 Modern Economy, 2012, 3, 408-423 http://dx.doi.org/10.4236/me.2012.34053 Published Online July 2012 (http://www.SciRP.org/journal/me) Network Economies for the Internet Hans W. Gottinger STRATEC, Munich, Germany Email: hg528@bingo-ev.de Received April 8, 2012; revised April 23, 2012; accepted May 20, 2012 ABSTRACT Most studies of resource allocation mechanisms have used a performance model of the resource, where the very concept of the resource is defined in terms of measurable qualities of the service such as utilization, thruput, response time (de- lay) and so on. Optimization of resource allocation is defined in terms of these measurable qualities. One novelty intro- duced by the economic approach is to design a system which takes into account the diverse Quality of Service (QoS) requirements of users, and therefore use multiobjective (utilities) optimization techniques to characterize and compute optimum allocations. Economic (mechanism design) modelling of computer and communication resource sharing uses a uniform paradigm described by two level modelling: QoS requirements as inputs into a performance model that is sub- ject to economic optimization. In a first step, one transforms QoS requirements of users to a performance (example: queueing service model). This model establishes quantifiable parameterization of resource allocation. For example, av- erage delay QoS requirement, when based on a FIFO queueing model, is a function of resources, bandwidth and buffer, and user traffic demands. These parameters are then used to establish an economic optimization model. The question of whether the resource is a piece of hardware, a network link, a software resource such as a database or a server, or a vir- tual network entity such as a TCP connection is not of primary importance. The first modeling transformation elimi- nates the details and captures the relevant behaviors and the optimization parameters. We consider a decentralized model of network and server economies , where we show efficient QoS provisioning and Pareto allocation of resources (network and server resources) among agents and suppliers, which are either network routes or servers (content provid- ers). We show how prices for resources are set at the suppliers based on the QoS demands from the agents. Keywords: Internet; Network Economy; Mechanism Design; Distributed Economic System; Economic Agents 1. Introduction With advances in computer and networking technology, numerous heterogeneous computers can be intercon- nected to provide a large collection of computing and communication resources. These systems are used by a growing and increasingly heterogeneous set of users which are identified with the present Internet. A macro- scopic view of distributed computer systems reveals the complexity of the organization and management of the resources and services they provide. The complexity arises from the system size (e.g. no. of systems, no. of users) and heterogeneity in applications (e.g. online transaction processing, e-commerce, multimedia, intelli- gent information search) and resources (CPU, memory, I/O bandwidth, network bandwidth and buffers, etc.). The complexity of resource allocation is further in- creased by several factors. First, in many distributed sys- tems, the resources are in fact owned by multiple or- ganizations. Second, the satisfaction of users and the performance of applications are determined by the si- multaneous application of multiple resources. For exam- ple, a multimedia server application requires I/O band- width to retrieve content, CPU time to execute server logic and protocols, and networking bandwidth to deliver the content to clients. The performance of applications may also be altered by trading resources. For example, a multimedia server application may perform better by releasing memory and acquiring higher CPU priority, resulting in smaller buffers for I/O and networking but improving the performance of the communication proto- col execution. Finally, in a large distributed system, the set of sys- tems, users and applications is continuously changing. In line with identifying the strategic factors of Internet en- terprises, we address some of the issues of Quality of Service (QoS) and pricing, and efficient allocation of resources (computational resources) in networks and systems. We review and consider problems of Quality of Ser- vice (QoS) provisioning, resource allocation and pricing in computer networks and information systems. We con- sider such complex systems as economies where multiple classes of users (consumers) compete for resources and C opyright © 2012 SciRes. ME
 H. W. GOTTINGER 409 services from suppliers: network providers and informa- tion providers (servers). The resources under contention are network bandwidth and buffers, server processing rate and memory. We model and solve problems using economic princi- ples of market mechanism, where resources are priced by suppliers based on demand, and users buy resources to satisfy their Quality of Service (QoS) needs. We focus on the issues of QoS provisioning, and the reasons in choos- ing economic paradigms to solve some of the problems. Resource allocation in networks relate to computa- tional models of networks, as developed in the works of Radner , Mount and Reiter 2, Mount and Reiter 3, Chap. 4, van Zandt 4 see also Gottinger 5, Chaps. 8, 9. Here they emanate from certain types of queueing systems, Kleinrock 6, Wolff 7 on generalized net- works. Network pricing could be looked at as a mecha- nism design problem (Hurwicz and Reiter 8). More specific mechanism design approaches for distributed networks and grid-type systems are covered by Narahari et al. 9 and Neumann et al. 10 see also Meinel and Tison 1. Massive complexity makes traditional approaches to resource allocation impractical in modern distributed systems such as the Internet. Traditional approaches attempt to optimize some system-wide measure of per- formance (e.g. overall average response time, thruput etc.). Optimization is performed either by a centralized algo- rithm with complete information, or by a decentralized consensus based algorithm. The current and future complexity of resource alloca- tion problems described makes it impossible to define an acceptable system-wide performance metric. What single, system-wide performance metric adequately reflects the performance objectives of multimedia server and an online transaction processing system? Centralized or consensus based algorithms are impractical in dynamic systems owned by multiple organizations. Resource al- location complexity due to decentralizations and hetero- geneity is also present in economic systems. In general, modern economies allocate resources in systems whose complexity overwhelms any algorithm or technique de- veloped for computer systems. As in economic mecha- nism design we focus on the similarities between com- plex distributed systems and economies. Competitive economic models could provide algorithms and tools for allocating resources in distributed computer systems (Deng and Graham 2) in particular Ackermann et al. 3, Chen et al. 4, Iong et al. 5. The tools used are algorithmic mechanism design, dynamic game theory, complexity and computational equilibrium. There is another motivation that has come about due to the commercialization of the Internet. The debate has begun in the area of multiple QoS levels and pricing. How should pricing be introduced to provide many ser- vice levels in the Internet? Should pricing be based on access cost or should it be based on usage and QoS re- ceived by the end user? Will usage based pricing help the Internet economy grow, and help in accounting and im- prove the efficiency of the Internet, and make users benefit much more? Similar issues are being investigated in the ATM networking community. We address some of the issues of QoS and pricing, and efficient allocation of resources (computational resources) in networks and systems. 2. Internet Resources The evolution of Internet pricing poses interesting re- source allocation problems. Flat-rate pricing has been a key condition that allowed the Internet to expand very fast. In the initial stages of the Internet flat rate pricing has been more prevalent in the US than in Europe which partially explains higher user diffusion rates in the US than in (most of) Europe or Japan among private users. But as the net has grown in size and complexity, not discounting engineering advances in network (manage- ment) technologies, it is becoming more obvious that other pricing schemes being able to cope with severe congestion and deadlock should come forward. New pricing schemes should not only be able to cope with a growing Internet traffic but also be able to foster applica- tion development and deployment vital to service pro- viders. Usage-based pricing of this new kind should make the internet attractive to many new users. Casual users will find it more affordable, while business users will find a more stable environment. Without an incentive to economize on usage, conges- tion and higher exposure to security breaches could be- come quite serious. The problem is more serious for data networks like the Internet than for other congestible transportation network resources because of the tremen- dously wide range of usage rates. A single user at a modern workstation can send a few bytes of email or put a load of hundreds Mbps on the network, for example, by downloading videos demanding as much as 1 Mbps. In the 1990s the maximum thruput on given backbones used to be only 45 Mbps, so it it was clear that even a few users with relatively inexpensive equipment could block the network. There was witness of these problems after American Online (AOL) introduced a flat fee for its internet services and experienced a massive and persis- tent breakdown with disgruntled customers. A natural response by shifting resources to expand technology will be expensive and not a satisfactory solution in the long run. Aside from breakthrough technological advances such as broadband that could mitigate but not eliminate the problem many proposals rely on voluntary efforts to Copyright © 2012 SciRes. ME
