized travel at the houseeve1995or 20s indicatby the lvalue of the trr indexe 199el pectively. This was done to investi- ga - tant s increith the 199 non- than fhold ll for 01, aed arge ansfe. Th5 mod better explained trip variability for 2009 than 2001, even though the reverse would be expected since one would expect fewer changes in land use, household structure, and travel patterns in 2001 than in 2009. The 2001 model explained travel relatively well for the 2009 local model. With respect to total travel, all the transferred models explained trip variation less than the local models, as re- flected by their respective lower TI values. From Table 7, it is evident that the total travel models transferred better in time than the non-motorized models. It is worth noting that the MAE value yielded by the 2009 model’s applica- tion in estimation data was large compared to the trans- ferred models. This may be due in part to changes in variables explaining non-motorized travel that are not spe- cified in the model. Further, a comparison of the models’ ability to predict observed trips in 2009 by household size and household income was undertaken, and the results are depicted in Figures 3 and 4, res Model Application Trip Type Cons te whether modelers should model by market segmen- tation rather than the unified approach employed in this study. As observed in Figure 3, the relationship between non-motorized and total trip rate and household size for both 2009 observed trips and predicted trips by each- model was non-linear, with trip rate increasing monotoni- cally with increased household size. This was expected since the proportion of children in a household, who make fewer trips on average than adults, increased as house- hold size grew. In addition, the trip rate increase de- creased with the increase in household size, which was motorized travel in future contexts. Coefficient Transfer R2 MAE TI Table 7. Outputs for prediction of non Non-Motorized 0.1107 0.9247 0.0741 0.946 0.87 1995 4.881 0.92 Nd 0 2001 Non-zed 1990 2009 Non-zed 2001 Non-zed 1995 2009 Non-zed 2001 2009 Total 1.0893 1.1582 0.2833 on-Motorize 0.5223 0.7815 0.0555 1.106 .65 Total 0.7938 1.1551 0.3042 4.655 0.88 Motori 0.6447 0.9746 0.0684 1.237 0.80 Total 0.7369 1.0671 0.3213 3.910 0.96 Motori 0.3794 0.8882 0.0703 1.102 0.83 Total −0.4849 1.0208 0.3424 4.551 0.99 Motori 0.4776 1.0487 0.0733 1.238 0.86 Total −0.1068 0.8759 0.3253 4.203 0.97 Motori0.0036 1.1965 0.0800 1.331 0.94 Total 0.3501 0.8385 0.3182 4.209 0.95 Copyright © 2012 SciRes. JTTs
