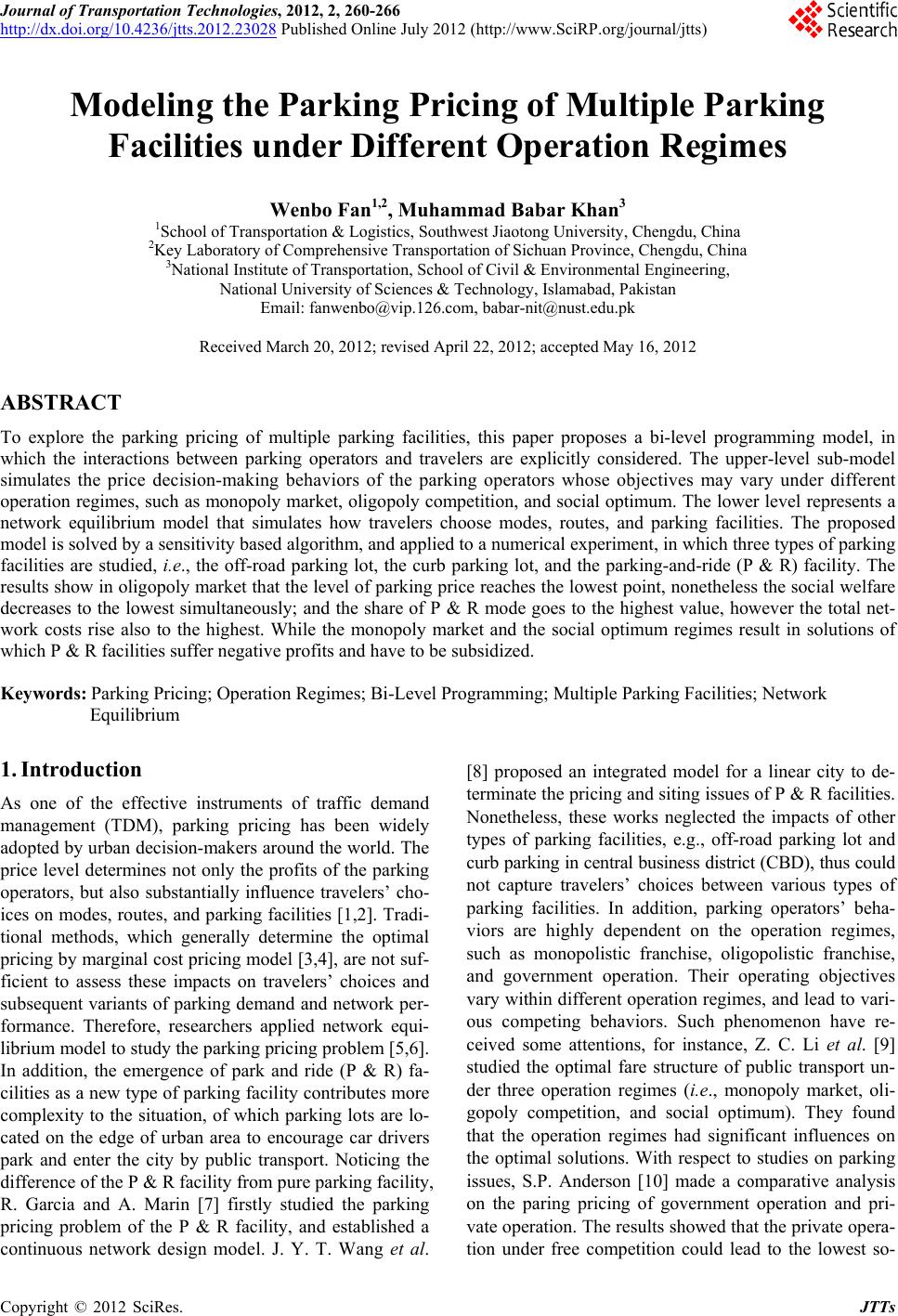 Journal of Transportation Technologies, 2012, 2, 260-266 http://dx.doi.org/10.4236/jtts.2012.23028 Published Online July 2012 (http://www.SciRP.org/journal/jtts) Modeling the Parking Pricing of Multiple Parking Facilities under Different Operation Regimes Wenbo Fan1,2, Muhammad Babar Khan3 1School of Transportation & Logistics, Southwest Jiaotong University, Chengdu, China 2Key Laboratory of Comprehensive Transportation of Sichuan Province, Chengdu, China 3National Institute of Transportation, School of Civil & Environmental Engineering, National University of Sciences & Technology, Islamabad, Pakistan Email: fanwenbo@vip.126.com, babar-nit@nust.edu.pk Received March 20, 2012; revised April 22, 2012; accepted May 16, 2012 ABSTRACT To explore the parking pricing of multiple parking facilities, this paper proposes a bi-level programming model, in which the interactions between parking operators and travelers are explicitly considered. The upper-level sub-model simulates the price decision-making behaviors of the parking operators whose objectives may vary under different operation regimes, such as monopoly market, oligopoly competition, and social optimum. The lower level represents a network equilibrium model that simulates how travelers choose modes, routes, and parking facilities. The proposed model is solved by a sensitivity based algorithm, and applied to a numerical experiment, in which three types of parking facilities are studied, i.e., the off-road parking lot, the curb parking lot, and the parking-and-ride (P & R) facility. The results show in oligopoly market that the level of parking price reaches the lowest point, nonetheless the social welfare decreases to the lowest simultaneously; and the share of P & R mode goes to the highest value, however the total net- work costs rise also to the highest. While the monopoly market and the social optimum regimes result in solutions of which P & R facilities suffer negative profits and have to be subsidized. Keywords: Parking Pricing; Operation Regimes; Bi-Level Programming; Multiple Parking Facilities; Network Equilibrium 1. Introduction As one of the effective instruments of traffic demand management (TDM), parking pricing has been widely adopted by urban decision-makers around the world. The price level determines not only the profits of the parking operators, but also substantially influence travelers’ cho- ices on modes, routes, and parking facilities [1,2]. Tradi- tional methods, which generally determine the optimal pricing by marginal cost pricing model [3,4], are not suf- ficient to assess these impacts on travelers’ choices and subsequent variants of parking demand and network per- formance. Therefore, researchers applied network equi- librium model to study the parking pricing problem [5,6]. In addition, the emergence of park and ride (P & R) fa- cilities as a new type of parking facility contributes more complexity to the situation, of which parking lots are lo- cated on the edge of urban area to encourage car drivers park and enter the city by public transport. Noticing the difference of the P & R facility from pure parking facility, R. Garcia and A. Marin [7] firstly studied the parking pricing problem of the P & R facility, and established a continuous network design model. J. Y. T. Wang et al. [8] proposed an integrated model for a linear city