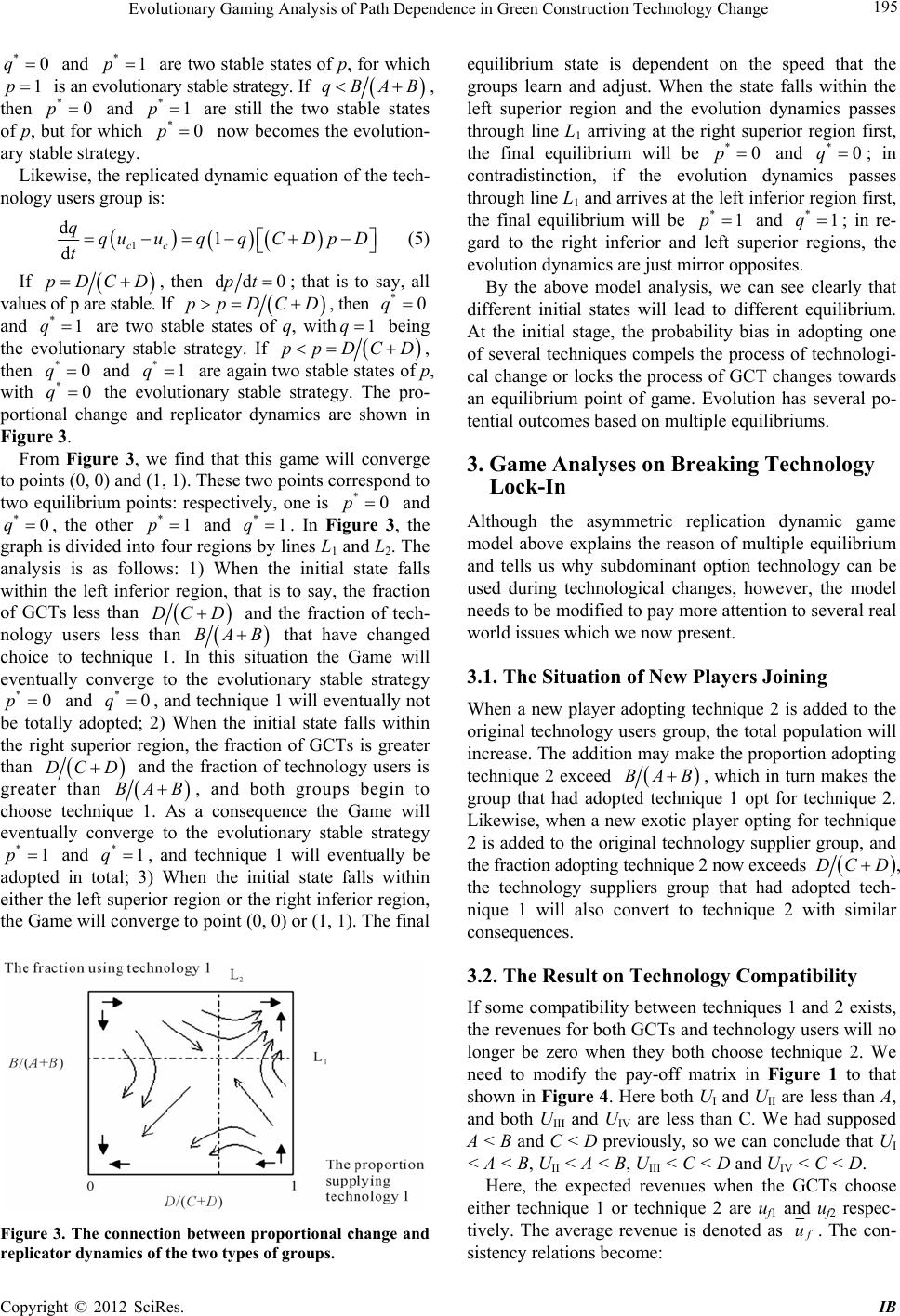
Evolutionary Gaming Analysis of Path Dependence in Green Construction Technology Change 195
*0q
1p
and are two stable states of p, for which
is an evolutionary stable strategy. If
*1p
qBAB,
then and are still the two stable states
of p, but for which now becomes the evolution-
ary stable strategy.
