 Psychology 2012. Vol.3, Special Issue, 74 3-748 Published Online Seotenber 2012 in SciRes (http://www.SciRP.org/journal/psych) http://dx.doi.org/10.4236/psych.2012.329112 Copyright © 2012 SciRes. 743 Depression and Internet Use among Older Adolescents: An Experience Sampling Approach Megan A. Moreno1*, Lauren Jelenchick1, Rosali nd K of f2, Jens Eickhoff3 1Department of Pediatr ics, University of Wisconsi n, Madison, USA 2Communications, Cultur e and Technology Departm ent, Georgetown University, Washington, USA 3Department of Biostat i s t i c s , University of Wisconsin, Madison, USA Email: *mamoreno@pediatrics.wisc.edu Received June 10th, 2012; re vi sed J uly 9th, 2012; accepted August 5th, 2012 Background: Depression is common and consequential among adolescents. Previous work has found varied relationships between depression and internet use. The purpose of this study was to examine inter- net use and depression by applying a rigorous assessment tool: experience sampling method (ESM). Methods: Older adolescents between the ages of 18 and 23 years were recruited from a large state uni- versity. Participants received 6 text message surveys randomly each day during a 7-day ESM campaign. Survey questions assessed whether they were currently online and for how long. Participants also com- pleted the PHQ-9 depression survey. Calculation of internet use time included multilevel modeling and probability modeling. Analysis of covariance (ANCOVA) assessed the association between internet use and depression. Results: Among our 189 participants, the mean age was 18.9 (SD = .9), 58.8% were fe- male and most were Caucasian (90.5%). Total daily internet use time was calculated as 66 minutes by ESM summary, 55 minutes by ESM modeling and 65 minutes by probability modeling. We found a dif- ference in PHQ-9 scores when comparing low daily internet use (<30 minutes), regular use (30 minutes to 3 hours) and high use (>3 hours) (p = .01) with a significant U-shaped association (p = .004). The high use group had a mean PHQ-9 score of 7.3 (SD = 5.1) compared to the regular use group score of 4.9 (SD = 3.9) (p = .02). Conclusions: Results suggest a U shaped association between internet use and depres- sion. These findings may present statistical differences that lack clinical significance. Keywords: Adolescent; Internet Use; Depression; Experience Sampling Approach; College Student Introduction Depression among adolescents and young adults is common and consequential (Zogby, 2011). The most common form of depression in this age group is major depressive disorder, with a yearly incidence of approximately 8% (Eisenberg, Golber- stein, & Gollust, 2007; Hunt & Eisenberg, 2010; Vance, Howe, & Dellavalle, 2009). Among college students the prevalence of depression diagnoses has increased over the last six years (As- sociation, 2009; Gallagher, 2007; Hunt & Eisenberg, 2010). Adverse outcomes associated with depression include increased rates of substance use, co-morbid psychiatric conditions and suicide (Deas & Brown, 2006; Garlow et al., 2008; Kessler, Foster, Saunders, & Stang, 1995; Uma Rao, 2006; U. Rao & Chen, 2009). Given the prevalence and negative consequences associated with depression, understanding behaviors or exposures that increase the risk of developing depression is of critical impor- tance. One possible behavioral risk factor is internet use. Inter- net use has seen exponential growth in the last decade, particu- larly among adolescents and young adults. The vast majority of adolescents have Internet access, and most report daily use (Lenhart, Purcell, Smith, & Zickuhr, 2010). Approximately 20% of media consumption by adolescents takes place using mobile platforms such as cell phones, providing internet access at any hour of the day (Moore et al., 2012). Previous studies have begun to explore the association be- tween depression and internet use; findings are varied often conflicting. A previous study suggested a U-shaped relationship between internet use and depression, with increased risks for depression at both the high and low end s of use (Hyde, Mezulis, & Abramson, 2008). Another study proposed an inverted U association, with low and high internet users less likely to report significant distress and depressed mood (Mathers et al., 2009). A few studies have found no association between internet use and depression (Beck, 2008; Miranda & Persons, 1988; Royer, Keller, & Heidrich, 2009), while others have reported a positive association (Hankin & Abramson, 2001; Smith, Alloy, & Ab- ramson, 2006). The recent social media report by the Ameri- can Academy of Pediatrics suggested a causal relationship be- tween use of the internet website Facebook and depression (Smarr & Keefer, 2011). A key factor that may have contributed to the variation in these previous findings is that internet use has been defined and measured using a variety of different scales and instruments. In one study, total internet use was categorized as approximate daily time spent over the la st month, which may be challenging to accurately remember or estimate over an entire month. (Hyde et al., 2008) In another study, internet use was reported on school days only and excluded academic work done using the internet, an estimation that may be challenging to calculate (Manea, Gilbody, & McMillan, 2012). Further, some studies excluded online gaming, while others categorized computer use rather than specifying internet use (Mathers et al., 2009; Smith *Corresponding author.
 M. A. MORENO ET AL. et al., 2006). Thus a potential contributor to the variation in previous study results is that internet use was self-reported by adolescents, and subject to recall bias. The accuracy of self- reported internet use is unclear, a 2005 study examined the gaps between self-reported and tracked internet use found that self- report tended to overestimate the total time spent on the com- puter over the course of a day (Cameron et al., 2011). Given adolescents’ and young adults’ nearly ubiquitous ex- posure to the internet, and varying findings regarding internet use and depression, there is a critical need to better understand this relationship. A understanding of the directionality of this relationship would contribute towards a richer understanding of how internet use may impact depression. For example, it is possible that adolescents with depression may be more likely to spend time on the internet due to a lack of motivation; however, they may also use the internet for shorter periods of time due to a lack of concentration. However, before these hypotheses can be more fully developed, the association between internet use time and depression symptoms must be better understood. To do so, methods beyond self-reported internet use are needed. Given adolescents’ access to technology via mobile phones, using technology to measure technology may provide a more robust and real-time assessment tool. A real-time exami- nation of internet use using mobile phones can be accomplished using experience sampling method (ESM). ESM, also called ecological momentary assessment (EMA), is characterized by a collection of data in real-world environments that focus on individual’s current or very recent behaviors. This approach has the advantage of being within the participant’s own habitat, enhancing the external validity of the results (Arroll et al., 2010). ESM is well suited for rich description and understand- ing the most complete description of the phenomenon within the daily life of the individual. ESM also avoids the reliance on retrospective reports which may be subject to biases that chal- lenge their reliability and validity (Cameron, Reid, & Lawton, 2010). Experience sampling method includes multiple discrete as- sessments over time to evaluate a participant’s current moods, thoughts, symptoms or behaviors (Cannon et al., 2007; Mal- pass, Shaw, Kessler, & Sharp, 2010; Lavin, Marvin, McLar- ney, Nola, & Scott, 1999; Shiffman, Kirchner, Ferguson, & Scharf, 2009; Trull & Ebner-Priemer, 2009). An ESM approach using electronic media has an advantage over more traditional methods using paper diaries, as with paper diaries the re- searcher cannot ensure that the diary was completed at the specified times. Sending and receiving text messages via cell phones presents additional advantages as it is faster than email and less intrusive than a phone call. A recent pilot study explored the relationship between inter- net use and depression with an ESM approach using cell phones and calls made by researchers to participants. This pre- vious study examined 46 participants diagnosed with Major Depressive Disorder and 60 controls to evaluate the relationship between internet use and depression and found no association (Royer et al., 