to de- terminate the pricing and siting issues of P & R facilities. Nonetheless, these works neglected the impacts of other types of parking facilities, e.g., off-road parking lot and curb parking in central business district (CBD), thus could not capture travelers’ choices between various types of parking facilities. In addition, parking operators’ beha- viors are highly dependent on the operation regimes, such as monopolistic franchise, oligopolistic franchise, and government operation. Their operating objectives vary within different operation regimes, and lead to vari- ous competing behaviors. Such phenomenon have re- ceived some attentions, for instance, Z. C. Li et al. [9] studied the optimal fare structure of public transport un- der three operation regimes (i.e., monopoly market, oli- gopoly competition, and social optimum). They found that the operation regimes had significant influences on the optimal solutions. With respect to studies on parking issues, S.P. Anderson [10] made a comparative analysis on the paring pricing of government operation and pri- vate operation. The results showed that the private opera- tion under free competition could lead to the lowest so- C opyright © 2012 SciRes. JTTs
 W. B. FAN, M. B. KHAN 261 cial costs in total. J. F. Tsai and C. P. Chu [11] consi- dered in parking pricing decision three types of players, i.e., the government, the operation corporation, and the customers, and established a three-stage Stachelberg game model to describe the interactions between these players. These existing valuable studies enriched our understand- ing about the parking pricing under different operation regimes, but had not yet explored the impacts of multiple parking facilities. The objective of this paper is to model the parking pricing of multiple parking facilities under three opera- tion regimes (i.e., the monopoly market, the oligopoly competition, and the social optimum). The following sec- tion presents several assumptions to facilitate the model- ing. Section 3 formulates the pricing model with opera- tors’ objective functions under the three operation re- gimes. Section 4 discusses multimodal equilibrium mo- del to represent the travelers’ minimum travel disutility. A comprehensive solution algorithm is illustrated step- wise in Section 5, followed by numerical experiments. Finally, conclusive remarks are made along with recom- mendations for future researches. 2. Assumptions and Variable Descriptions Given a multimodal network G = (N, L), where N is the set of all notes; L is the set of all links. G includes two sub-networks, the auto network and the metro network . Without loss of genera- lity, the following basic assumptions are made in this paper. , aaa GNL , bbb GNL A1. There are two types of players in the network: parking operators and auto travelers. The operators make their pricing decisions based on the parking demand, which in turn varies as a result of travelers’ adjustment to their journey costs. A2. Consider three operation regimes: monopoly mar- ket, oligopoly competition, and social optimum; and three types of parking facilities: off-road parking lot, curb par- king lot, and P & R facility. A3. There are two modes for travelers to accomplish their journey, the automobile and the P & R. For conve- nience of the following formulation, let “a” and “b” de- note the two modes, and use the multinomial