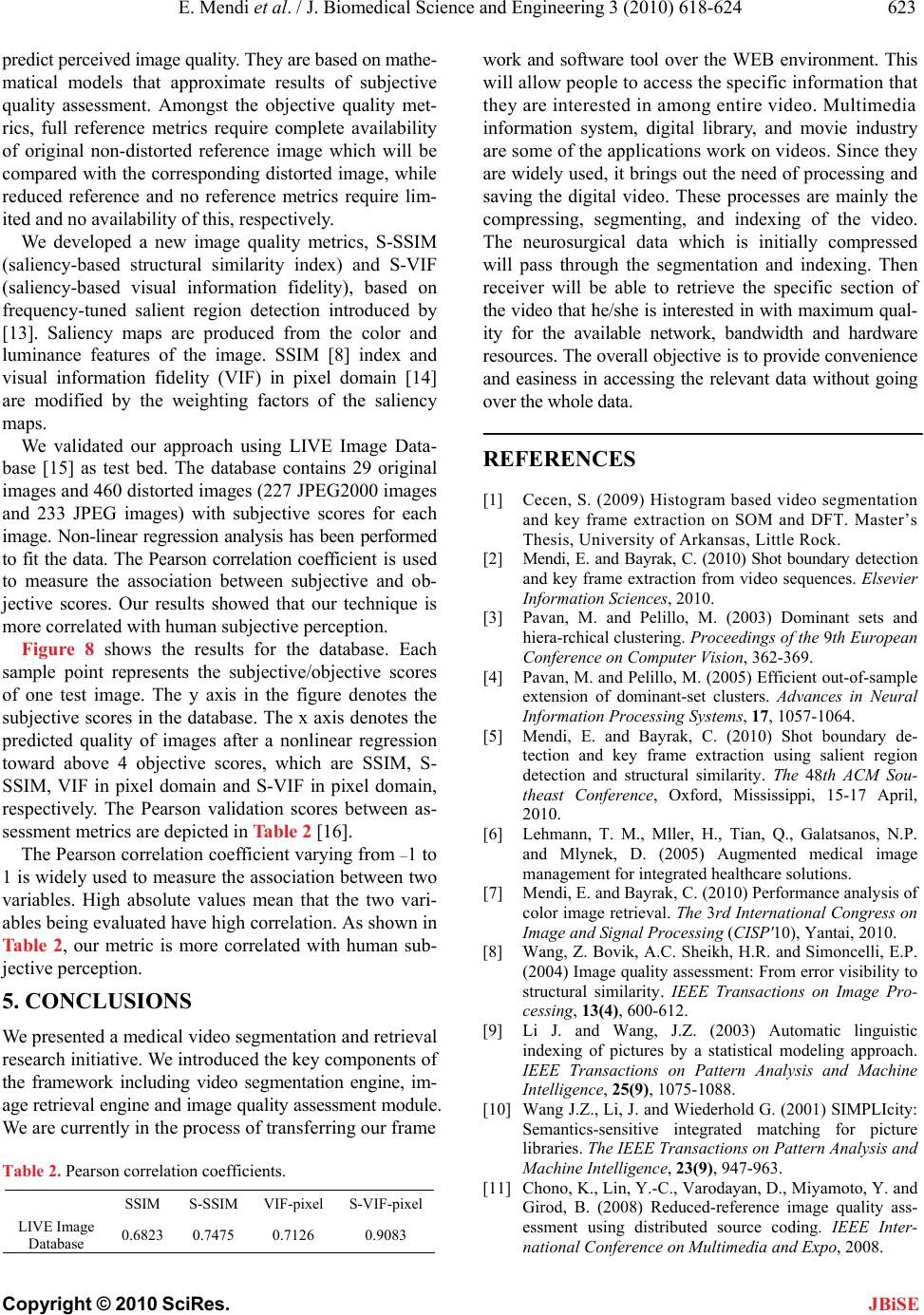
E. Mendi et al. / J. Biomedical Science and Engineering 3 (2010) 618-624
Copyright © 2010 SciRes.
623
JBiSE
predict perceived image quality. They are based on mathe-
matical models that approximate results of subjective
quality assessment. Amongst the objective quality met-
rics, full reference metrics require complete availability
of original non-distorted reference image which will be
compared with the corresponding distorted image, while
reduced reference and no reference metrics require lim-
ited and no availability of this, respectively.
We developed a new image quality metrics, S-SSIM
(saliency-based structural similarity index) and S-VIF
(saliency-based visual information fidelity), based on
frequency-tuned salient region detection introduced by
[13]. Saliency maps are produced from the color and
luminance features of the image. SSIM [8] index and
visual information fidelity (VIF) in pixel domain [14]
are modified by the weighting factors of the saliency
maps.
