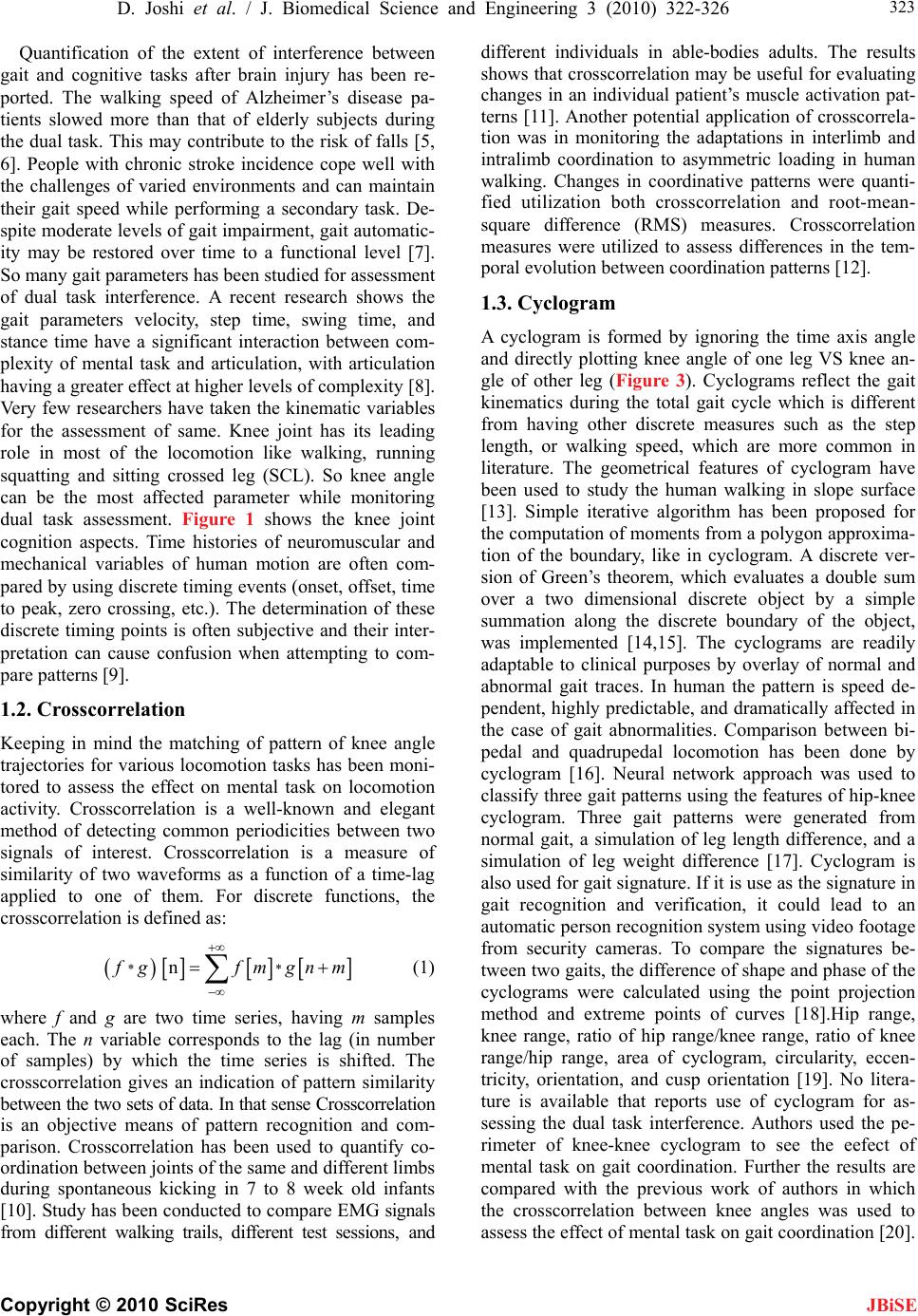
D. Joshi et al. / J. Biomedical Science and Engineering 3 (2010) 322-326
Copyright © 2010 SciRes
323
JBiSE
Quantification of the extent of interference between
gait and cognitive tasks after brain injury has been re-
ported. The walking speed of Alzheimer’s disease pa-
tients slowed more than that of elderly subjects during
the dual task. This may contribute to the risk of falls [5,
6]. People with chronic stroke incidence cope well with
the challenges of varied environments and can maintain
their gait speed while performing a secondary task. De-
spite moderate levels of gait impairment, gait automatic-
ity may be restored over time to a functional level [7].
