 Open Journal of Statistics, 2012, 2, 163-171 http://dx.doi.org/10.4236/ojs.2012.22018 Published Online April 2012 (http://www.SciRP.org/journal/ojs) 163 A Tight Prediction Interval for False Discovery Proportion under Dependence Shulian Shang, Mengling Liu, Yongzhao Shao* Division of Biostatistics, New York University School of Medicine, New York, USA Email: *shaoy01@nyu.edu Received February 1, 2012; revised March 5, 2012; accepted March 16, 2012 ABSTRACT The false discovery proportion (FDP) is a useful measure of abundance of false positives when a large number of hy- potheses are being tested simultaneously. Methods for controlling the expected value of the FDP, namely the false dis- covery rate (FDR), have become widely used. It is highly desired to have an accurate prediction interval for the FDP in such applications. Some degree of dependence among test statistics exists in almost all applications involving multiple testing. Methods for constructing tight prediction intervals for the FDP that take account of dependence among test sta- tistics are of great practical importance. This paper derives a formula for the variance of the FDP and uses it to obtain an upper prediction interval for the FDP, under some semi-parametric assumptions on dependence among test statistics. Simulation studies indicate that the proposed formula-based prediction interval has good coverage probability under commonly assumed weak dependence. The prediction interval is generally more accurate than those obtained from ex- isting methods. In addition, a permutation-based upper prediction interval for the FDP is provided, which can be useful when dependence is strong and the number of tests is not too large. The proposed prediction intervals are illustrated using a prostate cancer dataset. Keywords: Multiple Testing; False Discovery Proportion; False Discovery Rate; Weak Dependence; Correlated Test Statistics; High-Dimensional Data Analysis; Prediction Interval; Upper Prediction Bound; Permutation-Based Method 1. Introduction When a large number of hypotheses are tested simulta- neously, a direct measure of the abundance of false posi- tive findings is the false discovery proportion (FDP), defined as FDP, or 1 V QR 1max ,1RR , where R denotes the total number of rejections, V denotes the number of rejections of true null hypotheses, and . Moti- vated by various genetic and genomic studies and other applications, many useful procedures have been proposed to control the expected value of FDP, namely the false discovery rate (FDR) [1-5]. Indeed, it is well known that controlling FDR has power advantages over the tradi- tional way of controlling family-wise type I error [1,2]. Suppose a study is properly designed to control the FDR at 5%. If such a study is independently repeated many times, the average of the FDPs in these repeated studies can be expected to be no more than 5%. However, for a particular study (without repetition), the FDP is more directly relevant than FDR. Therefore, when a study is designed to control FDR under common designs, it is still very much desirable to assess FDP, e.g. to construct a prediction interval for the FDP. One can also consider designing a study controlling FDP instead of FDR. This approach has been less successful since FDP is a random variable and is less straightforward to control than the FDR. Indeed, researchers have proposed various proce- dures aimed at controlling the FDP [6-13], from which confidence envelopes for the FDP can be obtained si- multaneously for all possible rejection regions. However, confidence envelopes from the existing FDP controlling procedures are often too conservative for predicting a tight range for the FDP. In particular, when weak corre- lations exist among test statistics, methods for construct- ing tight prediction interval for the FDP are still limited. In the multiple testing context, test statistics are often correlated, e.g. in microarray experiments and functional magnetic resonance imaging, correlations arise due to biological, spatial, temporal or technical factors. A major challenge for predicting FDP is to account for unknown correlations between test statistics. It has been shown via numerical studies that when test statistics are correlated, the variability of FDP can increase dramatically [14-17]. This can also be seen from the variance formula derived *Corresponding author. C opyright © 2012 SciRes. OJS