logit model to describe travelers’ mode choice behaviors, which could fit the diversity in people’s preferences. A4. Suppose travelers are very familiar with the net- work, and make their travel decisions in a deterministic manner to minimize the travel disutility. Therefore, by competition the user equilibrium (UE) would reach. A5. Introduce an elastic demand function to depict travelers’ responses to various level of parking charges, such as switching the departure time, or even not making the journey. Tables 1 and 2 present the parameters and variables that are used in the following model formulations. 3. Parking Pricing Model 3.1. Profit Function The net profit k of operator k can be formulated as the total revenue minus the operation costs, , kk kjjjj jJ jJ zvHCE C zvzjj (1) where, the bold symbols represent the vectors of the cor- responding variables. It is assumed that and E are linear functions of the parking capacity C, in forms of j j C C and 01 j j C EC . 3.2. Objective Functions 3.2.1. Mon op o l y Market In the monopoly market, there should be a single autho- rized agent who is responsible for operating all parking facilities. The objective of the agent would be to maxi- mize its total net profit by guiding all operators in the market. Given an elastic demand, the maximization is to find the optimal parking fees for each parking facilities, and can be expressed as follows. (U1) max , k k zzvz (2) where the parking demand can be obtained from the lower-level equilibrium model (given in Section 3), and operator k’s net profit k can be calculated by Equa- tion (1). 3.2.2. Oligop o l y Comp eti tion The oligopoly competition in this paper refers to the situ- ation, in which operators act independently and compete for their own profits. When the competitive equilibrium reaches, no operator could earn more profits by his own adjustment of the parking pricing. Thus, the oligopolistic equilibrium can be formulated as a Cournot-Nash game problem, (U2) max ,,,, k kk kk kkK zzzvzz (3) where, represents operator k’s pricing strategy for its parking facilities, and k zk z is the pricing strategy of other operators. 3.2.3. Social Optimum The social optimum represents the common situation where all parking facilities are operated by the govern- ment, who provides the parking as a public service. Therefore, the operational objective is to maximize the total social welfare (SW), which is defined here as a sum of the consumer surplus and the producer surplus. The Copyright © 2012 SciRes. JTTs
 W. B. FAN, M. B. KHAN 262 Table 1. Subscripts and parameters used in mathematical formulations. Symbol Description K = set of parking operators k = parking operator index k = set of parking facilities (i.e., parking lots and P & R facilities) of operator k , 1 = construction costs and operation costs of unit parking space, respectively 0 = fixed operation costs of parking facility j r, s = origin and destination (OD) of a trip p = index of a path between OD pair (r, s) m = set of travel modes (i.e., auto mode a and P & R mode b) 14 = parameters converting travel costs by auto mode into the same unit (e.g., monetary unit) 13 = parameters converting travel costs of P & R mode 1 4 = parameters converting the transferring costs between auto to metro into the same unit = a statistical calibrating parameter dependent on the interval of trains’ arrivals at station and