Applied Mathematics
Vol. 4 No. 2 (2013) , Article ID: 28420 , 7 pages DOI:10.4236/am.2013.42061
Wavelet Density Estimation and Statistical Evidences Role for a GARCH Model in the Weighted Distribution
Department of Statistics, Ferdowsi University of Mashhad, Mashhad, Iran
Email: abbaszadeh.mo@stu-mail.um.ac.ir, emadi@um.ac.ir
Received November 10, 2012; revised December 10, 2012; accepted December 17, 2012
Keywords: Density Estimation; GARCH Model; Weighted Distribution; Wavelets; Statistical Evidences; Strongly Mixing
ABSTRACT
We consider n observations from the GARCH-type model: Z = UY, where U and Y are independent random variables. We aim to estimate density function Y where Y have a weighted distribution. We determine a sharp upper bound of the associated mean integrated square error. We also make use of the measure of expected true evidence, so as to determine when model leads to a crisis and causes data to be lost.
1. Introduction
We suppose that is a sample of a strictly stationary and exponentially strongly mixing process
where, for any
,
(1)
is a sequence of identically distributed random variables with common known density
and
is a sequence of identically distributed random variables with common unknown density
. For any
,
and
are independent. We suppose that
is a weighted density of the form
(2)
where is a known positive function,
an unknown density of a random variable
and
is the unknown normalization parameter:
Our goal is to estimate when only
are observed. The Equation (1) is a GARCH-type time series model classically encountered in financial models see [1] and practical examples of Equation (2) can be found in e.g. [2-4].
In this article, we construct a linear wavelet estimator and measure its performance by determining upper bounds of the mean integrated squared error (MISE) over Besov space.
In what follows, we have also surveyed the role of data and evidential inference in the model. The data play a very important essential role in statistical analysis, to the extent that many statistical researchers believe in the famous saying: “Ask the data.” We consider the Test
(3)
for the model and we evaluate the sensitivity of the value in the test hypotheses. In this test, the evaluation criterion is the area between the curves of the cumulative distribution functions under and
hypotheses. Details on evidential inference can be found in [5,6]. Also [7] have studied about Comparing of record data and random observation based on statistical evidence.
Through the rest of the paper, at first assumptions and then an introduction about wavelets are presented in Section 2. The estimators and results are given in Section 3. In Section 4, general explanations regarding evidential inference and its application in a test. The proofs are gathered in Section 5.
2. Assumptions and Wavelets
2.1. Assumptions
We formulate the following assumptions:
• Without loss of generality, we assume that and
have the support
and
where
•
• We suppose that for any, the
-th strongly mixing coefficient of
by
where, for any, let
be the
-algebra generated by
and
is the
-algebra generated by
.
We suppose that there exist three (known) constants, and
such that
This assumption is satisfied by a large class of GARCH processes. See e.g. [8-10].
• For any, it follows from the independence of
and
that the density of
is
•
• We suppose that there exists two constants, and
, such that
(4)
and
(5)
2.2. Wavelets and Besov Balls
Let be a positive integer, and
and
be the Daubechies wavelets
which satisfy
.
Set
Then, there exists an integer such that, for any integer
, the collection
is an orthonormal basis of (the space of squareintegrable functions on 0,1). We refer to [11].
For any integer, any
can be expanded on
as
where and
are the wavelet coefficients of
defined by
(6)
Let and
. A function
belongs to
if and only if there exists a constant
(depending on
) such that the associated wavelet coefficients Equation (6) satisfy
We set. Details on Besov balls can be found in [12].
3. Estimators and Results
Firstly, we consider the following estimator for
(7)
Then, for any integer and any
, we estimate
(8)
where, for any,
is the operator
(9)
and
are similar with multiplicative censoring model (see [13]).
We are now in the position to define the considered estimators for. Suppose that
. We define the linear estimator
by
(10)
where is defined by Equation (8) and
is the integer satisfying
(11)
• Lemma 3.1
• Let be Equation (7) and
. Then we have
•
• Let be Equation (1),
be Equation (9) and for any integer
and any
,
. Then we have
•
• For any integer and any
, let
be Equation (8) and
.
Then, under the assumptions of Subsection 2.1, there exists a constant such that
Proposition 3.1 For any integer and any
Then, under the assumptions of Subsection 2.1•
Proposition 3.2 Let, for any integer
and any
, let
be Equation (8) and
. Then• there exists a constant
such that
•
• there exists a constant such that
•
• there exists a constant such that
Theorem 3.1 (Upper bound for) Consider Equation (1) under the assumptions of Subsection 2.1. Suppose that
with
. For any
and
be Equation (10), then there exists a constant
such that
Remark that is the slower than the optimal one in the standard density estimation problem i.e.