 S. SHANG ET AL. 164 in the next section (Formula (2)). Permutation-based me- thods are often considered in the presence of dependency, e.g. [15]. Permutation-based methods have several limita- tions. For instance, they are not applicable when no group structure (e.g., groups of cases and controls) is present as in some imaging studies [9]. Additionally, if the purpose of testing is to detect differences in means, then a per- mutation-based test can have an inflated Type I error rate by picking up signals due to unequal variances or skew- ness of two distributions [18]. Pan [3] and Xie et al. [19] also pointed out that permutation-based procedures tend to overestimate FDR. Finally, a permutation-based ap- proach is generally very computationally intensive; it often becomes not feasible when the number of tests is large. Other works on FDP have been proposed with efforts to accommodate the correlations among test statistics. For example, Ge et al. [12,20] proposed a formula for the upper prediction bound of the FDP assuming that test statistics under true null hypotheses are independent and also proposed a permutation algorithm to obtain a simul- taneous upper prediction band of the FDP. Under the assumption that p-values are independent or follow a conditional equicorrelated multivariate normal model, Roquain and Villers [21] provided exact calculations for the cumulative distribution function (CDF) and moments of FDP for the step-up and step-down procedures. Ghosal and Roy [22] proposed a nonparametric Bayesian proce- dure to obtain the posterior distribution of FDP under the intraclass or autoregressive correlation structure. In all these studies on FDP under dependence, the correlation among test statistics is either ignored or assumed to fol- low some parametric models. A flexible semiparametric approach to modeling dependency among test statistics has not emerged. In this paper, we first derive an explicit formula for the variance of the FDP under a semiparametric weak de- pendence assumption among the test statistics. The vari- ance formula is easily interpretable and elucidates the effect of correlation on the variability of FDP. Using the variance formula, we obtain an upper prediction interval for the FDP. This approach is semiparametric in nature because only the average of the pairwise Pearson correla- tion between test statistics needs to be estimated. The formula-based prediction interval is easy to evaluate even when testing a vast number of hypotheses where no other methods are computationally feasible. Simulation studies indicate that the formula-based prediction interval has good coverage probabilities under weak to moderate de- pendence. In many situations, as illustrated, the predic- tion interval is quite short (tight) and generally more ac- curate than competitors. In addition, we discuss a per- mutation-based upper prediction interval for FDP which is useful under strong dependence. We illustrate the pro- posed prediction intervals using a prostate cancer data- set. 2. Methods 2.1. Notation Consider testing m hypotheses simultaneously. Rejec- tions are made based on p-values, with a fixed rejection region 0, for some α. Denote the rejection status of the ith test by i RIp i , where pi denotes the p-value of the ith test and m RR is an indicator function. Denote the power of the ith test as 1 – βi. Let V and U be the total number of incorrect and cor- rect rejections, respectively. The total number of rejec- tions or discoveries is 1i i . Let M0 denote the index set of the m0 tests for which null hypotheses are true and M1 the index set of m1 = m – m0 tests for which alternative hypotheses are true. The proportion of true null hypotheses is 0 0 πm m . When test statistics are de- pendent, as in [23], we have 0 0 ,, 00 Var 11 111, ij V ij Mij V Vm mm corr , ij Vij RR 0 ,ij M for , and where 0 ,, 00 =1 ij V ij Mij Vmm is the average correlation coeffi- cient. Similarly, for the correct rejections, 1 1 ,, Var 1 11, ii iM ij Ui ijj ij Mij U corr , ij Uij RR 1 ,M for ij . Denote where 1 1 1 =i iM m . If effect sizes are all equal, i.e. = i for all i, we can obtain a simplified formula 11 Var111 U Um m , where 1 ,, 11 =1 ij U ij Mij Umm . Additionally, let corr , ij UVi j RR 1 M0 jM for i, . De- note the average correlation 10 01 ij UV iM jM UV mm Under some general regularity conditions including weak . Table 1 summarizes the outcomes of m tests and their expected values. 2.2. Formula-Based Prediction Interval 2.2.1. De rivation of Predicti on I n t erval Copyright © 2012 SciRes. OJS
