 Applied Mathematics, 2011, 2, 1546-1550 doi:10.4236/am.2011.212220 Published Online December 2011 (http://www.SciRP.org/journal/am) Copyright © 2011 SciRes. AM Test of Generating Function and Estimation of Equivalent Radius in Some Weapon Systems and Its Stochastic Simulation Famei Zheng School of Mathematical Science, Huaiyin Normal University, Huai’an, China E-mail: hysyzfm@163.com, 16032@hytc.edu.cn, hssky10@163.com Received November 16, 2011; revised December 6, 2011; accepted December 14, 2011 Abstract We discuss three-dimensional uniform distribution and its property in a sphere; give a method of assessing the tactical and technical indices of cartridge ejection uniformity in some type of weapon systems. Mean- while we obtain the test of generating function and the estimation of equivalent radius. The uniformity of distribution is tested and verified with ω2 test method on the basis of stochastic simulation example. Keywords: Uniform Distribution in a Sphere, Weapon Systems, Generating Function, Equivalent Radius, Stochastic Simulation 1. Introduction Uniform distribution is very important in the probability statistics, many scholars pay attention to it. The follow- ing questions have been explored: the estimate of inter- val length about uniform distribution in [a,b] [1,2], the estimate of regional area about two dimension uniform distribution in a rectangle [3], the estimate of cuboid volume about three dimension uniform distribution [4], the estimate of regional area about two-dimensional uni- form distribution in a circle [5,6], estimate of radius on three-dimensional uniform distribution in a sphere [7]. In addition, many scholars get useful test statistics and limit theorems [8-12]. In this paper, basing on some articles [13-18], according to t the indices of cartridge ejection uniformity in some type of weapon systems, we give the test of generating function and the estimation of equiva- lent radius by simulation example. Definition 1 [7]. If (,,) YZ is three-dimensional continuous random variable, its probability density func- tion is 3 0 3,(,,) 4π (, ,) 0(,,) , . yz G R fxyz yz G (1.1) where 2222 00 (, ,),0Gxyzxyz RR, then we call that (,,) YZ obeys uniform distribution in 2222 0 (, ,)GxyzxyzR , recorded as (X,Y,Z)~U(G). Give a transformation 0 sin cos sin sin(0,0π,0 2π) cos xr yr rR zr (1.2) The probability density function of three-dimensional r.v.(,, )R is (, ,) ( ,,)(sincos,sinsin,cos)(, , ) yz hrf rrrr (1.3) in which 0 0,0π,0 2π,rR (, ,) (, , ) yz r is Jacobi determinant of the transformation (1.2), and 2 (, ,) (, , ) sin coscos cossin sin sinsincos sinsincos cos sin0 sin xxx r xyzy yy rr zzz r rr rr r r (1.4)