 H. W. GOTTINGER 410 control congestion. Many participants in congestion dis- cussions suggest that peer pressure and user ethics will be sufficient to control congestion costs. But as Mac Kie- Mason and Varian suggest we essentially have to deal with a problem like “the tragedy of the commons”, that is overgrazing the commons, e.g. by overusing a generally accessible communication network. A few pro- posals would require users to indicate the priority they want each of the sessions to receive, and for routers to be programmed to maintain multiple queues for each prior- ity class. The success of such schemes would depend on the users’ discipline to stick to the assigning of appropri- ate priorities to some of their traffic. On the other hand, priority scheduling flies in the face of “network neutral- ity” requested, that is, that all internet traffic should be delivered at the same speed and reliability. There are no effective sanction and incentive schemes that would con- trol such traffic, and therefore such a scheme is liable to be ineffective. This is why alternative pricing schemes have gained foremost attention and various approaches and models have been discussed in the network commu- nity (Shenker 7; Wang et al. 8). 2.1. Quality of Service (QoS) With the Internet we observe a single quality of service (QoS): “best effort packet service”. Packets are trans- ported first come, first-served with no guarantee of suc- cess. Some packets may experience severe delays, while others may be dropped and never arrive. Different kinds of data place different demands on network services (Shenker et al. 7) Email and file transfer requires 100 percent accuracy, but can easily tolerate delay. Real-time voice broadcasts require much higher bandwidth than file transfers, and can tolerate minor delays but they can’t tolerate significant distortion. Real-time video broadcasts have very low tolerance for delay and distortion. Because of these different requirements, network allocation algo- rithms should be designed to treat different types of traf- fic differently but the user must truthfully indicate which type of traffic he/she is preferring, and this would only happen through incentive compatible pricing schemes. Network pricing could be looked at as a mechanism design problem. The user can indicate the “type” of trans- mission and the workstation in turn reports this type to the network. To ensure truthful revelation of preferences, the reporting and billing mechanism must be incentive compatible. 2.2. Pricing Congestion The social cost of congestion is a result of the existence of network externalities. Charging for incremental capac- ity requires usage information. We need a measure of the user’s demand during the expected peak period of usage over some period, to determine the share of the incre- mental capacity requirement. In principle, it might seem that a reasonable approach would be to charge a premium price for usage during the pre-determined peak periods (a positive price if the base usage price is zero), as is rountinely done for electricity pricing (Wilson 9). However, in terms of internet usage, peak demand peri- ods are much less predictable than for other utility ser- vices. Since the use of computers would allow to sched- ule some activities during off-peak hours, in addition to different time zones around the globe, we face the prob- lem of shifting peaks. By identifying social costs for network externalities the suggestion by MacKie-Mason was directed toward a scheme for internalizing this cost as to impose a congestion price that is determined by a real-time Vickrey auction. The scheme requires that packets should be prioritized based on the value that the user puts on getting the packet through quickly. To do this, each user assigns his/her packets a bid measuring his/her willingness-to-pay (indicating effective demand) for immediate servicing. At congested routers, packets are prioritized based on bids. In line with the design of a Vickrey auction, in order to make the scheme incentive compatible, users are not charged the price they bid, but rather are charged the bid of the lowest priority packet that is admitted to the network. It is well-known that this mechanism provides the right incentives for truthful revelation (Nisan and Ronen 20). Such a scheme has a number of desirable characteristics. In particular, not only do those users with the highest cost of delay get served first, but the prices also send the right signals for capacity expansion in a competitive market for network services. If all of the congestion revenues are reinvested in new capacity, then capacity will be expanded to the point where its marginal value is equal to its marginal cost. 3. Quality of Service Parameters 3.1. Internet Communication Technologies: ATM and B-ISDN The Internet and Asynchronous Transfer Mode (ATM) have strongly positioned themselves for defining the fu- ture information infrastructure. The Internet is success- fully operating one of the popular information systems, the World Wide Web (WWW), which suggests that the information highway is forming on the Internet. However, such a highway is limited in the provision of advanced multimedia services such as those with guaranteed qual- ity of service (QoS). Guaranteed services are easier to support the ATM technology. Its capability far exceeds that of the current Internet, and it is expected to be used as the backbone technology for the future information infrastructure. ATM proposes a new communications Copyright © 2012 SciRes. ME
 H. W. GOTTINGER 411 paradigm. ATM allows integration of different types of services such as digital voice, video and data in a single network consisting of high speed links and switches. It supports a Broadband Integrated Services Digital Net- work (B-ISDN), so that ATM and B-ISDN are some- times used interchangeably, where ATM is referred to as the technology and B-ISDN as the underlying technical standard. ATM allows efficient utilization of network resources, and simplifies the network switching facilities compared to other proposed techniques in that it will only require one type of switching fabric (packet switch). This simplifies the network management process (Acker- mann et al. 3). The basic operation of ATM, and gen- erally of packet-switched networks, is based on statistical multiplexing. In order to provide QoS, the packets need to be served by certain scheduling (service) disciplines. Resource allocation algorithms depend heavily on the scheduling mechanism deployed. The scheduling is to be done at the entrance of the network as well as the switching points. The term “cell” designates the fixed- size packet in ATM networks. ATM allows variable bit rate sources to be statistically multiplexed. Statistical multiplexing produces more efficient usage of the chan- nel at the cost of possible congestion at the buffers of an ATM switch. When the congestion persists, buffer over- flow occurs, and cells are discarded (or packets are dropped). Therefore, resources (i.e. bandwidth and buffer space) need to be carefully allocated to meet the cell loss and the delay requirements of the user. The delay and the cell loss probability that the user wishes the network to guarantee are referred to as the QoS parameters. Overall, QoS is usually defined in terms of cell loss probability, delay bounds and other delay and drop-off parameters. How can one provide such QoS with guarantees? The general approach is to have an admission or performance algorithm that takes into account the traffic characteris- tics of the source and assigns suitable amounts of re- sources to the new connection during channel establish- ment. The admission algorithm is responsible for calcu- lating the bandwidth and buffer space necessary to meet the QoS requirements specified by the user. The algo- rithm depends on how the traffic is characterized and the service disciplines supported by the switches. Although the Internet is capable of transporting all types of digital information, it is difficult to modify the existing Internet to support some features that are vital for real time communications. One important feature to be supported is the provision of performance guarantees. The Internet uses the Internet Protocol (IP), in which each packet is forwarded independently of the others. The Internet is a connectionless network where any source can send packets any time at speeds that are nei- ther monitored nor negotiated. Congestion is bound to happen in this type of network. If congestion is to be avoided and real-time services are to be supported, then a negotiation (through pricing or rationing) between the user and the network is necessary. ATM is a connec- tion-oriented network that supports this feature. A virtual channel is established, and resources are reserved to pro- vide QoS prior to data transfer. This is referred to as channel establishment. 