2009). As previous studies have suggested that internet use may be related to differences among levels of de- pression symptoms, a similarly robust examination of internet use from a more general sample is warranted (Hyde et al., 2008). Thus, the purpose of the current study was to contribute to the body of literature examining internet use and depression using ESM. Our goal was to use a robust ESM methodology that would limit the recall bias inherent in self-report, and that would also be feasible to conduct in larger, community-acquired samples. Methods Setting and S ub jects This study took place at a large state university and received approval from the University of Wisconsin Institutional Review Board. Potential subjects were identified within a communica- tions classroom, a course requirement for several majors and a general distribution requirement for many departments. Inclu- sion criteria required students to be current undergraduates be- tween the ages of 18 and 23 years. Subjects were required to have a cellular phone with texting ability. Recent campus data reports that approximately half of students own a cellular phone with texting ability, and 59% own a phone with texting and internet access (Martin, Rief, Klaiberg, & Braehler, 2006). Recruitment Students were initially recruited to participate in a brief online health survey for extra credit in the class. They were given several extra credit options within the semester and were allowed to participate in up to five of these assignments. Over 75% of students in the class pursued one or more extra credit assignments during the semester. Among these five extra credit options, one was a brief online health survey that included a depression screen. We recruited students who completed this online survey to the experience sampling method (ESM) por- tion of the study using email and phone calls. Experience Sampling Method ESM has been used in a variety of studies to collect real-time data regarding thoughts, moods or behaviors that occur inter- mittently (Gangadharbatla, 2008; Kroenke, Spitzer, & Williams, 2001; Shiffman, Stone, & Hufford, 2008). This study adminis- tered ESM surveys by sending text messages to and receiving data from participants’ cell phones. Text messaging using par- ticipants’ personal phones may be an advantageous approach for large-scale ESM data collections as it is both costeffective and capable of being fully automated. In order to create a program that could be programmed to send the text messages and receive the incoming data, we con- sulted with an Academic Application Developer from the Uni- versity of Wisconsin Division of Information Technology’s Academic Technology section. These application developers then designed and programmed a short message service (SMS) survey tool web application that allowed for the configuration and deployment of randomized several-day survey campaigns. The survey tool web application was paired with a commercial communication application (Twilio, http://www.twilio.com/), which was designed to securely relay high volumes of income- ing and outgoing scheduled text messages. Returned text mes- sage surveys were trac ked by the SMS survey tool database. Data Collection Procedure Each participant received a total of 43 text messages over the 7 day ESM campaign. The SMS program delivered text surveys 6 times a day starting at 6:00 am with the last message to be received no later than 1:00 am. Each day the SMS program generated a randomized schedule for each subject that distrib- Copyright © 2012 SciRes. 744
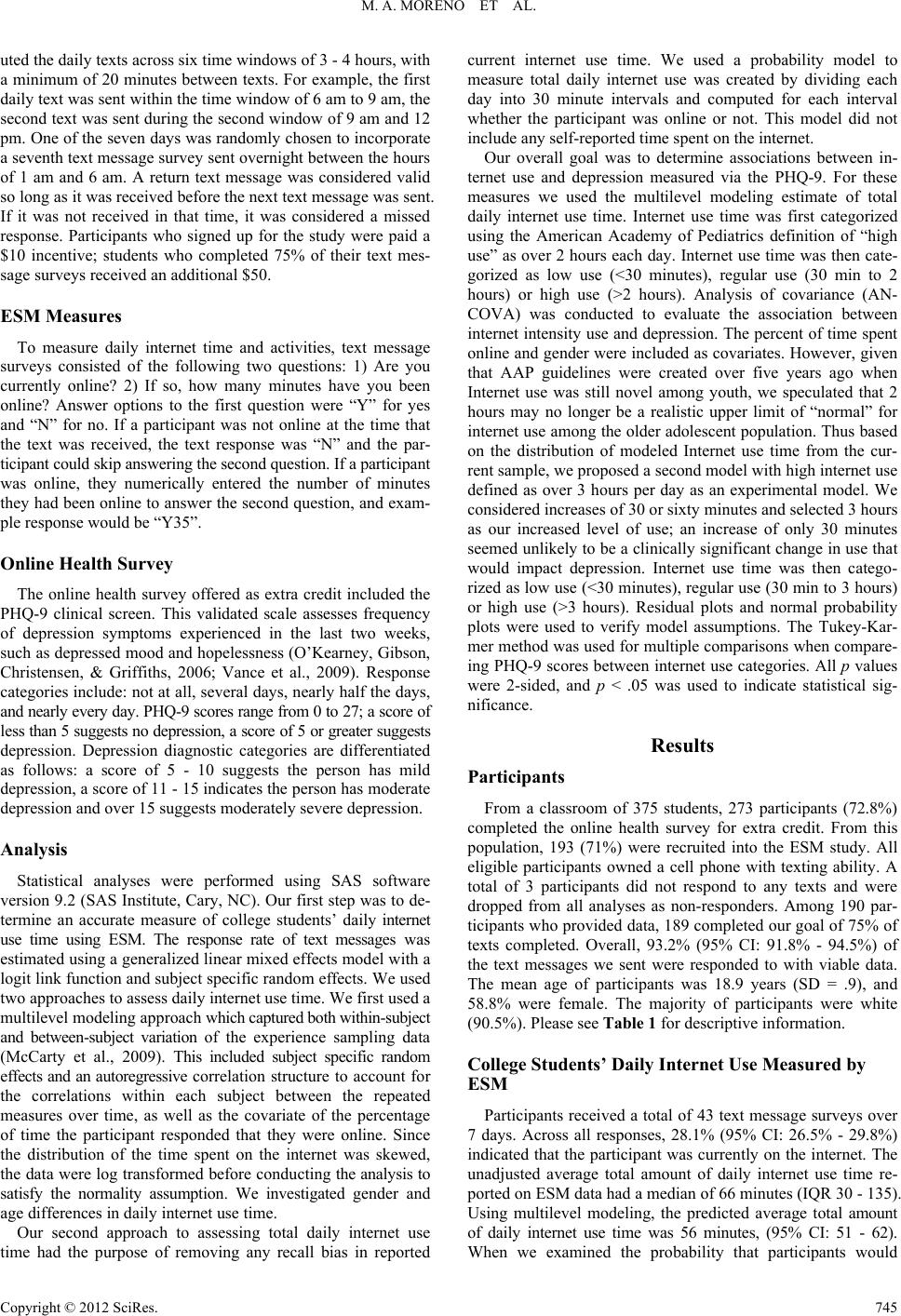 M. A. MORENO ET AL. uted the daily texts across six time windows of 3 - 4 hours, with a minimum of 20 minutes between texts. For example, the first daily text was sent within the time window of 6 am to 9 am, the second text was sent during the second window of 9 am and 12 pm. One of the seven days was randomly chosen to incorporate a seventh text message survey sent overnight between the hours of 1 am and 6 am. A return text message was considered valid so long as it was received before the next text message was sent. If it was not received in that time, it was considered a missed response. Participants who signed up for the study were paid a $10 incentive; students who completed 75% of their text mes- sage surveys received an additional $50. ESM Measure s To measure daily internet time and activities, text message surveys consisted of the following two questions: 1) Are you currently online? 