 Advances in Remote Sensing, 2012, 1, 19-34 http://dx.doi.org/10.4236/ars.2012.12003 Published Online September 2012 (http://www.SciRP.org/journal/ars) An Optical Model for the Remote-Sensing of Absorption Coefficients of Phytoplankton in Oceanic/Coastal Waters Surya Prakash Tiwari, Palanisamy Shanmugam Department of Ocean Engineering, Indian Institute of Technology Madras, Chennai, India Email: pshanmugam@iitm.ac.in Received July 2, 2012; revised July 31, 2012; accepted August 20, 2012 ABSTRACT A new model for the remote sensing of absorption coefficients of phytoplankton aph(λ) in oceanic and coastal waters is developed and tested with SeaWiFS and MODIS-Aqua data. The model is derived from a relationship of the remote sensing reflectance ratio Rrs(670)/Rrs(490) and aph(λ) (from large in-situ data sets). When compared with over 470 inde- pendent in-situ data sets, the model provides accurate retrievals of the aph(λ) across the visible spectrum, with mean relative error less than 8%, slope close to unity and R2 greater than 0.8. Further comparison of the SeaWiFS-derived aph(λ) with in-situ aph(λ) values gives similar and consistent results. The model when used for analysis of MODIS-Aqua imagery, provides more realistic values of the phytoplankton absorption coefficients capturing spatial structures of the massive algal blooms in surface waters of the Arabian Sea. These results demonstrate that the new algorithm works well for both the coastal and open ocean waters observed and suggest a potential of using remote sensing to provide knowl- edge on the shape of phytoplankton absorption spectra that are a requirement in many inverse models to estimate phytoplankton pigment concentrations and for input into bio-optical models that predict carbon fixation rates for the global ocean. Keywords: Remote Sensing; Phytoplankton Absorption; Bio-Optical Models; Coastal Waters; MODIS-Aqua; SeaWiFS; Arabian Sea 1. Introduction Phytoplanktons play a critical role in the cycling of bio- geochemical properties, and are responsible for much of the oxygen present in the Earth’s atmosphere through a process known as photosynthesis. Their cumulative en- ergy fixation in carbon compounds that account for ap- proximately half of the world’s total primary productivity is the basis for the majority of oceanic food chains. They are highly diverse in shape, size, and pigmentation, hav- ing a predominant influence on the colour of seawater measured by satellite sensors [1,2]. Light absorption by particulate phytoplankton—which determines the amount of radiant energy captured by them —is an important source of optical variability in surface waters of the ocean. This variability has consequences for light attenuation, primary production, remote sensing of pigment biomass and mixed layer heating [3-8]. The spectra of phytoplankton absorption (aph(λ)) vary widely both in terms of magnitude and spectral behaviour [9-11] in seawaters because of differences in phytoplankton community, cell size, and pigment packages among sites [11-13]. For these reasons and because of the advent of remote sensing capabilities, there is increasing demand for a fundamental knowledge of the magnitude, range and sources of variability in phytoplankton optical prop- erties in marine surface waters. Remote sensing offers the potential for synoptic assessment of pigment biomass and primary production, but this requires the ability to accu- rately estimate phytoplankton absorption coefficients from remotely measured signals using an appropriate optical model that has potential applications in ocean colour remote sensing. To estimate aph(λ) coefficients from remote sensing data, several models have been reported in the recent stud- ies which enable retrieval of two or more in-water con- stituents and properties simultaneously. For these models, an inversion technique is usually applied to a parameter- ized ocean colour model whose parameters have been determined from in-situ bio-optical measurements. Garver and Siegel [14] developed a nonlinear statistical method for the inversion of ocean colour data, which assumed the known spectral shapes of specific absorption coeffi- cients for phytoplankton. Later, this model was improved and optimized by Maritorena et al. [15] (the GSM01 model) using simulated annealing, thus the model could be applied to global ocean colour data for improved re- trievals of pigment concentrations. However, GSM model provides absorption coefficients of phytoplankton at spe- C opyright © 2012 SciRes. ARS
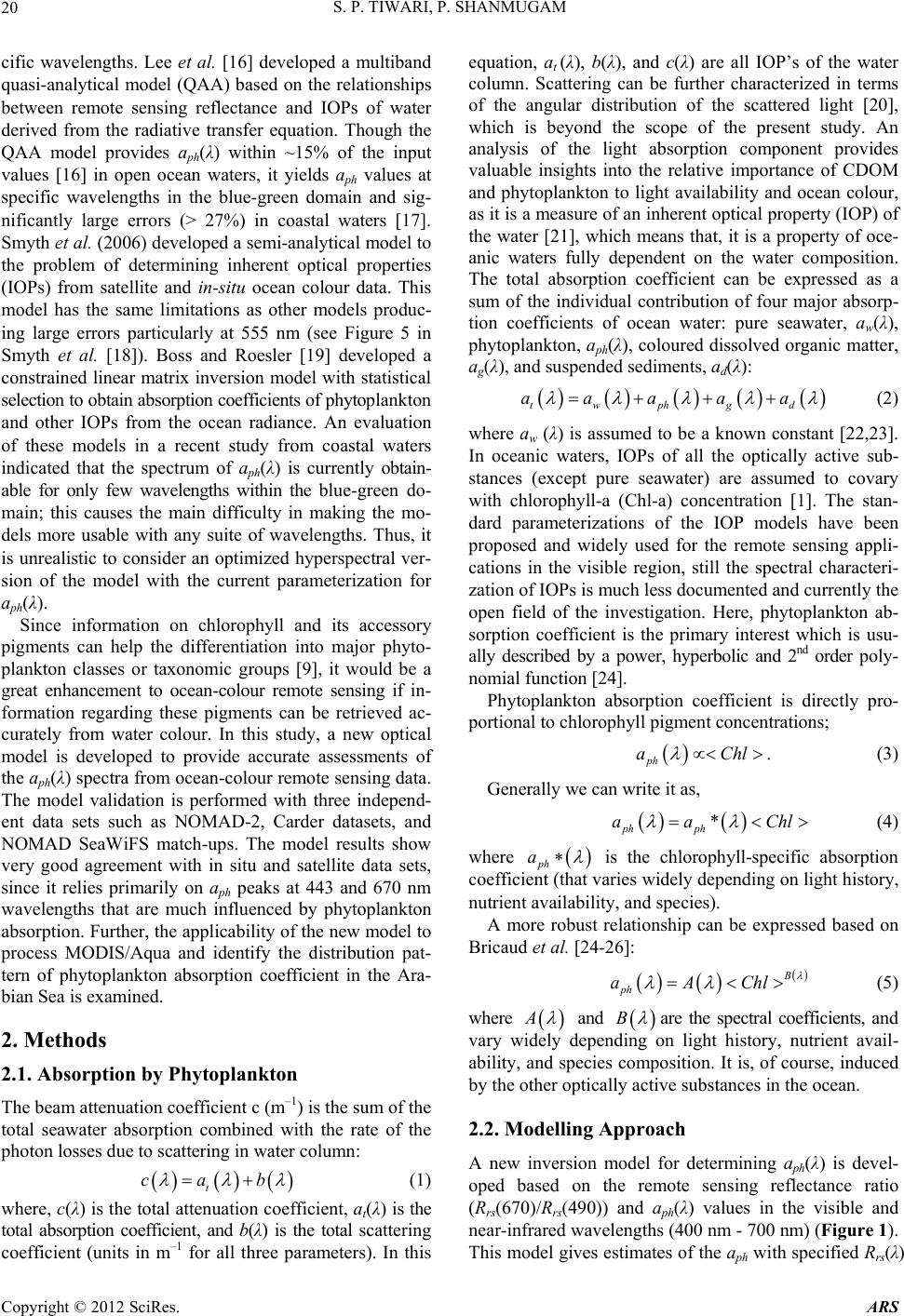 S. P. TIWARI, P. SHANMUGAM 20 cific wavelengths. Lee et al. [16] developed a multiband quasi-analytical model (QAA) based on the relationships between remote sensing reflectance and IOPs of water derived from the radiative transfer equation. Though the QAA model provides aph(λ) within ~15% of the input values [16] in open ocean waters, it yields aph values at specific wavelengths in the blue-green domain and sig- nificantly large errors (> 27%) in coastal waters [17]. Smyth et al. (2006) developed a semi-analytical model to the problem of determining inherent optical properties (IOPs) from satellite and in-situ ocean colour data. This model has the same limitations as other models produc- ing large errors particularly at 555 nm (see Figure 5 in Smyth