We validated our approach using LIVE Image Data-
base [15] as test bed. The database contains 29 original
images and 460 distorted images (227 JPEG2000 images
and 233 JPEG images) with subjective scores for each
image. Non-linear regression analysis has been performed
to fit the data. The Pearson correlation coefficient is used
to measure the association between subjective and ob-
jective scores. Our results showed that our technique is
more correlated with human subjective perception.
Figure 8 shows the results for the database. Each
sample point represents the subjective/objective scores
of one test image. The y axis in the figure denotes the
subjective scores in the database. The x axis denotes the
predicted quality of images after a nonlinear regression
toward above 4 objective scores, which are SSIM, S-
SSIM, VIF in pixel domain and S-VIF in pixel domain,
respectively. The Pearson validation scores between as-
sessment metrics are depicted in Table 2 [16].
The Pearson correlation coefficient varying from –1 to
1 is widely used to measure the association between two
variables. High absolute values mean that the two vari-
ables being evaluated have high correlation. As shown in
Table 2, our metric is more correlated with human sub-
jective perception.
5. CONCLUSIONS
We presented a medical video segmentation and retrieval
research initiative. We introduced the key components of
the framework including video segmentation engine, im-
age retrieval engine and image quality assessment module.
We are currently in the process of transferring our frame
Table 2. Pearson correlation coefficients.
SSIM S-SSIMVIF-pixel S-VIF-pixel
LIVE Image
Database 0.6823 0.7475 0.7126 0.9083
work and software tool over the WEB environment. This
will allow people to access the specific information that
they are interested in among entire video. Multimedia
information system, digital library, and movie industry
are some of the applications work on videos. Since they
are widely used, it brings out the need of processing and
saving the digital video. These processes are mainly the
compressing, segmenting, and indexing of the video.
The neurosurgical data which is initially compressed
will pass through the segmentation and indexing. Then
receiver will be able to retrieve the specific section of
the video that he/she is interested in with maximum qual-
ity for the available network, bandwidth and hardware
resources. The overall objective is to provide convenience
and easiness in accessing the relevant data without going
over the whole data.
REFERENCES
[1] Cecen, S. (2009) Histogram based video segmentation
and key frame extraction on SOM and DFT. Master’s
Thesis, University of Arkansas, Little Rock.
[2] Mendi, E. and Bayrak, C. (2010) Shot boundary detection
and key frame extraction from video sequences. Elsevier
Information Sciences, 2010.
[3] Pavan, M. and Pelillo, M. (2003) Dominant sets and
hiera-rchical clustering. Proceedings of the 9th European
Conference on Computer Vision, 362-369.
[4] Pavan, M. and Pelillo, M. (2005) Efficient out-of-sample
extension of dominant-set clusters. Advances in Neural
Information Processing Systems, 17, 1057-1064.
[5] Mendi, E. and Bayrak, C. (2010) Shot boundary de-
tection and key frame extraction using salient region
detection and structural similarity. The 48th ACM Sou-
theast Conference, Oxford, Mississippi, 15-17 April,
2010.
[6] Lehmann, T. M., Mller, H., Tian, Q., Galatsanos, N.P.
and Mlynek, D. (2005) Augmented medical image
management for integrated healthcare solutions.
[7] Mendi, E. and Bayrak, C. (2010) Performance analysis of
color image retrieval. The 3rd International Congress on
Image and Signal Processing (CISP'10), Yantai, 2010.
[8] Wang, Z. Bovik, A.C. Sheikh, H.R. and Simoncelli, E.P.
(2004) Image quality assessment: From error visibility to
structural similarity. IEEE Transactions on Image Pro-
cessing, 13(4), 600-612.
[9] Li J. and Wang, J.Z. (2003) Automatic linguistic
indexing of pictures by a statistical modeling approach.
IEEE Transactions on Pattern Analysis and Machine
Intelligence, 25(9), 1075-1088.
[10] Wang J.Z., Li, J. and Wiederhold G. (2001) SIMPLIcity:
Semantics-sensitive integrated matching for picture
libraries. The IEEE Transactions on Pattern Analysis and
Machine Intelligence, 23(9), 947-963.
[11] Chono, K., Lin, Y.-C., Varodayan, D., Miyamoto, Y. and
Girod, B. (2008) Reduced-reference image quality ass-
essment using distributed source coding. IEEE Inter-
national Conference on Multimedia and Expo, 2008.