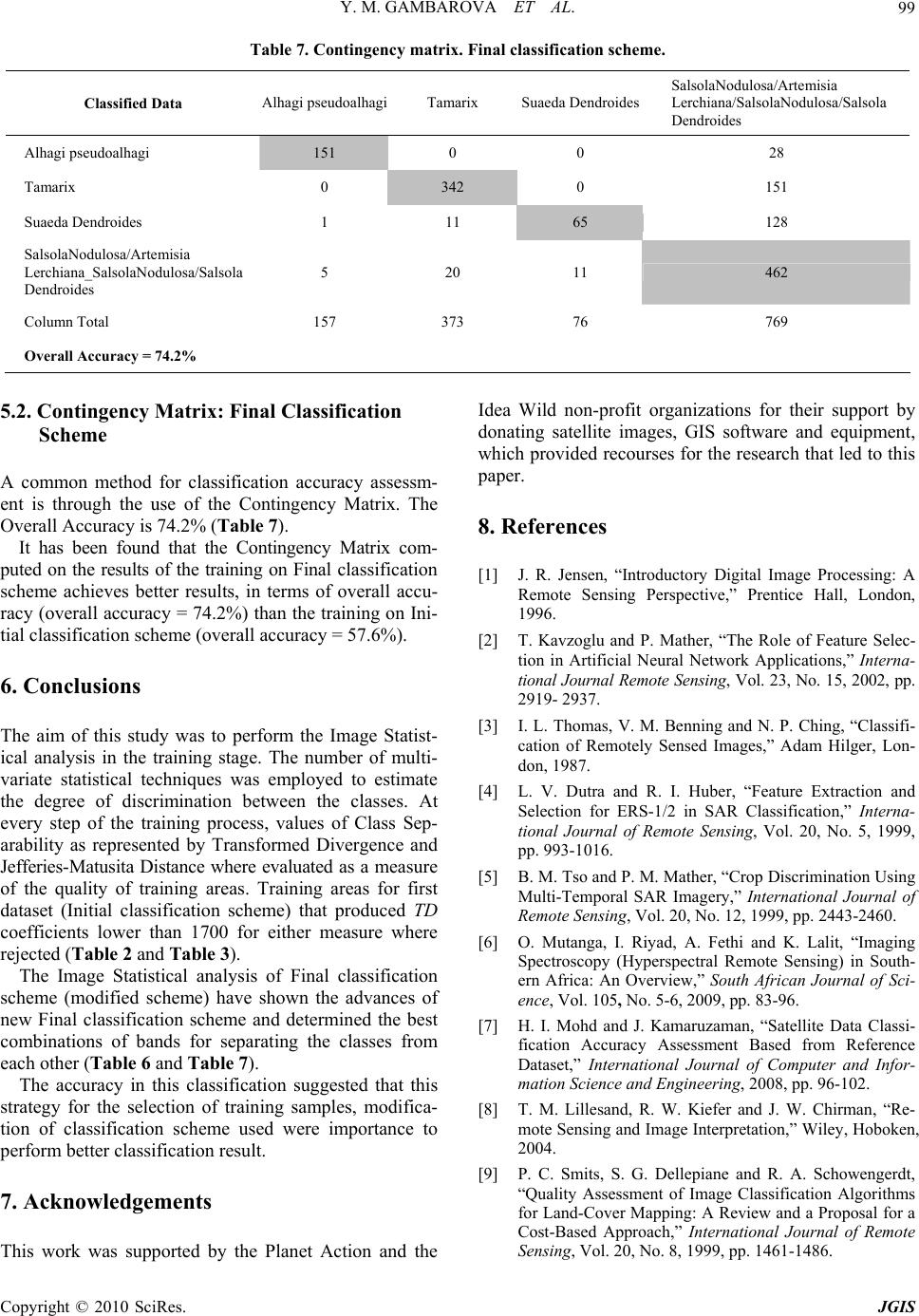
Y. M. GAMBAROVA ET AL.99
Table 7. Contingency matrix. Final classification scheme.