also the distribution of passengers’ arrival times m = parameter reflecting traveler’s preference to mode m = traveler’s perception variation on travel disutility = parameter reflecting the elasticity of the demand to the travel disutility n = iteration counter in the solving algorithm la = index of a link on roadway network consumer surplus equals consumers’ total utility minus their all disutility; and the producer surplus is the net profits of all operators. The objective function under the regime of social optimum can be given as, 1 0 ,, (U3) max SWd , rs Q rsrs rs rs rs k kK Duu Q zvz (4) where, the first part on the right-hand of Equation (4) is the consumer surplus; and the second part is the total net profits of all operators. 4. Multimodal Equilibrium Model 4.1. Equilibrium Conditions According to the A4, travelers’ behaviors satisfy the UE condition. That is to say, in the equilibrium state, the travel alternatives chosen by travelers have the minimum travel disutility. Such situation can be expressed as fol- lows. , , , , if, 0 , if, 0 mm rsrs jp m rs jpmm rsrs jp f Uf (5) Table 2. Variables used in mathematical formulations. Symbol Description k = net profit of operator k , jj zv = parking fee and demand of parking facility j j , j E= construction costs and the operation costs of parking facility j, respectively j C = parking capacity of parking facility j rs Q = demand accommodated between (r, s) rs = travel disutility between (r, s) 1 rs = inverse function of the elastic demand Q rs rs Q = potential demand between (r, s) m rs q = travel demand by mode m between (r, s) , m rs jp U,, m rs jp = travel disutility and flow of mode m (i.e ., a and b) on route p via parking facility j, between OD pair (r, s) ,rj p T = actual travel time by auto from origin r via path p to parking facility j nearby the destination s j = parking search time within parking facility j = average occupancy converting the parking fee into per person js w = walking time from parking lot j to destination s 1 , rj p T = auto travel time from the origin r to the P & R facility j via path p jj = costs of transferring from automobile to metro 2 , js p T = in-vehicle time by metro b = additional penalty for the transferring jj w = walking time from P & R facility j to the adjacent metro stationj ; T jw = waiting time at station j b = dispatch frequency of the metro trains = a pre-specified precision a l t, a l v= travel time and traffic flow on auto link a l 0 a l t, a l C= free-flow travel time and capacity on link la 0 j d = free-flow parking search time of parking facility j where, the travel disutility functions of mode a and b are given as the following Equations (6) and (7), respec- tively. ,1,2 34 a rs jprj pjjjs UT z w (6) When the traveler chosen mode b to travel from origin r and park at j, and continue the journey by taking metro at station j to destination s. Thus, his/her actual travel disutility can be given formulated as, 12 ,1,2 3, b rsjprjpjjj spb UT T (7) where, the transferring costs j can be given as, 123 4 w jj jjj zw j T (8) where, the waiting time at station w jT can be calcu- Copyright © 2012 SciRes. JTTs