et al. [18]). Boss and Roesler [19] developed a constrained linear matrix inversion model with statistical selection to obtain absorption coefficients of phytoplankton and other IOPs from the ocean radiance. An evaluation of these models in a recent study from coastal waters indicated that the spectrum of aph(λ) is currently obtain- able for only few wavelengths within the blue-green do- main; this causes the main difficulty in making the mo- dels more usable with any suite of wavelengths. Thus, it is unrealistic to consider an optimized hyperspectral ver- sion of the model with the current parameterization for aph(λ). Since information on chlorophyll and its accessory pigments can help the differentiation into major phyto- plankton classes or taxonomic groups [9], it would be a great enhancement to ocean-colour remote sensing if in- formation regarding these pigments can be retrieved ac- curately from water colour. In this study, a new optical model is developed to provide accurate assessments of the aph(λ) spectra from ocean-colour remote sensing data. The model validation is performed with three independ- ent data sets such as NOMAD-2, Carder datasets, and NOMAD SeaWiFS match-ups. The model results show very good agreement with in situ and satellite data sets, since it relies primarily on aph peaks at 443 and 670 nm wavelengths that are much influenced by phytoplankton absorption. Further, the applicability of the new model to process MODIS/Aqua and identify the distribution pat- tern of phytoplankton absorption coefficient in the Ara- bian Sea is examined. 2. Methods 2.1. Absorption by Phytoplankton The beam attenuation coefficient c (m–1) is the sum of the total seawater absorption combined with the rate of the photon losses due to scattering in water column: t ca b (1) where, c(λ) is the total attenuation coefficient, at(λ) is the total absorption coefficient, and b(λ) is the total scattering coefficient (units in m–1 for all three parameters). In this equation, at (λ), b(λ), and c(λ) are all IOP’s of the water column. Scattering can be further characterized in terms of the angular distribution of the scattered light [20], which is beyond the scope of the present study. An analysis of the light absorption component provides valuable insights into the relative importance of CDOM and phytoplankton to light availability and ocean colour, as it is a measure of an inherent optical property (IOP) of the water [21], which means that, it is a property of oce- anic waters fully dependent on the water composition. The total absorption coefficient can be expressed as a sum of the individual contribution of four major absorp- tion coefficients of ocean water: pure seawater, aw(λ), phytoplankton, aph(λ), coloured dissolved organic matter, ag(λ), and suspended sediments, ad(λ): twphgd aaa aa (2) where aw (λ) is assumed to be a known constant [22,23]. In oceanic waters, IOPs of all the optically active sub- stances (except pure seawater) are assumed to covary with chlorophyll-a (Chl-a) concentration [1]. The stan- dard parameterizations of the IOP models have been proposed and widely used for the remote sensing appli- cations in the visible region, still the spectral characteri- zation of IOPs is much less documented and currently the open field of the investigation. Here, phytoplankton ab- sorption coefficient is the primary interest which is usu- ally described by a power, hyperbolic and 2nd order poly- nomial function [24]. Phytoplankton absorption coefficient is directly pro- portional to chlorophyll pigment concentrations; . ph aChl (3) Generally we can write it as, * ph ph aa Chl (4) aph where B ph aAChl is the chlorophyll-specific absorption coefficient (that varies widely depending on light history, nutrient availability, and species). A more robust relationship can be expressed based on Bricaud et al. [24-26]: (5) A B and where are the spectral coefficients, and vary widely depending on light history, nutrient avail- ability, and species composition. It is, of course, induced by the other optically active substances in the ocean. 2.2. Modelling Approach A new inversion model for determining aph(λ) is devel- oped based on the remote sensing reflectance ratio (Rrs(670)/Rrs(490)) and aph(λ) values in the visible and near-infrared wavelengths (400 nm - 700 nm) (Figure 1). This model gives estimates of the aph with specified Rrs(λ) Copyright © 2012 SciRes. ARS
 S. P. TIWARI, P. SHANMUGAM 21 values like the chlorophyll (Chl) parameterization. Similar parameterizations for determining the shapes of aph(λ) for all wavelengths (400 nm - 700 nm) are derived (Figure 2, Table 1). The relationships between the spectral absorp- tion coefficients of phytoplankton at 443 and 670 nm ver- sus the remote sensing reflectance ratio (Rrs(670)/Rrs(490)) provide the best-fit relationships with notably high correla- tion coefficients for these wavelengths. The model con- stants obtained from these relationships represent a third order polynomial equation which takes the form of equa- tion: 23 3 XaX 01 2ph aaaXa (6) where the X refers to the spectral band ratio of the re- mote sensing reflectance i.e., X = log10[Rrs(670)/Rrs(490)], and the phytoplankton absorption coefficient term can be expressed by non-linear cubic polynomial model given as below: 01 10 103 10 670 log 490 670 log