2) If so, how many minutes have you been online? Answer options to the first question were “Y” for yes and “N” for no. If a participant was not online at the time that the text was received, the text response was “N” and the par- ticipant could skip answering the second question. If a participa nt was online, they numerically entered the number of minutes they had been online to answer the second question, and exam- ple response would be “Y35”. Online Health Survey The online health survey offered as extra credit included the PHQ-9 clinical screen. This validated scale assesses frequency of depression symptoms experienced in the last two weeks, such as depressed mood and hopelessness (O’Kearney, Gibson, Christensen, & Griffiths, 2006; Vance et al., 2009). Response categories include: not at all, several days, nearly half the days, and nearly every day. PHQ-9 scores range from 0 to 27; a score of less than 5 suggests no depression, a score of 5 or greater suggests depression. Depression diagnostic categories are differentiated as follows: a score of 5 - 10 suggests the person has mild depression, a score of 11 - 15 indicates the person has moderate depression and over 15 suggests moderately severe depression. Analysis Statistical analyses were performed using SAS software version 9.2 (SAS Institute, Cary, NC). Our first step was to de- termine an accurate measure of college students’ daily internet use time using ESM. The response rate of text messages was estimated using a generalized linear mixed effects model with a logit link function and subject specific random effects. We used two approaches to assess daily internet use time. We first used a multilevel modeling approach which captured both within-subject and between-subject variation of the experience sampling data (McCarty et al., 2009). This included subject specific random effects and an autoregressive correlation structure to account for the correlations within each subject between the repeated measures over time, as well as the covariate of the percentage of time the participant responded that they were online. Since the distribution of the time spent on the internet was skewed, the data were log transformed before conducting the analysis to satisfy the normality assumption. We investigated gender and age differences in daily internet use time. Our second approach to assessing total daily internet use time had the purpose of removing any recall bias in reported current internet use time. We used a probability model to measure total daily internet use was created by dividing each day into 30 minute intervals and computed for each interval whether the participant was online or not. This model did not include any self-reported time spent on the internet. Our overall goal was to determine associations between in- ternet use and depression measured via the PHQ-9. For these measures we used the multilevel modeling estimate of total daily internet use time. Internet use time was first categorized using the American Academy of Pediatrics definition of “high use” as over 2 hours each day. Internet use time was then cate- gorized as low use (<30 minutes), regular use (30 min to 2 hours) or high use (>2 hours). Analysis of covariance (AN- COVA) was conducted to evaluate the association between internet intensity use and depression. The percent of time spent online and gender were included as covariates. However, given that AAP guidelines were created over five years ago when Internet use was still novel among youth, we speculated that 2 hours may no longer be a realistic upper limit of “normal” for internet use among the older adolescent population. Thus based on the distribution of modeled Internet use time from the cur- rent sample, we proposed a second model with high internet use defined as over 3 hours per day as an experimental model. We considered increases of 30 or sixty minutes and selected 3 hours as our increased level of use; an increase of only 30 minutes seemed unlikely to be a clinically significant change in use that would impact depression. Internet use time was then catego- rized as low use (<30 minutes), regular use (30 min to 3 hours) or high use (>3 hours). Residual plots and normal probability plots were used to verify model assumptions. The