Classified Data Alhagi pseudoalhagiTamarix Suaeda DendroidesSalsolaNodulosa/Artemisia
Lerchiana/SalsolaNodulosa/Salsola
Dendroides
Alhagi pseudoalhagi 151 0 0 28
Tamarix 0 342 0 151
Suaeda Dendroides 1 11 65 128
SalsolaNodulosa/Artemisia
Lerchiana_SalsolaNodulosa/Salsola
Dendroides 5 20 11 462
Column Total 157 373 76 769
Overall Accuracy = 74.2%
5.2. Contingency Matrix: Final Classification
Scheme
A common method for classification accuracy assessm-
ent is through the use of the Contingency Matrix. The
Overall Accuracy is 74.2% (Table 7).
It has been found that the Contingency Matrix com-
puted on the results of the training on Final classification
scheme achieves better results, in terms of overall accu-
racy (overall accuracy = 74.2%) than the training on Ini-
tial classification scheme (overall accuracy = 57.6%).
6. Conclusions
The aim of this study was to perform the Image Statist-
ical analysis in the training stage. The number of multi-
variate statistical techniques was employed to estimate
the degree of discrimination between the classes. At
every step of the training process, values of Class Sep-
arability as represented by Transformed Divergence and
Jefferies-Matusita Distance where evaluated as a measur e
of the quality of training areas. Training areas for first
dataset (Initial classification scheme) that produced TD
coefficients lower than 1700 for either measure where
rejected (Table 2 and Table 3).
The Image Statistical analysis of Final classification
scheme (modified scheme) have shown the advances of
new Final classification scheme and determined the best
combinations of bands for separating the classes from
each other (Table 6 and Table 7).
The accuracy in this classification suggested that this
strategy for the selection of training samples, modifica-
tion of classification scheme used were importance to
perform better classification result.
7. Acknowledgements
This work was supported by the Planet Action and the
Idea Wild non-profit organizations for their support by
donating satellite images, GIS software and equipment,
which provided recourses for the research that led to this
paper.
8. References
[1] J. R. Jensen, “Introductory Digital Image Processing: A
Remote Sensing Perspective,” Prentice Hall, London,
1996.
[2] T. Kavzoglu and P. Mather, “The Role of Feature Selec-
tion in Artificial Neural Network Applications,” Interna-
tional Journal Remote Sensi ng, Vol. 23, No. 15, 2002, pp.
2919- 2937.
[3] I. L. Thomas, V. M. Benning and N. P. Ching, “Classifi-
cation of Remotely Sensed Images,” Adam Hilger, Lon-
don, 1987.
[4] L. V. Dutra and R. I. Huber, “Feature Extraction and
Selection for ERS-1/2 in SAR Classification,” Interna-
tional Journal of Remote Sensing, Vol. 20, No. 5, 1999,
pp. 993-1016.
[5] B. M. Tso and P. M. Mather, “Crop Discrimination Using
Multi-Temporal SAR Imagery,” International Journal of
Remote Sensing, Vol. 20, No. 12, 1999, pp. 2443-2460.
[6] O. Mutanga, I. Riyad, A. Fethi and K. Lalit, “Imaging
Spectroscopy (Hyperspectral Remote Sensing) in South-
ern Africa: An Overview,” South African Journal of Sci-
ence, Vol. 105, No. 5-6, 2009, pp. 83-96.
[7] H. I. Mohd and J. Kamaruzaman, “Satellite Data Classi-
fication Accuracy Assessment Based from Reference
Dataset,” International Journal of Computer and Infor-
mation Science and Engineering, 2008, pp. 96-102.
[8] T. M. Lillesand, R. W. Kiefer and J. W. Chirman, “Re-
mote Sensing and Image Interpretation,” Wiley, Hoboken,
2004.
[9] P. C. Smits, S. G. Dellepiane and R. A. Schowengerdt,
“Quality Assessment of Image Classification Algorithms
for Land-Cover Mapping: A Review and a Proposal for a
Cost-Based Approach,” International Journal of Remote
Sensing, Vol. 20, No. 8, 1999, pp. 1461-1486.
Copyright © 2010 SciRes. JGIS