log 490 rs ph rs rs rs R aaa R a R 2 23 670 490 rs rs a RR R (7) By using a linear extrapolation method the wavelength dependent constant coefficients are determined for all the wavelengths (400 nm - 700 nm) (Figure 2). In the above equations, λ is the wavelength, and a0, a1, a2, and a3 are the constants. The spectral values of the coefficients a0, a1, a2, and a3 of the cubic equation represent the variation of aph(λ) as a function of remote sensing reflectance ratio at 670 and 490 nm. Thus, this model can be easily ap- plied to any other independent data set and generalized for other types of phytoplankton absorption coefficient measurements. If some measurements are available, which have explicit non-linear dependence on aph(λ) (Equation (7)), and can be easily computed by the new model. The more precise aph model can be constructed with the use of some supplementary data in addition to more Rrs(λ), which has no explicit linear dependence on aph(λ). Figure 3 shows the tight relationships between the in-situ aph(443) and aph(670) and in-situ chlorophyll-a con- centrations (top panels). Similar relationships are ob- served between the model-derived aph(443) and aph(670) and in-situ chlorophyll-a concentrations (bottom panels), The range of aph(λ) value varied from 0.001 (m–1) - 1 (m–1) corresponding to a wide rage of the different Chl concentrations 0.01 (mg·m–3 ) - 100 (mg·m–3 ). 2.3. Assessment of Model Performance The performance of the new model is assessed by com- paring its predicted aph(λ) values with in situ aph(λ) val- ues. Three basic statistical methods are used such as the mean normalized bias (MNB), root mean square error (RMSE), and mean relative error (MRE). The accuracy of aph(λ) predictions (for all data acquired) is also as- sessed based on the slope (S), intercept (I), and correla- tion coefficient (R2) of the linear regression between the in-situ and predicted aph(λ) values Systematic and ran- dom errors are characterized by the mean relative error (MRE) and root mean square error (RMSE), respectively (IOCCG, 2006); these metrics are defined as: 12 N2 i1 log log RMSE N2 predicted insitu phi phi aa (8) 1 log log MRE 100% log predicted insitu Nphi phi insitu iphi aa a (9) 1 log log 1 MNB log redicted insitu Nphi phi insitu iphi aa Na (10) where redicted a insitu phi stands for the model-derived values, hi stands for the in-situ measurements, and N is the number of valid retrievals. The root mean square error (RMSE) for the derived aph(λ) is calculated based on the comparison of in-situ data with model data for the key SeaWiFS wavelengths 412, 443, 490, 510, 530, 555, 670, and 683 nm. These errors are calculated after the log transformation. Table 2 summarizes the statistical results of the new model validation with an in situ datasets. a 3. Data Sets 3.1. In-Situ Data An updated NASA bio-Optical Marine Algorithm Data- set (hereafter referred to as NOMAD) was obtained from the NASA Ocean Biology Processing Group. The NO- MAD dataset is a global, high quality in-situ bio-optical data set collected over a wide range of optical properties, trophic status, and geographical locations in open ocean waters, estuaries, and coastal waters (including Arabian Sea and coastal waters of India). It consists of two types of datasets; i.e., the in-situ bio-optical data set and con- current SeaWiFS observations of the remote sensing re- flectance Rrs(λ) at key wavelengths. These datasets are acquired over 4459 stations and stored in the system for use in algorithm development and validation (O’Reilly et al., 1998, 2000). The NOMAD in-situ data sets split into two data sets in the present study, namely NOMAD-1 and NOMAD-2. It should be noted that the NOMAD-1 in-situ data set is used for model parameterizations, whilst another suite of NOMAD-2 data set (composed of Copyright © 2012 SciRes. ARS
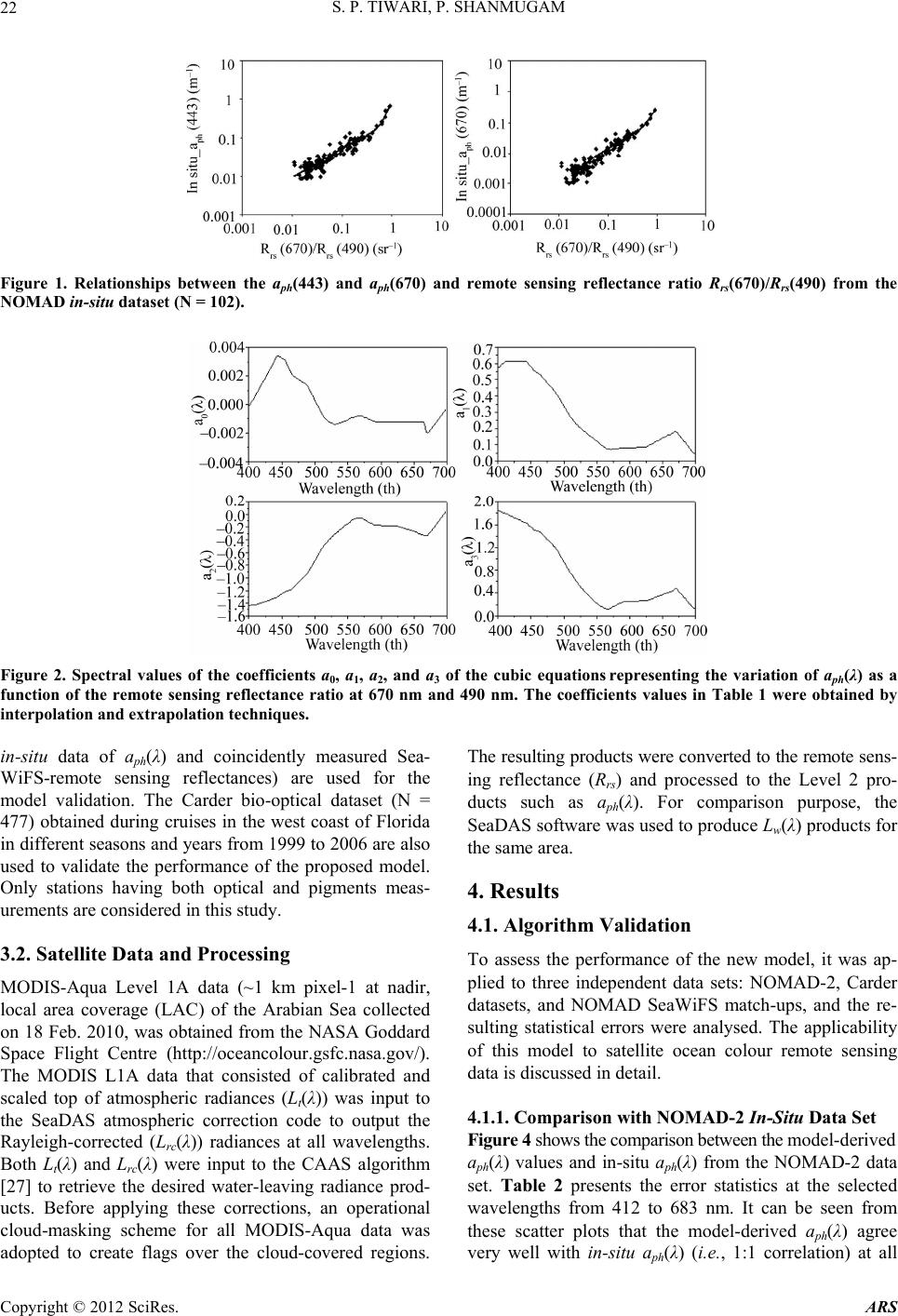 S. P. TIWARI, P. SHANMUGAM Copyright © 2012 SciRes. ARS 22 Figure 1. Relationships between the aph(443) and aph(670) and remote sensing reflectance ratio Rrs(670)/Rrs(490) from the NOMAD in-situ dataset (N = 102). Figure 2. Spectral values of the coefficients a0, a1, a2, and a3 of the cubic equations representing the variation of aph(λ) as a function of the remote sensing reflectance ratio at 670 nm and 490 nm. The coefficients values in Table 1 were obtained by interpolation and extrapolation techniques. in-situ data of aph(λ) and coincidently measured Sea- WiFS-remote sensing reflectances) are used for the model validation. The Carder bio-optical dataset (N = 477) obtained during cruises in the west coast of Florida in different seasons and years from 1999 to 2006 are also used to validate the performance of the proposed model. Only stations having both optical and pigments meas- urements are considered in this study. The resulting products were converted to the remote sens- ing reflectance (Rrs) and processed to the Level 2 pro- ducts such as aph(λ). For comparison purpose, the SeaDAS software was used to produce Lw(λ) products for the same area. 4. Results 4.1. Algorithm Validation 3.2. Satellite Data and Processing To assess the performance of the new model, it was ap- plied to three independent data sets: NOMAD-2, Carder datasets, and NOMAD SeaWiFS match-ups, and the re- sulting statistical errors were analysed. The applicability of this model to satellite ocean colour remote sensing data is discussed in detail. MODIS-Aqua Level 1A data (~1 km pixel-1 at nadir, local area coverage (LAC) of the Arabian Sea collected on 18 Feb. 2010, was obtained from the NASA Goddard Space Flight Centre (http://oceancolour.gsfc.nasa.gov/). The MODIS L1A data that consisted of calibrated and scaled top of atmospheric radiances (Lt(λ)) was input to the SeaDAS atmospheric correction code to output the Rayleigh-corrected (Lrc(λ)) radiances at all wavelengths. Both Lt(λ) and Lrc(λ) were input to the CAAS algorithm [27] to retrieve the desired water-leaving radiance prod- ucts. Before applying these corrections, an operational cloud-masking scheme for all MODIS-Aqua data was adopted to create flags over the cloud-covered regions. 