(see e.g. [14, Chapter 10]). This deterioration is due to the presence of GARCH model and weighted distribution.
4. Statistical Evidence
4.1. Statistical Inference
The evidential approach to statistical inference concerns a novel approach in statistical analysis. Evidential inference is solely based on data as evidence and calculation of the evidence strength. It is not influenced by mental and personal components and factors such as former beliefs and loss functions. Using evidential inference in the model Equation (1), we will survey when censoring of data will lead to considerable data loss, and we will determine the time when data is lost by determining an appropriate criterion. In the model for
, the data observed from the variable
are denoted by the subscript (cen), and the data observed from the variable
are denoted by the subscript (ncen). Considering the Test Equation (3) in the above model, due to the symmetry of the test hypotheses in evidential methods and without losing the generality of the problem, the value of is assumed to be
. In order to support
and
hypotheses, we now use the following criterion:
(12)
where and
are the measure of expected true evidence in the censored and uncensored data respectively, and
is the criterion of the support of data from
hypothesis against
hypothesis. This support criterion is optimal when the area between the two curves of
cumulative functions under
and
is maximum, please see [15]. This area which is denoted by
in the form of
where is the cumulative distribution function of
and
is the mean value of
under
hypotheses. In view of [6], the support criterion
is defined as follows:
(13)
where is the likelihood ratio and for the two censored and uncensored cases we have
(14)
where and
are likelihood functions for
and
variables respectively, in the Equation (1) under
hypotheses.
4.2. Measuring Statistical Evidence
We consider i.i.d case for variables in the Equations (1) and (2), also set and then, we investigate the behavior of
by means of simulation. In addition, we analyze
and
hypotheses in Test Equation (3) by determining support criterion
of the measure of expected true evidence
. The programming codes of this part are written in the MAPLE (15) environment.
Example 1. In this example, we generate data from gamma and uniform distribution as follows, considering multiplicative censoring model:
Then, according to Equations (13) and (14), we calculate the support criterion and the likelihood ratio via below relations:
such that
and
such that
For different values of and
, we calculate the value of
according to Equation (12). The results can be observed in Table 1. By carefully considering this table, it is observed that as the value of
increases (which implies the distance growth between
and
), the value of
gets closer to one. In other words,
approaches
more and more. This fact can be interpreted in this way that if the distance between
and
is large, the data lost in censored data
is negligible. That is to say, evidential inference draws our attention to the time when
is close to
. The above analysis can also be observed in Figure 1.
In what follows, the variations of sample volume ratio increase against are investigated, and the value of
in the
equation is determined for different values of
and
.
If then
. The result can be viewed in Figure 2, If
then
. The result can be viewed in Figure 3, If
then. The result can be viewed in Figure 4.
The above results can be interpreted in this way that as the sample volume increases from a certain stage on, the value of remains constant. In other words, it can be intuitively said that the
ratio tends to the constant
value, which this also leads to an increase in evidential strength.
5. Proofs
In this section, denotes any constant that does not depend on
and
. Its value may change from one term to another and may depends on
or
.
Table 1. Computed values for γ.
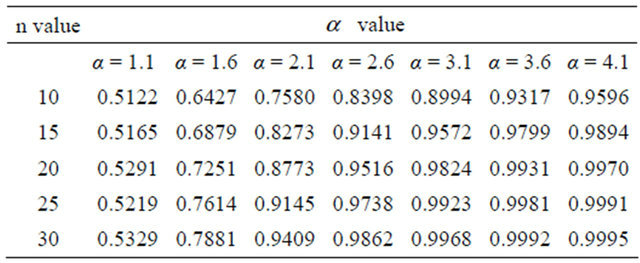
Figure 1. γ computed from gamma distribution for different values of n.
Figure 2. The most relative changes when sample size n1 = 20 increases to n2 = 25 happens in α = 1.6.
Figure 3. The most relative changes when sample size n1 = 10 increases to n2 = 15 happens in α = 2.1.
Proof of Lemma 3.1.
Proof can be found in [13].
Proof of proposition 3.1.
1. By Equation (9) and Equation (5), we have
(15)
Figure 4. The most relative changes when sample size n1 = 10 increases to n2 = 20 happens in α = 2.2.