3.2. Traffic in B-ISDN In a B-ISDN environment high-bandwidth applications such as video, voice and data are likely to take advantage of compression. Different applications have different performance requirements, and the mechanisms to con- trol congestion should be different for each class of traf- fic. Classification of these traffic types is essential in providing efficient services. There are two fundamental classes of traffic in B-ISDN: real-time and non real-time (best effort) traffic. The majority of applications on the Internet currently are non-real-time ones (Paxon 21) based on TCP/IP. TCP/IP is being preserved with an ATM technology (Chao 22). The Internet can support data traffic well but not real-time traffic due to the limi- tations in the functionality of the protocols. B-ISDN needs to support both non-real-time and real-time traffic with QoS guarantees. Most data traffic requires low cell loss, but is insensitive to delays and other QoS parame- ters. Applications such as Telnet require a real-time re- sponse and should therefore be considered real-time ap- plications, the same applies to Voice of Internet Protocol (VOIP). Video is delay-sensitive and, unlike Telnet, re- quires high bandwidth. High throughput and low delay are required of the ATM switches for the network to support video services to the clients. This puts a con- straint on the ATM switch design in that switching should be done in hardware and the buffer sizes should be kept reasonably small to prevent long delays. On the other hand, best effort traffic tends to be bursty, and its traffic characteristics are hard to predict. This puts an- other, opposite constraint on an ATM switch, which re- quires large buffers at the switching point, further com- plicating its design. 3.3. Congestion Control Statistical multiplexing can offer the best use of re- sources, however, this is done at the price of possible congestion. Congestion in an ATM network can be han- dled basically in two ways: reactive control and preven- tive control. Reactive control mechanisms are commonly used in the Internet, where control is triggered to allevi- ate congestion after congestion has been detected. Typi- cal examples of reactive control are 1) explicit conges- tion notification (ECN); 2) node to node flow control and 3) selective cell discarding. In the more advanced pre- Copyright © 2012 SciRes. ME
 H. W. GOTTINGER 412 ventive control approach, congestion is avoided by allo- cating the proper amount of resources and controlling the rate of data transfers by properly scheduling cell depar- tures. Some examples of preventive control mechanisms are 1) admission and priority control; 2) usage parameter control and 3) traffic shaping. Reactive and preventive control can be used concur- rently, but most reactive controls are unsuitable for high- bandwidth real-time applications in an ATM network, since reactive control simply is not fast enough to handle congestion in time. Therefore, preventive control is more appropriate for high speed networks. 3.4. Service Discipline Traffic control occurs at various places in the network. First, the traffic entering the network is controlled at the input, second, the traffic is controlled at the switching nodes. In either case, traffic is controlled by scheduling the cell departures. There are various ways how to sched- ule departure times, and these mechanisms are part of service disciplines. The service discipline must transfer traffic at a given bandwidth by scheduling the cells and make sure that it does not exceed the buffer space re- served (or the delay bound assigned) for each channel. These functions are usually build into the hardware of the ATM switch and into the switch controller. When im- plementing a service discipline in an ATM network, it is important to choose it simple enough that it can be easily integrated into an ATM switch. However, the discipline must support the provision of quality of service guaran- tees. This also means that the service discipline is re- sponsible for protecting “well-behaved” traffic from the “ill-behaved” traffic and must be able to provide certain levels of QoS guarantees. The service discipline also needs to be flexible enough to satisfy the diverse re- quirements of a variety of traffic types, and to be effi- cient, that is, to permit a high utilization of the network. Various service disciplines have been proposed, and many of them have been investigated thoroughly and compared. An important class is that of disciplines used in rate-allocating servers. 3.5. Bandwidth-Buffer Tradeoff A simple example on the representation of QoS parame- ters is the bandwidth-buffer tradeoff. Bandwidth can be traded for buffer space and vice versa to provide the same QoS. If a bandwidth is scarce, then a resource pair that uses less bandwidth and more buffer space should be used (Gottinger 23). Resource pricing is targeted to exploit this tradeoff to achieve efficient utilization of the available resources. The pricing concept for a scarce re- source is well-known in economics, but in the context of exploiting the bandwidth-buffer tradeoff, Low and Va- raiya 24 use non-linear optimization theory to deter- mine centralized optimal shadow prices in large networks. With respect to large scale application, however, the complex optimization process limits the frequency of pricing updates, which causes inaccurate information about available resources. In order to make pricing in the context of a buffer-bandwidth tradeoff more adjustable and flexible it should be based on decentralized pricing procedures according to competitive bidding in large markets where prices will be optimal prices if the mar- kets are efficient. This would also allow flexible pricing which results in accurate representation of available re- sources in that prices are updated as the instance connect request arrives. The subsequent procedure is based on distributed pricing as a more feasible alternative to opti- mal pricing. 4. The Rationale of Economic Models in Networking There are intrinsic interfaces between human information processing and networking that show the usefulness of economic modeling. Decentralization: in an economy, decentralization is provided by the fact that economic models consist of agents which selfishly attempt to achieve their goals. There are two types of economic agents: suppliers and consumers. A consumer attempts to optimize its individ- ual performance criteria by obtaining the resources it requires, and is not concerned with system-wide perfor- mance. A supplier allocates its individual resources to consumers. A supplier’s sole goal is to optimize its indi- vidual resources to consumers. A supplier’s sole goal is to optimize its individual satisfaction (profit) derived from its choice of resource allocation to consumers. Models of economic agents are summarized in the Ap- pendix. Limiting Complexity: economic models provide sev- eral interesting contributions to resource sharing algo- rithms. The first is a set of tools for limiting the com- plexity by decentralizing the control of resources. The second is a set of mathematical models that can yield several new insights into resource sharing problems. Pricing and Performance: most economic models in- troduce money and pricing as the technique for coordi- nating the selfish behavior of agents. Each consumer is endowed with money that it uses to purchase required resources. Each supplier owns a set of resources, and charges consumers for the use of its resources. The sup- plier prices its resources based on the demand by the agents, and the available supply. Consumers buy re- sources or services such that the benefit they receive is maximized. Consumer-agents buy resources based on maximizing performance criteria. As a whole the system Copyright © 2012 SciRes. ME
 H. W. GOTTINGER 413 performance is determined by some combination of the individual performance criteria. Usage Accounting, Billing and Dimensioning: by us- ing economic models for service provisoning in distrib- uted systems, accounting for QoS becomes an important task for suppliers, as they have to keep track of the re- source usage in order to price the resources, and thereby charge or bill the users for QoS. In addition, pricing can be used to understand the user demands and thereby di- mension systems appropriately. Administrative Domains: often large distributed sys- tems and computer networks spread over several do- mains, the control of resources is shared by multiple or- ganizations that own distinct parts of the network. In such an environment, each organization will have a set of services that it supports. Economic principles of pricing and competition provide several valuable insights into decentralized control mechanisms between the multiple organizations and efficient service provisioning. Scalability: a key issue in designing architectures for services in large computer networks and distributed sys- tems is scalability. With the ever growing demand for new services, flexible service architectures that can scale to accommodate new services are needed. Economic models of competition provide, in a natural fashion, mechanisms for scaling services appropriately based on service demand and resource availability. 