Tukey-Kar- mer method was used for multiple comparisons when compare- ing PHQ-9 scores between internet use categories. All p values were 2-sided, and p < .05 was used to indicate statistical sig- nificance. Results Participants From a classroom of 375 students, 273 participants (72.8%) completed the online health survey for extra credit. From this population, 193 (71%) were recruited into the ESM study. All eligible participants owned a cell phone with texting ability. A total of 3 participants did not respond to any texts and were dropped from all analyses as non-responders. Among 190 par- ticipants who provided data, 189 completed our goal of 75% of texts completed. Overall, 93.2% (95% CI: 91.8% - 94.5%) of the text messages we sent were responded to with viable data. The mean age of participants was 18.9 years (SD = .9), and 58.8% were female. The majority of participants were white (90.5%). Please see Table 1 for descrip t i ve in f o rmation. College Students’ Daily Internet Use Measured by ESM Participants received a total of 43 text message surveys over 7 days. Across all responses, 28.1% (95% CI: 26.5% - 29.8%) indicated that the participant was currently on the internet. The unadjusted average total amount of daily internet use time re- ported on ESM data had a median of 66 minutes (IQR 30 - 135). Using multilevel modeling, the predicted average total amount of daily internet use time was 56 minutes, (95% CI: 51 - 62). When we examined the probability that participants would Copyright © 2012 SciRes. 745
 M. A. MORENO ET AL. Copyright © 2012 SciRes. 746 ESM Daily Internet Use Time Associated with Depression respond to the text survey reporting that they were currently online, we found the average total daily amount of time spent on the internet was 55.6 minutes (range 20 - 102 minutes). The median time reported as current internet use time for each indi- vidual text query was 30 minutes (IQR 20 - 60). Using the mul- tilevel modeled ESM data to evaluate daily internet time and demographic characteristics, there were no gender or age dif- ferences noted. Table 1. Demographic information. N = 190 Study Sample M (SD)/n ( %) Age (years) 18.9 (.8) Gender Male 79 (41.6) Female 111 (58.4) Ethnicity Caucasian/White 174 (90.5) Hispanic/Latino 6 (3.2) Asian/Asian American 5 (2.6) African American 2 (1.0) Native American/Alaskan Native 0 (.0) Multi-Racial 5 (2.63) We used the AAP’s guidelines to compare low use (<30 minutes), regular use (30 minutes to 2 hours) and high use (>2 hours) measured by modeled ESM data. Few differences were seen in association with mean total PHQ score. The low inter- net use group had a mean PHQ-9 score of 6.2 (SD 4.4), the regular use group had a mean PHQ-9 score of 5.2 (SD = 4.1) while the high use group had a mean PHQ-9 score of 5.5 (SD = 4.4). ANCOVA results showed no significant differences be- tween these levels of internet use and PHQ-9 score (p =.32). However, using our modified AAP criteria to compare low internet use (30 minutes), regular internet use (defined as 30 minutes to 3 hours) and high internet use (defined as over 3 hours), results differed. An overall difference in the total PHQ- 9 scores between the three internet use categories was detected (p = .01). There was a significant quadratic trend (U-shape association, p = .004). The low internet use group had a mean PHQ-9 score of 6.2 (SD = 4.4) compared to a mean PHQ-9 score of 4.9 (SD = 3.9) in the regular use group (p = .6). The high internet use group had a mean PHQ-9 score of 7.3 (SD = 5.1) which was significantly higher compared to the mean score in the regular use group (p = .02, Tukey-Kramer adjustment for multiple comparisons) (Figure 1). N=36 N=116 N=33 0 1 2 3 4 5 6 7 8 < 30 M i nutes30 - 120 Mi nut es > 120 M i nutes ESM Time Online PHQ Total Score (a) (b) Figure 1. PHQ-9 depression scores (means and standard error bars) and estimated daily internet use estimated by ESM (a) <30 minutes, 30 - 120 minutes, >120 minutes; (b) <30 minutes, 30 - 180 minutes, >180 minutes (*Tukey-Kramer adjustment for multiple comparisons).