So many gait parameters has been studied for assessment
of dual task interference. A recent research shows the
gait parameters velocity, step time, swing time, and
stance time have a significant interaction between com-
plexity of mental task and articulation, with articulation
having a greater effect at higher levels of complexity [8].
Very few researchers have taken the kinematic variables
for the assessment of same. Knee joint has its leading
role in most of the locomotion like walking, running
squatting and sitting crossed leg (SCL). So knee angle
can be the most affected parameter while monitoring
dual task assessment. Figure 1 shows the knee joint
cognition aspects. Time histories of neuromuscular and
mechanical variables of human motion are often com-
pared by using discrete timing events (onset, offset, time
to peak, zero crossing, etc.). The determination of these
discrete timing points is often subjective and their inter-
pretation can cause confusion when attempting to com-
pare patterns [9].
1.2. Crosscorrelation
Keeping in mind the matching of pattern of knee angle
trajectories for various locomotion tasks has been moni-
tored to assess the effect on mental task on locomotion
activity. Crosscorrelation is a well-known and elegant
method of detecting common periodicities between two
signals of interest. Crosscorrelation is a measure of
similarity of two waveforms as a function of a time-lag
applied to one of them. For discrete functions, the
crosscorrelation is defined as:
**n
gfmgn
m (1)
where f and g are two time series, having m samples
each. The n variable corresponds to the lag (in number
of samples) by which the time series is shifted. The
crosscorrelation gives an indication of pattern similarity
between the two sets of data. In that sense Crosscorrelation
is an objective means of pattern recognition and com-
parison. Crosscorrelation has been used to quantify co-
ordination between joints of the same and different limbs
during spontaneous kicking in 7 to 8 week old infants
[10]. Study has been conducted to compare EMG signals
from different walking trails, different test sessions, and
different individuals in able-bodies adults. The results
shows that crosscorrelation may be useful for evaluating
changes in an individual patient’s muscle activation pat-
terns [11]. Another potential application of crosscorrela-
tion was in monitoring the adaptations in interlimb and
intralimb coordination to asymmetric loading in human
walking. Changes in coordinative patterns were quanti-
fied utilization both crosscorrelation and root-mean-
square difference (RMS) measures. Crosscorrelation
measures were utilized to assess differences in the tem-
poral evolution between coordination patterns [12].
1.3. Cyclogram
A cyclogram is formed by ignoring the time axis angle
and directly plotting knee angle of one leg VS knee an-
gle of other leg (Figure 3). Cyclograms reflect the gait
kinematics during the total gait cycle which is different
from having other discrete measures such as the step
length, or walking speed, which are more common in
literature. The geometrical features of cyclogram have
been used to study the human walking in slope surface
[13]. Simple iterative algorithm has been proposed for
the computation of moments from a polygon approxima-
tion of the boundary, like in cyclogram. A discrete ver-
sion of Green’s theorem, which evaluates a double sum
over a two dimensional discrete object by a simple
summation along the discrete boundary of the object,
was implemented [14,15]. The cyclograms are readily
adaptable to clinical purposes by overlay of normal and
abnormal gait traces. In human the pattern is speed de-
pendent, highly predictable, and dramatically affected in
the case of gait abnormalities. Comparison between bi-
pedal and quadrupedal locomotion has been done by
cyclogram [16]. Neural network approach was used to
classify three gait patterns using the features of hip-knee
cyclogram. Three gait patterns were generated from
normal gait, a simulation of leg length difference, and a
simulation of leg weight difference [17]. Cyclogram is
also used for gait signature. If it is use as the signature in
gait recognition and verification, it could lead to an
automatic person recognition system using video footage
from security cameras. To compare the signatures be-
tween two gaits, the difference of shape and phase of the
cyclograms were calculated using the point projection
method and extreme points of curves [18].Hip range,
knee range, ratio of hip range/knee range, ratio of knee
range/hip range, area of cyclogram, circularity, eccen-
tricity, orientation, and cusp orientation [19]. No litera-
ture is available that reports use of cyclogram for as-
sessing the dual task interference. Authors used the pe-
rimeter of knee-knee cyclogram to see the eefect of
mental task on gait coordination. Further the results are
compared with the previous work of authors in which
the crosscorrelation between knee angles was used to
assess the effect of mental task on gait coordination [20].