4.1.1. Comparison with NOMAD-2 In-Situ Data Set Figure 4 shows the comparison between the model-derived aph(λ) values and in-situ aph(λ) from the NOMAD-2 data set. Table 2 presents the error statistics at the selected wavelengths from 412 to 683 nm. It can be seen from these scatter plots that the model-derived aph(λ) agree very well with in-situ aph(λ) (i.e., 1:1 correlation) at all
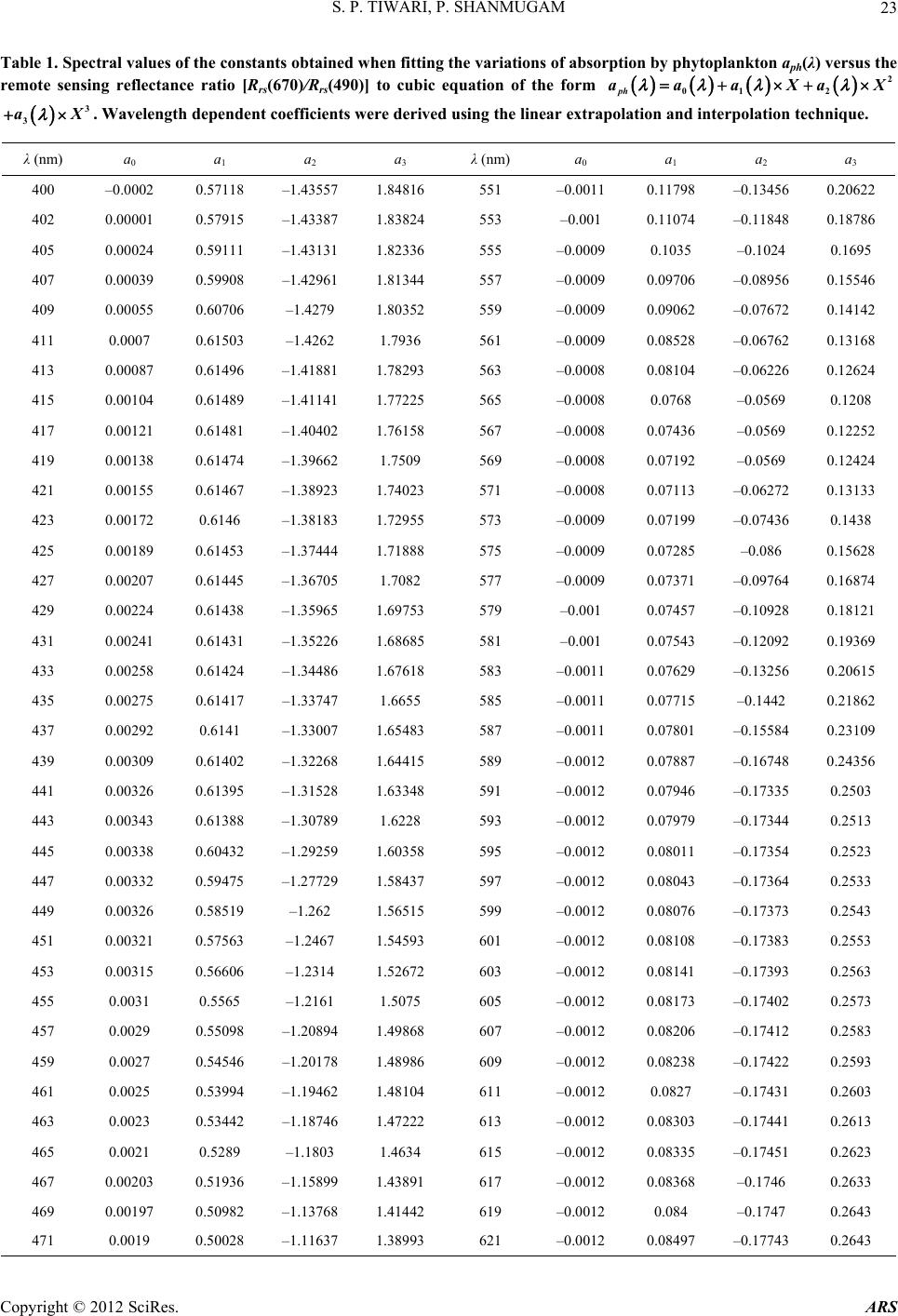 S. P. TIWARI, P. SHANMUGAM 23 Table 1. Spectral values of the constants obtained when fitting the variations of absorption by phytoplankton aph(λ) versus the remote sensing reflectance ratio [Rrs(670)/Rrs(490)] to cubic equation of the form 2 2 XaX 3 3 aX 01ph aaa . Wavelength dependent coefficients were derived using the linear extrapolation and interpolation technique. λ (nm) a0 a 1 a 2 a 3 λ (nm) a0 a 1 a 2 a 3 400 –0.0002 0.57118 –1.43557 1.84816 551 –0.0011 0.11798 –0.13456 0.20622 402 0.00001 0.57915 –1.43387 1.83824 553 –0.001 0.11074 –0.11848 0.18786 405 0.00024 0.59111 –1.43131 1.82336 555 –0.0009 0.1035 –0.1024 0.1695 407 0.00039 0.59908 –1.42961 1.81344 557 –0.0009 0.09706 –0.08956 0.15546 409 0.00055 0.60706 –1.4279 1.80352 559 –0.0009 0.09062 –0.07672 0.14142 411 0.0007 0.61503 –1.4262 1.7936 561 –0.0009 0.08528 –0.06762 0.13168 413 0.00087 0.61496 –1.41881 1.78293 563 –0.0008 0.08104 –0.06226 0.12624 415 0.00104 0.61489 –1.41141 1.77225 565 –0.0008 0.0768 –0.0569 0.1208 417 0.00121 0.61481 –1.40402 1.76158 567 –0.0008 0.07436 –0.0569 0.12252 419 0.00138 0.61474 –1.39662 1.7509 569 –0.0008 0.07192 –0.0569 0.12424 421 0.00155 0.61467 –1.38923 1.74023 571 –0.0008 0.07113 –0.06272 0.13133 423 0.00172 0.6146 –1.38183 1.72955 573 –0.0009 0.07199 –0.07436 0.1438 425 0.00189 0.61453 –1.37444 1.71888 575 –0.0009 0.07285 –0.086 0.15628 427 0.00207 0.61445 –1.36705 1.7082 577 –0.0009 0.07371 –0.09764 0.16874 429 0.00224 0.61438 –1.35965 1.69753 579 –0.001 0.07457 –0.10928 0.18121 431 0.00241 0.61431 –1.35226 1.68685 581 –0.001 0.07543 –0.12092 0.19369 433 0.00258 0.61424 –1.34486 1.67618 583 –0.0011 0.07629 –0.13256 0.20615 435 0.00275 0.61417 –1.33747 1.6655 585 –0.0011 0.07715 –0.1442 0.21862 437 0.00292 0.6141 –1.33007 1.65483 587 –0.0011 0.07801 –0.15584 0.23109 439 0.00309 0.61402 –1.32268 1.64415 589 –0.0012 0.07887 –0.16748 0.24356 441 0.00326 0.61395 –1.31528 1.63348 591 –0.0012 0.07946 –0.17335 0.2503 443 0.00343 0.61388 –1.30789 1.6228 593 –0.0012 0.07979 –0.17344 0.2513 445 0.00338 0.60432 –1.29259 1.60358 595 –0.0012 0.08011 –0.17354 0.2523 447 0.00332 0.59475 –1.27729 1.58437 597 –0.0012 0.08043 –0.17364 0.2533 449 0.00326 0.58519 –1.262 1.56515 599 –0.0012 0.08076 –0.17373 0.2543 451 0.00321 0.57563 –1.2467 1.54593 601 –0.0012 0.08108 –0.17383 0.2553 453 0.00315 0.56606 –1.2314 1.52672 603 –0.0012 0.08141 –0.17393 0.2563 455 0.0031 0.5565 –1.2161 1.5075 605 –0.0012 0.08173 –0.17402 0.2573 457 0.0029 0.55098 –1.20894 1.49868 607 –0.0012 0.08206 –0.17412 0.2583 459 0.0027 0.54546 –1.20178 1.48986 609 –0.0012 0.08238 –0.17422 0.2593 461 0.0025 0.53994 –1.19462 1.48104 611 –0.0012 0.0827 –0.17431 0.2603 463 0.0023 0.53442 –1.18746 1.47222 613 –0.0012 0.08303 –0.17441 0.2613 465 0.0021 0.5289 –1.1803 1.4634 615 –0.0012 0.08335 –0.17451 0.2623 467 0.00203 0.51936 –1.15899 1.43891 617 –0.0012 0.08368 –0.1746 0.2633 469 0.00197 0.50982 –1.13768 1.41442 619 –0.0012 0.084 –0.1747 0.2643 471 0.0019 0.50028 –1.11637 1.38993 621 –0.0012 0.08497 –0.17743 0.2643 Copyright © 2012 SciRes. ARS
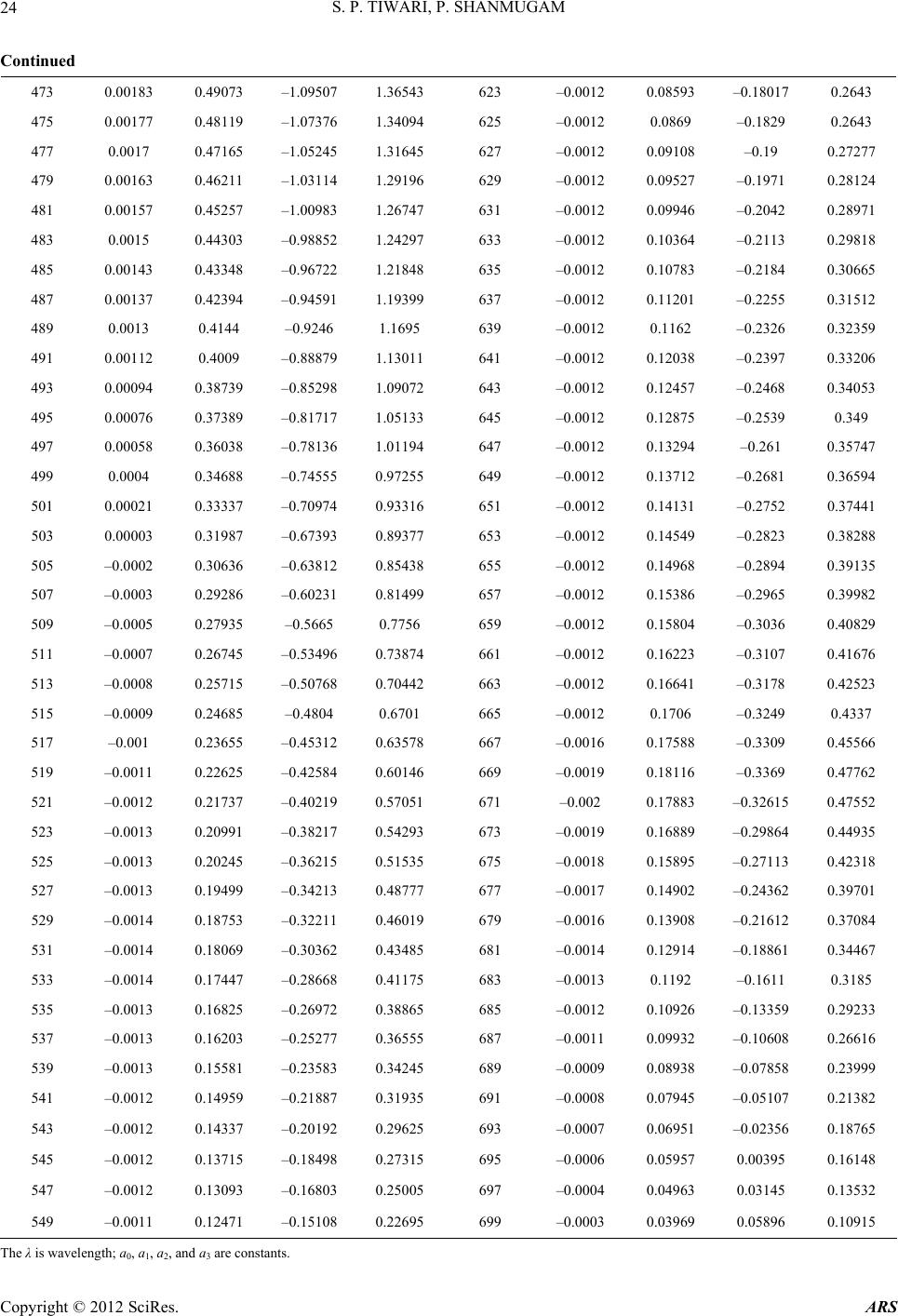 S. P. TIWARI, P. SHANMUGAM 24 Continued 473 0.00183 0.49073 –1.09507 1.36543 623 –0.0012 0.08593 –0.18017 0.2643 475 0.00177 0.48119 –1.07376 1.34094 625 –0.0012 0.0869 –0.1829 0.2643 477 0.0017 0.47165 –1.05245 1.31645 627 –0.0012 0.09108 –0.19 0.27277 479 0.00163 0.46211 –1.03114 1.29196 629 –0.0012 0.09527 –0.1971 0.28124 481 0.00157 0.45257 –1.00983 1.26747 631 –0.0012 0.09946 –0.2042 0.28971 483 0.0015 0.44303 –0.98852 1.24297 633 –0.0012 0.10364 –0.2113 0.29818 485 0.00143 0.43348 –0.96722 1.21848 635 –0.0012 0.10783 –0.2184 0.30665 487 0.00137 0.42394 –0.94591 1.19399 637 –0.0012 0.11201 –0.2255 0.31512 489 0.0013 0.4144 –0.9246 1.1695 639 –0.0012 0.1162 –0.2326 0.32359 491 0.00112 0.4009 –0.88879 1.13011 641 –0.0012 0.12038 –0.2397 0.33206 493 0.00094 0.38739 –0.85298 1.09072 643 –0.0012 0.12457 –0.2468 0.34053 495 0.00076 0.37389 –0.81717 1.05133 645 –0.0012 0.12875 –0.2539 0.349 497 0.00058 0.36038 –0.78136 1.01194 647 –0.0012 0.13294 –0.261 0.35747 499 0.0004 0.34688 –0.74555 0.97255 649 –0.0012 0.13712 –0.2681 