 S. SHANG ET AL. 165 Table 1. Outcome and expected outcome of testing m hypo- theses. Outcome Reject H0 Accept H0 Total H0 is true V m0 – V m0 H1 is true U m1 – U m1 Total R m – R m Expected Outcome Reject H0 Accept H0 Total H0 is true m0α 01m m0 H1 is true 1 1 m 1 m m1 Total 01 1mm 01 1mm m dependence among test statistics, Farcomeni [24] proved that the FDP, V ,0,1 1 QR , as a stochastic process indexed by α, is an asymptotically Gaussian en process (see Theorem 2 of [24]). In particular, for a fixed α, the FDP has an asymptotically normal distribution under weak dependence as discussed in Farcomeni [24]. More specifically, assuming that 0 0π1, when test statistics are independent or weaklyt, depend0 V , 0 U and 0 UV as m, we show tP a Normutiontotically with special mean and variance (see Appendix A (A.1)). No assump- tions about higher-order correlation terms are required. When effect sizes are all equal, explicit forms for the hat FD follows al diasym m strib p ean Q and variance 2 Q of the FDP can be easily ed using the delethod and are given by obtainta m 0 π 00 π1π1 Q , (1) 2 00 2 00 π1π π1π Q 4 11 1 , (2) where 0 0 0 00 π 1 1π 1π π2π1 UU m 1 1V V m (3) and 1 . For a moderate to large sample size n, ragthe averror e Type II e0 . Then 2 000 ππ2π QVUUV cmm (4) 11 where 2 00 4 00 π1 1π π1 π c . From (4), it is evident that when all the test statistics are independent, 2 Q is ly proportional to m; wh dependent, correlations also contribute to the variance. ic inverse en some test statistics are The rejection threshold α in multiple testing is typally less than 0.05 and thus ω is small, making the last two terms of (4) small. The average correlation among true null test statistics, which is represented by V , can have a large influence on the variance of FDP. When all the parameters are known, a prediction in- terval could be derived based on the asympic distribu- tion of FDP. We shall discuss the estimat tot ion of parame- ters in details next section. In multiple testing we are primarily concerned about high FDPs so an upper predic- tion interval is of interest. A 1001 % upper predic- tion interval for FDP is given by 0,QQ z , where z is the 1001 th quantile ostandard normal distribution. f the ice, the distrib FDP under dependenc ch as the log-transformation to be practi- ca With finite sample size in practution of e is often skewed, suggesting trans- formations su lly useful. Moreover, by the delta method (see Appen- dix A for more details), when the FDP is asymptotically normal, Y = log(FDP) is also asymptotically normal, i.e., 2 ~, YY YN asymptotically, where formulas for Y and 2 Y are derived in Appendix A (A.2). Thus it is not surprising that Y = log(FDP) is closer to normal than the FDP itself, part ima icularly under weak dependence. The ap- proxte mean and variance of Y are: 0 00 π loglog π1π YQ 1 (5) 2 0 2 2 00 0 1π11 ππ 1π1 Y where (6) is in (3). Applying the expone mation, a ntial transfor- 1001 % upper prediction interval for the FDP can be constructed as 0, expz . tion sed prediction interv YY 2.2.2. Estima To calculate the formula-baal 0, expz YY , we need to estimate necessary pa- rameters in Y and Y first. We adopt the estimator 0 π proposed for by Storey [2]: 0 ˆ π 1 i m #p for 0,1 the choice of 0.5 with . We use t he odmeth of moment to estimate . Because 01 1ER mm , plugging in the estimate of he o of rej v 0 πand tbserved total numberections R, we hae 0 ˆ π ˆ1Rm 0 ˆ 1πm The resulting estimator ˆQ is . Copyright © 2012 SciRes. OJS
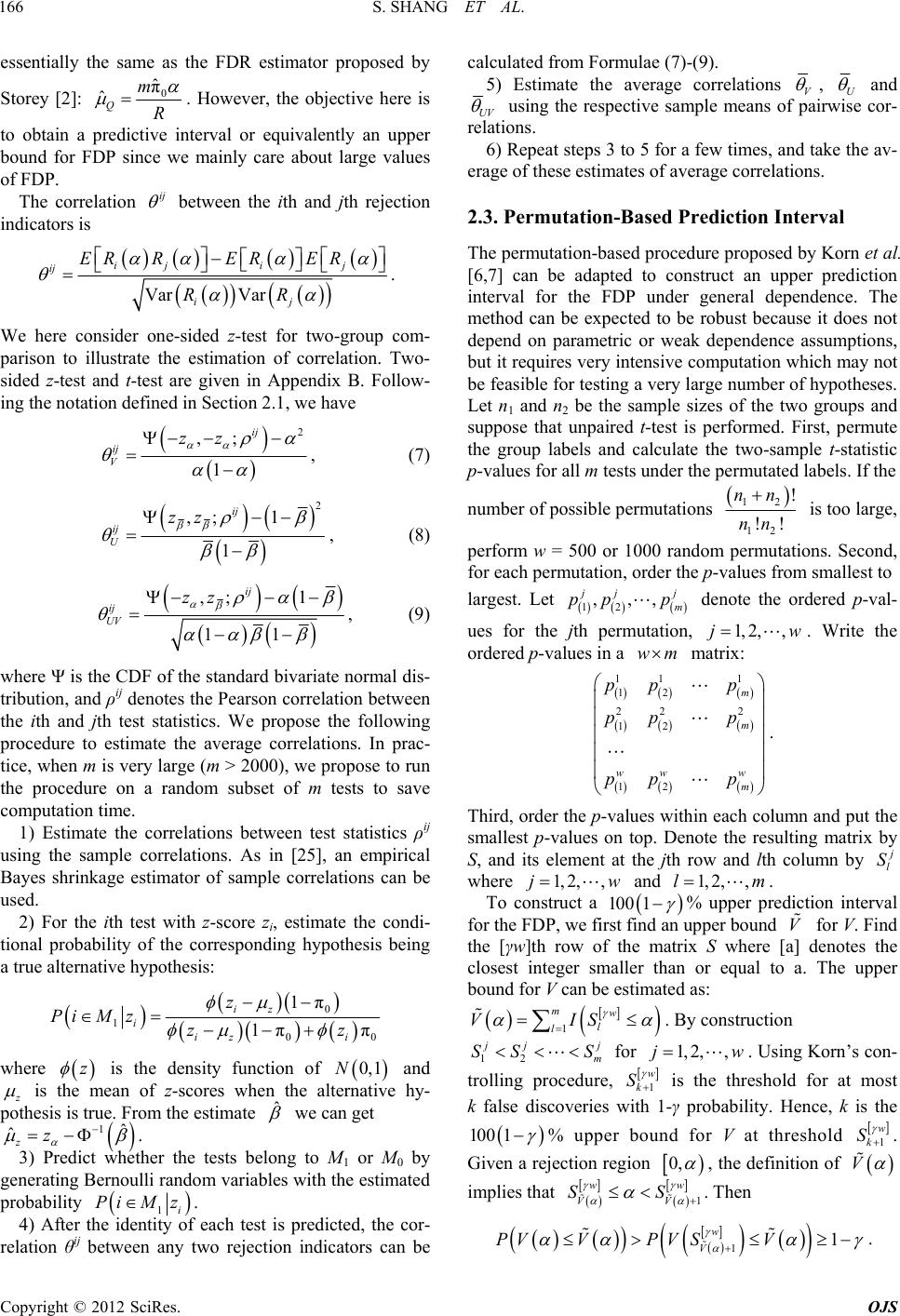 S. SHANG ET AL. 166 essentially the same as the FDR estimator proposed by Storey [2]: 0 ˆ π ˆQ m R er ce ij . However, the objective he is to obtain a predictive interval or equivalently an upper bound for FDP sinwe mainly care about large values of FDP. The correlation between the ith and jth rejection indicators is Var Var iji j ij RR ij ER RERER We here consider one-sided z-test for two-group com- parison to illustrate the estimation of correlation. Two- sided z-test and t-test are given in Appendix B. Follow- . ing the notation defined in Section 2.1, we have 2 ,; 1 ij ij V zz , (7) 2 1 ij ,; 1 ij U zz , (8) ,; ij ij UV zz 1 11 where Ψ is the CDF of the standard bivariate normal dis- tribution, and ρij denotes the Pearson correlati the ith and jth test statistics. We propose the following , (9) on between procedure to estimate the average correlations. In prac- tice, when m is very large (m > 2000), we propose to run the procedure on a random subset of m tests to save computation time. 1) Estimate the correlations between test statistics ρij using the sample correlations. As in [25], an empirical Bayes shrinkage estimator of sample correlations can be used. 2) For the ith test with z-score zi, estimate the condi- tional probability of the corresponding hypothesis being a true alternative hypothesis: 0 1 00 1π 1ππ iz i iz i z PiM zzz where z is the density of 0,1N and function is the mean of z-scoreshe alternative hy- pothesis is true. Frome esti when t mate ˆ th we can get 1ˆ ˆzz . 