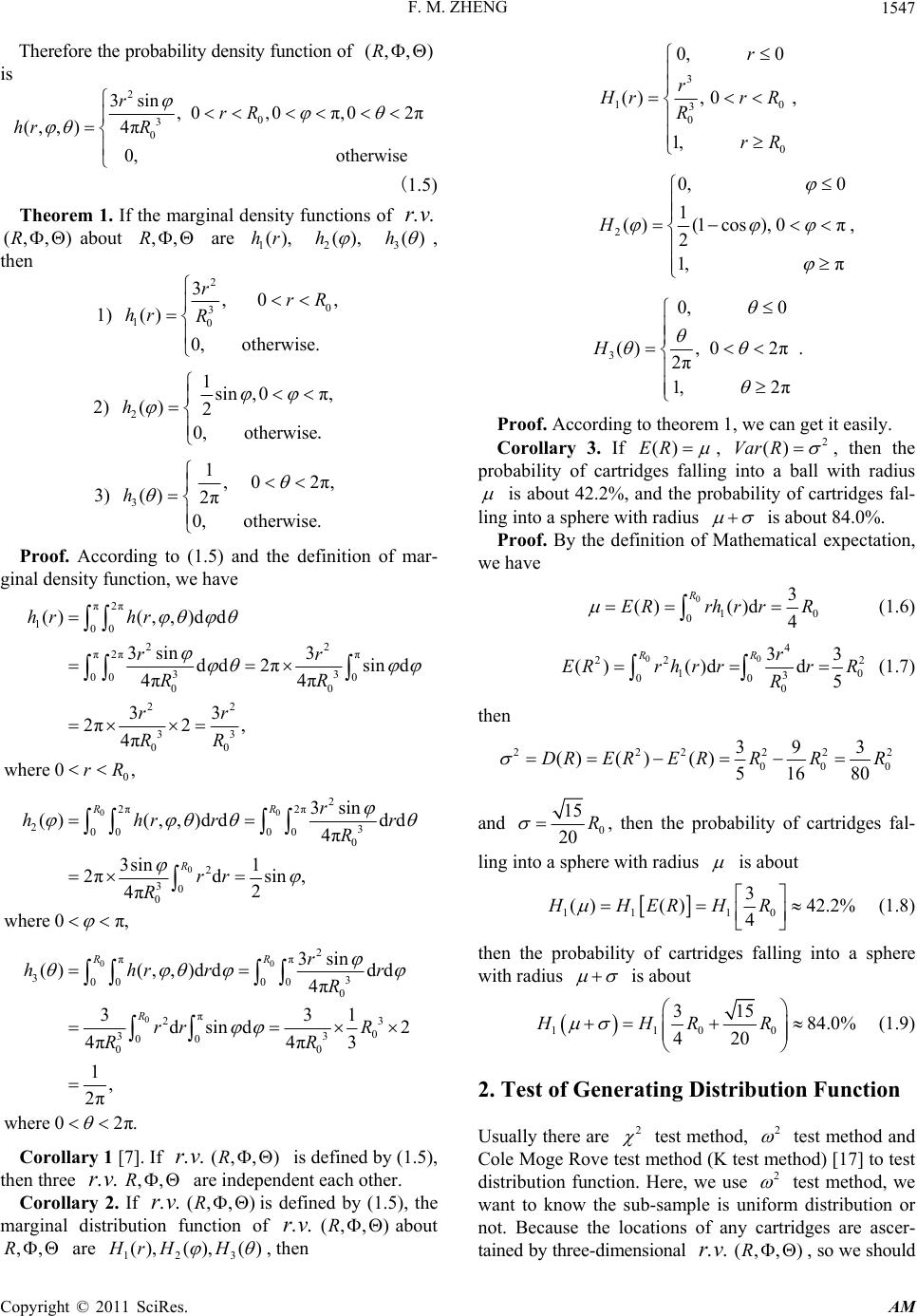 F. M. ZHENG1547 Therefore the probability density function of (, , )R is 2 0 3 0 3sin,0,0 π,0 2π (, , )4π 0, otherwise rrR hr R (1.5) Theorem 1. If the marginal density functions of about are r.v. (, , )R ,,R 1(),hr 2(),h 3()h , then 1) 2 0 3 10 3,0 () 0, otherwise. rrR hr R , 2) 2 1sin ,0π, () 2 0, otherwise h . 3) 3 1,0 2π, () 2π 0, otherwise. h Proof. According to (1.5) and the definition of mar- ginal density function, we have π2π 100 22 π2ππ 33 00 0 00 22 33 00 0 ()(,, )d d 3sin 3 dd 2πsin d 4π4π 33 2π2, 4π where 0, hr hr rr RR rr RR rR 00 0 2 2π2π 23 00 00 0 2 30 0 3sin ( )(,, )dddd 4π 3sin 1 2πdsin, 2 4π where 0π, RR R r hhrr R rr R r 00 0 2 ππ 33 00 00 0 π 23 0 33 00 00 3sin ( )(,,)dddd 4π 33 dsind 2 3 4π4π 1, 2π where 02π. RR R r hhrr R rr R RR 1 r Corolla ry 1 [7]. If r.v.(, , )R is defined by (1.5), then three are independent each other. r.v.,,R Corollary 2. If is defined by (1.5), the marginal distribution function of about are r.v. 12 (),H (, , )R 3 (),( )H r.v.(, , )R ,,R Hr , then 3 10 3 0 0 0, 0 () ,0 1, r r rr R rR R , 2 0, 0 1 ()(1 cos ),0π 2 1, π H , 3 0, 0 (),02π 2π 1,2 π H . Proof. According to theorem 1, we can get it easily. Corollary 3. If ()ER , 2 ()Var R , then the probability of cartridges falling into a ball with radius is about 42.2%, and the probability of cartridges fal- ling into a sphere with radius is about 84.0%. Proof. By the definition of Mathematical expectation, we have 0 1 0 3 ()()d 4 R ERrh rrR 0 (1.6) 00 4 22 10 3 00 0 33 ()()dd 5 RR r ERrh rrrR R 2 (1.7) then 22222 00 39 3 ()()()51680 DRERE RRRR 2 0 and 0 15 20 R , then the probability of cartridges fal- ling into a sphere with radius is about 11 10 3 () ()42.2% 4 HHERHR (1.8) then the probability of cartridges falling into a sphere with radius is about 1100 31584.0% 420 HHRR (1.9) 2. Test of Generating Distribution Function Usually there are 2 test method, 2 test method and Cole Moge Rove test method (K test method) [17] to test distribution function. Here, we use 2 test method, we want to know the sub-sample is uniform distribution or not. Because the locations of any cartridges are ascer- tained by three-dimensional , so we should r.v.(, ,)R Copyright © 2011 SciRes. AM