 M. A. MORENO ET AL. Discussion This study assessed the relationship between quantity of internet use measured by ESM and depression measured by a validated clinical screen. Using standard definitions of recom- mended internet use quantity provided by the American Acad- emy of Pediatrics, we found no relationship between internet use and depression. However, when we applied a modified internet use quantity categorization with 3 hours as the upper limit of normal, we found a weak U shaped association between internet use and depression. These findings are consistent with past work that demonstrated a similar U shaped association. (Hyde et al., 2008) While our study found a U shaped association between internet use and depression that had statistical significance, it is important to consider the clinical relevance of these differences. While the regular internet use group had a mean depression score of 4.9 (SD 3.9) and the high use group had a mean score of 7.3 (SD 5.1), the range of scores in the mild depression category is between 5 and 9. Thus, the difference in these scores does not suggest a significant change between depression cate- gories. Rather, it suggests a difference that may be of numerical rather than clinical significance. Thus, when considering our results in the context of related studies, our findings suggest that depression symptoms may in fact vary with differences in Internet use. However, differences in symptoms are likely to represent fluctuations at the mild end of the symptom spec- trum. Therefore, whether these differences are captured across studies may be highly dependent how Internet use is measured and categorized. It is possible that using the rigorous ESM method to collect data regarding internet use time led to improved accuracy compared to what is often found in studies using self-reported internet use. ESM provides an innovative method to assess daily internet use time that decreases recall bias, replication of this method in diverse samples may contribute additional data to determine the relationship between internet use and depress- sion. ESM may also provide an avenue for further evaluating how differences in content of Internet use may be related to depression symptoms. Recent findings suggest that media mul- titasking is highly prevalent among older adolescents. (Moreno et al., 2012) Thus, isolating relationships between specific types of usage and self-report measures alone may be highly chal- lenging. Our study findings are limited in that we only examined stu- dents from one university and within a single classroom setting. In order to maximize generalizability we selected a large class- room that draws from multiple majors, and is a distribution requirement for several academic programs. A second limita- tion is that we only measured depression at one time point in the study, so we are not able to assess symptom patterns over time. However, we assessed depression in the same time period as our internet use assessment. A third limitation is that we do not have independent verification of the reported duration of time reported by participants. We do have confidence in the completeness of the data captured by each participant, as the response rate to texts across the entire study length was over 90%. As most other studies of internet use and depression have relied upon a single measure of retrospective internet use over time, the ESM methods used in this study provide a more rich and in-time assessment of self-reported internet use. A fourth consideration is that the life of a college student includes vari- ous activities such as classes, work and socialization. Further, activities may include both beneficial or risky health activities such as exercise or alcohol use. These factors may impact the frequency of internet use, and we did not control for all these individual factors within our data collection measures. However, applying consistent and randomized data collection measures to assess internet use allowed us to determine internet use across both weekdays and weekends in the course of the seven day data collection period. Study findings are also limited in that our study sample included very few minority participants, which is consistent with the demographic of our university. Conclusion Despite these limitations, our findings have important impli- cations for future work. Our goal in this study was to contribute a key finding to the unresolved question regarding the relation- ship between internet use and depression. We found a weak U-shaped association between internet use and mild symptoms of depression that was of statistical significance, but unlikely to represent major clinical