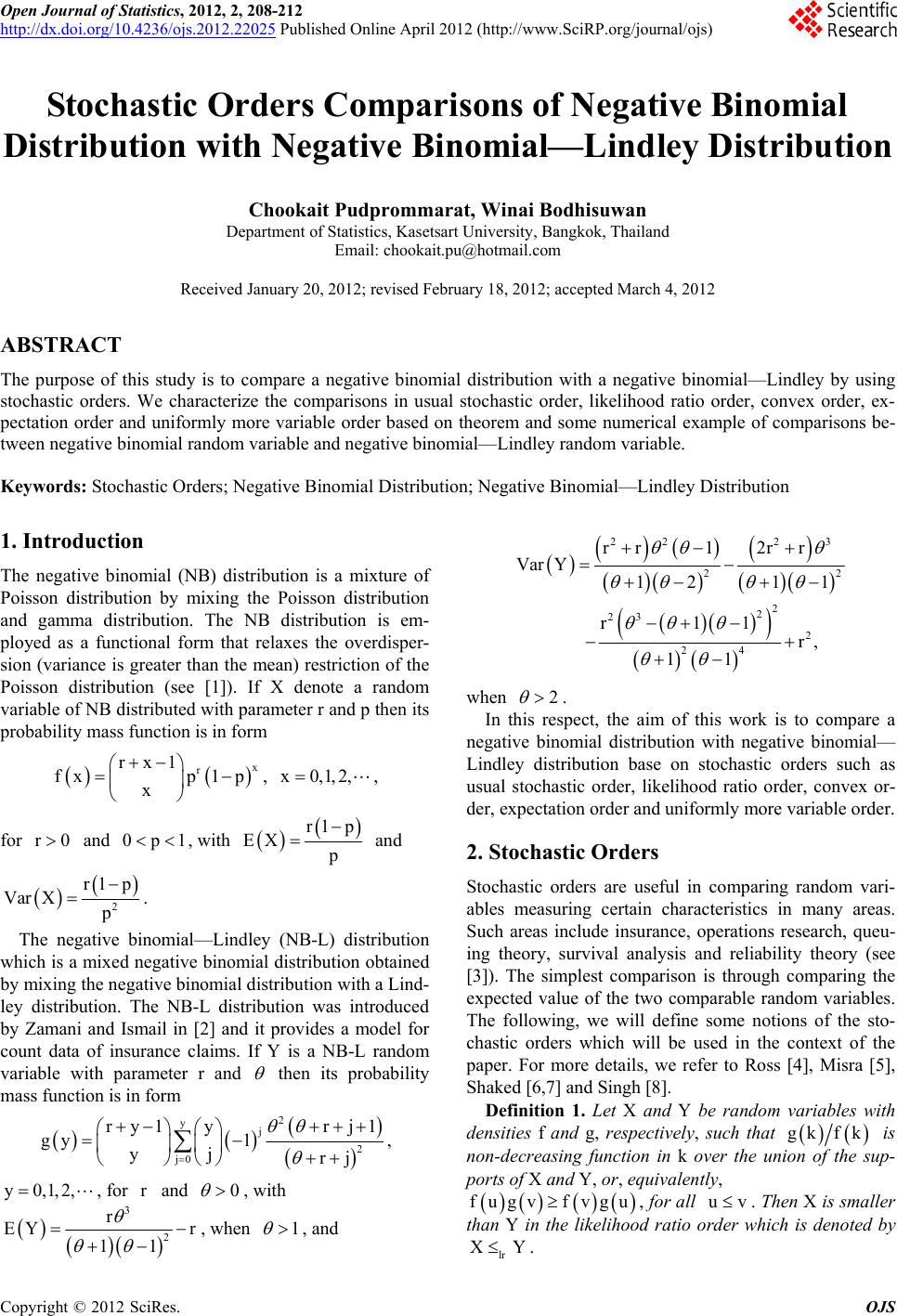 Open Journal of Statistics, 2012, 2, 208-212 http://dx.doi.org/10.4236/ojs.2012.22025 Published Online April 2012 (http://www.SciRP.org/journal/ojs) Stochastic Orders Comparisons of Negative Binomial Distribution with Negative Binomial—Lindley Distribution Chookait Pudprommarat, Winai Bodhisuwan Department of Statistics, Kasetsart University, Bangkok, Thailand Email: chookait.pu@hotmail.com Received January 20, 2012; revised February 18, 2012; accepted March 4, 2012 ABSTRACT The purpose of this study is to compare a negative binomial distribution with a negative binomial—Lindley by using stochastic orders. We characterize the comparisons in usual stochastic order, likelihood ratio order, convex order, ex- pectation order and uniformly more variable order based on theorem and some numerical example of comparisons be- tween negative binomial random variable and negative binomial—Lindley random variable. Keywords: Stochastic Orders; Negative Binomial Distribution; Negative Binomial—Lindley Distribution 1. Introduction The negative binomial (NB) distribution is a mixture of Poisson distribution by mixing the Poisson distribution and gamma distribution. The NB distribution is em- ployed as a functional form that relaxes the overdisper- sion (variance is greater than the mean) restriction of the Poisson distribution (see [1]). If X denote a random variable of NB distributed with parameter r and p then its probability mass function is in form rx fx x r 1 p 1 p x 0p1 , , x0,1,2, for and , with r0r1 p EX and 2 r1 Xp p Var . The negative binomial—Lindley (NB-L) distribution which is a mixed negative binomial distribution obtained by mixing the negative binomial distribution with a Lind- ley distribution. The NB-L distribution was introduced by Zamani and Ismail in [2] and it provides a model for count data of insurance claims. If Y is a NB-L random variable with parameter r and then its probability mass function is in form 2 yj ry1 y gy 1 2 j0 rj1 yj rj r 0 , y0,1,2,, for and , with 3 r 11 1 2rEY , when , and 22 23 22 2 2 23 2 24 rr1 2rr Var Y 12 11 r11 r, 11 2 when . In this respect, the aim of this work is to compare a negative binomial distribution with negative binomial— Lindley distribution base on stochastic orders such as usual stochastic order, likelihood ratio order, convex or- der, expectation order and uniformly more variable order. 2. Stochastic Orders Stochastic orders are useful in comparing random vari- ables measuring certain characteristics in many areas. Such areas include insurance, operations research, queu- ing theory, survival analysis and reliability theory (see [3]). The simplest comparison is through comparing the expected value of the two comparable random variables. The following, we will define some notions of the sto- chastic orders which will be used in the context of the paper. For more details, we refer to Ross [4], Misra [5], Shaked [6,7] and Singh [8]. Definition 1. Let X and Y be random variables with densities f and g, respectively, such that gkf k is non-decreasing function in k over the union of the sup- ports of X and Y, or, equivalently, fugvfvguuv lr XY , for all . Then X is smaller than Y in the likelihood ratio order which is denoted by . C opyright © 2012 SciRes. OJS