0.36594 501 0.00021 0.33337 –0.70974 0.93316 651 –0.0012 0.14131 –0.2752 0.37441 503 0.00003 0.31987 –0.67393 0.89377 653 –0.0012 0.14549 –0.2823 0.38288 505 –0.0002 0.30636 –0.63812 0.85438 655 –0.0012 0.14968 –0.2894 0.39135 507 –0.0003 0.29286 –0.60231 0.81499 657 –0.0012 0.15386 –0.2965 0.39982 509 –0.0005 0.27935 –0.5665 0.7756 659 –0.0012 0.15804 –0.3036 0.40829 511 –0.0007 0.26745 –0.53496 0.73874 661 –0.0012 0.16223 –0.3107 0.41676 513 –0.0008 0.25715 –0.50768 0.70442 663 –0.0012 0.16641 –0.3178 0.42523 515 –0.0009 0.24685 –0.4804 0.6701 665 –0.0012 0.1706 –0.3249 0.4337 517 –0.001 0.23655 –0.45312 0.63578 667 –0.0016 0.17588 –0.3309 0.45566 519 –0.0011 0.22625 –0.42584 0.60146 669 –0.0019 0.18116 –0.3369 0.47762 521 –0.0012 0.21737 –0.40219 0.57051 671 –0.002 0.17883 –0.32615 0.47552 523 –0.0013 0.20991 –0.38217 0.54293 673 –0.0019 0.16889 –0.29864 0.44935 525 –0.0013 0.20245 –0.36215 0.51535 675 –0.0018 0.15895 –0.27113 0.42318 527 –0.0013 0.19499 –0.34213 0.48777 677 –0.0017 0.14902 –0.24362 0.39701 529 –0.0014 0.18753 –0.32211 0.46019 679 –0.0016 0.13908 –0.21612 0.37084 531 –0.0014 0.18069 –0.30362 0.43485 681 –0.0014 0.12914 –0.18861 0.34467 533 –0.0014 0.17447 –0.28668 0.41175 683 –0.0013 0.1192 –0.1611 0.3185 535 –0.0013 0.16825 –0.26972 0.38865 685 –0.0012 0.10926 –0.13359 0.29233 537 –0.0013 0.16203 –0.25277 0.36555 687 –0.0011 0.09932 –0.10608 0.26616 539 –0.0013 0.15581 –0.23583 0.34245 689 –0.0009 0.08938 –0.07858 0.23999 541 –0.0012 0.14959 –0.21887 0.31935 691 –0.0008 0.07945 –0.05107 0.21382 543 –0.0012 0.14337 –0.20192 0.29625 693 –0.0007 0.06951 –0.02356 0.18765 545 –0.0012 0.13715 –0.18498 0.27315 695 –0.0006 0.05957 0.00395 0.16148 547 –0.0012 0.13093 –0.16803 0.25005 697 –0.0004 0.04963 0.03145 0.13532 549 –0.0011 0.12471 –0.15108 0.22695 699 –0.0003 0.03969 0.05896 0.10915 The λ is wavelength; a0, a1, a2, and a3 are constants. Copyright © 2012 SciRes. ARS
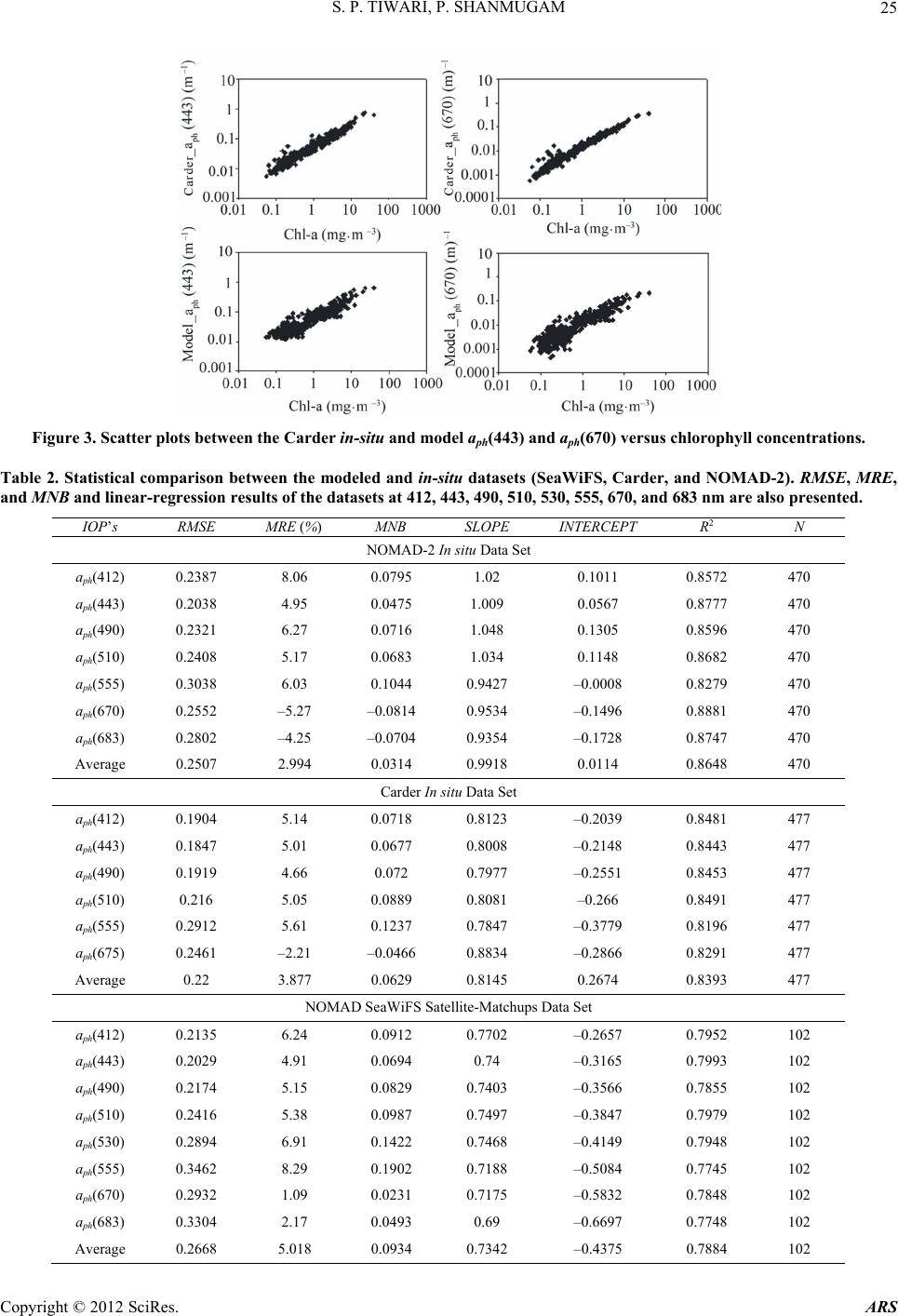 S. P. TIWARI, P. SHANMUGAM 25 Figure 3. Scatter plots between the Carder in-situ and model aph(443) and aph(670) versus chlorophyll concentrations. Table 2. Statistical comparison between the modeled and in-situ datasets (SeaWiFS, Carder, and NOMAD-2). RMSE, MRE, and MNB and linear-regression results of the datasets at 412, 443, 490, 510, 530, 555, 670, and 683 nm are also presented. IOP’s RMSE MRE (%) MNB SLOPE INTERCEPT R2 N NOMAD-2 In situ Data Set aph(412) 0.2387 8.06 0.0795 1.02 0.1011 0.8572 470 aph(443) 0.2038 4.95 0.0475 1.009 0.0567 0.8777 470 aph(490) 0.2321 6.27 0.0716 1.048 0.1305 0.8596 470 aph(510) 0.2408 5.17 0.0683 1.034 0.1148 0.8682 470 aph(555) 0.3038 6.03 0.1044 0.9427 –0.0008 0.8279 470 aph(670) 0.2552 –5.27 –0.0814 0.9534 –0.1496 0.8881 470 aph(683) 0.2802 –4.25 –0.0704 0.9354 –0.1728 0.8747 470 Average 0.2507 2.994 0.0314 0.9918 0.0114 0.8648 470 Carder In situ Data Set aph(412) 0.1904 5.14 0.0718 0.8123 –0.2039 0.8481 477 aph(443) 0.1847 5.01 0.0677 0.8008 –0.2148 0.8443 477 aph(490) 0.1919 4.66 0.072 0.7977 –0.2551 0.8453 477 aph(510) 0.216 5.05 0.0889 0.8081 –0.266 0.8491 477 aph(555) 0.2912 5.61 0.1237 0.7847 –0.3779 0.8196 477 aph(675) 0.2461 –2.21 –0.0466 0.8834 –0.2866 0.8291 477 Average 0.22 3.877 0.0629 0.8145 0.2674 0.8393 477 NOMAD SeaWiFS Satellite-Matchups Data Set aph(412) 0.2135 6.24 0.0912 0.7702 –0.2657 0.7952 102 aph(443) 0.2029 4.91 0.0694 0.74 –0.3165 0.7993 102 aph(490) 0.2174 5.15 0.0829 0.7403 –0.3566 0.7855 102 aph(510) 0.2416 5.38 0.0987 0.7497 –0.3847 0.7979 102 aph(530) 0.2894 6.91 0.1422 0.7468 –0.4149 0.7948 102 aph(555) 0.3462 8.29 0.1902 0.7188 –0.5084 0.7745 102 aph(670) 0.2932 1.09 0.0231 0.7175 –0.5832 0.7848 102 aph(683) 0.3304 2.17 0.0493 0.69 –0.6697 0.7748 102 Average 0.2668 5.018 0.0934 0.7342 –0.4375 0.7884 102 Copyright © 2012 SciRes. ARS
 S. P. TIWARI, P. SHANMUGAM Copyright © ARS 26 the wavelengths from 412 nm - 683 nm, producing low statistical errors (RMSE 0.2038 - 0. 3038 with an av- erage of 0.2507, MRE—5.270% - 8.06% with an aver- age of ~3.0 %, slope 0.935 - 1.048, R2 0.8279 - 0.888, intercept values——0.172 - 0.13). These results confirm that the aph(λ) predicted by the model at all these wave- lengths matched closely with their corresponding in-situ aph(λ) values very well, although slightly deviating from linearity at the higher end which may be due to prob- lems with the in-situ data sampling techniques. wide range of coastal and oceanic waters were used to assess the performance of the new model. Figure 5 compares the model estimates of aph(λ) with the in-situ measurements of aph(λ). The statistical results are sum- marized in Table 2 for all the selected wavelengths from 412 to 683 nm. Note that the model aph(λ) values show very good agreement with in-situ aph(λ) coefficient val- ues at 412, 443, 490, 555, and 670 nm, with low statistic- cal errors (RMSE 0.184 - 0. 291 with an average of 0.22, MRE—2.21% - 5.14% with an average of ~3.87%, slope 0.784 - 0.883, R2 0.819 - 0.849, intercept values –0.203 - –0.377). Compared with the previous validation, the RMSE is low, but other statistics become slightly worse. 4.1.2. Comparison with Carder In-Situ Dat a Set The in-situ aph(λ) made by Carder and his colleagues in a Figure 4. Comparison of modelled aph(λ) with in-situ data taken from the NOMAD-2 database at wavelengths from 412 to 683 (N = 470). 2012 SciRes.
