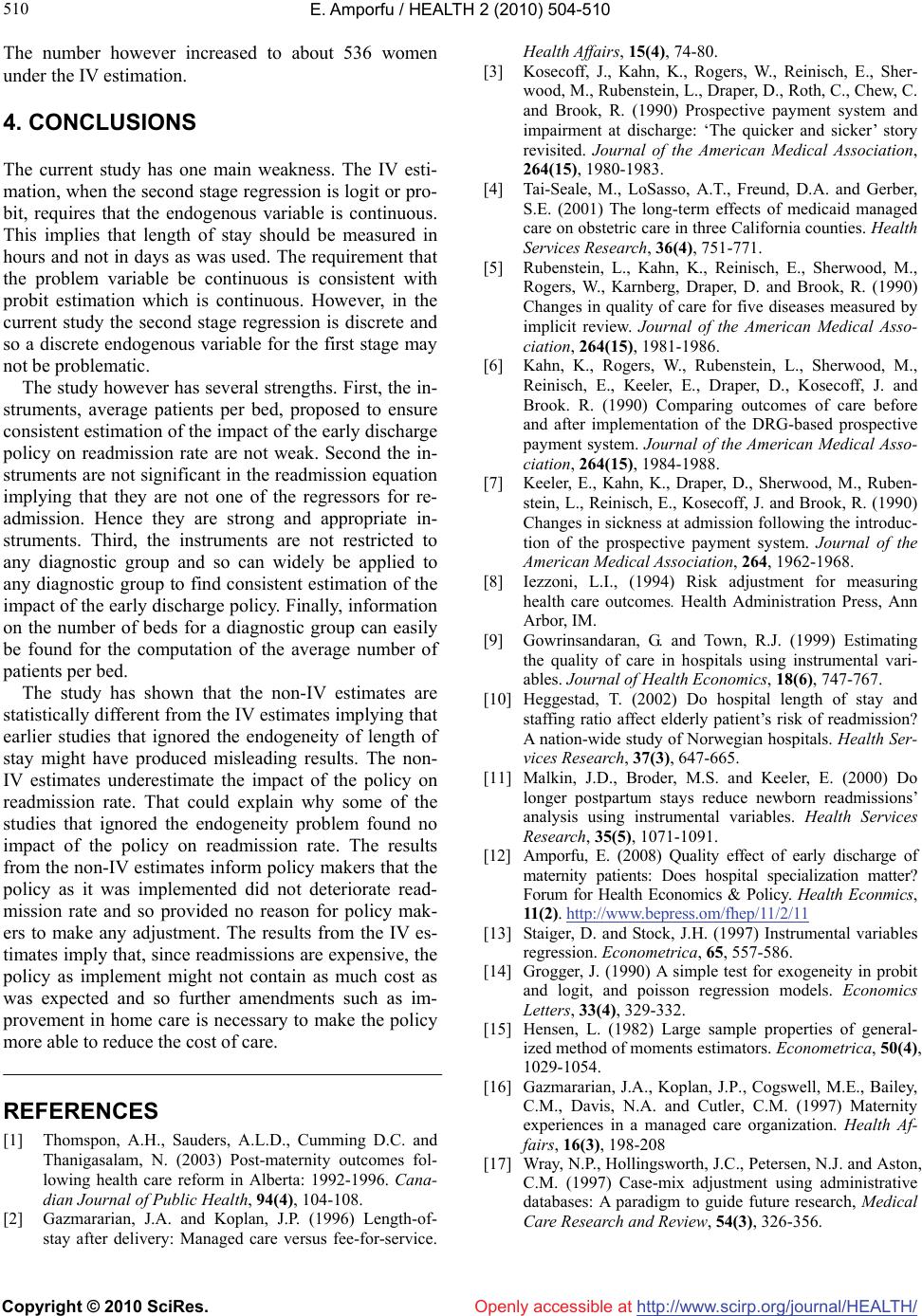
E. Amporfu / HEALTH 2 (2010) 504-510
Copyright © 2010 SciRes. http://www.scirp.org/journal/HEALTH/
510
The number however increased to about 536 women
under the IV estimation.
Openly accessible at
4. CONCLUSIONS
The current study has one main weakness. The IV esti-
mation, when the second stage regression is logit or pro-
bit, requires that the endogenous variable is continuous.
This implies that length of stay should be measured in
hours and not in days as was used. The requirement that
the problem variable be continuous is consistent with
probit estimation which is continuous. However, in the
current study the second stage regression is discrete and
so a discrete endogenous variable for the first stage may
not be problematic.
The study however has several strengths. First, the in-
struments, average patients per bed, proposed to ensure
consistent estimation of the impact of the early discharge
policy on readmission rate are not weak. Second the in-
struments are not significant in the readmission equation
implying that they are not one of the regressors for re-
admission. Hence they are strong and appropriate in-
struments. Third, the instruments are not restricted to
any diagnostic group and so can widely be applied to
any diagnostic group to find consistent estimation of the
impact of the early discharge policy. Finally, information
on the number of beds for a diagnostic group can easily
be found for the computation of the average number of
patients per bed.
