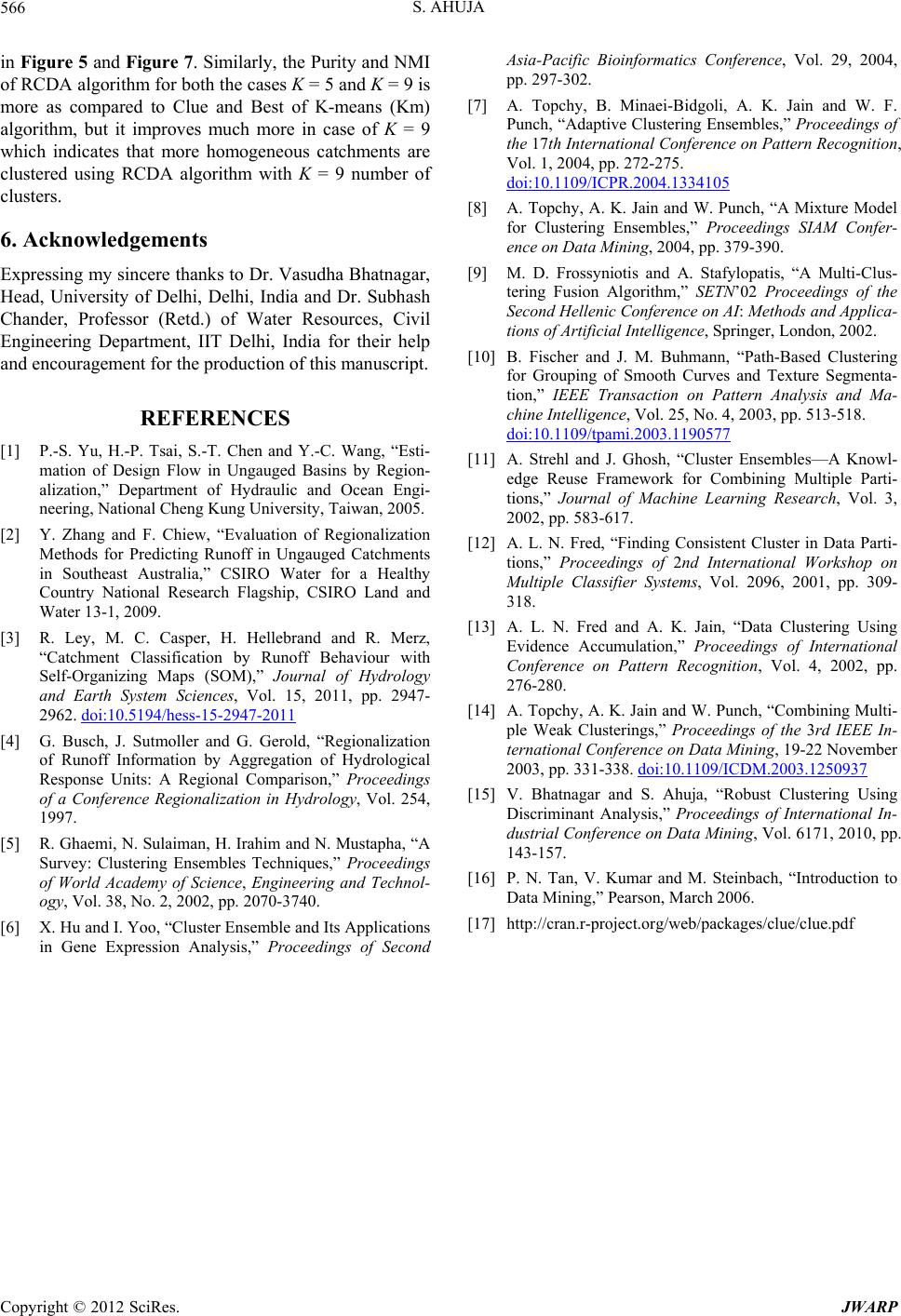
S. AHUJA
Copyright © 2012 SciRes. JWARP
566
in Figure 5 and Figure 7. Similarly, the Purity and NMI
of RCDA algorithm for both the cases K = 5 and K = 9 is
more as compared to Clue and Best of K-means (Km)
algorithm, but it improves much more in case of K = 9
which indicates that more homogeneous catchments are
clustered using RCDA algorithm with K = 9 number of
clusters.