 W. B. FAN, M. B. KHAN 263 lated by, w j T b F (9) It is to be noted that passengers’ arrival times is as- sumed to follow the normal distribution and the metro trains’ interval time is constant. Then, following refer- ence [1], it can be derived that 0.5 . The traveler’s choice model can be formulated ac- cording to the assumption A2 as, exp exp m rs mm mm rs rsrs m q Q (10) where, rs is supposed to be a continuous monotone decreasing function of the travel disutility between (r, s), and is given in the following form: Q exp rs rsrs QQ (11) where, the travel disutility rs , according to the nature of logit model, can be expressed by ln exp mm rs rs m . 4.2. Variational Inequality Model Following the work of W. H. K. Lam et al. [2] on net- work with multiple parking facilities, the aforementioned equilibrium conditions (Equations (5)-(11)) can be ex- pressed as a variational inequality problem (of which the proof can be done by using Karush-Kuhn-Tucker con- ditions but omitted for brevity of this paper) as follows. * ,, , , * * * 1* * (L) 1ln () 0 mm m rs jprs jprs jp rsmp j mmmm rs rs rs rs mrs rs rsrsrs rs Uff qqq Q DQ QQ q a b (12) Subject to: {,} m rs rs mab Q (13) , , a rs j a rsrs jp pP qf (14) , , b rs j b rsrs jp pP qf (15) , ,, 0 mm rsrsrs jp Qq f (16) where, * , m rs jp , , and rs represent the optimal solu- tions; the Equations (12)-(15) are demand conservation constraints for network and modes; Equation (16) is non- negativity constraints for the OD demand, parking de- mand, and route flows. *m rs q* Q 5. Solution Algorithm A sensitivity analysis based algorithm [12] is proposed in this section to solve equilibrium model. The fundamental logic is to convert the intractable non-linear problem into a quadratic programming problem by computing the de- cision variable’s gradient information. The detailed step- wise procedure is given as follows: Step 1. Initialization. Set an initial parking fee , and let the iteration counter 0 z 0n . Step 2. Lower-level assignment. Substitute the given into the lower-level problem, and calculate the equi- librium solution, and get the parking demand , the OD travel disutility n zn v n , and the accommodated demand . n QStep 3. Sensitivity Analysis. Compute the equilibrium solution’s gradient information with respect to the deci- sion variable z, and get vzz , z, and Qz. Step 4. Linearization. Linearize the parking demand , the OD travel disutility v , and the accommodated demand , and get the Equations (17)-(19). Q () n kjj jn n jj jJ jzz v vv zz z z vzj (17) , () n jj rs n n rsrsj j rs jzz Q QQ zz z z zz (18) , () n jj rs n n rsrsj j rs jzz zz z z zz (19) Step 5. Substitute the above Equations (17)-(19) in to the upper-level objective function, and get a quadratic programming problem of variable , which can be so- lved by Newton methods. Yield an auxiliary solution . z n yStep 6. Update the parking fee by 1nnnn n zzyz. Step 7. Check convergence. If 1 max nn jj zz stands, then stop and report the solution; otherwise, let 1nn , and go to step 2. The is a pre-specified precision. Remark. In step 2, the logit assignment can be under- taken by the Dial approach [13], and the deterministic network assignment can be preceded by the all-or-noth- ing approach [14] combined with Moore’s shortest route algorithm [15]. 6. Numerical Experiments 6.1. Data Inputs This section presents a numerical experiment that is car- ried out with an illustrated network as shown in Figure 1. Copyright © 2012 SciRes. JTTs