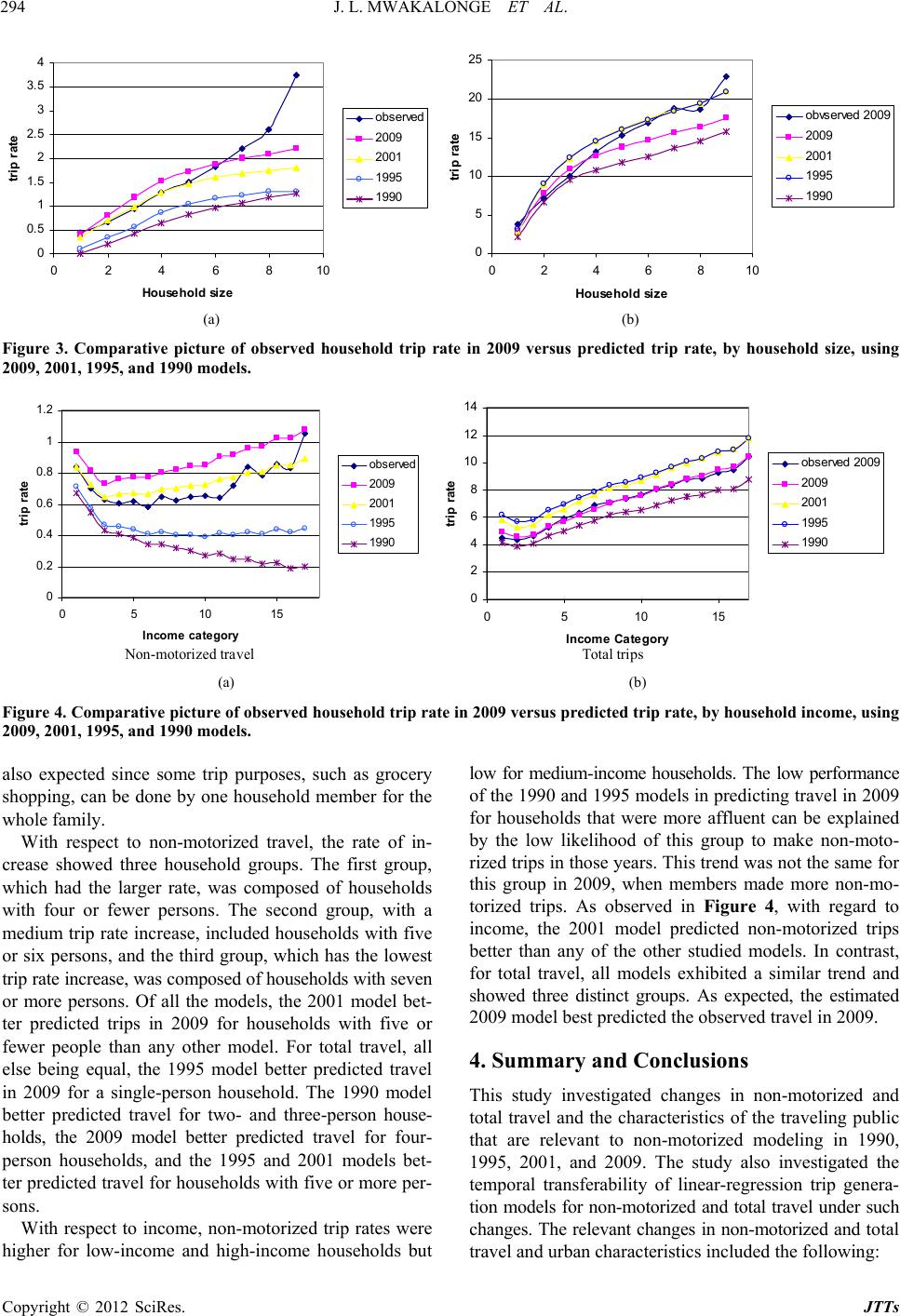 J. L. MWAKALONGE ET AL. 294 0 0. 5 1 1. 5 2 2. 5 3 3. 5 4 0246810 Household siz e trip r ate obs erved 2009 2001 1995 1990 0 5 10 15 te 20 25 02468 10 Househol d size trip ra obvser9ved 200 2009 2001 1995 1990 (a) (b) Figure 3. Comparative picture of observed household trip rate in 2009 versus predicted trip rate, by household size, using 2009, 2001, 1995, and 1990 models. 0 0. 2 0. 4 0. 6 0. 8 1 1. 2 051015 Income category trip rate obs erved 2009 2001 1995 1990 on-motorized travel 0 2 4 6 8 10 12 14 051015 Income Category tri p rate obs erved 2009 2009 2001 1995 1990 Total trips (a) (b) Figure 4. Comparative picture of observed household trip rate in 2009 versus predicted trip rate, by household income, using 2009, 2001, 1995, and 1990 models. also expected since some tr With respect to non-motorized travel, the rate of in- ewer persons. The second group, with a m higher for low-income and high-income households but seholds. The low performance by the low likelihood of this group to make non-moto- l travel and urban characteristics included the following: ip purposes, such as grocery low for medium-income hou shopping, can be done by one household member for the whole family. of the 1990 and 1995 models in predicting travel in 2009 for households that were more affluent can be explained crease showed three household groups. The first group, which had the larger rate, was composed of households with four or f edium trip rate increase, included households with five or six persons, and the third group, which has the lowest trip rate increase, was composed of households with seven or more persons. Of all the models, the 2001 model bet- ter predicted trips in 2009 for households with five or fewer people than any other model. For total travel, all else being equal, the 1995 model better predicted travel in 2009 for a single-person household. The 1990 model better predicted travel for two- and three-person house- holds, the 2009 model better predicted travel for four- person households, and the 1995 and 2001 models bet- ter predicted travel for households with five or more per- sons. With respect to income, non-motorized trip rates were rized trips in those years. This trend was not the same for this group in 2009, when members made more non-mo- torized trips. As observed in Figure 4, with regard to income, the 2001 model predicted non-motorized trips better than any of the other studied models. In contrast, for total travel, all models exhibited a similar trend and showed three distinct groups. As expected, the estimated 2009 model best predicted the observed travel in 2009. 