difference. Our findings underscore the need for further explore this potential relationship; we suggest further research in larger populations to measure daily internet use via ESM to determine whether these findings can be repli- cated. Acknowledgements The work described was supported by funding from the Uni- versity of Wisconsin Graduate School. The authors would like to acknowledge Greg Downey, Ph.D., Kaitlyn Bare, BS and Sandon Jurowski, BS for their assistance with this project. REFERENCES Arroll, B., Goodyear-Smith, F., Crengle, S., Gunn, J., Kerse, N., Fish- man, T., & Hatcher, S. (2010). Validation of PHQ-2 and PHQ-9 to screen for major depression in the primary care population. The An- nals of Family Medicine, 8, 348-353. doi:10.1370/afm.1139 Association, A. C. H. (2009). American College Health Association: National College Health Assessment II: Reference Group Data Re- port Fall 2008. Baltimore: American College Health Association. Beck, A. T. (2008). The evolution of the cognitive model of depression and its neurobiological correlates. American Psychiatric Association, 165, 969-977. doi:10.1176/appi.ajp.2008.08050721 Cameron, I. M., Cardy, A., Crawford, J. R., du Toit, S. W., Hay, S., Lawton, K., & Reid, I. C. (2011). Measuring depression severity in general practice: discriminatory performance of the PHQ-9, HADS-D, and BDI-II. British Journal of General Practice, 61, e419-426. doi:10.3399/bjgp11X583209 Cameron, I. M., Reid, I. C., & Lawton, K. (2010). PHQ-9: Sensitivity to change over time. British Journal of General Practice, 60, 535- 536. doi:10.3399/bjgp10X514909 Cannon, D. S., Tiffany, S. T., Coon, H., Scholand, M. B., McMahon, W . M., & Leppert, M. F. (2007). The PHQ-9 as a brief assessment of lifetime major depression. Psychological Assessment, 19, 247-251. doi:10.1037/1040-3590.19.2.247 Deas, D., & Brown, E. S. (2006). Adolescent substance abuse and psy- chiatric comorbidities. Journal of Clinical Psychiatry, 67, e02. doi:10.4088/JCP.0706e02 Eisenberg, D., Golberstein, E., & Gollust, S. E. (2007). Help-seeking and access to mental health care in a university student population. Medical Care, 45, 594-601. doi:10.1097/MLR.0b013e31803bb4c100005650-200707000-00003 Gallagher, R. P. (2007). National Survey of Couseling Center Directors, 2006. URL (last checked 1 October 2010). Copyright © 2012 SciRes. 747
 M. A. MORENO ET AL. http://www.education.pitt.edu/survey/nsccd/archive/2006/monograph .pdf Gangadharbatla, H. (2008). Facebook me: Collective self-esteem, need to belong, and internet self-efficacy as predictors of the iGeneration’s attitudes toward social networking sites. Journal of Interactive Ad- vertising, 8, 5-15. Garlow, S. J., Rosenberg, J., Moore, J. D., Haas, A. P., Koestner, B., Hendin, H., & Nemeroff, C. B. (2008). Depression, desperation, and suicidal ideation in college students: Results from the American Foundation for Suicide Prevention College Screening Project at Emory Un iversity. Depress i on a n d Anxiety, 25, 482-488. doi:10.1002/da.20321 Hankin, B. L., & Abramson, L. Y. (2001). Development of gender dif- ferences in depression: An elaborated cognitive vulnerability-trans- actional stress theory. Psychological Bull et i n , 127, 773-796. doi:10.1037/0033-2909.127.6.773 Hunt, J., & Eisenberg, D. (2010). Mental health problems and help- seeking behavior among college students. Journal of Adolescent Health, 46, 3-10. doi:10.1016/j.jadohealth.2009.08.008 Hyde, J. S., Mezulis, A. H., & Abramson, L. Y. (2008). The ABCs of depression: integrating affective, biological, and cognitive models to explain the emergence of the gender difference in depression. Psy- chological Review, 115, 291-313. doi:10.1046/j.1525-1497.2001.016009606.x Kessler, R. C., Foster, C. L., Saunders, W. B., & Stang, P. E. (1995). Social consequences of psychiatric disorders I: Educational attain- ment. The American Journal of Psychiatry, 152, 1026-1032. Kroenke, K., Spitzer, R. L., & Williams, J. B. (2001). The PHQ-9: Va- lidity of a brief depression severity measure. Journal of General In- ternal Medicine, 16, 606-613. Lavin, M., Marvin, K., McLarney, A., Nola, V., & Scott, L. (1999). Sensation seeking and collegiate vulnerability to internet dependence. CyberPsychology & Behavior, 2, 425-430. Lenhart, A., Purcell, K., Smith, A., & Zickuhr, K. (2010). Social media and young adults. Washington DC: Pew Internet and American