 S. P. TIWARI, P. SHANMUGAM 27 However, the scatters of data are closely aligned with the 1:1 line indicating the validity of the model. 4.1.3. Comparison with SeaWiFS Satellite Data Set A validation of the model was also performed by com- paring satellite (SeaWiFS) estimates of aph(λ) with con- current in-situ aph(λ) measurements. Figure 6 shows the scatter plots of the predicted aph(λ) values versus the in-situ values. Table 2 presents the statistical analysis results at the wavelengths from 412 to 683 nm. When applied to the SeaWiFS match-up remote sensing reflec- tance, it can be seen that the aph(λ) values from the model closely agree with the in-situ data, without much scatters above or below the 1:1 line. The good agreement be- tween these data sets can also be observed in Table 2 (RMSE 0.202 - 0. 34 with an average of 0.26, MRE 1.09% - 8.29% with an average of ~5.01%), slope 0.69 - 0.77, R2 0.774 - 0.797, and intercept values –0.66 - –0.26). Although these errors are slightly higher than those observed with the previous data sets, the model still produced the observed aph(λ) values and resulted in low statistical errors. These results clearly indicate that the new model has the potential to retrieve accurately the aph(λ) values in both clear and turbid coastal waters, and would be useful for applications with remote sensing data in these waters. 4.1.4. Error Plots Figure 7 provides greater clarity in the variations of MRE between the derived and in-situ values of aph(λ) at 412 nm - 683 nm. Though the MRE values for the new model are notably small at all wavelengths for the three independent data sets, it shows a significant variability across these wavelengths. For the NOMAD-2 data set, the MRE value is high at 412 nm (~8.06%), and gradu- ally decreases towards the longer wavelengths. By con- trast, for the Carder and SeaWiFS match-up data sets, the MRE values are low in the blue wavelengths, increasing at the green wavelengths and sharply decreasing towards the longer wavelengths. However, these values are still in Figure 5. Comparison of modelled aph(λ) with in-situ data taken from the Carder database at wavelengths from 412 to 675 (N = 477). Copyright © 2012 SciRes. ARS
 S. P. TIWARI, P. SHANMUGAM 28 Figure 6. Comparisons of the modelled aph(λ) with those from the in-situ dataset (NOMAD SeaWiFS match-ups dataset) at the wavelengths from 412 to 683 nm (N = 102). the acceptable range as far as the aph(λ) modeling is con- cerned, because the current models produce very high errors in moderately turbid to highly turbid coastal wa- ters [28]. 4.2. Application to Satellite Ocean Colour Data To further assess the efficiency of the new aph model, the MODIS-Aqua Level 1A (~1 km/pixel at nadir) imagery acquired over bloomed waters of the Arabian Sea on 18 February 2010, was processed using a regional Complex water Atmospheric correction Algorithm Scheme (CAAS) [27] to avoid known issues with the SeaDAS atmos- pheric correction algorithm in these waters. Subsequently, the proposed model was applied to the atmospherically corrected imagery to envisage the phytoplankton absorp- tion coefficients at 443 and 670 nm. Figure 8(a) and Figure 8(b) show the regional distribution patterns of aph (443) and aph(670) in the Arabian Sea. As expected, the distribution patterns illustrate the influence of coastal waters on the phytoplankton absorption coefficients across the entire Arabian Sea during 18 Feb. 2010. Figure 8(c) pre- sents an example of aph spectra from this new model us- ing the same MODIS-Aqua data, which typically have two peaks (same as the measured aph spectra) around 443 and 670 nm. There is relatively lower absorption between 550 and 650 nm. These peaks and troughs are essentially due to the presence of Chl pigment. The width of the peaks around 443 and 670nm varies from sample to sam- ple, due to the change in accessory pigments present and Copyright © 2012 SciRes. ARS
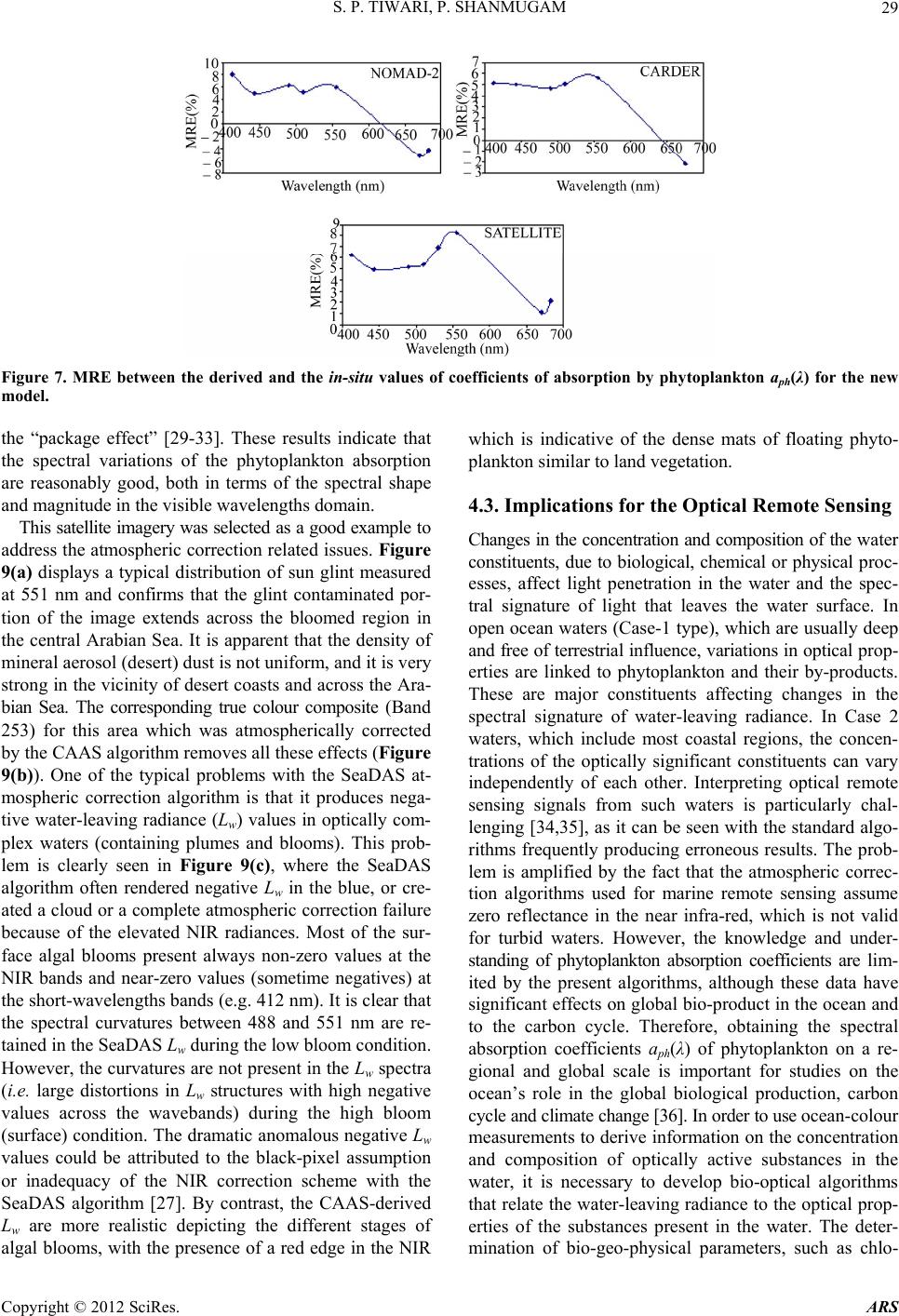 S. P. TIWARI, P. SHANMUGAM 29 Figure 7. MRE between the derived and the in-situ values of coefficients of absorption by phytoplankton aph(λ) for the new model. the “package effect” [29-33]. These results indicate that the spectral variations of the phytoplankton absorption are reasonably good, both in terms of the spectral shape and magnitude in the visible wavelengths domain. This satellite imagery was selected as a good example to address the atmospheric correction related issues. Figure 9(a) displays a typical distribution of sun glint measured at 551 nm and confirms that the glint contaminated por- tion of the image extends across the bloomed region in the central Arabian Sea. It is apparent that the density of mineral aerosol (desert) dust is not uniform, and it is very strong in the vicinity of desert coasts and across the Ara- bian Sea. The corresponding true colour composite (Band 253) for this area which was atmospherically corrected by the CAAS algorithm removes all these effects (Figure 9(b)). One of the typical problems with the SeaDAS at- mospheric correction algorithm is that it produces nega- tive water-leaving radiance (Lw) values in optically com- plex waters (containing plumes and blooms). This prob- lem is clearly seen in Figure 9(c), where the SeaDAS algorithm often rendered negative Lw in the blue, or cre- ated a cloud or a complete atmospheric correction failure because of the elevated NIR radiances. Most of the sur- face algal blooms present always non-zero values at the NIR bands and near-zero values (sometime negatives) at the short-wavelengths bands (e.g. 412 nm). It is clear that the spectral curvatures between 488 and 551 nm are re- tained in the SeaDAS Lw during the low bloom condition. However, the curvatures are not present in the Lw spectra (i.e. large distortions in Lw structures with high negative values across the wavebands) during the high bloom (surface) condition. The dramatic anomalous negative Lw values could be attributed to the black-pixel assumption or inadequacy of the NIR correction scheme with the SeaDAS algorithm [27]. By contrast, the CAAS-derived Lw are more realistic depicting the different stages of algal blooms, with the presence of a red edge in the NIR which is indicative of the dense mats of floating phyto- plankton similar to land vegetation. 4.3. Implications for the Optical Remote Sensing Changes in the concentration and composition of the water constituents, due to biological, chemical or physical proc- esses, affect light penetration in the water and the spec- tral signature of light that leaves the water surface. In open ocean waters (Case-1 type), which are usually deep and free of terrestrial influence, variations in optical prop- erties are linked to phytoplankton and their by-products. These are major constituents affecting changes in the spectral signature of water-leaving radiance. In Case 2 waters, which include most coastal regions, the concen- trations of the optically significant constituents can vary independently of each other. Interpreting optical remote sensing signals from such waters is particularly chal- lenging [34,35], as it can be seen with the standard algo- rithms frequently producing erroneous results. The prob- lem is amplified by the fact that the atmospheric correc- tion algorithms used for marine remote sensing assume zero reflectance in the near infra-red, which is not valid for turbid waters. However, the knowledge and under- standing of phytoplankton absorption coefficients are lim- ited by the present algorithms, although these data have significant effects on global bio-product in the ocean and to the carbon cycle. Therefore, obtaining the spectral absorption coefficients aph(λ) of phytoplankton on a re- gional and global scale is important for studies on the ocean’s role in the global biological production, carbon cycle and climate change [36]. In order to use ocean-colour measurements to derive information on the concentration and composition of optically active substances in the water, it is necessary to develop bio-optical algorithms that relate the water-leaving radiance to the optical prop- erties of the substances present in the water. The deter- mination of bio-geo-physica parameters, such as chlo- l Copyright © 2012 SciRes. ARS