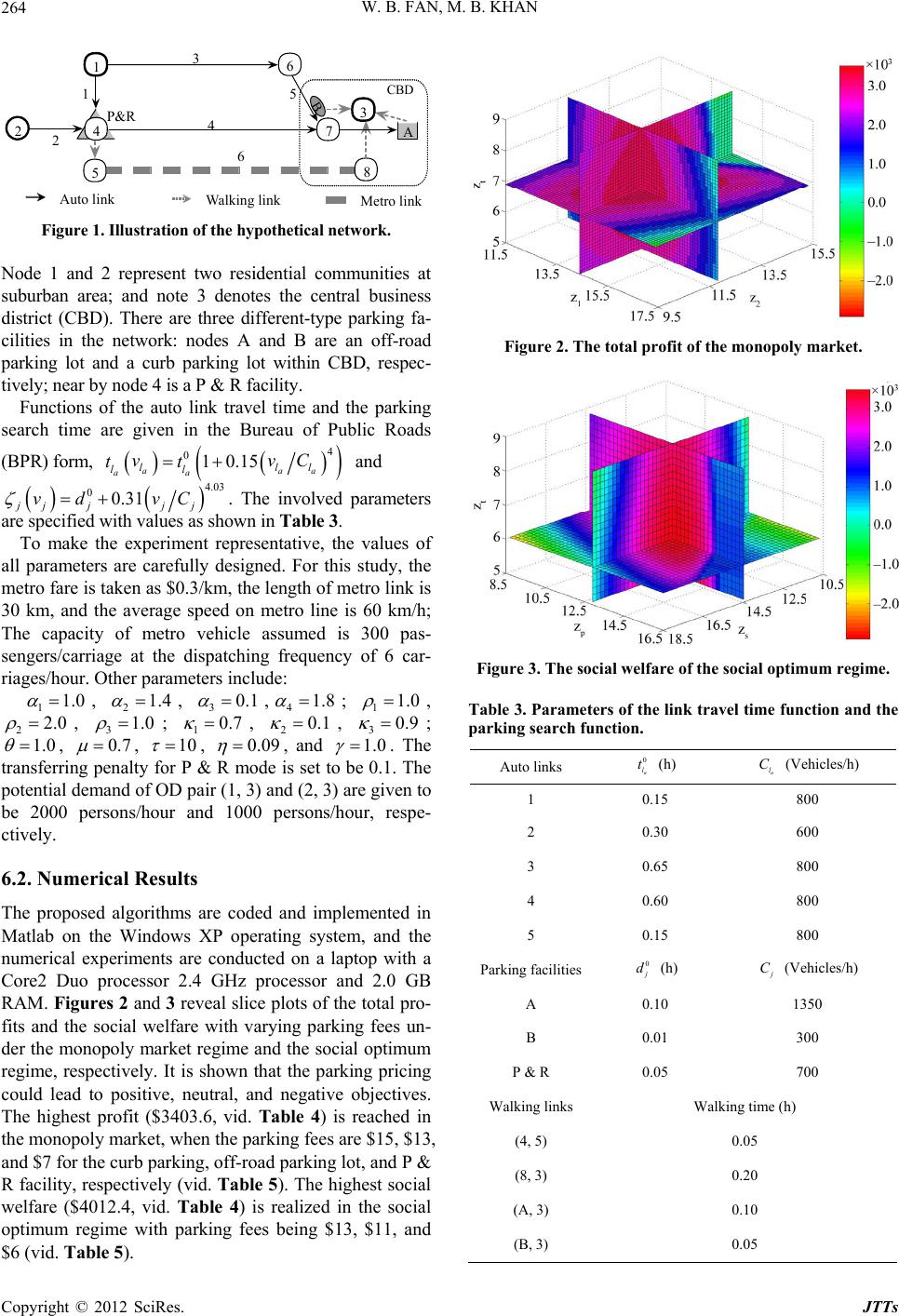 W. B. FAN, M. B. KHAN 264 CBD P&R 8 5 7 3 B 4 6 A 1 Auto linkWalking linkMe ro link 1 2 3 4 5 2 6 Figure 1. Illustration of the hypothetical network. Node 1 and 2 represent two residential communities at suburban area; and note 3 denotes the central business district (CBD). There are three different-type parking fa- cilities in the network: nodes A and B are an off-road parking lot and a curb parking lot within CBD, respec- tively; near by node 4 is a P & R facility. Functions of the auto link travel time and the parking search time are given in the Bureau of Public Roads (BPR) form, 4 010.15 aa aa ll ll vv tt a l C and 4.03 00.31 jjjj j vd vC . The involved parameters are specified with values as shown in Table 3. To make the experiment representative, the values of all parameters are carefully designed. For this study, the metro fare is taken as $0.3/km, the length of metro link is 30 km, and the average speed on metro line is 60 km/h; The capacity of metro vehicle assumed is 300 pas- sengers/carriage at the dispatching frequency of 6 car- riages/hour. Other parameters include: 11.0 , 21.4 , 30.1 ,41.8 ; 11.0 , 22.0 , 31.0 ; , 2, 10.7 0.1 30.9 ; 1.0 , 0.7 , 10 , 0.09 , and 1.0 . The transferring penalty for P & R mode is set to be 0.1. The potential demand of OD pair (1, 3) and (2, 3) are given to be 2000 persons/hour and 1000 persons/hour, respe- ctively. 6.2. Numerical Results The proposed algorithms are coded and implemented in Matlab on the Windows XP operating system, and the numerical experiments are conducted on a laptop with a Core2 Duo processor 2.4 GHz processor and 2.0 GB RAM. Figures 2 and 3 reveal slice plots of the total pro- fits and the social welfare with varying parking fees un- der the monopoly market regime and the social optimum regime, respectively. It is shown that the parking pricing could lead to positive, neutral, and negative objectives. The highest profit ($3403.6, vid. Table 4) is reached in the monopoly market, when the parking fees are $15, $13, and $7 for the curb parking, off-road parking lot, and P & R facility, respectively (vid. Table 5). The highest social welfare ($4012.4, vid. Table 4) is realized in the social optimum regime with parking fees being $13, $11, and $6 (vid. Table 5). Figure 2. The total profit of the monopoly market. Figure 3. The social welfare of the social optimum regime. Table 3. Parameters of the link travel time function and the parking search function. Auto links 0 a l t (h) a l C (Vehicles/h) 1 0.15 800 2 0.30 600 3 0.65 800 4 0.60 800 5 0.15 800 Parking facilities0 j d (h) j C (Vehicles/h) A 0.10 1350 B 0.01 300 P & R 0.05 700 Walking links Walking time (h) (4, 5) 0.05 (8, 3) 0.20 (A, 3) 0.10 (B, 3) 0.05 Copyright © 2012 SciRes. JTTs
 W. B. FAN, M. B. KHAN 265 Table 4. The networ k per f or mance under three re gime s ($). Regimes Total profits Social welfare Network costs Monopoly market 3403.6 3967.7 1252.8 Oligopoly competition 2095.9 3155.7 1734.7 Social optimum 3351.5 4012.4 1362.8 Table 5. The optimal pricing and corresponding profits of three parking facilities ($). Curb parking Off-road parking P & R facilities Regimes Parking fees Profits Parking fees Profits Parking fees Profits Monopoly market 15 2877 13 872.6 7 −345 Oligopoly competition 6 1189 5 854.6 5 52.5 Social optimum 13 2449 11 1245 6 −342 Table 5 shows the optimal pricing solution and the corresponding profits of three parking facilities. It can be found that the monopoly market yields the highest level of parking fee, while