*0p*1
*0p
p
Likewise, the replicated dynamic equation of the tech-
nology users group is:
cc
u
d1
d
qqCDp D
t
1
qu
q
(5)
If pDCD , then dd 0pt; that is to say, all
values of p are stable. If
ppDCD , then *0q
and are two stable states of q, with
*
q11q
being
the evolutionary stable strategy. If
ppD CD,
then and are again two stable states of p,
with the evolutionary stable strategy. The pro-
portional change and replicator dynamics are shown in
Figure 3.
*
q
q0
*0
*
q1
From Figure 3, we find that this game will converge
to points (0, 0) and (1, 1). These two points correspond to
two equilibrium points: respectively, one is *0p
and
, the other and . In Figure 3, the
graph is divided into four regions by lines L1 and L2. The
analysis is as follows: 1) When the initial state falls
within the left inferior region, that is to say, the fraction
of GCTs less than
*0q*1
p*1q
DC D and the fraction of tech-
nology users less than
BAB that have changed
choice to technique 1. In this situation the Game will
eventually converge to the evolutionary stable strategy
and , and technique 1 will eventually not
be totally adopted; 2) When the initial state falls within
the right superior region, the fraction of GCTs is greater
than
*0p*0q
DC D and the fraction of technology users is
greater than
BAB, and both groups begin to
choose technique 1. As a consequence the Game will
eventually converge to the evolutionary stable strategy
and q, and technique 1 will eventually be
adopted in total; 3) When the initial state falls within
either the left superior region or the right inferior region,
the Game will converge to point (0, 0) or (1, 1). The final
*1p*1
Figure 3. The connection between proportional change and
replicator dynamics of the two types of groups.
equilibrium state is dependent on the speed that the
groups learn and adjust. When the state falls within the
left superior region and the evolution dynamics passes
through line L1 arriving at the right superior region first,
the final equilibrium will be and
*0p*0q
; in
contradistinction, if the evolution dynamics passes
through line L1 and arrives at the left inferior region first,
the final equilibrium will be and
*1p*1q
; in re-
gard to the right inferior and left superior regions, the
evolution dynamics are just mirror opposites.
By the above model analysis, we can see clearly that
different initial states will lead to different equilibrium.
At the initial stage, the probability bias in adopting one
of several techniques compels the process of technologi-
cal change or locks the process of GCT changes towards
an equilibrium point of game. Evolution has several po-
tential outcomes based on multiple equilibriums.
3. Game Analyses on Breaking Technology
Lock-In
Although the asymmetric replication dynamic game
model above explains the reason of multiple equilibrium
and tells us why subdominant option technology can be
used during technological changes, however, the model
needs to be modified to pay more attention to several real
world issues which we now present.
3.1. The Situation of New Players Joining
When a new player adopting technique 2 is added to the
original technology users group, the total population will
increase. The addition may make the proportion adopting
technique 2 exceed
BAB, which in turn makes the
group that had adopted technique 1 opt for technique 2.
Likewise, when a new exotic player opting for technique
2 is added to the original technology supplier group, and
the fraction adopting technique 2 now exceeds
DC D
,
the technology suppliers group that had adopted tech-
nique 1 will also convert to technique 2 with similar
consequences.
3.2. The Result on Technology Compatibility
If some compatibility between techniques 1 and 2 exists,
the revenues for both GCTs and technology users will no
longer be zero when they both choose technique 2. We
need to modify the pay-off matrix in Figure 1 to that
shown in Figure 4. Here both UI and UII are less than A,
and both UIII and UIV are less than C. We had supposed
A < B and C < D previously, so we can conclude that UI
< A < B, UII < A < B, UIII < C < D and UIV < C < D.
Here, the expected revenues when the GCTs choose
either technique 1 or technique 2 are uf1 and uf2 respec-
tively. The average revenue is denoted as
u. The con-
sistency relations become:
Copyright © 2012 SciRes. IB