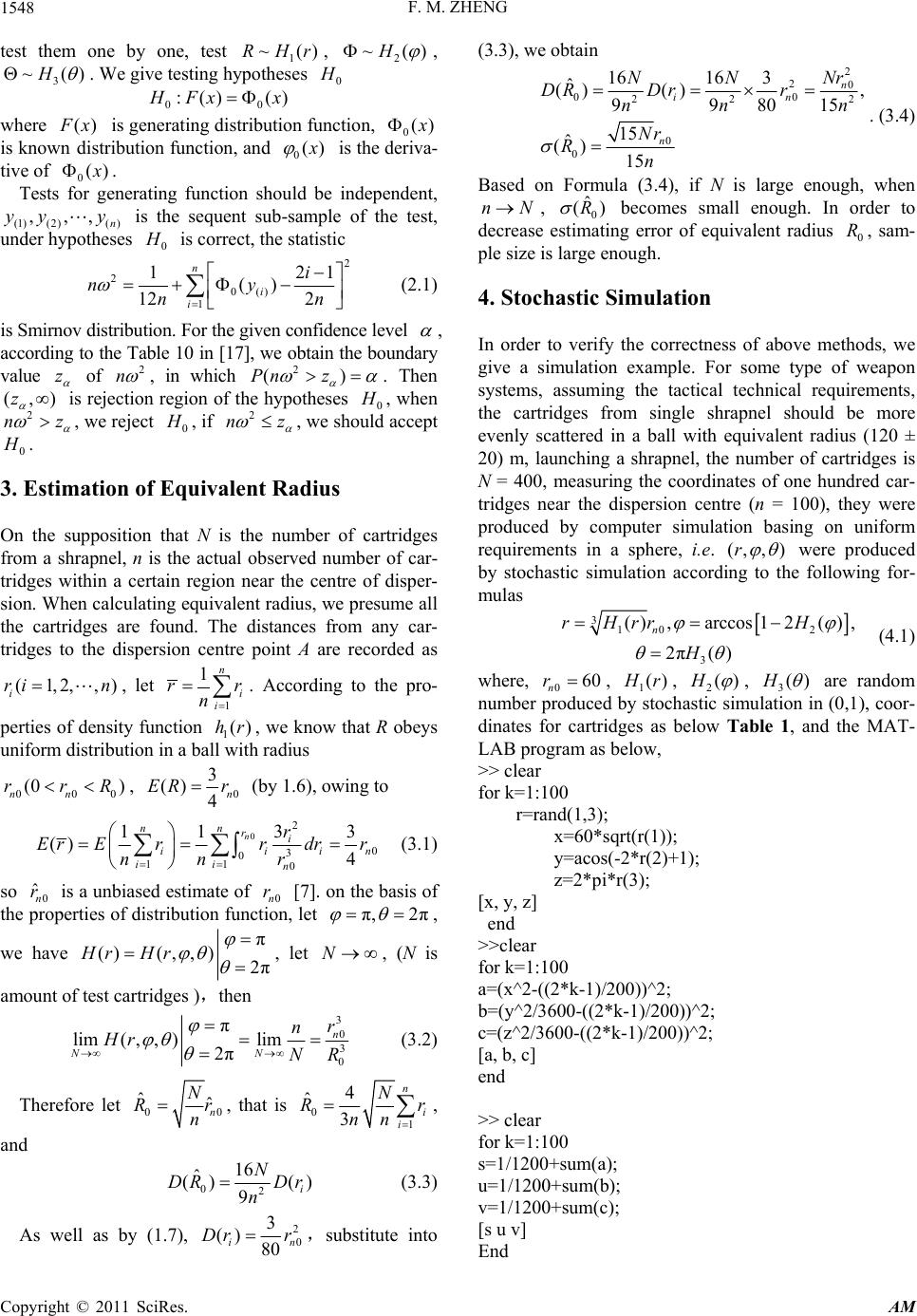 F. M. ZHENG 1548 ))test them one by one, test 1, ~(RHr 2 ~(H , 3 ~(H) . We give testing hypotheses 0 00 ():() Fx x where () x is generating distribution function, 0() is known distribution function, and 0() is the deriva- tive of 0() ,,yy . Tests for generating function should be independent, (1)(2)() is the sequent sub-sample of the test, under hypotheses , n y 0 is correct, the statistic 2 21 2 1 1 12 n i n 0( ) () 2 i i y nn (2.1) is Smirnov distribution. For the given confidence level , according to the Table 10 in [17], we obtain the boundary value z of 2 n , in which 2 (Pn ) z . Then is rejection region of the hypotheses 0 (,)z , when 2 nz , we reject 0 , if 2 nz , we should accept 0 . 3. Estimation of Equivalent Radius On the supposition that N is the number of cartridges from a shrapnel, n is the actual observed number of car- tridges within a certain region near the centre of disper- sion. When calculating equivalent radius, we presume all the cartridges are found. The distances from any car- tridges to the dispersion centre point A are recorded as (1,2, i ri,)n, let 1 1n i r ()hr i r n . According to the pro- perties of density function 1, we know that R obeys uniform distribution in a ball with radius 00 (0 nn rr 0 )R, 0 3 () 4n ER r (by 1.6), owing to 0 2 3 0 11 0 3 11 () n nn ri ii ii n r Er Errr nn r 0 3 4 in dr (3.1) so 0 is a unbiased estimate of 0n [7]. on the basis of the properties of distribution function, let ˆ n r r π,2π , we have π ()(, , )2π HrHr , let , (N is N amount of test cartridges ),then 3 0 3 0 n N r R π lim( ,,)lim 2π N n Hr N (3.2) Therefore let 0 ˆˆ n N R n 0 r , that is 0 4 3 1 ˆn i i N Rr nn , and 02 16 ˆ () () 9i N DR Dr n As well as by (1.7), (3.3) 2 0 3 () 80 in Dr rsubstitute into (3.3 , ), we obtain 2 0 16 () Nr NN DR 20 0 22 2 0 0 16 3 ˆ() , 80 99 15 15 ˆ () 15 n in n Dr r nn n Nr Rn . (3.4) Based on Formula (3.4), if N is large enough, , becomes small enough. In order to ss of above methods, we some type of weapon when nN le size 0 ˆ ()R large e decrease estimating error of equivalent radius 0 R, sam- pis nough. 