the oligopoly competition results in the lowest level. In addition, both the monopoly market and the social optimum maximize the overall objective at cost of the P & R operator’s loss, which means subsidies for P & R operation should be necessary. Table 4 presents the comparison of the three regimes in terms of total profits, social welfare, and network costs. In the view of parking operators, the monopoly market allow them obtain the highest profits; from the point of public administration, the social optimum regime results in the highest social welfare. Interestingly, the oligopoly competition yields positive profits for each operator, nonetheless the lowest profits and social welfare in total. Furthermore, the highest network costs emerge in the oligopoly competition in spite of more auto travelers are induced to choose the P & R mode (17.74%, vid. Table 6). To this result, the explanation can be found in Table 6, which shows that the most demand is accommodated on the network under the oligopoly competition regime. Consequently, although the average travel costs decrease to the least of all ($2.05/person), the total network costs rise ($1734.7). The aforementioned analyses show that operation re- gimes have significant influence on the optimal pricing solution, the operators’ profits, the demand split, and also the overall network performance. It is believed in this paper that the least level of pricing is due to interactive competitions between the three parking facilities. 7. Conclusions This paper explores the optimal pricing of three-type Table 6. The equilibrium solution under three regim es. Regimes Curb parking Off-road parking P & R facility Accommodated demand (person) Monopoly market 47.71%37.92% 14.37% 451 Oligopoly competition 30.25%52.01% 17.74% 848 Social optimum 40.81%44.71% 14.48% 528 parking facilities under three operation regimes (i.e., monopoly market, oligopoly competition, and social op- timum). To handle the interactions between pricing deci- sion and parking demand, we propose a bi-level pro- gramming model simulating the decision-making of parking operators and auto travelers, simultaneously. In the upper level, operators optimize the parking pricing to achieve certain objectives, which may differ with the operation regimes; the lower-level sub-model is the net- work equilibrium, where travelers choose the best travel alternative according to parking fee, metro fare, travel time, walking time, etc. In the light of the complexity of the proposed non-linear model, a sensitivity analysis based algorithm is adopted. A numerical experiment is de- signed to assess the proposed model. The results verify that operation regimes play an influential role on the pricing decisions and the final outcomes. It is to be men- tioned that parking operators’ competition under the oli- gopoly regime decreases the overall level of pricing, at- tracts more demand, and promotes the share of the P & R mode. These phenomena remind our policy-makers should carefully design the operation regimes to adjust the in- terests of multiple parking operators and mass travelers. Our further researches will address important issues to relax the constraints made in this study. For instance, travelers’ information about the network is not perfect, and the network is uncertain due to some random inci- dents, such as signal failure, road construction, and acci- dents. Interesting results are expected in evaluating the impacts of network uncertainty under different regimes. 