5. Modelling Approaches Most studies of resource allocation mechanisms have used a performance model of the resource, where the very concept of the resource is defined in terms of meas- urable qualities of the service such as utilization, thruput, response time (delay) and so on. Optimization of re- source allocation is defined in terms of these measurable qualities. One novelty introduced by the economic ap- proach is to design a system which takes into account the diverse QoS requirements of users, and therefore use multiobjective (utilities)optimization techniques to char- acterize and compute optimum allocations. Economic modeling of computer and communication resource sharing uses a uniform paradigm described by two level modeling: QoS requirements as inputs into a perform- ance model that is subject to economic optimization. In the first step, one transforms QoS requirements of users to a performance (example: queueing service model).This model establishes quantifiable parameteriza- tion of resource allocation. For example, average delay QoS requirement, when based on a FIFO queueing model, is a function of resources, bandwidth and buffer, and user traffic demands. These parameters are then used to estab- lish an economic optimization model. The question of whether the resource is a piece of hardware, a network link, a software resource such as a database or a server, or a virtual network entity such as a TCP connection is not of primary importance. The first modeling transfor- mation eliminates the details and captures the relevant behaviors and the optimization parameters. Our approach evolves in the following sequence. Many users present QoS demands, which are translated into demands on resources based on a performance model. The suppliers compute the optimal allocations based on principles of economic optimization and market mechanisms. Once the optimization is done, the results provide inputs to mechanisms for QoS provisioning, such as scheduling of resources and admission of users in networks and load balancing in distributed systems. We present briefly an overview of the problems and contri- butions 5.1. Optimal Allocation and QoS We motivate and solve a problem of allocating resources and providing services (QoS) to several classes of users at a single link. The resources at the link are buffer space and bandwidth. The link (network provider) prices per unit buffer and bandwidth resources. The consumers (user traffic classes), via economic agents, buy resources such that their QoS needs are satisfied. The network pro- vider prices resources based on demand from the con- sumers. The ingredients are as follows: Economic models: we use competitive economic models to determine the resource partitions between user traffic classes, which compete to obtain buffer and bandwidth resources from the switch suppliers. Optimal allocations using economic principles: we look for Pareto optimal allocations that satisfy QoS needs of agents. Agents represent QoS via utility functions which capture the multiple performance objectives. Pricing based on QoS: we compute equilibrium prices based on the QoS demands of consumers. Prices are set such that the market demand and supply are met. Prices help in determining the cost of providing a ser- vice. Priorities: using the economic framework, we show a simple way to support priority service among the user-classes (or agents). Decentralization: we show a natural separation be- tween the interactions of the user-classes (represented by agents) and the network switch suppliers. The in- teraction is purely competitive and market based. This decentralization promotes scalable network system design. 5.2. Scheduling and Pricing Mechanisms We consider a dynamic system where sessions arrive and Copyright © 2012 SciRes. ME
 H. W. GOTTINGER 414 leave a traffic class, and demand fluctuates over time. In such a setting, we investigate practical mechanisms, such as packet level scheduling to provide bandwidth and buffer guarantees, admission control mechanisms to pro- vide class QoS guarantees, practical pricing to capture the changing demand, and charging mechanisms for user sessions within a class. Scheduling algorithms for class based QoS provi- sioning: we provide novel scheduling mechanisms, which allocates bandwidth and buffer for meeting the demand from traffic classes. The scheduling mecha- nism allocates bandwidth, which is computed from the economic optimization. Admission Region and Control: we compute the ad- mission control region of the agents on the economic model. Due to the natural separation between who controls the admission of sessions into the traffic class, the admission region can be determined. We propose simple pricing models which capture the changing demand, and are easy to implement. We also propose novel QoS based charging mechanisms for sessions in a class with applications to charging in ATM Networks and Integrated Services Internet. We first consider a network economy, of many parallel routes or links, where several agents (representing user classes) compete for resources from several suppliers, where each supplier represents a route (or a path) be- tween a source and destination. Agents buy resources from suppliers based on the QoS requirements of the class they represent. Suppliers price resources, inde- pendently, based on demand from the agents. The sup- pliers connect consumers to information providers, who are at the destination; the flow of information is from information providers to the consumers. We formulate and solve problems of resource allocation and pricing in such an environment. We then consider a server economy in a distributed system. Again, we use a similar model of interaction between agents and suppliers (servers). The servers sell computational resources such as processing rate and memory to the agents for a price. The prices of resources are set independently by each server based on QoS de- mand from the agents. Agents represent user classes such as transactions in database servers or sessions for Web servers that have QoS requirements such as response time. Using such economic models, our contributions are as follows: We propose a decentralized model of network and server economies, where we show efficient QoS pro- visioning and Pareto allocation of resources (network and server resources) among agents and suppliers, which are either network routes or servers (content providers). We show how prices for resources are set at the sup- pliers based on the QoS demands from the agents. We propose alternative dynamic routing algorithms and admission control mechanisms based on QoS preferences by the user classes for the network econ- omy, and we propose a simple way to perform trans- action routing. We also show static optimal routing policies by the agents for the network and server economies. 5.3. Network and Server Economies Consider a large scale distributed information system with many consumers and suppliers. Suppliers are con- tent providers such as web servers, digital library servers, multimedia database and transaction servers. Consumers request for and access information objects from the vari- ous suppliers and pay a certain fee or no fee at all for the services rendered. Consider that third party suppliers provide information about suppliers to consumers in order to let consumers find and choose the right set of suppliers. Access and dissemination: consumers query third- party providers for information about the suppliers, such as services offered and the cost (price). Likewise, suppli- ers advertise their services and the costs via the third party providers in order to attract consumers. Consumers prefer an easy and simple way to query for supplier in- formation, and suppliers prefer to advertise information securely and quickly across many regions or domains. For example, consider a user who wishes to view a mul- timedia object (such as a video movie). The user would like to know about the suppliers of this object, and the cost of retrieval of this object from each supplier. Performance requirements: users wish to have good response time for their search results once the queries are submitted. However, there is a tradeoff. For more infor- mation about services offered, advanced searching mechanisms are needed, but at the cost of increased re- sponse time. In other words, users could have prefer- ences over quality of search information and response time. For example, users might want to know the service costs in order to view a specific information object. In large networks, there could be many suppliers of this object, and users may not want to wait forever to know about all the suppliers and their prices. Instead, they would prefer to get as much information as possible within a certain period of time (response time). From the above example, in order to let many con- sumers find suppliers, a scalable decentralized architec- ture is needed for information storage, access and up- dates. Naming of services and service attributes of suppliers becomes a challenging issue when hundreds of suppliers spread across the globe. A simple naming scheme to Copyright © 2012 SciRes. ME