 C. PUDPROMMARAT ET AL. 209 Definition 2. Let X and Y be two random variables such that , for all . Then X is smaller than Y in the usual stochastic order which is denoted by . PrYk XY XkPr k EY st Definition 3. Let X and Y be two random variables such that , for every real valued convex function EX where expectations are assumed to be existed. Then X is smaller than Y in convex order which is denoted by . cx Definition 4. Let X and Y be two random variables such that , where expectations are as- sumed to be existed. Then X is smaller than Y in the ex- pectation order which is denoted by . XY XYEE XY E Definition 5. Let X and Y be two random variables with densities f and g, respectively. Recall that supp (X) and supp(Y) denote the respective support of X and re- spective support of Y, such that supp(X) supp(Y) and the ratio fk gk uv XY is a unimodal function over supp(Y). Then X is smaller than Y in uniformly more variable order w hi c h is denoted by . 3. Comparison We make comparisons between the negative binomial random variable and negative binomial—Lindley random variable with respect to the likelihood ratio order, sto- chastic order, convex order, expectation order and uni- form more variable order. The following lemma will be useful in proving the main results. Lemma 1. Define, k1 j j0 kj j0 k1 1 j ak 1k(1) j 2 2 rj 1 rj rj1 rj and j 1 r 1 mm1m k0,1,2, ak k0,1,2, 2, km k0,1,2, k k j0 jr j , m, , Then, 0m1 1) is a non- increasing function of , 2) For each fixed , is concave function of . k0,1, m0,1 Proof. 1) We may write for , that ak k d EW d h k1 r 0 k r 0 e1e h; ak 1 e1e h; , where is the Lindley distribution defined by 2 z zehz; 1 1 and 0, z0 and k W is a random variable havinprobaility density function: g the b k rz z kk 1e1eh z; z2 j 2 j0 krj 1 (1) jrj , z0. For fixed k0,1,2,, the ratio k1 k xx is obviously a non-increasing function of x0. Then, by 1 and 2, welrk1 Definitions have k WW , which yields kst k1 WW and therefore EW kk1 EW or, equivalently, akak 1. This proves ak is a non-increasing function of k0,1 2) For k0 ,2,. , note that mm is bothex and concave. For k1,2, 0 conv rite , we can w j 1 j r k jk1 jr1 m1m1m, m, 0m1. (1) The relationship between negative binomial and beta probabilities is of the form 1p jr1 rk1 jk kr1!rr1 0 1pt1t dt jk , 1 !r1! k0,1,2, . Therefore, km in Equation (1) can be written as 1 r 1m 0 m1 m r1 k k kr! t1tdt k!r1 ! , , 0m1 . Thus, k1 11 21 rr k kr! 1 mm1m0 rr 1!r! 2 m , m , 0m1 . which proves concavity. □ Theorem 1. Let X~NB r,p, Y~NB-L r, and 2 r2 r p 03 r1 , 1 2r 12 r1 p r , 2 23 11 p . Th 210 0pp p 1 Furthermore, 1) lr XY en . if an 0 d only ifp, 2) st XY if and only if 1 p, 3) X Ely if 2E Y if and on p, of. he likeltwn Y and X can be written as Pro 1) Tihood ratio ordeeer be Copyright © 2012 SciRes. OJS