4. Summary and Conclusions This study investigated changes in non-motorized and total travel and the characteristics of the traveling public that are relevant to non-motorized modeling in 1990, 1995, 2001, and 2009. The study also investigated the temporal transferability of linear-regression trip genera- tion models for non-motorized and total travel under such changes. The relevant changes in non-motorized and tota Copyright © 2012 SciRes. JTTs
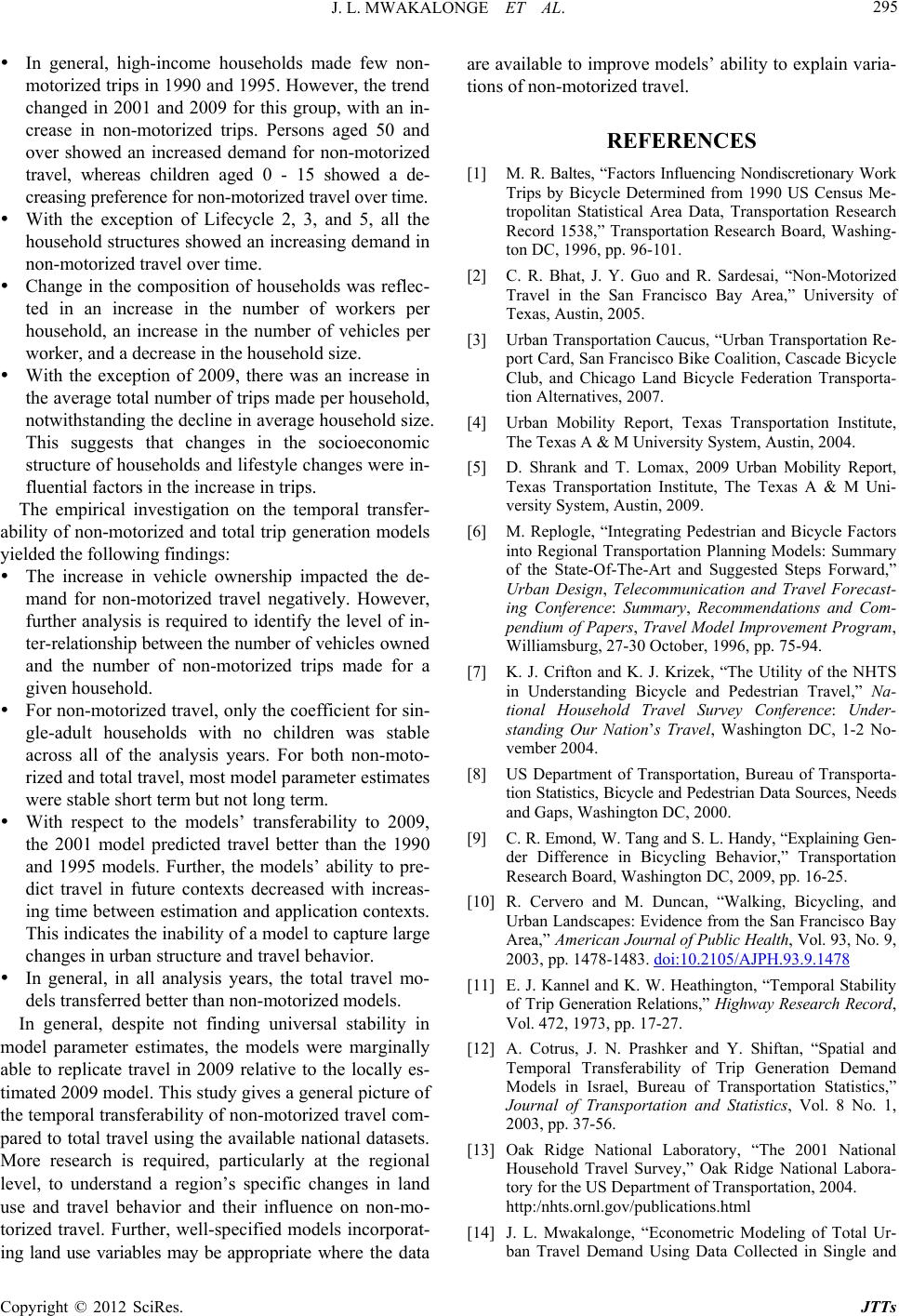 J. L. MWAKALONGE ET AL. 295 In general, high-income households made few non- to ing land use vari [3] Urban Transportation Caucus, “Urban Transportation Re- port Card, Sann, Cascade Bicycle Club, and Chieration Transporta- ute, The Texas A & M Uni- rtation Planning Models: Summary el Model Improvement Program, HTS 5. motorized trips in 1990 and 1995. However, the trend changed in 2001 and 2009 for this group, with an in- crease in non-motorized trips. Persons aged 50 and over showed an increased demand for non-motorized travel, whereas children aged 0 - 15 showed a de- creasing preference for non-motorized travel over time. With the exception of Lifecycle 2, 3, and 5, all the household structures showed an increasing demand in non-motorized travel over time. Change in the composition of households was reflec- ted in an increase in the number of workers per household, an increase in the number of vehicles per worker, and a decrease in the household size. With the exception of 2009, there was an increase in the average total number of trips made per household, notwithstanding the decline in average household size. This suggests that changes in the socioeconomic structure of households and lifestyle changes were in- fluential factors in the increase in trips. The empirical investigation on the temporal transfer- ability of non-motorized and total trip generation models yielded the following findings: The increase in vehicle ownership impacted the de- mand for non-motorized travel negatively. However, further analysis is required to identify the level of in- ter-relationship between the number of vehicles owned and the number of non-motorized trips made for a given household. For non-motorized travel, only the coefficient for sin- gle-adult households with no children was stable across all of the analysis years. For both non-moto- rized and total travel, most model parameter estimates were stable short term but not long term. With respect to the models’ transferability to 2009, the 2001 model predicted travel better than the 1990 and 1995 models. Further, the models’ ability to pre- dict travel in future contexts decreased with increas- ing time between estimation and application contexts. This indicates the inability of a model to capture large changes in urban structure and travel behavior. In general, in all analysis years, the total travel mo- dels transferred better than non-motorized models. In general, despite not finding universal stability in model parameter estimates, the models were marginally able to replicate travel in 2009 relative to the locally es- timated 2009 model. This study gives a general picture of the temporal transferability of non-motorized travel com- pared to total travel using the available national datasets. More research is required, particularly at the regional level, to understand a region’s specific changes in land use and travel behavior and their influence on non-mo- rized travel. Further, well-specified models incorporat- ables may be appropriate where the data are available to improve models’ ability to explain varia- tions of non-motorized travel. REFERENCES [1] M. R. Baltes, “Factors Influencing Nondiscretionary Work Trips by Bicycle Determined from 1990 US Census Me- tropolitan Statistical Area Data, Transportation Research Record 1538,” Transportation Research Board, Washing- ton DC, 1996, pp. 96-101. [2] C. R. Bhat, J. Y. Guo and R. Sardesai, “Non-Motorized Travel in the San Francisco Bay Area,” University of Texas, Austin, 2005. Francisco Bike Coalitio cago Land Bicycle Fed tion Alternatives, 2007. [4] Urban Mobility Report, Texas Transportation Institute, The Texas A & M University System, Austin, 2004. [5] D. Shrank and T. Lomax, 2009 Urban Mobility Report, Texas Transportation Instit versity System, Austin, 2009. [6] M. Replogle, “Integrating Pedestrian and Bicycle Factors into Regional Transpo of the State-Of-The-Art and Suggested Steps Forward,” Urban Design, Telecommunication and Travel Forecast- ing Conference: Summary, Recommendations and Com- pendium of Papers, Trav Williamsburg, 27-30 October, 1996, pp. 75-94. [7] K. J. Crifton and K. J. Krizek, “The Utility of the N in Understanding Bicycle and Pedestrian Travel,” Na- tional Household Travel Survey Conference: Under- standing Our Nation’s Travel, Washington DC, 1-2 No- vember 2004. [8] US Department of Transportation, Bureau of Transporta- tion Statistics, Bicycle and Pedestrian Data Sources, Needs and Gaps, Washington DC, 2000. [9] C. R. Emond, W. Tang and