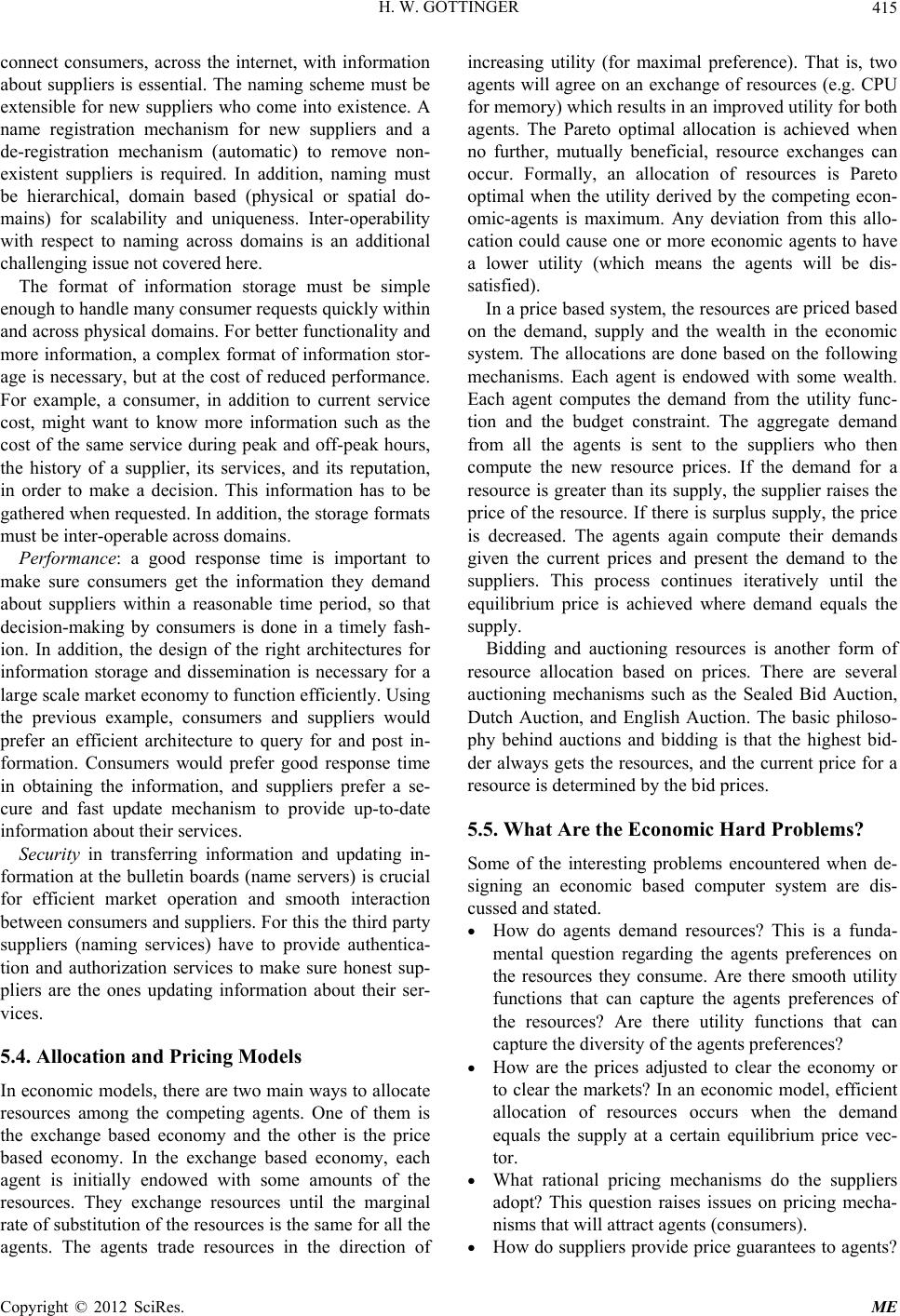 H. W. GOTTINGER 415 connect consumers, across the internet, with information about suppliers is essential. The naming scheme must be extensible for new suppliers who come into existence. A name registration mechanism for new suppliers and a de-registration mechanism (automatic) to remove non- existent suppliers is required. In addition, naming must be hierarchical, domain based (physical or spatial do- mains) for scalability and uniqueness. Inter-operability with respect to naming across domains is an additional challenging issue not covered here. The format of information storage must be simple enough to handle many consumer requests quickly within and across physical domains. For better functionality and more information, a complex format of information stor- age is necessary, but at the cost of reduced performance. For example, a consumer, in addition to current service cost, might want to know more information such as the cost of the same service during peak and off-peak hours, the history of a supplier, its services, and its reputation, in order to make a decision. This information has to be gathered when requested. In addition, the storage formats must be inter-operable across domains. Performance: a good response time is important to make sure consumers get the information they demand about suppliers within a reasonable time period, so that decision-making by consumers is done in a timely fash- ion. In addition, the design of the right architectures for information storage and dissemination is necessary for a large scale market economy to function efficiently. Using the previous example, consumers and suppliers would prefer an efficient architecture to query for and post in- formation. Consumers would prefer good response time in obtaining the information, and suppliers prefer a se- cure and fast update mechanism to provide up-to-date information about their services. Security in transferring information and updating in- formation at the bulletin boards (name servers) is crucial for efficient market operation and smooth interaction between consumers and suppliers. For this the third party suppliers (naming services) have to provide authentica- tion and authorization services to make sure honest sup- pliers are the ones updating information about their ser- vices. 5.4. Allocation and Pricing Models In economic models, there are two main ways to allocate resources among the competing agents. One of them is the exchange based economy and the other is the price based economy. In the exchange based economy, each agent is initially endowed with some amounts of the resources. They exchange resources until the marginal rate of substitution of the resources is the same for all the agents. The agents trade resources in the direction of increasing utility (for maximal preference). That is, two agents will agree on an exchange of resources (e.g. CPU for memory) which results in an improved utility for both agents. The Pareto optimal allocation is achieved when no further, mutually beneficial, resource exchanges can occur. Formally, an allocation of resources is Pareto optimal when the utility derived by the competing econ- omic-agents is maximum. Any deviation from this allo- cation could cause one or more economic agents to have a lower utility (which means the agents will be dis- satisfied). In a price based system, the resources are priced based on the demand, supply and the wealth in the economic system. The allocations are done based on the following mechanisms. Each agent is endowed with some wealth. Each agent computes the demand from the utility func- tion and the budget constraint. The aggregate demand from all the agents is sent to the suppliers who then compute the new resource prices. If the demand for a resource is greater than its supply, the supplier raises the price of the resource. If there is surplus supply, the price is decreased. The agents again compute their demands given the current prices and present the demand to the suppliers. This process continues iteratively until the equilibrium price is achieved where demand equals the supply. Bidding and auctioning resources is another form of resource allocation based on prices. There are several auctioning mechanisms such as the Sealed Bid Auction, Dutch Auction, and English Auction. The basic philoso- phy behind auctions and bidding is that the highest bid- der always gets the resources, and the current price for a resource is determined by the bid prices. 5.5. What Are the Economic Hard Problems? Some of the interesting problems encountered when de- signing an economic based computer system are dis- cussed and stated. How do agents demand resources? This is a funda- mental question regarding the agents preferences on the resources they consume. Are there smooth utility functions that can capture the agents preferences of the resources? Are there utility functions that can capture the diversity of the agents preferences? How are the prices adjusted to clear the economy or to clear the markets? In an economic model, efficient allocation of resources occurs when the demand equals the supply at a certain equilibrium price vec- tor. What rational pricing mechanisms do the suppliers adopt? This question raises issues on pricing mecha- nisms that will attract agents (consumers). How do suppliers provide price guarantees to agents? Copyright © 2012 SciRes. ME
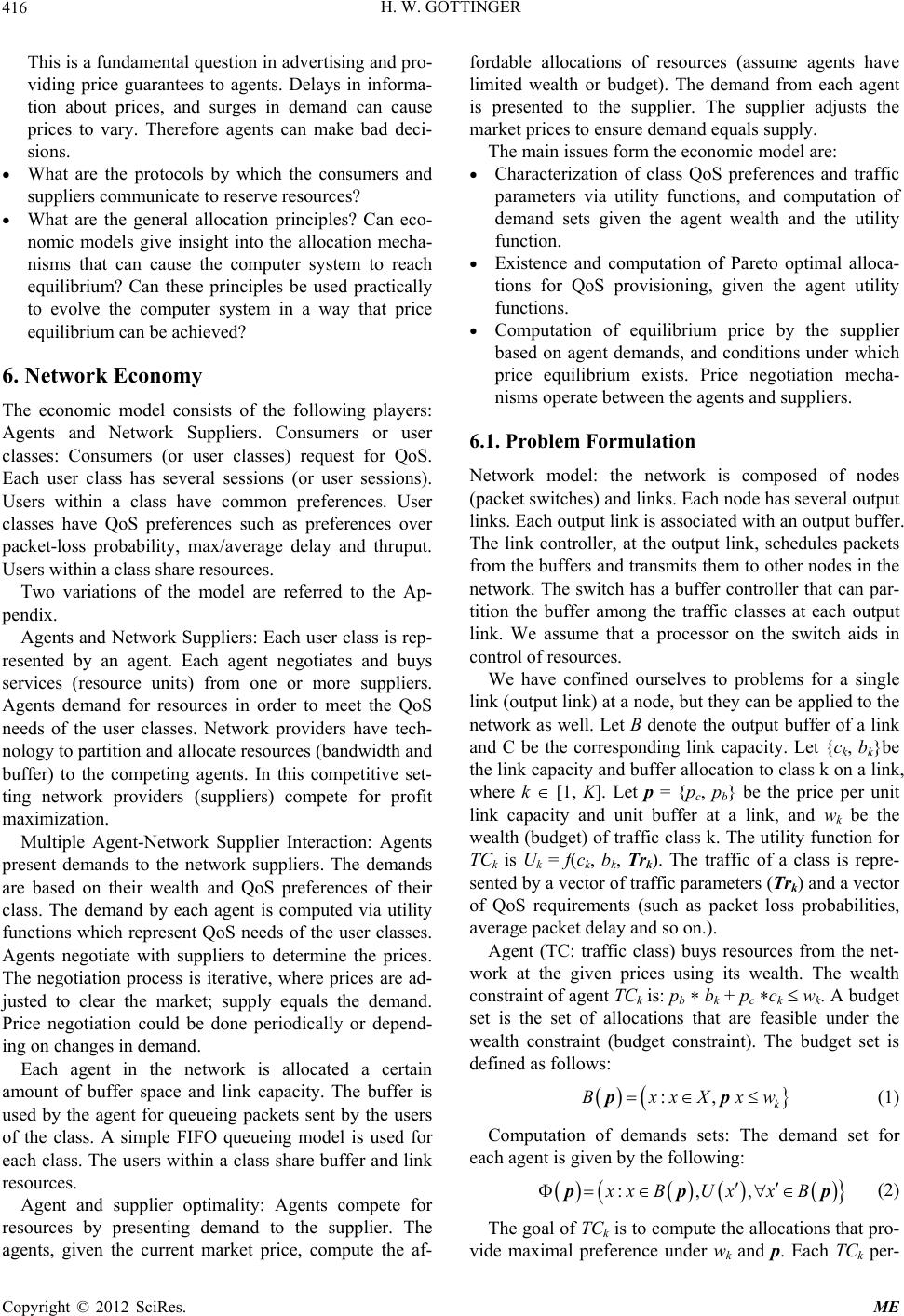 H. W. GOTTINGER 416 This is a fundamental question in advertising and pro- viding price guarantees to agents. Delays in informa- tion about prices, and surges in demand can cause prices to vary. Therefore agents can make bad deci- sions. What are the protocols by which the consumers and suppliers communicate to reserve resources? What are the general allocation principles? Can eco- nomic models give insight into the allocation mecha- nisms that can cause the computer system to reach equilibrium? Can these principles be used practically to evolve the computer system in a way that price equilibrium can be achieved? 6. Network Economy The economic model consists of the following players: Agents and Network Suppliers. Consumers or user classes: Consumers (or user classes) request for QoS. Each user class has several sessions (or user sessions). Users within a class have common preferences. User classes have QoS preferences such as preferences over packet-loss probability, max/average delay and thruput. Users within a class share resources. Two variations of the model are referred to the Ap- pendix. Agents and Network Suppliers: Each user class is rep- resented by an agent. Each agent negotiates and buys services (resource units) from one or more suppliers. Agents demand for resources in order to meet the QoS needs of the user classes. Network providers have tech- nology to partition and allocate resources (bandwidth and buffer) to the competing agents. In this competitive set- ting network providers (suppliers) compete for profit maximization. Multiple Agent-Network Supplier Interaction: Agents present demands to the network suppliers. The demands are based on their wealth and QoS preferences of their class. The demand by each agent is computed via utility functions which represent QoS needs of the user classes. Agents negotiate with suppliers to determine the prices. The negotiation process is iterative, where prices are ad- justed to clear the market; supply equals the demand. Price negotiation could be done periodically or depend- ing on changes in demand. Each agent in the network is allocated a certain amount of buffer space and link capacity. The buffer is used by the agent for queueing packets sent by the users of the class. A simple FIFO queueing model is used for each class. The users within a class share buffer and link resources. Agent and supplier optimality: Agents compete for resources by presenting demand to the supplier. The agents, given the current market price, compute the af- fordable allocations of resources (assume agents have limited wealth or budget). The demand from each agent is presented to the supplier. The supplier adjusts the market prices to ensure demand equals supply. The main issues form the economic model are: Characterization of class QoS preferences and traffic parameters via utility functions, and computation of demand sets given the agent wealth and the utility function. Existence and computation of Pareto optimal alloca- tions for QoS provisioning, given the agent utility functions. Computation of equilibrium price by the supplier based on agent demands, and conditions under which price equilibrium exists. Price negotiation mecha- nisms operate between the agents and suppliers. 6.1. Problem Formulation Network model: the network is composed of nodes (packet switches) and links. Each node has several output links. Each output link is associated with an output buffer. The link controller, at the output link, schedules packets from the buffers and transmits them to other nodes in the network. The switch has a buffer controller that can par- tition the buffer among the traffic classes at each output link. We assume that a processor on the switch aids in control of resources. We have confined ourselves to problems for a single link (output link) at a node, but they can be applied to the network as well. Let B denote the output buffer of a link and C be the corresponding link capacity. Let {ck, bk}be the link capacity and buffer allocation to class k on a link, where k [1, K]. Let p = {pc, pb} be the price per unit link capacity and unit buffer at a link, and wk be the wealth (budget) of traffic class k. The utility function for TCk is Uk = f(ck, bk, Trk). The traffic of a class is repre- sented by a vector of traffic parameters (Trk) and a vector of QoS requirements (such as packet loss probabilities, average packet delay and so on.). Agent (TC: traffic class) buys resources from the net- work at the given prices using its wealth. The wealth constraint of agent TCk is: pb bk + pc ck wk. A budget set is the set of allocations that are feasible under the wealth constraint (budget constraint). The budget set is defined as follows: :, k BxxXxw pp (1) Computation of demands sets: The demand set for each agent is given by the following: :,,xx BUxxB pp p (2) The goal of TCk is to compute the allocations that pro- vide maximal preference under wk and p. Each TCk per- Copyright © 2012 SciRes. ME