 C. PUDPROMMARAT ET AL. 210 2 k k r krj1 , X kp1p 1 k0,1,2,. (2) By Definition 1, we have j 2 j0 1 j Pr Y kr j lk Pr lr k1 j j0 kj j0 0 XYlklk+1, , 2 2 0,1,2, , 1rj1 1 rj p1, krj1 1 jrj pak, , 0,1,2,, ppa0. kk kk Since k+ j ak is non-increasing in k (by part 1) in e, then 0 Lmma 1) p which provides a necessary and conditio for the sufficientn lk in Equation (2) to be o b nn-decreasing. This completes the proof of the result. 2) Let st XYy Definition 2, we have 2 r 2 r1 PrY 0PrX 0p, r 1 2r 1 2 r1 pp. r Conversely, suppose1 0p p1 that . k0 For ,1,2,, consider ki r i0 2 ki j 2 i0 j0 ir1 kp 1p rj1ir1i 1, ij 1rj X~ 1 X~NB r,p, then 1st 1 XX. q k i 11 1 pkPrX kPrYi and if lr XX Conse NB r,p and nce, we get ly, . He uent k i rr i0 i0 ir1 ir 1p p1p ii kk , pp . For fixed and therefore 1 k0,1,2,, 1 2r 2 r1 r , 1 p pexp and ~ Lindley . We get 1 k kp i r p1 1 i0 ki j 2 i0 j0 1p i rj1ir1i 1, ij 1rj 1 i 1 krr r p i0 ki r i0 ir1 kEP1EP i ir1 EP1P, i Using concave function (by part 2) in Lemma 1), 1 pk can be written as 1 rr pk k kEPEP . Applying Jensen’s inequality to concave function, we have rr EPE P and k0 for 2 ir1 kk1 p k , 0,1,2,. Conversely, where XYkst at im- plies th X 0PrY 0Pr . This proves 1 p. 3) The proofs of the results are obvious. □ Theorem 2. Suppose that, for every 0t1, tp 1Pr0 , then 1) No value of 0p1 ca that XYn ensurest , 2uv XY ) if an. d only if 1 0pp 1 Proof. 1) We find Pr Xk0,1, Rk Pr Yk , k, b tor and 2,y re- frdowing: ying the numeraenominator as foll j r jk 1p r1 k1 r1 0 r k1p , j kr1! t1 tdt, k1!r1! 1p1tdt, k1!r1! 1p 1p kr1! . k1!r1!r1p k For any 1 0pp 1 k1 0 1p kr 1! jr1 p Pr X , k0,1,2,, 2 ij 2 ik j0 i r 0 i r ik 0 1e r1 k1 0 rj1 ri1i Pr Yk1, ij rj ri1 e1eh;d, i ri1 e1eh;d, i kr1! t1tdt k1!r1! ik 0 1 log 1t 1r1 k1 00 1r1 k1 0 h; d, kr1! t1th;ddt, k1!r1! kr1! 1 t1tHlog;dt, k1!r1! 1t Copyright © 2012 SciRes. OJS
 C. PUDPROMMARAT ET AL. Copyright © 2012 SciRes. OJS 211 Table 1. Stochastic orders comparisons of NB random variables with NB-L random variables. Random Variables Order Comparisons of NB Random Variables with NB-L Random Variables NBr, p NB-Lr, Usual stochastic orderLikelihood ratio orderConvex orderExpectation order Uniformly more variable order B-L 3,1.5 1 Y~N st lr 5 XY 1E XY - 2 Y~N st 2 XY 2E XY - 3 Y~NB-L3,3.5 - - - E3 XYuv 3 XY 4 Y~NB-L3,4.5 - - - E XYuv 4 XY 5 Y~NB-L3,5.7 E XYuv 5 XY 1 XY - B-L3,2.0 - - 4 X~NB 3,0.8 - - cx 5 XY5 where H is cumulative distribution function of Lindley distribution: z 1z e 1 Hz; 1 , z0 and 0 . 1 1p r1 1 k r1 11 kr1! Pr YkHdt, 1!Hp; p 1 k t 11 0k1 !r1! p ;p r k1!r 1 1p. k ! k So, k r1 11 p1p Rk 1p r1 pp Hp; . r 1 k1 Since k r 11 k1 p1p lim 0 1p r1 ppH; , we have then r1 k1 p k lim Rk0, 1 p1. lidates the result. ose that The part 2) in 2, it is clear that ran- domd Y are not ordered by the usual sto- chastfrom the arguments used in the proof of pa 1, since 0 Thert follows that, for any 0p1, there ex- ists a sufficiently large k such that PrXk PrYk efore, i . This va 1 0p p rt 1) in Theorem 2) Supp Theorem 1.n, from 1 and pa variables X an ic order. Also, rt 1) in Theorem p it follows that Pr s non-increasing and unimodal, hat . The converse part follows by mints. □ hat 2 XkPrYk i uv XY lar argume Suppose t implying t using the si Theorem 3. p . Then, uv 2) cx XY ows from part 2) in Theorem 2, we ha , where 1 1) XY . Proof. 