Therefore
(16)
2. By Equation (9) and Equation (5), we have
(17)
By Equation (4) and under the assumptions of Subsection 2.1 and also doing the change of variables, we have
(18)
Using again Equation (4) and the assumptions of Subsection 2.1, the equality
and doing the change of variables, we obtain
(19)
Combining Equations (17)-(19), we obtain
(20)
The proof of Proposition 3.1 is complete.
Proof of Proposition 3.2.
1. We have
(21)
Using Equation (20) we obtain
(22)
also
(23)
By the Davydov inequality (see [16]) and for any we obtain
(24)
Putting Equation (24), Equation (16) and Equation (20) together, we have
(25)
Since, we obtain
(26)
Putting Equations (21) and (22) and Equation (26) together, we have
(27)
2. We have
(28)
By Equation (5), we have
(29)
By the Davydov inequality (see [16]) we obtain
(30)
Combining Equations (28)-(30), we obtain
(31)
3. Using Lemma (3.1), Equation (27) and Equation (31), then
(32)
The proof of Proposition 3.2 is complete.
Proof of Theorem 3.1. For any integer, any
can be expanded on
as
where
We obtain
where
Using Proposition 3.2 and inequalitity Equation (11)
and since, we have
. Hence
Hence we have
This ends the proof of Theorem 3.1.
REFERENCES
- M. Carrasco and X. Chen, “Mixing and Moment Properties of Various GARCH and Stochastic Volatility Models,” Econometric Theory, Vol. 18, No. 1, 2002, pp. 17-39. doi:10.1017/S0266466602181023
- S. T. Buckland, D. R. Anderson, K. P. Burnham and J. L. Laake, “Distance Sampling: Estimating Abundance of Biological Populations,” Chapman and Hall, London, 1993.
- D. Cox, “Some Sampling Problems in Technology,” In: N. L. Johnson and H. Smith Jr., Eds., New Developments in Survey Sampling, Wiley, New York, 1969, pp. 506-527.
- J. Heckman, “Selection Bias and Self-Selection,” The New Palgrave: A Dictionary of Economics, MacMillan Press, Stockton, 1985, pp. 287-296.
- R. Royall, “Statistical Evidence,” A Likelihood Paradigm, Chapman and Hall, London, 1997.
- R. Royall, “On the Probability of Observing Misleading Statistical Evidence,” Journal of the American Statistical Association, Vol. 95, No. 451, 2000, pp. 760-780. doi:10.1080/01621459.2000.10474264
- M. Emadi, J. Ahmadi and N. R. Arghami, “Comparing of Record Data and Random Observation Based on Statistical Evidence,” Statistical Papers, Vol. 48, No. 1, 2007, pp. 1-21. doi:10.1007/s00362-006-0313-z
- C. Chesneau and H. Doosti, “Wavelet Linear Density Estimation for a GARCH Model under Various Dependence Structures,” Journal of the Iranian Statistical Society, Vol. 11, No. 1, 2012, pp. 1-21.
- P. Doukhan, “Mixing Properties and Examples,” Lecture Notes in Statistics 85, Springer Verlag, New York, 1994.
- D. Modha and E. Masry, “Minimum Complexity Regression Estimation with Weakly Dependent Observations,” IEEE Transactions on Information Theory, Vol. 42, No. 6, 1996, pp. 2133-2145. doi:10.1109/18.556602
- A. Cohen, I. Daubechies, B. Jawerth and P. Vial, “Wavelets on the Interval and Fast Wavelet Transforms,” Applied and Computational Harmonic Analysis, Vol. 24, No. 1, 1993, pp. 54-81. doi:10.1006/acha.1993.1005
- Y. Meyer, “Wavelets and Operators,” Cambridge University Press, Cambridge, 1992.
- M. Abbaszadeh, C. Chesneau and H. Doosti, “Nonparametric Estimation of Density under Bias and Multiplicative Censoring via Wavelet Methods,” Statistics and Probability Letters, Vol. 82, No. 5, 2012, pp. 932-941. doi:10.1016/j.spl.2012.01.016
- W. Härdle, G. Kerkyacharian, D. Picard and A. Tsybakov, “Wavelet, Approximation and Statistical Applications,” Lectures Notes in Statistics, Springer Verlag, New York, 1998, Vol. 129.
- M. Emadi and N. R. Arghami, “Some Measure of Support for Statistical Hypotheses,” Journal of Statical Theory and Applications, Vol. 2, No. 2, 2003, pp. 165-176.
- Y. Davydov, “The Invariance Principle for Stationary Processes,” Theory of Probability & Its Applications, Vol. 15, No. 3, 1970, pp. 498-509. doi:10.1137/1115050