 S. P. TIWARI, P. SHANMUGAM 30 Figure 8. (a,b) MODIS/AQUA data for 18th February 2010 over Arabian Sea, showing the model implementation for the fields of aph(443) and aph(670), (c) aph spectra obtained using the CAAS estimated reflectance values. rophyll concentration, based on water-leaving radiances, is relatively less complex for Case 1 waters where the spectral signature of the emerging light is mostly affected by phytoplankton and their by-products. The situation is very different in Case 2 coastal and estuarine waters that are characterized by higher optical and biological com- plexity, since other substances such as detritus, mineral particles, dissolved organic and inorganic material, also affect the light signal measured by the satellite sensor. The new aph(λ) model when applied with the CAAS al- gorithm particularly provides more reliable aph products for coastal and estuarine waters. 5. Discussion Though a wide variety of models-with varying degrees of complexity ranging from empirical to complex semi -analytical approaches-for determination of the aph(λ) coefficients were developed in the past, no models have the potential to provide reliable aph(λ) products in coastal waters. Thus, accurate estimation of aph(λ) in these wa- ters is still a daunting challenge. Hoge et al. [37,38] found that aph(λ) products at the wavelengths of 490, 510 and 555 nm are often estimated with large errors, when derived from a linear matrix inversion model. In another study, aph(675) was obtained by an inversion model us- ing the spectral remote sensing reflectance ratio between 412:443 and 443:551, which assumed the values of sev- eral algebraic constraints [30]. aph(675) values were de- termined by fitting a hyperbolic tangent function to aph(675) and defaulted to an empirical band ratio algo- rithm when solution was not reached. Many other reflec- tance-based models (inversion models) are also available in the literature such as QAA, LM, and GSM [19]. However, these models are applicable only in clear oce- anic waters, and provide no aph data at the longer wave- lengths (in the red domain). This could be because of the fact that the total absorption coefficient is generally dominated by pure seawater in oceanic waters, except for eutrophic waters when aph(λ) makes significant contribu- tions to the total absorption coefficients (a(λ)). Other limitations are that the derivation of aph at some specific wavelengths using one set of equations and at other wavelengths using different equations. After a thorough investigation and comparison of our results with those from the other models (not shown for brevity since it is already discussed in Shanmugam et al. [28], it was found Copyright © 2012 SciRes. ARS
 S. P. TIWARI, P. SHANMUGAM 31 Figure 9. MODIS/AQUA data for 18th February 2010 over Arabian Sea, showing the spectral variation of radiance retrieved from CAAS and SeaDAS in case of Low bloom, Medium bloom, High bloom, and Ve ry high bloom w a ters. Copyright © 2012 SciRes. ARS
 S. P. TIWARI, P. SHANMUGAM 32 that the new model is inherently more flexible for deter- mination of aph coefficients at any wavelengths in the visible domain. 6. Conclusion The new model has significant advantages over other models, since it relies on the Rrs(670) /Rrs(490) ratio which is not significantly influenced by materials other than phytoplankton. Validation of the model with inde- pendent in-situ data sets gave encouraging results. The model-predicted aph(λ) values were found to be in good agreement with in-situ data from coastal/oceanic waters. The model wavelengths of the SeaWiFS sensor (412 to 683 nm). Though the errors were low (e.g., MRE 8%), scatter plots showed slight differences between the model and in-situ aph(λ) values. The difference may arise due to several reasons; for instance, Rrs(λ) measurements made with different instruments with different calibration and correction procedures as well as environmental conditions. It was demonstrated that the atmospheric correction of satellite ocean colour data could introduce very high errors in complex waters. However, such problems could be eliminated when the water-leaving radiance signals are estimated with the CAAS algorithm. Thus, the aph(λ) model may be applied along with the CAAS algorithm, in order to retrieve more reliable aph(λ) values in opti- cally complex waters. A MODIS-Aqua example showed striking features of the distribution pattern of phyto- plankton absorption coefficients in bloomed waters in the Arabian Sea. In conclusion, this is the first study to esti- mate aph(λ) values at all the visible wavelengths. Thus, it provides new opportunities for improving the phyto- plankton inversion modelling based on the coefficients as given in Table 1. Our future effort will include addi- tional validation and tests based on more in-situ and sat- ellite data, and refining the model coefficients in order to provide more accurate phytoplankton absorption coef- ficients in complex waters. 7. Acknowledgements This work was supported by INCOIS under the grant (OEC/1011/102/INCO/PSHA) of the SATCORE pro- gram. The authors would like to thank the NASA Ocean Biology Processing Group for making available the global, high quality bio-optical (NOMAD) data set and the LAC MODIS-Aqua to this study. The authors would like to thank J. P. Cannizzaro and C. Hu for providing the bio-optical datasets of Prof. K. L. Carder. REFERENCES [1] A. Morel and L. Prieur, “Analysis of Variations in Ocean Colour,” Limnology and Oceanography, Vol. 22, No. 4, 1977, pp. 709-722. doi:10.4319/lo.1977.22.4.0709 [2] A. Morel and S. Maritorena, “Bio-Optical Properties of Oceanic Waters: A Reappraisal,” Journal of Geophysical research, Vol. 106, No. C4, 2001, pp. 7163-7180. doi:10.1029/2000JC000319 [3] H. R. Gordon, O. B. Brown, R. H. Evans, J. W. Brown, R. C. Smith, K. S. Baker and D. K. Clark, “A Semi-Analytic Model of Ocean Colour,” Journal of Geophysical Re- search, Vol. 9, No. D9, 1988, pp. 10909-10924. doi:10.1029/JD093iD09p10909 [4] A. Morel, “Optical Modeling of the Upper Ocean in Rela- tion to Its Biogenous Matter Content (Case I Waters),” Journal of Geophysical Research, Vol. 93, No. C9, 1988, pp. 10749-10768. doi:10.1029/JC093iC09p10749 [5] T. Platt and S. Sathyendranath, “Oceanic Primary Pro- duction: Estimation by Remote Sensing at Local and Re- gional Scales,” Science, Vol. 241, No. 4873, 1988, pp. 1613-1620. doi:10.1126/science.241.4873.1613 [6] H. M. Sosik and B. G. Mitchell, “Light Absorption by Phytoplankton, Photosynthetic Pigments and Detritus in the California Current System,” Deep Sea-Research, Vol. 42, No. 10, 1995, pp. 1717-1748. doi:10.1016/0967-0637(95)00081-G [7] E. H. S. Van Duin, G. Blom, F. J. Los, R. Maffione, R. Zimmerman, C. F. Cerco, D. Mark, E. P. H. Best, Van Duin, et al., “Modeling Underwater Light Climate in Re- lation to Sedimentation, Resuspension, Water Quality and Autotrophic Growth,” Hydrobiologia, Vol. 444, No. 1-3, 2001, pp. 25-42. doi:10.1023/A:1017512614680 [8] A. Morel, B. Gentili, M. Chami and J. Ras, “Bio-Optical Properties of High Chlorophyll Case 1 Waters, and of