1) Follve uv XY2 p. uv XY and 2) Since 3 2 r1 r EYr X p 11 , pE byed in [4], X with negative binomial—Lindley random variable in usual stochastic order, likelihood ratio order, convex or- der, expectation order and uniformly more variable order and the results are provided in Table 1. Then, we explain that negative binomial random vari- able (X) is smaller than negative binomial—Lindley ran- order implies that XY dom variable (Y) in the usual stochastic E. In addition, if X and Y have respective supp(X) supports supp(X) and supp(Y), such that supp(Y) and the ratio PrX kPrYk is a uni- modal funtion over supp but X and Ye not or- dered in t usual stochast order. Furthermre, if X and Y have a same mean. Thenuv XY implthat cx XY c he (Y) ic ar o ies . 4. Conclusion This paper shws stochast orders comparisof nega- tive binomial random variable with a negative binomial— , exd uniformly more variab compari inomial—Lindley random vanegatiial random vale (X) is smaller than negative binomial—Lindley ram variab (Y) in the usual stochastic order. Its us is that it gives a simple sufficient condition for Xxt, if supp(X) (Y) is tt it implies that the ratio oicn o Lindley random variable by usual stochastic order, like- lihood ratio order, convex orderpectation order an le order. Some advantages of sto- chastic orders son between negative binomial random variable and negative b riable are as follows: If ve binom riab ndo le efulness is smaller than Y in the expectation order. Ne suppha the result of Shakcx Y. Next, We shows some numerical examples of the comparisons between negative binomial random variable PrPr YkXk nimodal function over supp(Y) but X and Y are not ordered in the usual stochasticr. Finally, If X and Y have a same mean, it is known X is smaller than Y in uniformly more variable order im- n converder. This con- cl R is a u orde that plies that X is smaller than Y ix o usion is supported by numerical examples. 5. Acknowledgements We are grateful to the Commission on Higher Education, Ministry of Education, Thailand, for funding support under the Strategic Scholarships Fellowships Frontier esearch Network.
 C. PUDPROMMARAT ET AL. 212 REFERENCES [1] W. Rainer, “Econometric Analysis of Count Data,” 3rd Edition, Springer-Verlag, Berlin, 2000. [2] H. Zamani and N. Ismaal—Lindley Distribution and Its Application,” Journal of Mathematics and Statistics, Vol. 6, No. 1, 2010, pp. 4-9. il, “Negative Binomi doi:10.3844/jmssp.2010.4.9 [3] M. Shaked and J. G. Shanr, “Stochastic Ordthikuma Academic Press, New York, 2006. Stochastic Processes,” Wiley, New York, ers,” [4] S. M. Ross, “ 1983. [5] N. Misra, H. Singh and E. J. Harner, “Stochastic Com- parisons of Poisson and Binomial Random Variables with Their Mixtures,” Statistics and Probability Letters, Vol. 65, No. 4, 2003, pp. 279-290. doi:10.1016/j.spl.2003.07.002 [6] M. Shaked, “On Mixtures from Exponential Families,” Journal of the Roy No. 2, 1980, pp. 192-198. al Statistical Society: Series B, Vol. 42, [7] M. Shaked an“Stochastic Orders and Their ApPress, New York, fe Distributions,” d J. G. Shanthikumar, plications,” Academic 1994. [8] H. Singh, “On Partial Orderings of Li Naval Research Logistics, Vol. 36, No. 1, 1989, pp. 103- 110. doi:10.1002/1520-6750(198902)36:1<103::AID-NAV322 0360108>3.0.CO;2-7 Copyright © 2012 SciRes. OJS
|