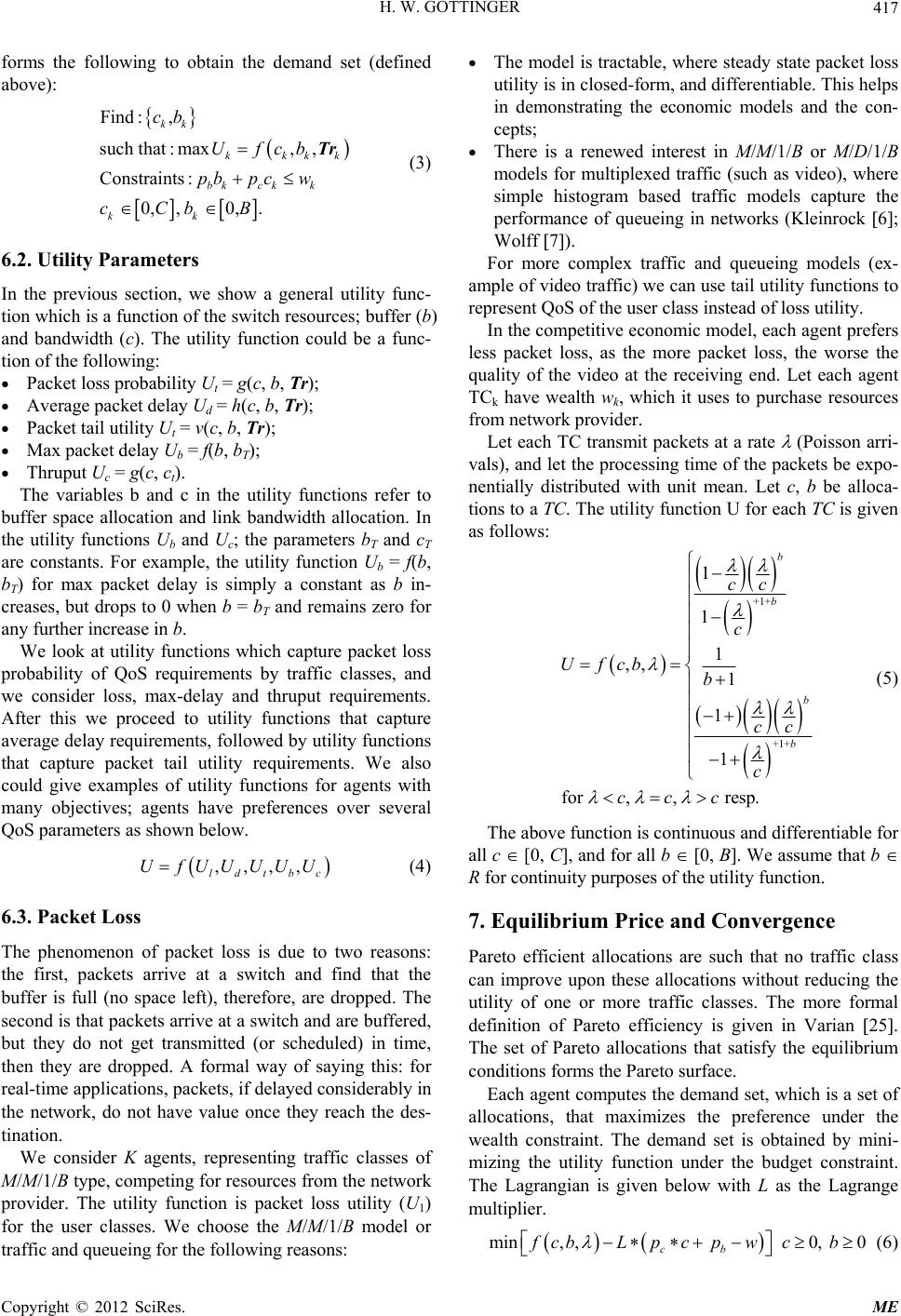 H. W. GOTTINGER 417 forms the following to obtain the demand set (defined above): Find :, such that:max Constraints : 0, ,0, kk bk kk cb Uf pb cCb , , . kk kk ck k cb pcw B Tr ,,,, tbc UUU (3) 6.2. Utility Parameters In the previous section, we show a general utility func- tion which is a function of the switch resources; buffer (b) and bandwidth (c). The utility function could be a func- tion of the following: Packet loss probability Ut = g(c, b, Tr); Average packet delay Ud = h(c, b, Tr); Packet tail utility Ut = v(c, b, Tr); Max packet delay Ub = f(b, bT); Thruput Uc = g(c, ct). The variables b and c in the utility functions refer to buffer space allocation and link bandwidth allocation. In the utility functions Ub and Uc; the parameters bT and cT are constants. For example, the utility function Ub = f(b, bT) for max packet delay is simply a constant as b in- creases, but drops to 0 when b = bT and remains zero for any further increase in b. We look at utility functions which capture packet loss probability of QoS requirements by traffic classes, and we consider loss, max-delay and thruput requirements. After this we proceed to utility functions that capture average delay requirements, followed by utility functions that capture packet tail utility requirements. We also could give examples of utility functions for agents with many objectives; agents have preferences over several QoS parameters as shown below. ld U fUU (4) 6.3. Packet Loss The phenomenon of packet loss is due to two reasons: the first, packets arrive at a switch and find that the buffer is full (no space left), therefore, are dropped. The second is that packets arrive at a switch and are buffered, but they do not get transmitted (or scheduled) in time, then they are dropped. A formal way of saying this: for real-time applications, packets, if delayed considerably in the network, do not have value once they reach the des- tination. We consider K agents, representing traffic classes of M/M/1/B type, competing for resources from the network provider. The utility function is packet loss utility (U1) for the user classes. We choose the M/M/1/B model or traffic and queueing for the following reasons: The model is tractable, where steady state packet loss utility is in closed-form, and differentiable. This helps in demonstrating the economic models and the con- cepts; There is a renewed interest in M/M/1/B or M/D/1/B models for multiplexed traffic (such as video), where simple histogram based traffic models capture the performance of queueing in networks (Kleinrock ; Wolff 7). For more complex traffic and queueing models (ex- ample of video traffic) we can use tail utility functions to represent QoS of the user class instead of loss utility. In the competitive economic model, each agent prefers less packet loss, as the more packet loss, the worse the quality of the video at the receiving end. Let each agent TCk have wealth wk, which it uses to purchase resources from network provider. Let each TC transmit packets at a rate (Poisson arri- vals), and let the processing time of the packets be expo- nentially distributed with unit mean. Let c, b be alloca- tions to a TC. The utility function U for each TC is given as follows: +1+ +1+ 1 1 1 ,, 1 1 1 for,, resp. b b b b cc c Ufcb b cc c ccc (5) The above function is continuous and differentiable for all c [0, C], and for all b [0, B]. We assume that b R for continuity purposes of the utility function. 7. Equilibrium Price and Convergence Pareto efficient allocations are such that no traffic class can improve upon these allocations without reducing the utility of one or more traffic classes. The more formal definition of Pareto efficiency is given in Varian 25. The set of Pareto allocations that satisfy the equilibrium conditions forms the Pareto surface. Each agent computes the demand set, which is a set of allocations, that maximizes the preference under the wealth constraint. The demand set is obtained by mini- mizing the utility function under the budget constraint. The Lagrangian is given below with L as the Lagrange multiplier. min,, 0, 0 cb fcbLp cpwcb (6) Copyright © 2012 SciRes. ME
 H. W. GOTTINGER 418 The function f(c, b, ) is smooth, strictly convex and compact, thus the demand set is just one element [1,2]. Using the Kuhn-Tucker optimality conditions, the opti- mal resource allocation vector is obtained, where L is the Lagrange multiplier , cb UU Lp cb ,pp Lp (7) From this the equilibrium condition is obtained. This condition states that the marginal rate of substitution is equal among the competing traffic classes. The economic problems are to establish competitive equilibrium, com- pute the equilibrium prices cb and Pareto optimal allocations. The equilibrium condition is shown as fol- lows: * * c b p Uc Ub p (8) Using the utility function given by Equation (5), the price ratio is given below. From the equation, it is evi- dent that the price ratio is a function of the resource allo- cations and the traffic parameter . ,, c b pgcb p log bbcb c cc c (9) This equation can be rewritten in the following way, where function N has a nice interpretation. It is the ratio of the effective queue utilization ( (1 − U)) to the effec- tive queue emptiness (1 – (1 − U)), where = /c. 1 1 U U where log 1 c b pN bN pc (10) This can also be interpreted as the effective number in an equivalent M/M/1 queueing system, where the system utilization is = (1 − U). For an M/M/1 system, the average number in the system is . 1 The following gives the equilibrium condition for K agents competing for resources from a single network provider. From this condition, and the resource con- straints, the Pareto allocations and the corresponding equilibrium price ratios can be computed. 112 2 112 2 log log c b p bN bN pc clog kK KK bN c (11) Using the buffer constraint b1 + b2 + b3+ ··· + bk = B, the equilibrium price ratio and optimal buffer allocation for each agent i can be represented by the following equations: log ii ii NB c c bi p p (12) log log iii i ii ii i NBc bN c 0 pp (13) The issue of determining the equilibrium prices, so that supply equal demand for different types of utility functions can use convex optimization tools (Chen, Ye and Zhang, 2007) Competitive Pricing Algorithm 1) Set initial prices: cc , . 0 pp i bb 2) Compute demand set, i.e. find minimum of min [Ui = fi(ci,bi, i)] 1, iK cii Dc [1,K] given pcci + pbbi wi (wealth constraint). 1 iK bii Db . 3) Demand: 4) If (Dc − C) < (>) 0, then, pc = pc – (+)Δc. If (Db − B) < (>) 0, then, pb = pb – (+)Δb. Go back to step (2). 5) Else if Dc = C at pc, and Db = B at pb, then the equi- librium is attained and prices are at equilibrium The algorithm computes iteratively the equilibrium prices in a competitive economy using the utility func- tions. Given the wealth in the economy, the prices con- verge to a point on the Pareto surface, which can be computed using the first-order conditions. There is a minimum price p , that each traffic class has to pay, if the equilibrium prices are lower than pi. Once the prices are computed, the network service provider releases the re- sources to the agents. 8. Example of Two Agents and One Supplier We consider two agents, representing traffic classes of the M/M/1/B model. The utility function is shown in Equation (5). The agents have wealth w1 and w2 respec- tively. The agents compete for resources, which then are used to provide services to users. Two Classes: We consider two competing traffic classes. Using the equilibrium conditions, the equilib- rium price ratio is given by, 1122 112 2 log log c b PNb Nb cc P (14) The above equation states that at equilibrium, the log of the ratio of utilizations of the two traffic classes is equal to the ratio of the time to evacuate the residual buffer space of the traffic classes. Rewriting the above equation: 1112 2122 log log Nb c cNb (15) By using the resource constraints c1 + c2 = C and b1 + b2 = B, the equilibrium conditions become a function of just two variables. The Pareto surface is the set of alloca- Copyright © 2012 SciRes. ME
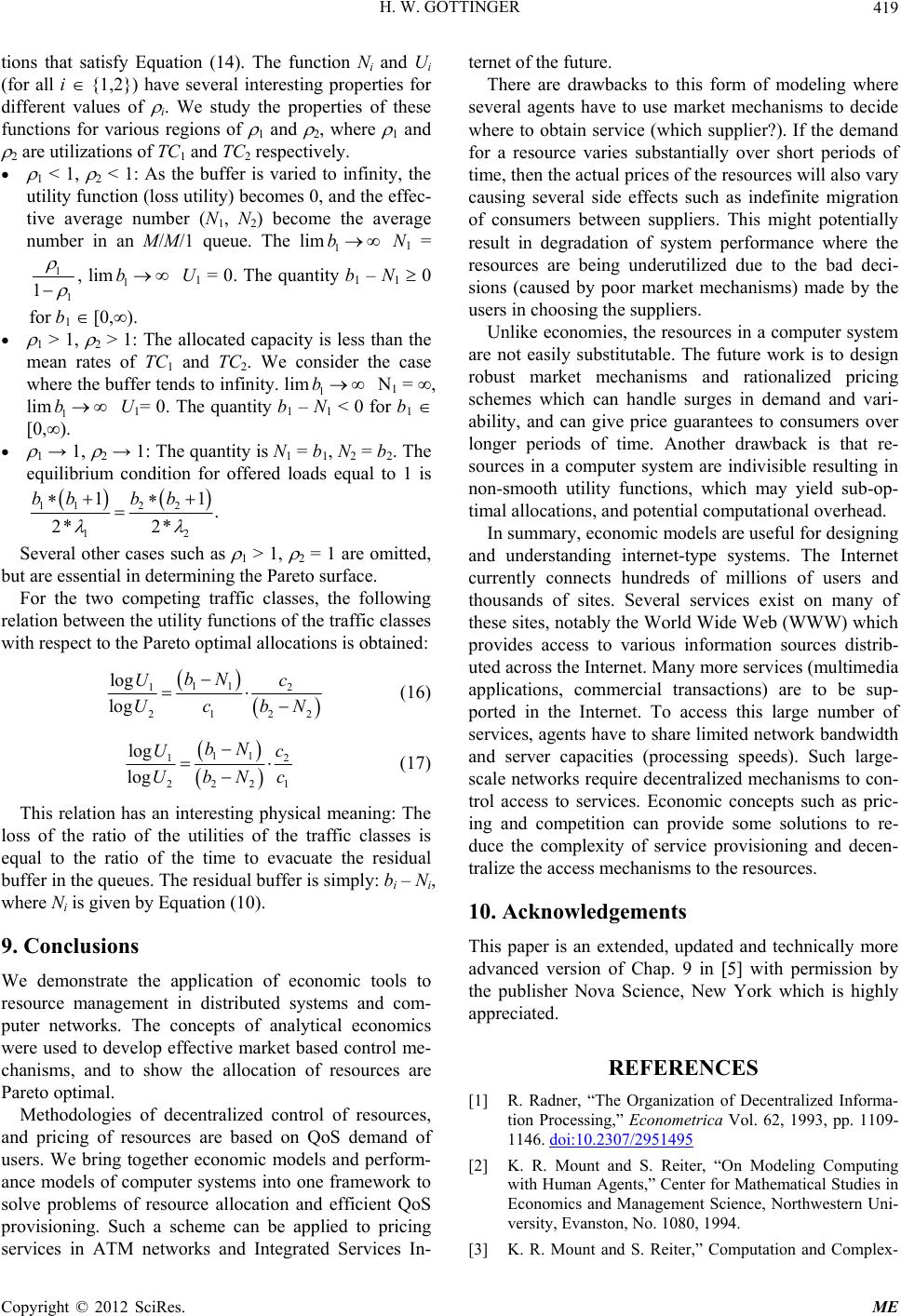 H. W. GOTTINGER 419 tions that satisfy Equation (14). The function Ni and Ui (for all i {1,2}) have several interesting properties for different values of i. We study the properties of these functions for various regions of 1 and 2, where 1 and 2 are utilizations of TC1 and TC2 respectively. 1 < 1, 2 < 1: As the buffer is varied to infinity, the utility function (loss utility) becomes 0, and the effec- tive average number (N1, N2) become the average number in an M/M/1 queue. The lim1 b N1 = 1 1 1 , lim U1 = 0. The quantity b1 – N1 0 1 b for b1 [0,∞). 1 > 1, 2 > 1: The allocated capacity is less than the mean rates of TC1 and TC2. We consider the case where the buffer tends to infinity. lim1 b N 1 = ∞, lim 1 b U1= 0. The quantity b1 – N1 < 0 for b1 [0,∞). 1 → 1, 2 → 1: The quantity is N1 = b1, N2 = b2. The equilibrium condition for offered loads equal to 1 is 11 2* bb 2 2 12 11 . 2* bb Several other cases such as 1 > 1, 2 = 1 are omitted, but are essential in determining the Pareto surface. For the two competing traffic classes, the following relation between the utility functions of the traffic classes with respect to the Pareto optimal allocations is obtained: 11 2 22 c bN 1 21 log log bN U Uc (16) 11 12 2221 bN Uc Ub Nc log log (17) This relation has an interesting physical meaning: The loss of the ratio of the utilities of the traffic classes is equal to the ratio of the time to evacuate the residual buffer in the queues. The residual buffer is simply: bi – Ni, where Ni is given by Equation (10). 