3) Predict whether the tests belong to M1 or M0 by generating Bernoulli random variables with the estimated probability i vercorrelations 1 PiM z. ntity4) After the ide of each test is predicted, the cor- ation θij between any two rejection indicators can be calculated from Formulae (7)-(9). 5) Estimate the aage rel , UV and UV using the respective sample means of pairwise cor- relations. 6) Repeat steps 3 to 5 for a few times, and take the av- ern tation-Based Prediction Interval iction The not age of these estimates of average correlatios. 2.3. Permu The permutation-based procedure proposed by Korn et al. [6,7] can be adapted to construct an upper pred interval for the FDP under general dependence. method can be expected to be robust because it does depend on parametric or weak dependence assumptions, but it requires very intensive computation which may not be feasible for testing a very large number of hypotheses. Let n1 and n2 be the sample sizes of the two groups and suppose that unpaired t-test is performed. First, permute the group labels and calculate the two-sample t-statistic p-values for all m tests under the permutated labels. If the number of possible permutations 12 12 ! !! nn nn is too large, perform w = 500 or 1000 random permutations. Second, for each permutation, order the p-vllest to alues from sma largest. Let 12 ,,, jj m pp p denordered p-val- te the o ues for the jth permutation, 1, 2,,jw. Write the ordered p-values in a wm matrix: 11 12 2 12 m www m pp p pp p . Third, order the p-values within each comn and put the smallest p-values on top. Denote the reslting matrix by S, and its element at the jth row and lth column by 1 m p 22 12 pp lu u l S where 1, 2,,jw and 1, 2,,lm. To construct a 1001 % upper prediction interval for the FDP, we first find an upper bound V for V. Find the [γw]th row of the matrix S where [a] denotes closest ller than o the integer smar equal to a. The upper bo estimatedund for V can be as: 1 mw l l VIS . By construction 12 jj m SSS for 1, 2,,jw . Using Korn’s con- trolling procedure, 1 w k S is the threshold for at most k false discoveries with 1ce, k is the -γ probability. Hen 1001% uppund for V er boat threshold 1 w k S . Given a rejection region 0, , the definition of V implies that 1 ww VV SS . Then w S 11PV VPV V V . Copyright © 2012 SciRes. OJS
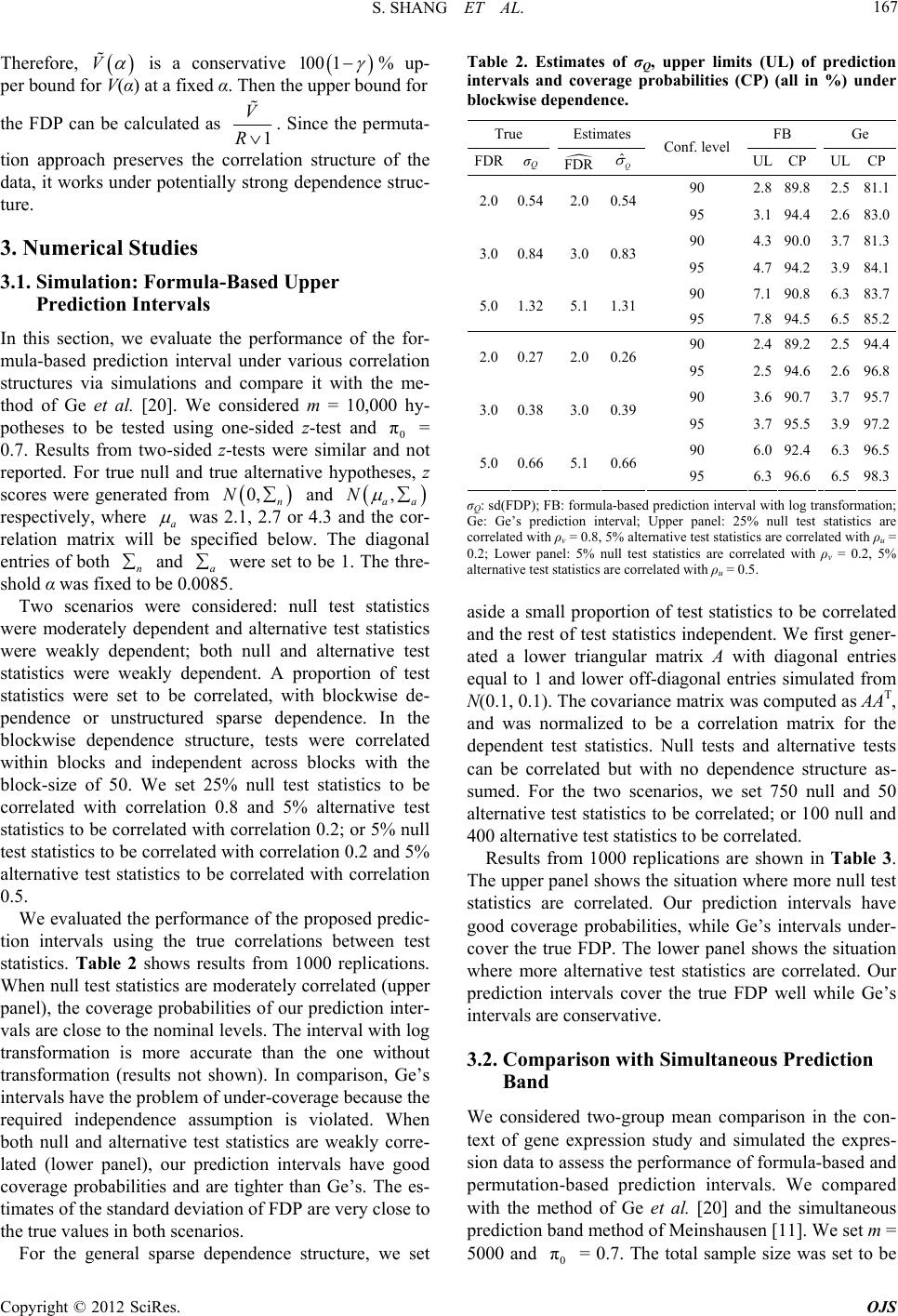 S. SHANG ET AL. 167 VTherefore, is a c 0 1onservative 10 % up- r V(α) at a er bound for the FDP can be calculated as per bound fo the uppfixed α. Then 1 V R . Since the perma- ch pre ediction Intervals the performance of the for- orrelation the me- thod considered m = 10,000 hy- ut tion approaserves the correlation structure of the data, it works under potentially strong dependence struc- ture. 3. Numerical Studies 3.1. Simulation: Formula-Based Upper Pr In this section, we evaluate mula-based prediction interval under various c structures via simulations and compare it with of Ge et al. [20]. We potheses to be tested using one-sided z-test and 0 π = 0.7. Results from two-sided z-tests were similar and not reported. For true null and true alternative hypotheses, z scores were generated from 0, n N and , aa N respectively, where a was 2.1, 2.7 or 4.3 and the cor- relation matrix will be specified below. The diagonal entries of both n and a were set to be 1. The thre- shold α was fixed to be 0.0085 Two scenarios were considered: null test statistics were moderately dependent and alternative test statistics were weakly de endent; both null and alternative test statistics were weakly dependent. A proportion of test st p FB Ge . atistics were set to be correlated, with blockwise de- pendence or unstructured sparse dependence. In the blockwise dependence structure, tests were correlated within blocks and independent across blocks with the block-size of 50. We set 25% null test statistics to be correlated with correlation 0.8 and 5% alternative test statistics to be correlated with correlation 0.2; or 5% null test statistics to be correlated with correlation 0.2 and 5% alternative test statistics to be correlated with correlation 0.5. We evaluated the performance of the proposed predic- tion intervals using the true correlations between test statistics. Table 2 shows results from 1000 replications. When null test statistics are moderately correlated (upper panel), the coverage probabilities of our prediction inter- vals are close to the nominal levels. The interval with log transformation is more accurate than the one without transformation (results not shown). In comparison, Ge’s intervals have the problem of under-coverage because the required independence assumption is violated. When both null and alternative test statistics are weakly corre- lated (lower panel), our prediction intervals have good coverage probabilities and are tighter than Ge’s. The es- timates of the standard deviation of FDP are very close to the true values in both scenarios. For the general sparse dependence structure, we set Table 2. Estimates of σQ, upper limits (UL) of prediction intervals and coverage probabilities (CP) (all in %) under blockwise dependence . True Estimates FDR σQ FDR Q ˆ Conf. level UL CP ULCP 90 2.8 89.82.581.1 2.0 0.542.00.5495 3.1 94.42.683.0 90 4.3 .03.7.3 3. 