Life Project. Malpass, A., Shaw, A., Kessler, D., & Sharp, D. (2010). Concordance between PHQ-9 scores and patients’ experiences of depression: A mixed methods study. British Journal of General Practice, 60, e231- 238. doi:10.3399/bjgp10X502119 Manea, L., Gilbody, S., & McMillan, D. (2012). Optimal cut-off score for diagnosing depression with the Patient Health Questionnaire (PHQ-9): A meta-analysis. Canadian Medical Association Journal, 184, 191-196. doi:10.1503/cmaj.110829 Martin, A., Rief, W., Klaiberg, A., & Braehler, E. (2006). Validity of the Brief Patient Health Questionnaire Mood Scale (PHQ-9) in the general population. General Hospital Psychiatry, 28, 71-77. doi:10.1016/j.genhosppsych.2005.07.003 Mathers, M., Canterford, L., Olds, T., Hesketh, K., Ridley, K., & Wake, M. (2009). Electronic media use and adolescent health and well-be- ing: Cross-sectional community study. American Academy of Pediat- rics, 9, 307-314. McCarty, C. A., K oster man, R., Mas on, W. A., M cCauley, E. , Hawk ins, J. D., Herrenkohl, T. I., & Lengua, L. J. (2009). Longitudinal asso- ciations among depression, obesity and alcohol use disorders in young adulthood. General Hospital Psychiatry, 31, 442-450. doi:10.1016/j.genhosppsych.2009.05.013 Miranda, J., & Persons, J. B. (1988). Dysfunctional attitudes are mood- state dependent. Journal of A bnormal Psychology, 97, 76- 79. doi:10.1037/0021-843X.97.1.76 Moore, M., Ali, S., Stuart, B., Leydon, G. M., Ovens, J., Goodall, C., & Kendrick, T. (2012). Depression management in primary care: An observational study of management changes related to PHQ-9 score for depression monitoring. British Journal of General Practice, 62, 451-457. doi:10.3399/bjgp12X649151 Moreno, M. A., Jelen chick, L., Koff, R., Eickhoff, J. E., Dier myer, C., & Christakis, D. A. (2012). Internet use and multitasking among older adolescents: An experience sampling approach. Computers and Human Behavior, 28, 1097-1102. O'Kearney, R., Gibson, M., Christensen, H., & Griffiths, K. M. (2006). Effects of a cognitive-behavioural internet program on depression, vulnerability to depression and stigma in adolescent males: A school- based controlled trial. Cognitive Behaviour Therapy, 35, 43-54. doi:10.1080/16506070500303456 Rao, U. (2006). Links between depression and substance abuse in ado- lescents: Neurobiological mechanisms. American Journal of Preven- tive Medicine, 31, S161-S174. doi:10.1016/j.amepre.2006.07.002 Rao, U., & Chen, L. A. (2009). Characteristics, correlates, and out- comes of childhood and adolescent depressive disorders. Dialogues in Clinical Neuroscience, 11, 45-62. Royer, H., Keller, M. L., & Heidrich, S. M. (2009). Young adolescents’ perceptions of romanti c relationships. Sex Education, 9, 395-408. doi:10.1080/14681810903265329 Shiffman, S., Kirchner, T. R., Ferguson, S. G., & Scharf, D. M. (2009). Patterns of intermittent smoking: An analysis using Ecological Mo- mentary Assessment. Addictive Behaviors, 34, 514-519. Shiffman, S., Stone, A. A., & Hufford, M. R. (2008). Ecological mo- mentary assessment. Annual Review of Clinical Psychology, 4, 1-32. doi:10.1146/annurev.clinpsy.3.022806.091415 Smarr, K. L., & Keefer, A. L. (2011). Measures of depression and de- pressive symptoms: Beck Depression Inventory-II (BDI-II), Center for Epidemiologic Studies Depression Scale (CES-D), Geriatric De- pression Scale (GDS) , Hospita l Anxiet y and Depression S cale (HA DS), and Patient Health Questionnaire-9 (PHQ-9). Arthritis Care & Re- search, 63, S454-S466. doi:10.1002/acr.20556 Smith, J. M., Alloy, L. B., & Abramson, L. Y. (2006). Cognitive vul- nerability to depression, rumination, hopelessness, and suicidal idea- tion: Multiple pathways to self-injurious thinking. Suicide and Life- Threatening Behavior, 36, 443-454. doi:10.1521/suli.2006.36.4.443 Trull, T., & Ebner-Priemer, U. (2009). Using experience sampling meth- ods/ecological momentary assessment (ESM/EMA) in clinical as- sessment and clinical research: Introduction to the special section. Psychological Assessment, 21, 457-462. doi:10.1037/a0017653 Vance, K., Howe, W., & Dellavalle, R. P. (2009). Social internet sites as a source of public health information. Dermatologic Clinics, 27, 133-136. Zogby. (2011). The State of K-12 Cyberethics, Cybersafety and Cy- bersecurity Curriculum in the United States. The National Cyber Se- curity Alliance. Copyright © 2012 SciRes. 748
|