4. Stochastic Simulation In order to verify the correctne ive a simulation example. For g systems, assuming the tactical technical requirements, the cartridges from single shrapnel should be more evenly scattered in a ball with equivalent radius (120 ± 20) m, launching a shrapnel, the number of cartridges is N = 400, measuring the coordinates of one hundred car- tridges near the dispersion centre (n = 100), they were produced by computer simulation basing on uniform requirements in a sphere, i.e. (, , )r were produced by stochastic simulation according to the following for- mulas 310 2 (),arccos12( ), n rHrr H 3 2π()H (4.1) where, 060 n r , 1() r, 2()H , 3()H are number (0,1 for cartres asow Table 1, a *sqrt(r(1)); 2*r(2)+1); 0 (2*k-1)/200))^2; ((2*k-1)/200))^2; )^2; :100 sum(a); m(b); random ), coor-produced by stochastic simulation in dinates idg belnd the MAT- LAB program as below, >> clear for k=1:100 nd(1,3); r=ra x=60 y=acos(- z=2*pi*r(3); [x, y, z] end >>clear 1:10for k= a=(x^2-( b=(y^2/3600- c=(z^2/3600-((2*k-1)/200) [a, b, c] end ar >> cle or k=1f s=1/1200+ u=1/1200+su v=1/1200+sum(c); [s u v] End Copyright © 2011 SciRes. AM
 F. M. ZHENG Copyright © 2011 SciRes. AM 1549 Table 1. Polar coordinates points of fall for cartridges. (, ,rm rad rad )(,, )rm rad rad (,, )rm rad rad (, ,rm rad rad ) 57.8262 1.1283 0.9007 1.7096 0.04390.4464 5.7960 0.6985 5.0936 56.0811 55.2025 47.5996 58.8251 1.3574 1.8961 1.4923 1.45132.1067 5.1923 2.1410 3.8108 33.9503 43.7655 33.0668 41.6750 1.5768 5.1315 19.1279 1.4612 4.8952 45.69072.4810 0.7106 48.3936 1.3183 6.2367 45.5454 2.0309 6.1921 54.1207 0.6013 1.9308 47.07931.1287 5.1026 51.7387 2.2742 2.3455 46.6895 1.1728 0.1093 48.7537 1.4576 5.8226 50.83271.0500 5.7070 15.1818 0.4352 3.3389 31.8301 0.6831 5.1484 39.0650 1.3001 4.2644 33.62452.6167 0.9827 56.5426 1.7679 1.1391 30.8478 48.6323 1. 1.4572 3. 5043 9025 3.5198 14. 43.0160 0717 2. 1.1647 0. 3512 4668 7. 0.4442 55. 5595 0. 4707 7407 0. 2.6500 7672 4.7922 53. 55.7802 2184 1. 2.6869 1347 3.1535 2.6528 53.9209 0.2431 1.5331 57.6967 2.1165 0.0748 48.04991.9865 4.5352 46.3803 2.4616 4.1494 44.1604 1.9052 5.1648 57.1905 2.6896 1.4275 35.84382.3398 4.0941 26.1303 0.6488 4.2330 42.6194 2.0355 1.6537 38.0081 1.6869 3.2440 28.16160.9503 4.7375 56.1754 0.5168 6.0149 39.4989 1. 