90 81 0 0.843.00.8395 4.7 94.23.984.1 90 7.1 90.86.383.7 5.0 1.325.11.3195 7.8 94.56.585.2 90 2.4 89.22.594.4 2.0 0.272.00.2695 2.5 94.62.696.8 90 3.6 90.73.795.7 3.0 0.383.00.3995 3.7 95.53.997.2 90 6.0 92.46.396.5 5.0 0.665.10.6695 6.3 96.66.598.3 σQ: sd(FDP); FB: formula-based predicintervaram Gedictin Upper l: 25% ll tice correlated with ρv = 0.8, 5% alternativetatistiati 0.2; Lower panel: 5% null test statistire corρ. alti stas alated ρu = 0. s simulated from N(0.1, 0.1). The covariance matrix was computed as AAT, e situation w y and simulated the expres- d d withthod of Ge et al. [20] and the simultaneous tion l with log tnsforation; e: G’s preion terval;pane test s nu cs are est sta correl tist ed w s ar th ρu = cs a with related with 5. v = 02, 5% ternave testtisticre corre aside a small proportion of test statistics to be correlated and the rest of test statistics independent. We first gener- ated a lower triangular matrix A with diagonal entries equal to 1 and lower off-diagonal entrie and was normalized to be a correlation matrix for the dependent test statistics. Null tests and alternative tests can be correlated but with no dependence structure as- sumed. For the two scenarios, we set 750 null and 50 alternative test statistics to be correlated; or 100 null and 400 alternative test statistics to be correlated. Results from 1000 replications are shown in Table 3. The upper panel shows the situation where more null test statistics are correlated. Our prediction intervals have good coverage probabilities, while Ge’s intervals under- cover the true FDP. The lower panel shows th here more alternative test statistics are correlated. Our prediction intervals cover the true FDP well while Ge’s intervals are conservative. 3.2. Comparison with Simultaneous Prediction Band We considered two-group mean comparison in the con- text of gene expression stud sion data to assess the performance of formula-based an permutation-based prediction intervals. We compare the me prediction band method of Meinshausen [11]. We set m = 5000 and 0 π = 0.7. The total sample size was set to be Copyright © 2012 SciRes. OJS
 S. SHANG ET AL. Copyright © 2012 SciRes. OJS 168 Table 3. Estimates of σQ, upper limits (UL) of prediction intervals and coverage probabilities (CP) (all in %) under sparse dependenc e. True Estimates FB Ge FDR σ Q FDR Q ˆ Conf. level UL CP ULCP 90 2.8 92.5 2.587.8 2.0 0.54 2.0 0.53 95 3.1 94.7 2.689.6 90 4.1 .0 3.6.6 3.0 0.79 90 88 3.0 0.7995 4.4 93.9 3.890.3 90 6.8 90.6 6.288.1 5.0 1.24 5.1 1.22 95 7.4 93.8 6.589.7 90 2.4 90.7 2.595.2 2.0 0.26 2.0 0.27 95 2.5 95.1 2.697.3 90 3.6 91.0 3.793.8 3.0 0.43 3.0 0.42 95 3.8 95.0 3.896.8 90 6.1 91.2 6.397.0 5.0 0.70 5.1 0.71 95 6.4 95.4 6.598.6 σQ: sd(FDP); FB: formula-based predictntervalm; G’rvr pane50 (11%utice ted with ion i l: 7 with ) n log tra ll test sta nsfor tis ation s are: Ge rrela s prediction inteal; Uppe co v = 0.33, 50 (1.lternasi rrelated with 7%) ative test tatistcs are co u = 0.16, and uv 096; L:) t rreith = 0.ower panel 100 (1.4% null tesstatistics are colated wv = 0.19, 4t s i 00 (13%) al ernative test statistic are correlated w th u = 0.13, anduv g p 0.6. The diagonn and a were 1. es to be correlated with correlation to be correl anthr ran- do The formula-based pr ur for- m l Data Example measured 13,935 mRNA ymphoblastoid cell lines ge FB Per Ge MN = 0.05. 100, 150 or 200 and equally divided between two groups. The data wereenerated from , nn N or , aa N for the two gros, where μn = 0 and μa = u al entries of both Blockwise correlation structure was used and block-size was 50. We set 20% null gen 0.8 within block, and 3.3% alternative genes ated with correlation 0.2 within block. One-sided t-test was performedd the eshold α was fixed at 0.01. For calculating the formula-based in- terval, correlations between test statistics were estimated from correlations between gene expression levels. Pair- wise Peason correlations were calculated from 500 mly chosen genes across all the subjects, after sub- tracting off each gene’s mean within each group as in [26]. Sample correlations were then shrunk using the Table 4. Comparison of the upper limits (UL) and covera True Estimates empirical Bayes method [25] to correct the well known inflation of variability in correlation estimates. The co- relations θ between rejection status were then calculated using the procedure in Section 2.2.2, repeating the pro- cedure for 3 times. For calculating the permutation-based prediction intervals, a total of 500 randomly chosen per- mutations of the groups were used. Coverage probabilities of four prediction intervals are given in Table 4 (200 replications). ediction intervals cover the true FDP well and are slightly conservative. Since the sample correlations are still over-dispersed after shrinkage, the variance of FDP is over-estimated. The permutetion-based interval is more conservative than the formula-based ones. In contrast, Ge’s prediction interval is too liberal. The simultaneous prediction bands are about twice as high as the for- mula-based intervals. Hence it is not very useful when point-wise intervals are needed. In terms of computa- tional efficiency, when the sample size was 100, the cen- tral processor unit (CPU) time for calculating the for- mula-based and permutation-based prediction intervals in one run of simulation was 81 seconds and 20 minutes respectively, on a 2.66 GHz processor with 4 GB of memory. The permutation-based approach will become more computationally intensive as m gets larger. We have also varied m, rejection region and correla- tion structures. When the dependence is weak, o ula-based prediction interval works well in various scenarios. It is the tightest one among all intervals that we study. 3.3. A Rea The study in Wang et al. [27] gene expression levels in 125 l derived from 62 aggressive and 63 nonaggressive pros- tate cancer patients. The purpose is to identify candidate genes whose expression levels are associated with ag- gressive phenotype of prostate cancer. Two sample two- probabilities (CP) of four prediction intervals (all in %). FDR σQ FDR ˆQ UL CP ULCP UL CP UL CP n Conf. level 90 5.392.0 6.595.5 4.0 85.0 10.0 100.0 3.2 1.22 3.31 100 6.2 7.6 4.2 86.0 11.2 90 100.0 2.5 0.90 2.5 1.02 150 2.0 0.74 2.0 0.87 200 100.0 .1 195 94.5 99.0 100.0 4.393.0 5.397.5 3.3 86.5 8.2 95 5.095.0 6.298.0 3.4 89.5 9.1 100.0 90 4.094.0 5.099.0 3.1 86.0 7.3 100.0 95 4.696.5 5.83.2 88.5 8.0 100.0 σQ: sd(FDP); FB: formula-based prediction interval with log transation; Per: peutatiosed upr prebounepred inte: simultaneous prediction bands using Meinshausen’s permutation algorithm [11]; n: sample size. formrmn-bapediction ds; G: Ge’s ictionrval; MN
 S. SHANG ET AL. 169 sid tesre prmedeane, andpro- portion of null htheses was estimted to be 0 ˆ π the ed tts we true erfo ypo for ch ge the a = 0.60. The FDR controlling procedure in [2] was used to ontrol FDR at 3% or 5%, rejecting 1708 or 2208 hy- c potheses respectively. Sample correlations were esti- mated from 2000 randomly sampled genes repeating estimation procedure for 3 times. The correlation is weak and the average of estimated ρV is very close to 0. The estimated V is 0.0059 at α = 0.006. The formula-based upper prediction intervals with log transformation (FB), permutation-based intervals (Per) and simultaneous pre- diction bands (MN) are shown in Tabl e 5. When FDR is controlled at 5%, with 90% probability the actual FDP is as high as 12.7% (FB). Hence with the correlations in this dataset, FDP could far exceed its mean with high probability. Since the purpose of the study is to identify target genes for a large-scale validation study, a smaller rejection region may be more appropriate to avoid exces- sive false positives. The permutation-based approach gives more conservative intervals than the formula-based one. The simultaneous prediction bands are high and too con- servative for fixed rejection regions. 4. Discussion It is feasible to construct a tight prediction interval for the FDP without specifying a parametric correlati tatistics. When the dependence is iction interval for the FDP based on the on wstructure for test s we derived a pred eak, variance formula which takes correlations into considera- tion. This formula-based approach is computationally efficient even when the number of tests is very large. The prediction interval could help investigators decide what rejection regions are suitable for a particular study to control FDR. If the upper limit of prediction interval is unacceptably high, then selecting a smaller rejection re- gion might be more appropriate. We also discussed a permutation procedure which can be employed to find a prediction interval for the FDP without assuming weak