6. Acknowledgements
Expressing my sincere thanks to Dr. Vasudha Bhatnagar,
Head, University of Delhi, Delhi, India and Dr. Subhash
Chander, Professor (Retd.) of Water Resources, Civil
Engineering Department, IIT Delhi, India for their help
and encouragement for the production of this manuscript.
REFERENCES
[1] P.-S. Yu, H.-P. Tsai, S.-T. Chen and Y.-C. Wang, “Esti-
mation of Design Flow in Ungauged Basins by Region-
alization,” Department of Hydraulic and Ocean Engi-
neering, National Cheng Kung University, Taiwan, 2005.
[2] Y. Zhang and F. Chiew, “Evaluation of Regionalization
Methods for Predicting Runoff in Ungauged Catchments
in Southeast Australia,” CSIRO Water for a Healthy
Country National Research Flagship, CSIRO Land and
Water 13-1, 2009.
[3] R. Ley, M. C. Casper, H. Hellebrand and R. Merz,
“Catchment Classification by Runoff Behaviour with
Self-Organizing Maps (SOM),” Journal of Hydrology
and Earth System Sciences, Vol. 15, 2011, pp. 2947-
2962. doi:10.5194/hess-15-2947-2011
[4] G. Busch, J. Sutmoller and G. Gerold, “Regionalization
of Runoff Information by Aggregation of Hydrological
Response Units: A Regional Comparison,” Proceedings
of a Conference Regionalization in Hydrology, Vol. 254,
1997.
[5] R. Ghaemi, N. Sulaiman, H. Irahim and N. Mustapha, “A
Survey: Clustering Ensembles Techniques,” Proceedings
of World Academy of Science, Engineering and Technol-
ogy, Vol. 38, No. 2, 2002, pp. 2070-3740.
[6] X. Hu and I. Yoo, “Cluster Ensemble and Its Applications
in Gene Expression Analysis,” Proceedings of Second
Asia-Pacific Bioinformatics Conference, Vol. 29, 2004,
pp. 297-302.
[7] A. Topchy, B. Minaei-Bidgoli, A. K. Jain and W. F.
Punch, “Adaptive Clustering Ensembles,” Proceedings of
the 17th International Conference on Pattern Recognition,
Vol. 1, 2004, pp. 272-275.
doi:10.1109/ICPR.2004.1334105
[8] A. Topchy, A. K. Jain and W. Punch, “A Mixture Model
for Clustering Ensembles,” Proceedings SIAM Confer-
ence on Data Mining, 2004, pp. 379-390.
[9] M. D. Frossyniotis and A. Stafylopatis, “A Multi-Clus-
tering Fusion Algorithm,” SETN’02 Proceedings of the
Second Hellenic Conference on AI: Methods and Applica-
tions of Artificial Intelligence, Springer, London, 2002.
[10] B. Fischer and J. M. Buhmann, “Path-Based Clustering
for Grouping of Smooth Curves and Texture Segmenta-
tion,” IEEE Transaction on Pattern Analysis and Ma-
chine Intelligence, Vol. 25, No. 4, 2003, pp. 513-518.
doi:10.1109/tpami.2003.1190577
[11] A. Strehl and J. Ghosh, “Cluster Ensembles—A Knowl-
edge Reuse Framework for Combining Multiple Parti-
tions,” Journal of Machine Learning Research, Vol. 3,
2002, pp. 583-617.
[12] A. L. N. Fred, “Finding Consistent Cluster in Data Parti-
tions,” Proceedings of 2nd International Workshop on
Multiple Classifier Systems, Vol. 2096, 2001, pp. 309-
318.
[13] A. L. N. Fred and A. K. Jain, “Data Clustering Using
Evidence Accumulation,” Proceedings of International
Conference on Pattern Recognition, Vol. 4, 2002, pp.
276-280.
[14] A. Topchy, A. K. Jain and W. Punch, “Combining Multi-
ple Weak Clusterings,” Proceedings of the 3rd IEEE In-
ternational Conference on Data Mining, 19-22 November
2003, pp. 331-338. doi:10.1109/ICDM.2003.1250937
[15] V. Bhatnagar and S. Ahuja, “Robust Clustering Using
Discriminant Analysis,” Proceedings of International In-
dustrial Conference on Data Mining, Vol. 6171, 2010, pp.
143-157.
[16] P. N. Tan, V. Kumar and M. Steinbach, “Introduction to
Data Mining,” Pearson, March 2006.
[17] http://cran.r-project.org/web/packages/clue/clue.pdf