9. Conclusions We demonstrate the application of economic tools to resource management in distributed systems and com- puter networks. The concepts of analytical economics were used to develop effective market based control me- chanisms, and to show the allocation of resources are Pareto optimal. Methodologies of decentralized control of resources, and pricing of resources are based on QoS demand of users. We bring together economic models and perform- ance models of computer systems into one framework to solve problems of resource allocation and efficient QoS provisioning. Such a scheme can be applied to pricing services in ATM networks and Integrated Services In- ternet of the future. There are drawbacks to this form of modeling where several agents have to use market mechanisms to decide where to obtain service (which supplier?). If the demand for a resource varies substantially over short periods of time, then the actual prices of the resources will also vary causing several side effects such as indefinite migration of consumers between suppliers. This might potentially result in degradation of system performance where the resources are being underutilized due to the bad deci- sions (caused by poor market mechanisms) made by the users in choosing the suppliers. Unlike economies, the resources in a computer system are not easily substitutable. The future work is to design robust market mechanisms and rationalized pricing schemes which can handle surges in demand and vari- ability, and can give price guarantees to consumers over longer periods of time. Another drawback is that re- sources in a computer system are indivisible resulting in non-smooth utility functions, which may yield sub-op- timal allocations, and potential computational overhead. In summary, economic models are useful for designing and understanding internet-type systems. The Internet currently connects hundreds of millions of users and thousands of sites. Several services exist on many of these sites, notably the World Wide Web (WWW) which provides access to various information sources distrib- uted across the Internet. Many more services (multimedia applications, commercial transactions) are to be sup- ported in the Internet. To access this large number of services, agents have to share limited network bandwidth and server capacities (processing speeds). Such large- scale networks require decentralized mechanisms to con- trol access to services. Economic concepts such as pric- ing and competition can provide some solutions to re- duce the complexity of service provisioning and decen- tralize the access mechanisms to the resources. 10. Acknowledgements This paper is an extended, updated and technically more advanced version of Chap. 9 in with permission by the publisher Nova Science, New York which is highly appreciated. REFERENCES [1] R. Radner, “The Organization of Decentralized Informa- tion Processing,” Econometrica Vol. 62, 1993, pp. 1109- 1146. doi:10.2307/2951495 [2] K. R. Mount and S. Reiter, “On Modeling Computing with Human Agents,” Center for Mathematical Studies in Economics and Management Science, Northwestern Uni- versity, Evanston, No. 1080, 1994. [3] K. R. Mount and S. Reiter,” Computation and Complex- Copyright © 2012 SciRes. ME
 H. W. GOTTINGER Copyright © 2012 SciRes. ME 420 ity in Economic Behavior and Organization,” Cambridge University Press, Cambridge, 2002. doi:10.1017/CBO9780511754241 [4] T. Van Zandt, “The Scheduling and Organization of Peri- odic Associative Computation: Efficient Networks,” Re- view of Economic Design, Vol. 3, 1998, pp. 93-127. doi:10.1007/s100580050007 [5] H. W. Gottinger, “Strategic Economics in Network In- dustries,” Nova Science Publishers, New York, 2009. [6] L. Kleinrock, “Queueing Systems, Vol. 2, Applications,” Wiley Interscience, New York, 1975. [7] R. W. Wolff, “Stochastic Modeling and the Theory of Queues,” Prentice Hall, Englewood Cliffs, 1989. [8] L. Hurwicz and S. Reiter, “Designing Economic Mecha- nisms,” Cambridge University Press, Cambridge, 2006. doi:10.1017/CBO9780511754258 [9] Y. Narahari, D. Garg, R. Narayanam and H. Prakash, “Game Theoretic Problems in Network Economics and Mechanism Design Solutions,” Springer, London, 2009. [10] D. Neumann, M. Baker, J. Altmann and O. F. Rana, Eds., “Economic Models and Algorithms for Distributed Sys- tems,” Birkhaeuser, Basel, 2010. doi:10.1007/978-3-7643-8899-7 [11] C. Meinel and S. Tison, Eds., “STACS 99: 16th Annual Symposion Theoretical Aspects of Computer Science,” Springer, Berlin, 1999. [12] X. Deng and F. C. Graham, “Internet and Network Eco- nomics,” Springer, Berlin, 2007. doi:10.1007/978-3-540-77105-0 [13] H. Ackermann, P. Briest, A. Fanghänel and B. Vöcking, “Who Should Pay for Forwarding Packets,” In: X. Deng and F. C. Graham, Eds., Internet and Network Economics, Springer, Berlin, 2007, pp. 208-219. doi:10.1007/978-3-540-77105-0_21 [14] L. Chen, Y. Ye and J. Zhang, “A Note on Equilibrium Pricing as Convex Optimization,” In X. Deng and F. C. Graham, Eds., Internet and Network Economic, Springer, New York, 2007, pp. 7-16. doi:10.1007/978-3-540-77105-0_5 [15] S. Iong, A. Man-Cho-So and M. Sundararajan, “Stochas- tic Mechanism Design,” In: X. Deng and F. C. Graham, Eds., Internet and Network Economics, Springer, Berlin, 2007, pp. 269-280. doi:10.1007/978-3-540-77105-0_26 [16] J. K. MacKie-Mason and H. R. Varian, “Pricing the Inter- net,” In: B. Kahin and J. Keller, Eds., Public Access to the Internet, MIT Press, Cambridge, 1995, pp. 269-314. [17] S. Shenker, “Service Models and Pricing Policies for an Integrated Services Internet,” In: B. Kahin and J. Keller, Eds., Public Access to the Internet, MIT Press, Cam- bridge, 1995, pp. 315-337. [18] Q. Wang, J. M. Peha and M. A. Sirbu, “Optimal Pricing for Integrated Services Network,” In: L. M. McKnight and J. P. Bailey, Eds., Internet Economics, MIT Press, Cambridge, 1997, pp. 353-376. [19] R. Wilson, “Nonlinear Pricing,” Oxford University Press, Oxford, 1993. [20] N. Nisan and A. Ronen, “Computationally Feasible VCG Mechanisms,” Games and Economic Behavior, Vol. 35, No. 1-2, 2001, pp. 166-196. doi:10.1006/game.1999.0790 [21] V. Paxon, “Growth Trends in TCP/IP,” IEEE Communi- cations Magazine, April 1994. [22] J. Chao and D. Ghosal, “IP on ATM on Local Area Net- works,” IEEE Communications Magazine, Vol. 32, No. 8, 1994, pp. 52-59. doi:10.1109/35.299838 [23] H. W. Gottinger, “Telecommunication, Internet, Regula- tion and Pricing,” In: M. Takashima, H. W. Gottinger and C. Umali, Eds., The Economics of Global Telecommuni- cation and the Internet, Nagasaki University Report, Na- gasaki, 1997, pp. 107-126. [24] S. Low and P. Varaiya, “A New Approach to Service Pro- visioning in ATM Networks,” IEEE Transaction on Net- working, Vol. 1, 1993. [25] H. R. Varian, “Microeconomic Analysis,” Norton, New York, 1993.
 H. W. GOTTINGER 421 Appendix 1. The Network Economy The network consists of V nodes (packet switches) and N links. Each node has several output links with an output buffer. The resources at output link are transmission ca- pacity (or link capacity) and buffer space. The link con- troller at the output link schedules packets from the buffer. This is based on how the buffer is partitioned among the traffic classes and the scheduling rule be- tween the traffic classes. Sessions are grouped into traffic classes based on similar traffic characteristics and com- mon QoS requirements. Sessions that belong to a class share buffer and link resources, and traffic classes com- pete for resources at a packet switch. Each session ar- rives to the network with a vector of traffic parameters Tr, vector of QoS requirements and wealth. A session is grouped or mapped to a corresponding traffic class. A traffic class has common QoS requirements, and we con- sider QoS requirements per traffic class rather than per session. Once a session is admitted along a path (a route), it will continue along that path until it completes. Each agent k performs the following to obtain the de- mand set on each link. The allocations are buffer (b) and bandwidth (c) on each link for each agent. The wealth is distributed across the links by each agent to buy re- sources. That is, the problem is to find pairs kk such that max Uk = f(ck, bk, Trk), constraints pb ** , cb b 1* , , k + pcck wk. In the above formulation, each agent k buys resources from each link. The allocation for agent k is 2 * , ** kkk k and ccc 1*2* , ,, c** kk bbk k . An agent can invest wealth in either some or all the links. We assume that at each link there is competition among at least some of the agents for buying resources. As pre- viously, Gottinger [1999], we show a general utility function which is a function of the switch resources: buffer (b) and bandwidth (c). A utility function of the agent could be a function of: b b bcctt xUxU Packet loss probability Ut = g(c, b, Tr); Average packet delay Ud = h(c, b, Tr); Packet tail probability Ul = v(c, b, Tr); Max packet delay Ub = f(b); Thruput Uc = g (c). We consider that an agent will place demands for re- sources based on a general utility function, which is a combination of the various QoS requirements: 1 ,, lddb UfcbTr xUxU xU where Ul is the packet loss probability utility function, Ud is the average delay utility function, Ut is the packet tail probability, Ub is the utility function for max-delay requirements, and Uc is for bandwidth (throughput) re- quirements. x1, xd, xb, xc, xt are constants. Agents could use such a utility function. As long as the convexity property with respect to buffer b and bandwidth c holds. Pareto optimal allocations and price equilibria exist. However, if they are not convex, then depending on the properties of the functions, local optimality and price equilibrium could exist. To show the main ideas for routing and admission control, we use packet loss prob- ability as the main utility function (Ul), which means we assume that x1 from the above equation are the only con- stant and the rest are zeros. For doing this, we need first some further specifications of the loss probability. We later show results for Pareto optimality and price equilib- rium, and then we propose routing and admission control algorithms. In general, one can assume that agents, on behalf of user classes, demand for resources from the link suppliers based on the utility function shown above. The agent uses the utility function to present the demand for resources over the whole network of parallel links. Loss Probability Specifications. At each output link j the resources are buffer space Bj and link capacity Cj. , Let j cb kk be the link capacity and buffer alloca- tion to class k on link j where k [1,K]. Let c and p b be the price per unit link capacity and unit buffer respectively at link j, and wk be the wealth (budget) of a traffic class k. For a link j from the source to the destina- tion, the packet loss probability (utility) for traffic class k is given by the following p loss 1 11 j lkj k UP Pp where k p is the packet loss probability at link j of agent k. The goal of the agent is to minimize the packet loss probability under its wealth or budget constraints. If the traffic classes have smooth convex preferences with re- spect to link capacity and buffer allocation variables at each link, then the utility function Ulk is convex with respect to the variables. 