dependence. This approach can be computationally quite Table 5. Comparison of upper limits (UL) of prediction intervals for the prostate cancer data (all in %). Method FDR ˆQ 90% UL 95% UL 3.0 (2.72) 9.6 13.3 FB 5.0 (3.63) 12.7 16.5 3.0 MN 10.1 13.4 Per 5.0 15.5 20.2 3.0 22.2 5.0 29.5 26.8 36.2 FB: foa-based predictirvals with log tormation; Pe tation-based prediction intMN: simultanrediction sing Meinshausen’s permutation algorithm. esially tumf tis l thank the reviewers for their careful read of our paphis research was partia ported by a Stony ld-Herbert Fation gand NCES tes,” Journal of the Royal Statistical Society Series B- Statistical Methodology, Vol. 64, No. 3, 2002, pp. 479- 498. doi:10.11 rmulon inte ervals; ransf eous p r: pe bands u rmu- intensive, pec whenhe nber oests arge. 5. Acknowledgements We would like to ing er. Tlly sup- Wooundrant the NYU Cancer Center Supporting Grant (2P30 CA16087- 23), and by the NYU NIEHS Center Grant (5P30 ES00260- 44). REFERE [1] Y. Benjamini and Y. Hochberg, “Controlling the False Discovery Rate—A Practical and Powerful Approach to Multiple Testing,” Journal of the Royal Statistical Society Series B-Methodological, Vol. 57, No. 1, 1995, pp. 289- 300. [2] J. D. Storey, “A Direct Approach to False Discovery Ra 11/1467-9868.00346 e Use of Permutation[3] W. Pan, “On th in and the Perfor- mance of a Class of Nonparametric Methods to Detect Differential Gene Expression,” Bioinformatics, Vol. 19, No. 11, 2003, pp. 1333-1340. doi:10.1093/bioinformatics/btg167 [4] J. D. Storey, J. E. Taylor and D. Siegmund, “Strong Con- gy, Vol. 66, No. 1, trol, Conservative Point Estimation and Simultaneous Conservative Consistency of False Discovery Rates: A Unified Approach,” Journal of the Royal Statistical So- ciety Series B-Statistical Methodolo 2004, pp. 187-205. doi:10.1111/j.1467-9868.2004.00439.x [5] X. D. Zhang, P. F. Kuan, M. Ferrer, X. Shu, Y. C. Liu, A. T. Gates, P. Kunapuli, E. M. S et al., “Hit Selection with False Di tec, M. Xu, S. D. Marine, scovery Rate Control in Genome-Scale Rnai Screens,” Nucleic Acids Research, Vol. 36, No. 14, 2008, pp. 4667-4679. doi:10.1093/nar/gkn435 [6] E. L. Korn, J. F. Troendle, L. M. McShane and R. Simon, “Controlling the Number of False Discoveries: Appli- cation to High-Dime Statistical Planning and Inference, Vol. nsional Genomic Data,” Journal of 124, No. 2, 2004, pp. 379-398. doi:10.1016/S0378-3758(03)00211-8 [7] E. L. Korn, M. C. Li, L. M. McShane and R. Simon, “An Investigation of Two Multivariate Permutation Methods for Controlling the False Discovery Proportion,” Statis- tics in Medicine, Vol. 26, No. 24, 2007, pp. 4428-4440. doi:10.1002/sim.2865 [8] C. R. Genovese and L. Wasserman, “A Stochastic Process Approach to False Discovery Control,” Annals of Sta- tistics, Vol. 32, No. 3, 2004, pp. 1035-1061. doi:10.1214/009053604000000283 [9] C. R. Genovese and L. Wasserman, “Exceedance Control of the False Discovery Proportion,” Journal of the Ameri- can Statistical Association, Vol. 101, No. 476, 2006, pp. 1408-1417. doi:10.1198/016214506000000339 Copyright © 2012 SciRes. OJS
 S. SHANG ET AL. 170 [10] M. J. van der Laan, S. Dudoit and K. S. Pollard, “Aug- mentation Procedures for Control of the Generalized Family-Wise Error Rate and Tail Probabilities for the Proportion of False Positives,” Statistical Applications in Genetics and Molecular Biology, Vol. 3, No. 1, 2004, Article 15. [11] N. Meinshausen, “False Discovery Control for Multiple Tests of Association under General Dependence,” Scandi- navian Journal of Statistics, Vol. 33, No. 2, 2006, pp. 227-237. doi:10.1111/j.1467-9469.2005.00488.x [12] Y. C. Ge, S. C. Sealfon and T. P. Speed, “Multiple Testing and Its Applications to Microarrays,” Statistical Methods in Medical Research, Vol. 18, No. 6, 2009, pp. 543-563. doi:10.1177/0962280209351899 [13] A. Farcomeni, “Generalized Augmentation to Control the False Discovery Exceedance in Multiple Testing,” Scan- isc o - dence,” Bioin- dinavian Journal of Statistics, Vol. 36, No. 3, 2009, pp. 501-517. [14] Q. Yang, J. Cui, I. Chazaro, L. A. Cupples and S. Demissie, “Power and Type I Error Rate of False D very Rate Approaches in Genome-Wide Association Stu- die,” BMC Genetics, Vol. 6, Suppl. 1, 2005. [15] Y. Pawitan, S. Calza and A. Ploner, “Estimation of False Discovery Proportion under General Depen formatics, Vol. 22, No. 24, 2006, pp. 3025-3031. doi:10.1093/bioinformatics/btl527 [16] R. Heller, “Correlated Z-Values and the Accuracy of Large-Scale Statistical Estimates Comment,” Journal of the American Statistical Association, Vol. 105, No. 491, 2010, pp. 1057-1059. doi:10.1198/jasa.2010.tm10240 [17] A. Schwartzman and X. H. Lin, “The Effect of Correlation in False Discovery Rate Estimation,” Biometrika, Vol. 98, No. 1, 2011, pp. 199-214. doi:10.1093/biomet/asq075 [18] Y. F. Huang, H. Y. Xu, V. Calian and J. C. Hsu, “To Permute or Not to Permute,” Bioinformatics, Vol. 2 18, 2006, pp. 2244-2248. 