The study has shown that the non-IV estimates are
statistically different from the IV estimates implying that
earlier studies that ignored the endogeneity of length of
stay might have produced misleading results. The non-
IV estimates underestimate the impact of the policy on
readmission rate. That could explain why some of the
studies that ignored the endogeneity problem found no
impact of the policy on readmission rate. The results
from the non-IV estimates inform policy makers that the
policy as it was implemented did not deteriorate read-
mission rate and so provided no reason for policy mak-
ers to make any adjustment. The results from the IV es-
timates imply that, since readmissions are expensive, the
policy as implement might not contain as much cost as
was expected and so further amendments such as im-
provement in home care is necessary to make the policy
more able to reduce the cost of care.
REFERENCES
[1] Thomspon, A.H., Sauders, A.L.D., Cumming D.C. and
Thanigasalam, N. (2003) Post-maternity outcomes fol-
lowing health care reform in Alberta: 1992-1996. Cana-
dian Journal of Public Health, 94(4), 104-108.
[2] Gazmararian, J.A. and Koplan, J.P. (1996) Length-of-
stay after delivery: Managed care versus fee-for-service.
Health Affairs, 15(4), 74-80.
[3] Kosecoff, J., Kahn, K., Rogers, W., Reinisch, E., Sher-
wood, M., Rubenstein, L., Draper, D., Roth, C., Chew, C.
and Brook, R. (1990) Prospective payment system and
impairment at discharge: ‘The quicker and sicker’ story
revisited. Journal of the American Medical Association,
264(15), 1980-1983.
[4] Tai-Seale, M., LoSasso, A.T., Freund, D.A. and Gerber,
S.E. (2001) The long-term effects of medicaid managed
care on obstetric care in three California counties. Health
Services Research, 36(4), 751-771.
[5] Rubenstein, L., Kahn, K., Reinisch, E., Sherwood, M.,
Rogers, W., Karnberg, Draper, D. and Brook, R. (1990)
Changes in quality of care for five diseases measured by
implicit review. Journal of the American Medical Asso-
ciation, 264(15), 1981-1986.
[6] Kahn, K., Rogers, W., Rubenstein, L., Sherwood, M.,
Reinisch, E., Keeler, E., Draper, D., Kosecoff, J. and
Brook. R. (1990) Comparing outcomes of care before
and after implementation of the DRG-based prospective
payment system. Journal of the American Medical Asso-
ciation, 264(15), 1984-1988.
[7] Keeler, E., Kahn, K., Draper, D., Sherwood, M., Ruben-
stein, L., Reinisch, E., Kosecoff, J. and Brook, R. (1990)
Changes in sickness at admission following the introduc-
tion of the prospective payment system. Journal of the
American Medical Association, 264, 1962-1968.
[8] Iezzoni, L.I., (1994) Risk adjustment for measuring
health care outcomes. Health Administration Press, Ann
Arbor, IM.
[9] Gowrinsandaran, G. and Town, R.J. (1999) Estimating
the quality of care in hospitals using instrumental vari-
ables. Journal of Health Economics, 18(6), 747-767.
[10] Heggestad, T. (2002) Do hospital length of stay and
staffing ratio affect elderly patient’s risk of readmission?
A nation-wide study of Norwegian hospitals. Health Ser-
vices Research, 37(3), 647-665.
[11] Malkin, J.D., Broder, M.S. and Keeler, E. (2000) Do
longer postpartum stays reduce newborn readmissions’
analysis using instrumental variables. Health Services
Research, 35(5), 1071-1091.
[12] Amporfu, E. (2008) Quality effect of early discharge of
maternity patients: Does hospital specialization matter?
Forum for Health Economics & Policy. Health Econmics,
11(2). http://www.bep ress.om/f he p/11/2/11
[13] Staiger, D. and Stock, J.H. (1997) Instrumental variables
regression. Econometrica, 65, 557-586.
[14] Grogger, J. (1990) A simple test for exogeneity in probit
and logit, and poisson regression models. Economics
Letters, 33(4), 329-332.
[15] Hensen, L. (1982) Large sample properties of general-
ized method of moments estimators. Econometrica, 50(4),
1029-1054.
[16] Gazmararian, J.A., Koplan, J.P., Cogswell, M.E., Bailey,
C.M., Davis, N.A. and Cutler, C.M. (1997) Maternity
experiences in a managed care organization. Health Af-
fairs, 16(3), 198-208
[17] Wray, N.P., Hollingsworth, J.C., Petersen, N.J. and Aston,
C.M. (1997) Case-mix adjustment using administrative
databases: A paradigm to guide future research, Medical
Care Research and Review, 54(3), 326-356.