2. The Server Economy We now discuss the server economy where servers offer processing resources and memory to agents representing user classes. The agents compete for these resources and buying as much as possible from suppliers. The agents perform load balancing based on the OoS preferences of the class it represents. The economic model consists of the following players: Agents and Server Suppliers, Consumers or user classes and Business. User sessions within a class have common preferences. User classes have QoS preferences over average delay and throughput, and in some cases com- pletion times of sessions (deadlines). Users within a class share resources at the servers. Copyright © 2012 SciRes. ME
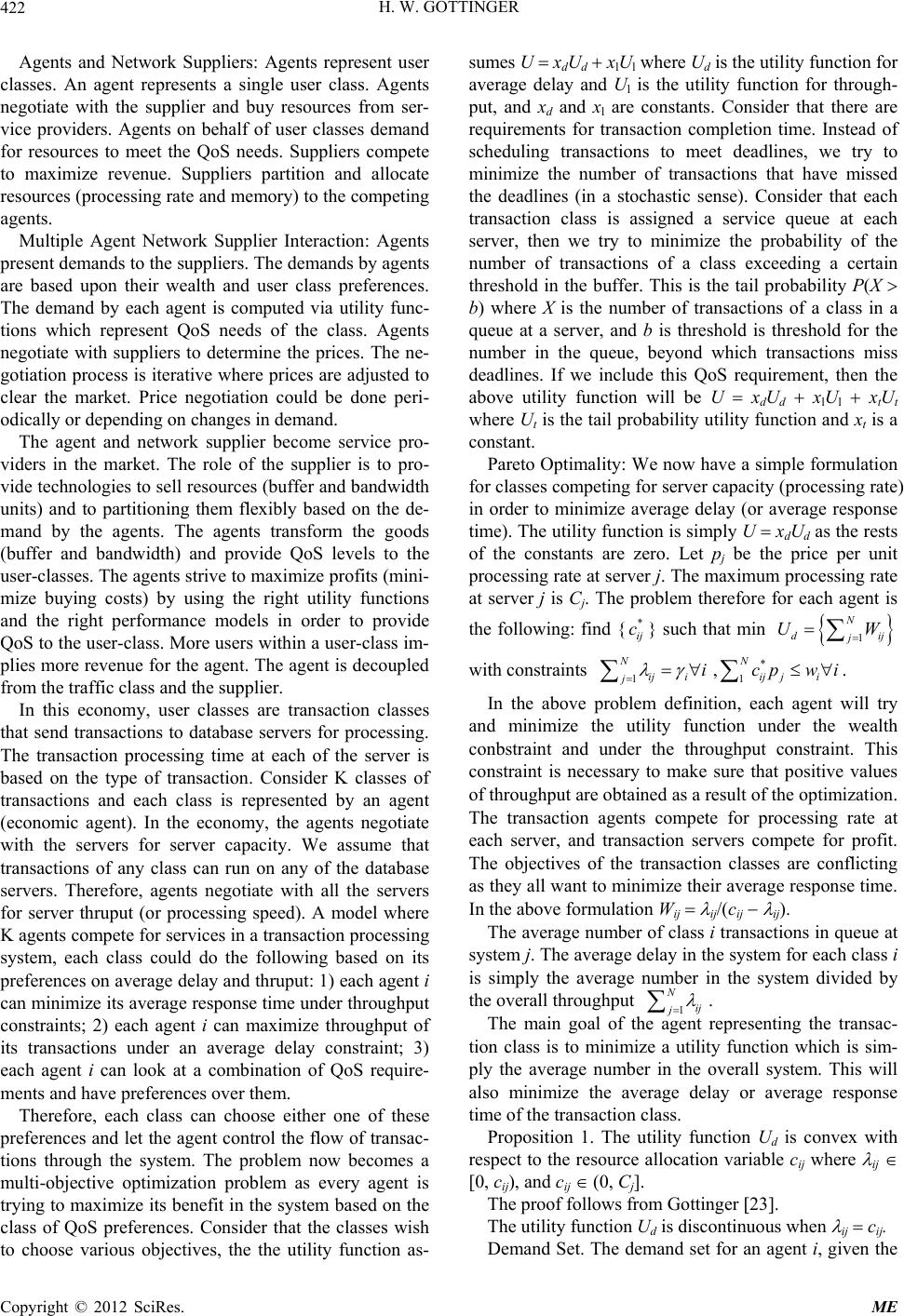 H. W. GOTTINGER 422 Agents and Network Suppliers: Agents represent user classes. An agent represents a single user class. Agents negotiate with the supplier and buy resources from ser- vice providers. Agents on behalf of user classes demand for resources to meet the QoS needs. Suppliers compete to maximize revenue. Suppliers partition and allocate resources (processing rate and memory) to the competing agents. Multiple Agent Network Supplier Interaction: Agents present demands to the suppliers. The demands by agents are based upon their wealth and user class preferences. The demand by each agent is computed via utility func- tions which represent QoS needs of the class. Agents negotiate with suppliers to determine the prices. The ne- gotiation process is iterative where prices are adjusted to clear the market. Price negotiation could be done peri- odically or depending on changes in demand. The agent and network supplier become service pro- viders in the market. The role of the supplier is to pro- vide technologies to sell resources (buffer and bandwidth units) and to partitioning them flexibly based on the de- mand by the agents. The agents transform the goods (buffer and bandwidth) and provide QoS levels to the user-classes. The agents strive to maximize profits (mini- mize buying costs) by using the right utility functions and the right performance models in order to provide QoS to the user-class. More users within a user-class im- plies more revenue for the agent. The agent is decoupled from the traffic class and the supplier. In this economy, user classes are transaction classes that send transactions to database servers for processing. The transaction processing time at each of the server is based on the type of transaction. Consider K classes of transactions and each class is represented by an agent (economic agent). In the economy, the agents negotiate with the servers for server capacity. We assume that transactions of any class can run on any of the database servers. Therefore, agents negotiate with all the servers for server thruput (or processing speed). A model where K agents compete for services in a transaction processing system, each class could do the following based on its preferences on average delay and thruput: 1) each agent i can minimize its average response time under throughput constraints; 2) each agent i can maximize throughput of its transactions under an average delay constraint; 3) each agent i can look at a combination of QoS require- ments and have preferences over them. Therefore, each class can choose either one of these preferences and let the agent control the flow of transac- tions through the system. The problem now becomes a multi-objective optimization problem as every agent is trying to maximize its benefit in the system based on the class of QoS preferences. Consider that the classes wish to choose various objectives, the the utility function as- sumes U xdUd xlUl where Ud is the utility function for average delay and Ul is the utility function for through- put, and xd and xl are constants. Consider that there are requirements for transaction completion time. Instead of scheduling transactions to meet deadlines, we try to minimize the number of transactions that have missed the deadlines (in a stochastic sense). Consider that each transaction class is assigned a service queue at each server, then we try to minimize the probability of the number of transactions of a class exceeding a certain threshold in the buffer. This is the tail probability P(X b) where X is the number of transactions of a class in a queue at a server, and b is threshold is threshold for the number in the queue, beyond which transactions miss deadlines. If we include this QoS requirement, then the above utility function will be U xdUd xlUl xtUt where Ut is the tail probability utility function and xt is a constant. Pareto Optimality: We now have a simple formulation for classes competing for server capacity (processing rate) in order to minimize average delay (or average response time). The utility function is simply U xdUd as the rests of the constants are zero. Let pj be the price per unit processing rate at server j. The maximum processing rate at server j is Cj. The problem therefore for each agent is the following: find {} such that min * ij c 1 N dij j UW * 11 , NN ijiij ji jicpwi with constraints N . In the above problem definition, each agent will try and minimize the utility function under the wealth conbstraint and under the throughput constraint. This constraint is necessary to make sure that positive values of throughput are obtained as a result of the optimization. The transaction agents compete for processing rate at each server, and transaction servers compete for profit. The objectives of the transaction classes are conflicting as they all want to minimize their average response time. In the above formulation Wij ij/(cij ij). The average number of class i transactions in queue at system j. The average delay in the system for each class i is simply the average number in the system divided by the overall throughput 1ij j The main goal of the agent representing the transac- tion class is to minimize a utility function which is sim- ply the average number in the overall system. This will also minimize the average delay or average response time of the transaction class. . Proposition 1. The utility function Ud is convex with respect to the resource allocation variable cij where ij [0, cij), and cij (0, Cj]. The proof follows from Gottinger 23. The utility function Ud is discontinuous when ij cij. Demand Set. The demand set for an agent i, given the Copyright © 2012 SciRes. ME
 H. W. GOTTINGER Copyright © 2012 SciRes. ME 423 prices (pj of server j) of the processing rates (or capaci- ties) at the servers is {ci1, ci2, ···, ciN} over all the servers. We use the standard techniques of optimization to find the demand set, which is given as follows for all j [1, N] Proposition 2. Consider K agents competing for proc- essing resources from N servers. If the utility function of these agents is Ud and the performance model at the servers is an M/M/1 model, then price equilibrium and Pareto optimality exist. 11 NN ijijiij jij jj cwp jij j pp KcC . The proof of this proposition is the same as described in Gottinger 23. The utility function Ud is continuous and decreasing convex with respect to the allocation variables cij. The function is discontinuous when ij cij. Price Equilibrium: Once the demand set is obtained, then using the wealth constraints, we can solve for the equilibrium price. This is not easily tractable. However, numerical results can be computed using the tatonnement process whereby agents compute the demand set, given the processing rate prices by each server. Due to this, Pareto allocations or price equilibrium may not exist. However, we solve this problem by stating that the agents, when they present their demands, have to make sure that the transaction throughput rate ij at a server has to be lower than the capacity allocation cij. If this is not met, then the price iteration process or the ta- tonnement process will not converge. We assume that the servers know the transaction thruput or arrival rate from each agent during the iteration process. An iteration process between the agents and the servers takes place. This will converge to an equilibrium price, when demand equals the supply which is 1ij j i . We now state formally the result for K agents com- peting for processing resources from N servers.
|