2, No. doi:10.1093/bioinformatics/btl383 [19] Y. Xie, W. Pan and A. B. Khodursky, “A Note on Using Permutation-Based False Discovery Rate Estimates to Compare Different Analysis Methods for Microarray Data,” Bioinformatics, Vol. 4288. 21, No. 23, 2005, pp. 4280- ti685doi:10.1093/bioinformatics/b Least Favorable [20] Y. C. Ge and X. Li, “Control of the False Discovery Proportion for Independently Tested Null Hypotheses,” Journal of Probability and Statistics, 2012, in Press. [21] E. Roquain and F. Villers, “Exact Calculations for False Discovery Proportion with Application to Configurations,” Annals of Statistics, Vol. 39, No. 1, 2011, pp. 584-612. doi:10.1214/10-AOS847 [22] S. Ghosal and A. Roy, “Predicting False Discovery Proportion under Dependence,” Journal of the American Statistical Association, Vol. 106, No. 495, 2011, pp. 1208- 1218. doi:10.1198/jasa.2011.tm10488 [23] Y. Shao and C. H. Tseng, “Sample Size Calculation with Dependence Adjustment for FDR-Control in Microarray Studies,” Statistics in Medicine, Vol. 26, No. 23, 2007, pp. 4219-4237. doi:10.1002/sim.2862 [24] A. Farcomeni, “Some Results on the Control of the False Discovery Rate under Dependence,” Scandinavian Jour- nal of Statistics, Vol. 34, No. 2, 2007, pp. 275-297. doi:10.1111/j.1467-9469.2006.00530.x [25] B. Efron, “Empirical Bayes Estimates for Large-Scale Prediction Problems,” Journal of the American Statistical Association, Vol. 104, No. 487, 2009, pp. 1015-1028. doi:10.1198/jasa.2009.tm08523 [26] B. Efron, “Correlation and Large-Scale Simultaneous Sig- nificance Testing,” Journal of the American Statistical Association, Vol. 102, No. 477, 2007, pp. 93-103. doi:10.1198/016214506000001211 [27] L. Wang, H. Tang, V. Thayanithy, S. Subramanian, A. L. arch, 7. Oberg, J. M. Cunningham, J. R. Cerhan, C. J. Steer and S. N. Thibodeau, “Gene Networks and MicroRNAs Impli- cated in Aggressive Prostate Cancer,” Cancer Rese Vol. 69, No. 24, 2009, pp. 9490-949 doi:10.1158/0008-5472.CAN-09-2183 Appendix A. Asymptotic Distribution of e FDP .1. Under the general weak dependence assumptions e FDP is asymptotically normal (e.g. Theorem 2 of at that asymptotically normal. In particular, we also [23], when test statistics under H0 are weakly dependent and th 0 V as m , the asymptotic normality im- plies A th 00 0 11 ~π,π1π V VN mmm . Farcomeni [24]). Thus in this appendix, we assume th some weak dependence assumption is satisfied so the FDP is assume approximate joint normality of V and R. As in Similarly, when test statistics under H1 are weakly de- pendent and 0 U as m, asymptotic normality implies Copyright © 2012 SciRes. OJS
 S. SHANG ET AL. 171 00 ~1π1, 1π UN 0 11 1π U m m 1 m . addition In, if 0 UV as m, then 01 Co1 10 UV mm as ptotically uncorrelated. al distribution, for R > V > 0, FDP has an approximate Normal distribution. v ,UV m, so U and V are asym When V and R have a joint Norm For large m, 1 2 PFD VVV RR Z V RZ , w here V , 2 V , , 2 are the asymptotic mean and v of V and ariance R, respectively. 12 , Z are jointly Normally distributed with mean 0, variance 1 and correlation ,VR . Then by Taylor expansion, 1 2 12 2 1 1 VV V RR RR RR VV VV 1 V 2 2 1 1 RR RR R RRR VV RRR RR Z ZZ Z Z Z Z Z Therefore, FDP has an approximate Normal distbu- tion. Assume that effect sizes are all equal. The variance of FDP can be derived using the delta method. ri 2 222 243 12Cov, VV QVR RR R VR where is in (3). A.2. By the delta method, 2 00 4 00 π1π11 π1π1 2 2 0, Q Q N log FDPlogQ in distribun. The Y = log(FDP) are: tio mean and variance of 0 00 π 1π1 loglog π YQ 2 0 2 2 1π11 Y 00 0 ππ 1π1 where is in (3). Appendix B. Correlation Formulas for θij For a two sample two-sided z-test, the commonower is p 22 2 11 izz Pz zzz . t R, ˆ From he observed can be estimated as in Sec- tion 2.2.2, and then ˆ is estimated from the ab equation. For 0 ,ij M ove , the correlation is 2 1 ij V C 1 , where 122 222 2,; ,; ij ij zzzzz .C For ,ij M 1 , 2 21 1 C ij U , where 222 22 222 ,; ,; 2,;, ij ij ij Czz zz zzz 22 zz and 22 zz her situa- tions, the correlation is . For ot 31 11 ij UV C , where ;, ;. ij zz 322 222 222 22 ,; ,; , ij ij ij Czzzzz zzz ed, we could convert the tics to z-scores by a bijective quantile transformation as in [26]. If t-tests are performt statis- 1 2ini zGt , 1,2,,im, where Gn–2 is the CDF of t distribution with n – 2 degrees of freedom. Then all the previous procedures apply. Copyright © 2012 SciRes. OJS
|