S. L. Handy, “Explaining Gen- der Difference in Bicycling Behavior,” Transportation Research Board, Washington DC, 2009, pp. 16-2 [10] R. Cervero and M. Duncan, “Walking, Bicycling, and Urban Landscapes: Evidence from the San Francisco Bay Area,” American Journal of Public Health, Vol. 93, No. 9, 2003, pp. 1478-1483. doi:10.2105/AJPH.93.9.1478 [11] E. J. Kannel and K. W. Heathington, “Temporal Stability Shiftan, “Spatial and o. 1, Collected in Single and of Trip Generation Relations,” Highway Research Record, Vol. 472, 1973, pp. 17-27. [12] A. Cotrus, J. N. Prashker and Y. Temporal Transferability of Trip Generation Demand Models in Israel, Bureau of Transportation Statistics,” Journal of Transportation and Statistics, Vol. 8 N 2003, pp. 37-56. [13] Oak Ridge National Laboratory, “The 2001 National Household Travel Survey,” Oak Ridge National Labora- tory for the US Department of Transportation, 2004. http:/nhts.ornl.gov/publications.html [14] J. L. Mwakalonge, “Econometric Modeling of Total Ur- ban Travel Demand Using Data Copyright © 2012 SciRes. JTTs
 J. L. MWAKALONGE ET AL. Copyright © 2012 SciRes. JTTs 296 he Estimation of Trip Generation and hold , 2008, pp. 195-206. Repeated Cross-Sectional Surveys,” Ph.D. Dissertation, Department of Civil and Environmental Engineering, Ten- nessee Technological University, Cookeville, 2010. [15] K. G. Goulias, R. M. Pendyala and R. Kitamura, “Practi- cal Method for t Trip Chaining,” Transportation Research Board, Washing- ton DC, 1991, pp. 47-56. [16] Oak Ridge National Laboratory, “The National House Travel Survey,” Oak Ridge National Laboratory for the US Department of Transportation, 2010. http:/nhts.ornl.gov/publications.html [17] G. F. Ulfarsson and V. N. Shankar, “Children’s Travel to School: Discrete Choice Modeling of Correlated Moto- rized and Non-Motorized Transportation Modes Using Covariance Heterogeneity,” Environment and Planning B: Planning and Design, Vol. 35, No. 2 doi:10.1068/b3360 [18] A. Hadayeghi, A. S. Shalaby, B. N. Persaud and C. Cheung, “Temporal Transferability and Updating of Zonal Level Accident Prediction Models,” Accident Analysis & Pre- vention, Vol. 38, No. 3, 2006, pp. 579-589. doi:10.1016/j.aap.2005.12.003 [19] D. A. Badoe, “An Investigation into the Long Range erability Ana- dels,” Transportation Re- Transferability of Work-Trip Discrete Mode Choice Mo- dels,” Ph.D. Dissertation, University of Toronto, Toronto, 1994. [20] F. S. Koppelman and C. G. Wilmot, “Transf lysis of Disaggregate Choice Mo search Board, Washington DC, 1982, pp. 18-24.
|