57.2477 1. 1188 0742 4.7350 49. 4.1444 32. 7335 0. 5254 1. 2389 7644 2.8790 32. 4.4183 44. 3887 4830 1.4809 0.5776 4.1670 34. 5.5512 45. 6740 1. 8214 2. 0105 6183 1.2057 0.6987 51.3376 2.0009 1.3452 50.4480 2.2554 3.6600 44.49392.3493 1.7103 14.1080 0.5078 3.5506 37.3488 2.1746 3.7831 41.5086 2.8378 3.1994 22.49511.6951 2.6352 40.5506 1.0797 6.0897 59.5588 2.9061 3.8007 52.2026 0.9810 0.4668 58.81271.2010 1.3383 57.3967 3.0969 0.1489 51.7199 1. 36.7509 2. 5174 5075 4.1438 57. 1.1523 49. 1376 1. 6781 1. 9904 6150 1.2139 31. 2.3851 43. 8798 6260 1.3179 2.3973 0.2237 56. 0.5102 41. 5065 0. 5920 1. 9570 5676 5.4676 0.1690 52.7956 1.4728 3.9992 59.6074 2.6175 1.7367 40.65541.3123 5.3445 57.5123 1.1385 3.2641 52.3921 2.2256 1.0700 55.5081 2.0117 4.8437 33.13990.5499 2.1375 46.9686 1.9237 1.2083 30.7568 2.2887 3.3904 32.0629 0.9956 1.9723 57.85630.9268 2.9292 49.4790 2.7288 4.4969 16.9386 0. 38.4027 1. 8401 3570 3.9169 56. 4.3096 34. 4546 1. 6080 0. 4698 8558 4.0099 41. 6.1990 54. 1545 1232 0.4487 1.7046 5.7416 51. 1.4363 52. 0491 2. 2896 1. 1332 9094 1.5752 5.8679 29.3040 1.6124 4.2556 51.6795 2.7865 3.1598 44.60630.7133 5.4161 51.0684 0.7404 0.8621 24.6450 2.0222 5.5091 52.4759 1.2780 5.9546 44.20101.6150 4.1255 52.8935 0.6280 3.2773 in d s i aper, using above MATLAB program, we obtain Take conspicuous level Accordg to the data in Table 1 anmethodn this p 222 0.0888, 0.0425,0.0749 r nnn 00 ˆˆ 117.4909, ()3.0984RR 10% , seeing the Table in [17], we obtain the boue 10 ndary valu0.3472z 2 for n . Because 2 r n , 2 n , 2 n are less than 0.3472, so we consider that cartridges obeys uniftion sphere. 5. Referen orm distribu in a ces nterval Estimate of the Interval Length on Uniform Distribution,” Pure and Applied Mathematics bution,” Journal im UDi,” Mt- ics, Vol. 23, No. 4, 2007, pp. 155-159. n- i and B. Deng, “On the Testing and ensional of Ball D ensionalniform stributionCollege athema [1] G. S. Chen, “I Uni , P Vol. 22, No. 3, 2006, pp. 349-354. [2] H. B. Zhang, “The Shortest Confidence Interval of the Interval Length on Uniform Distriof Xiaogan University, Vol. 27, No. 3, 2007, pp. 52-55. [3] Z. J. Liu, “Estimate of Rectangle Region Area on Two [4] Z. J. Liu, “Estimate of Cuboid Volume on Three-Dime sional Uniform Distribution,” Statistics and Decision, No. 5, 2007, pp. 