8. Acknowledgements The research is supported by National Natural Science Foundation of China (No. 51178403, 51108391). REFERENCES [1] Z. C. Li, H. J. Huang, W. H. K. Lam and S. C. Wong, “Optimization Of Time-Varying Parking Charges and Parking Supply in Networks with Multiple User Classes and Multiple Parking Facilities,” Tsinghua Science and Technology, Vol. 12, No. 2, 2007, pp. 167-177. doi:10.1016/S1007-0214(07)70024-X [2] W. H. K. Lam, Z. C. Li, H. J. Huang and S. C. Wong, Copyright © 2012 SciRes. JTTs
 W. B. FAN, M. B. KHAN Copyright © 2012 SciRes. JTTs 266 “Modeling Time-Dependent Travel Choice Problems in Road Networks with Multiple User Classes and Multiple Parking Facilities,” Transportation Research Part B, Vol. 40, No. 5, 2006, pp. 368-395. doi:10.1016/j.trb.2005.05.003 [3] A. Glazer and E. Niskanen, “Parking Fees and Conges- tion,” Regional Science and Urban Economics, Vol. 22, No. 2, 1992, pp. 123-132. doi:10.1016/0166-0462(92)90028-Y [4] E. Verhoef, P. Nijkamp and P. Rietveld, “The Economics of Regulatory Parking Polices: The (IM) Posssibilities of Parking Policies in Traffic Regulation,” Transportation Research Part A, Vol. 29, No. 2, 1995, pp. 141-156. doi:10.1016/0965-8564(94)E0014-Z [5] Z. Y. Mei, J. Chen and W. Wang, “Curb Parking Pricing Method Based on Parking Choice Behavior,” Journal of Southeast University (English Edition), Vol. 22, No. 4, 2006, pp. 559-563. [6] S. Carrese, S. Gori and T. Picano, “A Parking Equilib- rium Model for Park Pricing and Park & Ride Planning,” Transportation Systems, Vol. 3, No. 6, 1997, pp. 1235- 1239. [7] R. Garcia and A. Marin, “Parking Capacity and Pricing in Park’n Ride Trips: A Continuous Equilibrium Network Design Problem,” Annals of Operations Research, Vol. 116, No. 1-4, 2002, pp. 153-178. doi:10.1023/A:1021332414941 [8] J. Y. T. Wang, H. Yang and R. Lindsey, “Locating and Pricing Park-and-Ride Facilities in a Linear Monocentric City with Deterministic Mode Choice,” Transportation Research Part B, Vol. 38, No. 8, 2004, pp. 709-731. doi:10.1016/j.trb.2003.10.002 [9] Z. C. Li, W. H. K. Lam and S. C. Wong, “The Optimal Transit Fare Structure under Different Market Regimes with Uncertainty in the Network,” Networks and Spatial Economics, Vol. 9, No. 2, 2008, pp. 191-216. doi:10.1007/s11067-007-9058-z [10] S. P. Anderson and A. D. Palma, “The Economics of Pricing Parking,” Journal of Urban Economics, Vol. 55, 2004, pp. 1-20. doi:10.1016/j.jue.2003.06.004 [11] J. F. Tsai and C. P. Chu, “Economic Analysis of Collect- ing Parking Fees by a Private Firm,” Transportation Re- search Part A, Vol. 40, No. 8, 2006, pp. 690-697. doi:10.1016/j.tra.2005.12.001 [12] H. Yang, “Sensitivity Analysis for the Elastic-Demand Network Equilibrium Problem with Applications,” Trans- portation Research B, Vol. 31, No. 2, 1997, pp. 55-70. doi:10.1016/S0191-2615(96)00015-X [13] R. B. Dial, “A Probabilistic Multipath Traffic Assignment Algorithm Which Obviates Path Enumeration,” Trans- portation Research, Vol. 5, 1971, pp. 83-111. doi:10.1016/0041-1647(71)90012-8 [14] Y. Sheffi, “Urban Transportation Networks: Equilibrium Analysis with Mathematical Programming Methods,” Prentice-Hall, Englewood Cliffs, 1985. [15] E. F. Moore, “The Shortest Path through a Maze,” Har- vard University Press, Cambridge, 1957.
|