Yellow-Substance-Dominated Case 2 Waters,” Deep-Sea Research Part I, Vol. 53, No. 9, 2006, pp. 1439-1559. doi:10.1016/j.dsr.2006.07.007 [9] N. Hoepffner and S. Sathyendranath, “Determination of the Major Groups of Phytoplankton Pigments from the Absorption Spectra of Total Particulate Matter,” Journal of Geophysical Research, Vol. 98, No. C12, 1993, pp. 22789-22804. doi:10.1029/93JC01273 [10] A. Ciotti, M. R. Lewis and J. J. Cullen, “Assessment of the Relationship between Dominant Cell Size in Natural Phytoplakton Communities and the Spectral Shape of the Absorption Coefficient,” Limnology and Oceanography, Vol. 47, No. 2, 2002, pp. 404-417. doi:10.4319/lo.2002.47.2.0404 [11] J. R. Moisan, T. A. H. Moisan and M. A. Linkswiler, “An Inverse Modeling Approach to Estimating Phytoplankton Pigment Concentrations from Phytoplankton Absorption Spectra,” Journal of Geophysical Research, Vol. 116, No. C09018, 2011. [12] L. Prieur and S. Sathyendranath, “An Optical Classifica- tion of Coastal and Oceanic Waters Based on the Specific Spectral Absorption Curves of Phytoplankton Pigments, Dissolved Organic Matter, and Other Particulate Materi- als,” Limnology and Oceanography, Vol. 26, No. 4, 1981, pp. 671-689. doi:10.4319/lo.1981.26.4.0671 [13] E. Millan-Nunez, M. E. Sieracki, R. Millan-Nunez, J. R. Copyright © 2012 SciRes. ARS
 S. P. TIWARI, P. SHANMUGAM 33 Lara-Lara, G. Gaxiola-Castro and C. C. Trees, “Specific Absorption Coefficient and Phytoplankton Biomass in the Southern Region of the California Current,” Deep Sea Research, Part II, Vol. 51, No. 6-9, 2004, pp. 817-826. doi:10.1016/j.dsr2.2004.05.023 [14] S. A. Garver and D. Siegel, “Inherent Optical Property Inversion of Ocean Colour Spectra and Its Biogeochemi- cal Interpretation 1. Time Series from the Sargasso Sea,” Journal of Geophysical Research, Vol. 102, No. C8, 1997, pp. 18607-18625. doi:10.1029/96JC03243 [15] S. Maritorena, D. A. Siegel and A. R. Peterson, “Optimi- zation of a Semianalytical Ocean Colour Model for Global-Scale Applications,” Applied Optics, Vol. 41, No. 15, 2002, pp. 2705-2714. doi:10.1364/AO.41.002705 [16] Z. P. Lee, K. L. Carder and R. Arnone, “Deriving Inher- ent Optical Roperties from Water Colour: A Multi-Band Quasi-Analytical Algorithm or Optically Deep Waters,” Applied Optics, Vol. 41, No. 27, 2002, pp. 5755-5772. doi:10.1364/AO.41.005755 [17] P. Shanmugam, Y. H. Ahn, J. H. Ryu and B. Sundara- balan, “An Evaluation of Inversion Models for Retrieval of Inherent Optical Properties from Ocean Colour in Coastal and Open Sea Waters around Korea,” Journal of Oceanography, Vol. 66, No. 6, 2010, pp. 815-830. doi:10.1007/s10872-010-0066-0 [18] T. J. Smyth, G. F. Moore, T. Hirata and J. Aiken, “Se- mianalytical Model for the Derivation of Ocean Colour Inherent Optical Properties: Description, Implementation, and Performance Assessment,” Applied Optics, Vol. 45, No. 31, 2006, pp. 8116-8131. doi:10.1364/AO.45.008116 [19] E. Boss and C. Roesler, “Over Constrained Linear Matrix Inversion with Tatistical Selection,” In: Z. Lee, Ed., Re- mote Sensing of Inherent Optical Properties: Fundamen- tals, Tests of Algorithms, and Applications, IOCCG, Dartmouth, NS, Canada, IOCCG Rep. 5, 2006. [20] H. C. van de Hulst, “Light Scattering by Small Particles,” Dover, Mineola, 1981, 470 p. [21] R. W. Preisendorfer, “Application of Radiative Transfer theory to Light Measurements in the Sea,” Union of Geo- detic Geophysical Institute Monograph, Vol. 10, 1961, pp. 11-30. [22] M. Pope and E. S. Fry, “Absorption Spectrum (380 - 700 nm) of Pure Water. II. Integrating Cavity Measurements,” Applied Optics, Vol. 36, No. 33, 1997, pp. 8710-8723. doi:10.1364/AO.36.008710 [23] R. C. Smith and K. S. Baker, “Optical Properties of the Clearest Natural Waters,” Applied Optics, Vol. 20, No. 2, 1981, pp. 177-184. doi:10.1364/AO.20.000177 [24] A. Bricaud, A. Morel and L. Prieur, “A Absorption by Dissolved Organic Matter in the Sea (yellow substance) the UV and Visible Domains,” Limnology and Oceano- graphy, Vol. 26, No. 1, 1981, pp. 43-53. doi:10.4319/lo.1981.26.1.0043 [25] A. Bricaud, M. Babin, A. Morel and H. Claustre, “Variability in the Chlorophyll-Specific Absorption Co- efficients of Natural Phytoplankton: Analysis and Para- meterization,” Journal of Geophysical Research, Vol. 100, No. C7, 1995, pp. 13321-13332. doi:10.1029/95JC00463 [26] A. Bricaud, A. Morel, M. Babin, K. Allali and H. Claustre, “Variations of Light Absorption by Suspended Particles with Chlorophyll a Concentration in Oceanic (Case 1) Waters: Analysis and Implications for Bio- Optical Models,” Journal of Geophysical Research, Vol. 103, No. C13, 1998, pp. 31033-31044. doi:10.1029/98JC02712 [27] P. Shanmugam, “CAAS: An Atmospheric Correction Algorithm for the Remote Sensing of Complex Waters,” Annales Geophysicae, Vol. 30, No. 1, 2012, pp. 203-220. doi:10.5194/angeo-30-203-2012 [28] P. Shanmugam, Y. H. Ahn, J. H. Ryu and B. Sundara- balan, “An Evaluation of Inversion Models for Retrieval of Inherent Optical Properties from Ocean Colour in Coastal and Open Sea around Korea,” Journal of Ocean- ography, Vol. 66, 2010, pp. 815-830. doi:10.1007/s10872-010-0066-0 [29] K. L. Carder, S. K. Hawes, K. A. Baker, R. C. Smith, R. G. Steward and B. G. Mitchell, “Reflectance Model for Quantifying Chlorophyll a in the Presence of Productivity Degradation Products,” Journal of Geophysical Research , Vol. 96, No. C11, 1991, pp. 599-611. doi:10.1029/91JC02117 [30] K. L. Carder, F. R. Chen, Z. P. Lee, S. K. Hawes and D. Kamykowski, “Semianalytic Moderate-Resolution Imag- ing Spectrometer Algorithms for Chlorophyll-a and Absorption with Bio-Optical Domains Based on Nitrate Depletion Temperatures,” Journal of Geophysical Re- search, Vol. 104, No. C3, 1999, pp. 5403-5421. doi:10.1029/1998JC900082 [31] C. S. Yentsch and D. A. Phinney, “Spectral Fluorescence: An Ataaxonomic Tool for Studying the Structure of Phytoplankton Populations,” Journal of Plankton Re- search, Vol. 7, No. 5, 1985, pp. 617-632. doi:10.1093/plankt/7.5.617 [32] C. S. Yentsch and D. A. Phinney, “A Bridge between Ocean Optics and Microbial Ecology,” Limnology and Oceanography, Vol. 34, No. 8, 1989, pp. 1694-l705. doi:10.4319/lo.1989.34.8.1694 [33] J. E. O'Reilly, S. Moritorena, B. G. Mitchell, D. S. Seigel, K. L. Carder, S. A. Garver, et al., “Ocean Colour Chloro- phyll Algorithms for SeaWiFS,” Journal of Geophysical Research, Vol. 103, No. C11, 1998, pp. 937-953. [34] R. Miller, C. Del-Castillo and B. McKee, “Remote Sens- ing of Coastal Aquatic Environments,” Springer, Dord- recht, 2005, p. 347. doi:10.1007/978-1-4020-3100-7 [35] S. Sathyendranath, Ed., “Remote Sensing of Ocean Col- our in Coastal, and Other Optically Complex Waters,” Reports of the International Ocean-Colour Coordinating Group, IOCCG, Dartmouth, NS, Canada, Rep. 3, 2000, p. 140. [36] M. Tzortziou, A. Subramaniam, J. R. Herman, C. L. Gallegos, P. J. Neale and H. L. W. Jr., “Remote Sensing Reflectance and Inherent Optical Properties in the Mid Chesapeake Bay,” Estuarine, Coastal and Shelf Science, Vol. 72, No. 1-2, 2007, pp. 16-32. doi:10.1016/j.ecss.2006.09.018 [37] F. E Hoge and P. E. Lyon, “Satellite Retrieval of Inherent Copyright © 2012 SciRes. ARS
 S. P. TIWARI, P. SHANMUGAM Copyright © 2012 SciRes. ARS 34 Optical Properties by Linear Matrix Inversion of Oceanic Radiance Models: An Analysis of Model and Radiance Measurements,” Journal of Geophysical Research, Vol. 101, No. C7, 1996, pp. 16631-16648. doi:10.1029/96JC01414 [38] F. E. Hoge and P. E. Lyon, “Spectral Parameters of In- herent Optical Property Models: Method for Satellite Re- trieval by Matrix Inversion of an Oceanic Radiance Mod- el,” Applied Optics, Vol. 38, No. 9, 1999, pp. 1657-1662. doi:10.1364/AO.38.001657
|