23-24. [5] Z. X. Wang, “Parameter Estimation of Two-Dimensional Uniform Distribution in a Circle,” College Mathematics, Vol. 24, No. 2, 2008, pp. 150-152. [6] W. Q. Jin, D. S. Cu Estimation of Uniform Distribution in a Circle,” Acta Armamentarii, Vol. 22, No. 4, 2001, pp. 468-472. [7] F. M. Zheng, “Estimate of Radius on Three-Dim form Distribution in a Sphere,” Mathematics in Prac- tice and Theory, Vol. 40, No. 14, 2010, pp. 166-170. [8] Y. X. Liu and P. Cheng, “Uniform Distribution PCM Test Statistic on Dimension and Sample Size Berry-Esseen Boundary and LIL,” China Science Bulletin, Vol. 43, No. 13, 2005, pp. 1452-1453. [9] S. R. Xie, “Two Types of Stay Limit Theorems of Non- Stationary Gaussian Process,” Science in China, Series A, Vol. 23, No. 4, 1993, pp. 369-376.
 F. M. ZHENG 1550 r the Number and [10] Z. S. Hu and C. Su, “Limit Theorems fo Sum of Near-Maxima for Medium Tails,” Statistics & Probability Letters, Vol. 63, No. 3, 2003, pp. 229-237. doi:10.1016/S0167-7152(03)00085-3 [11] Y. Qi, “Limit Distributions for Products of Sums,” Statis- tics & Probability Letters, Vol. 62, No. 1, 2007, pp. 93- 100. doi:10.1016/S0167-7152(02)00438-8 [12] G. Rempala and J. Wesolowski, “Asymptotics for Prod- tical Anal ucts of Sums and U-Statistics,” Electronic Communica- tions in Probability, Vol. 7, No. 7, 2002, pp. 47-54. [13] K. T. Fang, J. Q. Fan, H. Jin, et al., “Statisysis of Directional Date,” Journal of Application of Statistics and Management, Vol. 9, No. 2, 1990, pp. 59-65. [14] M. D. Troutt, W. K. Pang and S. H. Hou, “Vertical Den- sity Representation and Its Applications,” World Scien- tific Publishing Co. Pte. Ltd, Singapore, 2004. doi:10.1142/9789812562616 [15] Z. S. Wei, “Probability Theory and Mathematic tics,” Higher Education Press, al Statis- Beijing, 1983. ility Theory [16] S. M. Berman, “Extreme Sojourns of a Gaussian Process with a Point of Maximum Variance,” Probab and Related Fields, Vol. 74, 1987, pp. 113-124. doi:10.1007/BF01845642 [17] C. P. Pan and Z. J. Han, “Probability and Statistic o Weapon Test,” National D f efence Industry Press, Beijing, sidual Quadratic Sums in the Dispersion Equation 1979. [18] N. I. Sidnyaev and K. S. Andreytseva, “Independence of the Re with Noncentral χ2-Distribution,” Applied Mathematics, Vol. 2, No. 2, 2011, pp. 1303-1308. Copyright © 2011 SciRes. AM
|